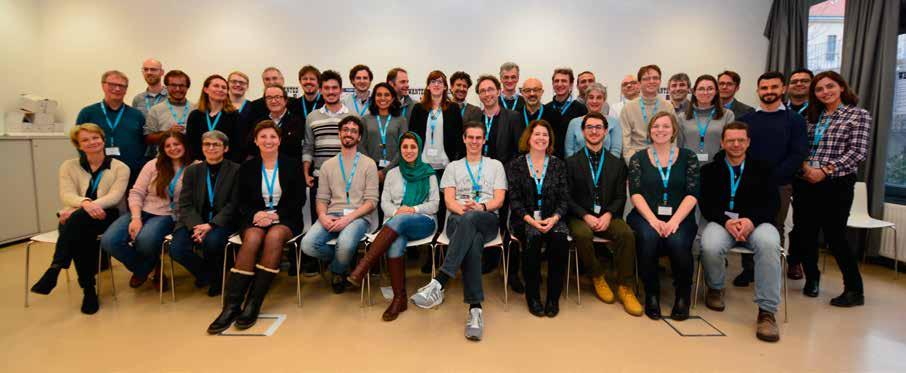
12 minute read
HYBRID
Diagnostic imaging is an important tool in modern medicine, but currently the different medical imaging modalities are often not being used to their full potential. The HYBRID project is an innovative training network (ITN), funded by the European Commission, that aims to train the next generation of imaging scientists and help them develop novel diagnostic procedures, as Professor Thomas Beyer and Dr Ivo Rausch explain.
Hybrid imaging
The emergence of hybrid medical imaging systems (see box) promises to enable more precise diagnosis and treatment of disease. In the HYBRID project, the aim is to provide training to the next generation of imaging scientists and help bridge the gap between different areas of medical imaging. “In the past, there has typically been a divide between nuclear medicine imaging and radiology,” says Dr Ivo Rausch, a post-doc researcher in the Quantitative Imaging and Medical Physics team at the Centre for Medical Physics and Biomedical Engineering (CMPBMT) at the Medical University of Vienna. “You may be a specialist in nuclear medicine imaging, or MRI, or general radiology, but there is a lack of people who understand all the different imaging modalities and are able to bring this together.” By training young scientists in different imaging areas, they can then combine the best aspects and come up with novel diagnostic procedures, which will give clinicians a fuller picture of a patient’s condition. HYBRID itself brings together academic and industrial partners across Europe, providing a platform for early stage researchers (ESRs) to work on different projects in the field of medical imaging. There are 15 ESRs working on projects within HYBRID, with the shared goal of developing tools that enable doctors to use medical imaging systems more effectively. By developing specific, image-based biomarkers, researchers hope to improve therapeutic decisionmaking. “We always strive to develop reproducible biomarkers. Several projects within HYBRID are geared towards improving the accuracy or reproducibility of these quantitative biomarkers as derived from molecular imaging,” says Thomas Beyer, Professor of Physics of Medical Imaging at the Medical University Vienna. The main focus in HYBRID is oncology, which is a primary area of application for hybrid imaging and where most of the consortium members engage in clinically. Here, we highlight three representative projects to illustrate the aims of HYBRID (see next page). In one project, Alejanda Valladares investigates ways of mimicking tumour heterogeneity by building phantom models that can be used to validate new imaging protocols and evaluation approaches at multiple imaging centres. In a second project, Dr David Wallis and Professor Irène Buvat in Paris developed an artificial intelligence (AI)-driven approach to identify cancer lesions on PET images. This supports faster and more accurate diagnostic work-up of patients. A similar approach was taken further and translated into the clinic in a third project by Nicolò Capobianco, who used an AI-model to predict outcome in lymphoma patients. Currently this approach is being extended to other diseases and imaging protocols.
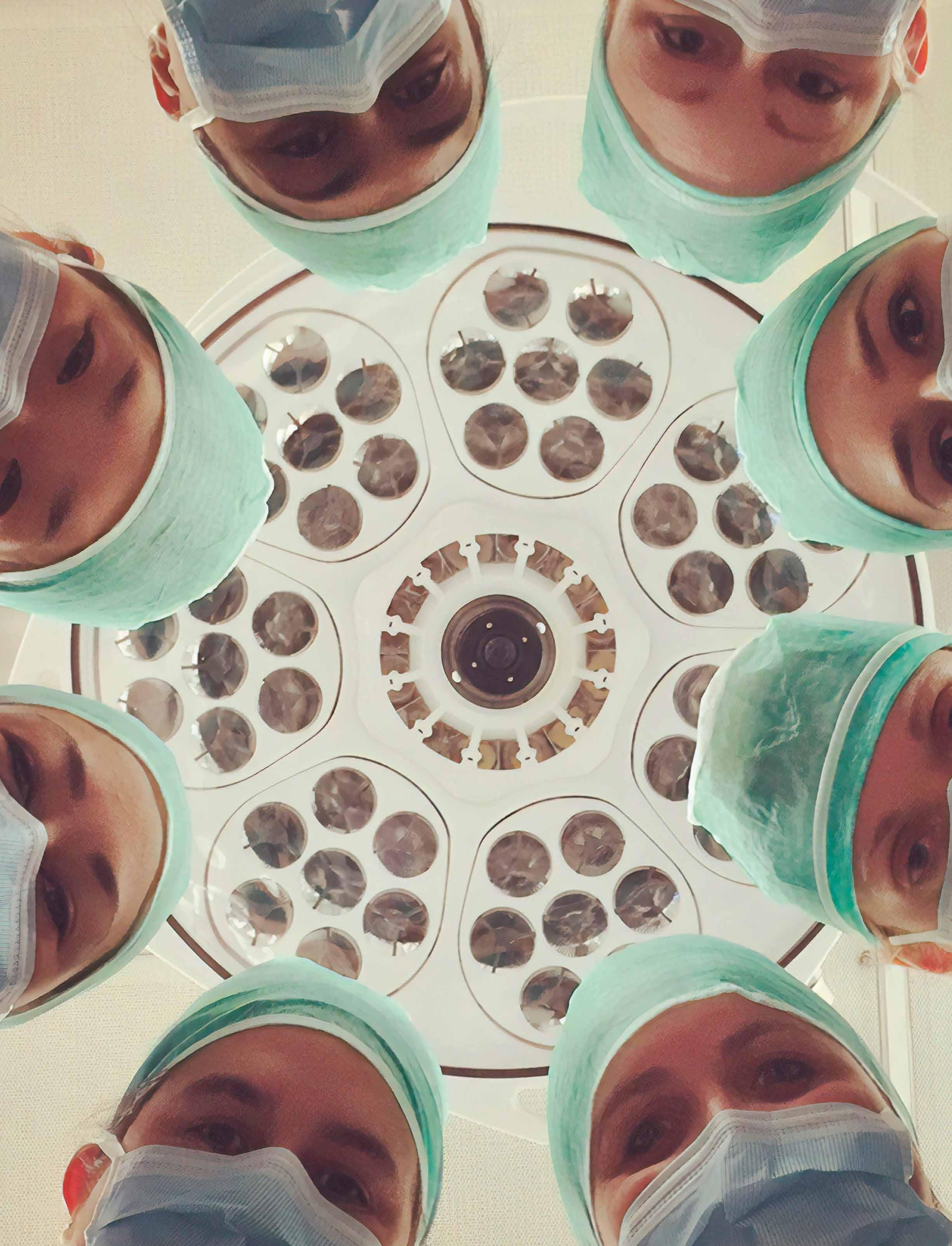
Future projects
The intention of HYBRID is not just that ESRs develop their research skills, but also that they gain experience in industrial settings, which will help prepare them for their future careers. “At the end of the HYBRID funding period, there will be 15 highly-skilled and well-trained researchers, who should be able to bridge the gap between academic research, industry and product development in the field of hybrid imaging for better patient care,” outlines Ivo Rausch. While some ESRs may choose to work in academia, others may decide to move into industry, and HYBRID has been designed to help them gain commercial experience, alongside technical skills. “For example, each ESR has a business coach that advises them on career paths in industry,” Thomas Beyer says. “The idea is that ESRs are exposed to different career options. This training programme should help them find their dream job.” The tools developed by the ESRs will be shared within the consortium, while other elements of their projects’ research could have a wider impact. For example, the bespoke imaging phantoms may become a standard for the validation of novel onsite imaging protocols and for gathering imaging studies across multiple sites. A further important outcome from HYBRID is a strong relationship between the participating institutes and industrial partners. This will provide solid foundations for further collaboration in future, believes Ivo Rausch. “Over the last couple of years, we’ve established a positive relationship between the different institutions and industrial partners, built on trust. There’s a real desire to work together in future.” The initial steps towards this are underway; with HYBRID nearing the end of its funding term, Thomas Beyer and his colleagues are in the process of establishing a new consortium. “We will build on this existing network, that has brought us sustainable and long-lasting collaborations.”
Medical Imaging
Medical imaging is a means to provide information on patients non-invasively, and help clinicians diagnose disease. A variety of different medical imaging modalities are available to assess patients, a few of which are briefly described below:
CT (Computed Tomography) – A CT scan is a type of radiology imaging, where patients are exposed to radiation from outside the body. A series of X-ray scans from different angles are combined in a CT scan to create cross-sectional images of the anatomy with high spatial resolution. SPECT (Single Photon Emission Computed Tomography) – A SPECT scan is a type of nuclear imaging, where the radiation source is inside the body. A radioisotope is delivered into the body, then a gamma camera takes images from different angles, yielding a 3-D picture of the distribution of the radioactively labeled biomolecule inside the body. PET (Positron Emission Tomography) – A radioactively labeled biomolecule (tracer) is also used with PET scans; in contrast to SPECT, PET employs positron emitting radioisotopes. These radiotracers are introduced into metabolic pathways. This technique can be used to measure changes in metabolic processes, and to identify both normal and abnormal activity. MRI (Magnetic Resonance Imaging) – With MRI, strong magnetic fields and radio waves are used to generate detailed images of a patient’s anatomy and physiological processes within the body. Hybrid imaging – Hybrid imaging denotes the physical combination of two complementary (anatomical and molecular) imaging modalities, such as PET and CT, or SPECT and CT. By combining two in one, diagnostic value can be increased and workflow can be improved.
The first meeting of the HYBRID consortium took place in March 2018 in Vienna, Austria.

Alejandra Valladares Irène Buvat David Wallis Sven Zuehlsdorff
Nicolò Capobianco
The HYBRID project is subdivided into three work packages, that each tackle a major problem with medical imaging techniques as they are currently used. The solutions proposed in these workpackages can be illustrated through the work of three ESRs within HYBRID.
Dealing with tumour heterogeneity Optimising data processing Clinical translation
EU Research:Which imaging modalities are you working with?
Alejandra Valladares: We work with
three different imaging modalities – PET, MRI and CT. In my project, I develop new imaging objects – called phantoms – which simulate some parts of the body or specific diseases. We use these phantoms to validate new techniques and imaging protocols in nuclear medicine.
EUR: Can you tell us a bit more about these phantoms?
AV: I am focused on developing physical phantoms to serve as tools for testing new imaging protocols and data analysis algorithms. The aim is to help to improve the characterisation of tumour heterogeneities. We want an object that simulates a tumour with different tracer uptake patterns in hybrid imaging.
EUR: How does a phantom simulate tumour heterogeneity?
AV: We have a standardised phantom, called the NEMA image quality phantom. This object is made of a number of homogenous spheres, simulating multiple lesions within the body. In terms of heterogeneity, we want to have different shapes and patterns inside these spheres, using different materials, which can be depicted in the three different imaging modalities. This simulates heterogeneity in lesions.
EUR: What are your plans for the future?
AV: New designs and phantom construction techniques (including 3D printing) are under evaluation. We hope that the results will allow us to create novel and standardised imaging objects for tumour heterogeneity characterisation suitable for multi-centre hybrid imaging studies. In the long-term, it would be nice to create imaging objects involving biological factors. This will give us a more realistic simulation of what is happening within a tumour than compared with the current phantoms.
EUR: What was the main focus of David Wallis’ project?
Irène Buvat: David worked on different aspects of the use of artificial intelligence in medical image analysis, in particular for automating image analysis. More precisely, his aim was to detect mediastinal cancer nodes in patients with lung cancer using PET/ CT images. He developed an algorithm that can automatically detect metastasis from the images, based on artificial intelligence.
EUR: What can these images tell you?
IB: The PET/CT images reflect what we call the radiomic phenotype – that is the characteristics of the tumours as displayed on the images. These characteristics partly reflect the biological properties of the tumour, which in turn determine whether a tumour will respond to treatment, or how the patient’s condition will evolve over time. Hence the importance of properly assessing the radiomic phenotype.
EUR: What benefits will this bring to oncologists?
IB: At the moment, oncologists mainly rely on a biopsy to determine the tumour type. But when a tumour is heterogenous, the sample may not represent the properties of the whole bulk. Medical images provide a more global perspective on tumour heterogeneity and spread than a biopsy, and in combination with AI, medical readers can render a diagnosis faster and more accurately.
EUR: How will David’s work help enable more personalised treatment of different forms of cancer?
IB: There are two main aspects of David’s work. First, he tried to automate some tasks, such as the detection of cancer nodes in lung cancer patients, for a more accurate radiomic phenotyping. Secondly, he worked to design models to predict the tumour type. He investigated how these models worked and what information they used. David tried to understand the basis of decisions made by the algorithms - what we call explainable AI.
EUR: What is the main goal in your project?
Nicolò Capobianco: I focused on
developing methods to analyse medical images, to obtain clinically relevant measurements. In my project I developed methods which would support physicians in automatically evaluating measurements such as the total tumour volume from an image. The total tumour volume is an indicator of the disease severity and may support clinicians in identifying high-risk patients who might benefit from more intensive treatments.
EUR: What were the main tasks in your research?
NC: One of the tasks I worked on is in automating the identification and segmentation of tumours from images. My project is about segmenting what is the tumour - and what isn’t - in the body. The overall idea is to automate the identification and classification of these tumours, but this is a challenging task. These tumours are identified by the increased uptake of the tracer in a PET image, but this is not a unique feature of the tumour. Therefore, I’ve been working with machine-learning, deeplearning methods, which have the distinctive ability to capture complex patterns.
EUR: What results have you gained so far?
NC: One important result involved tumour volume estimation in lymphoma subjects. Previously my colleagues at Siemens developed an algorithm which can automatically identify regions which look suspicious for tumours in PET/CT images of the whole body. We applied this method in 300 subjects – and we showed that this method can be used to automatically estimate the total tumour volume. Our other main result relates to prostate cancer, in particular hybrid images obtained by PET/CT, with a new tracer that has been developed. We worked with these deep learning algorithms to automatically identify and classify the tumours on these images. We were able to use these methods to label tumours, whether they are for example in lymph nodes, in bone, or in other organs.
AI-driven detection of tumour lesions on FDG-PET/CT images of lung cancer patients.
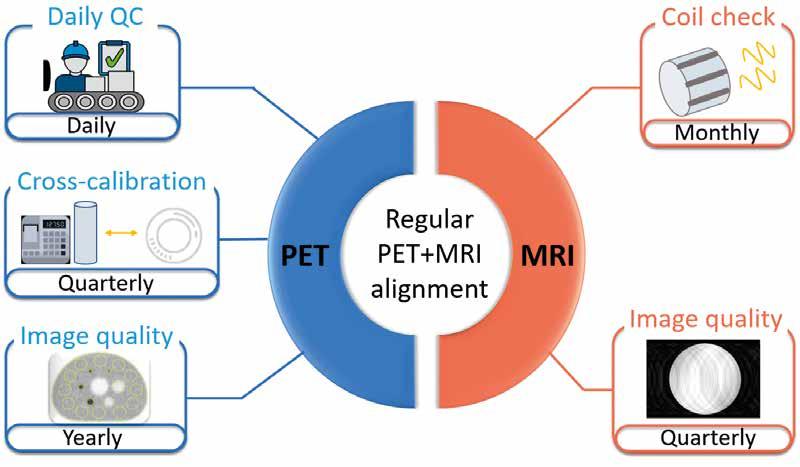
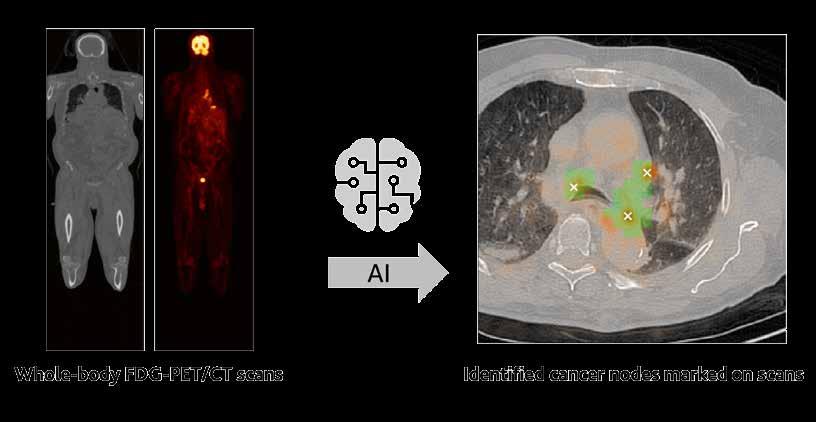
At the end of the HYBRID funding period, 15 highly-skilled and well-trained researchers will be around to help bridge the gap between academic research, industry and product development in the field of translational hybrid imaging for better patient care.
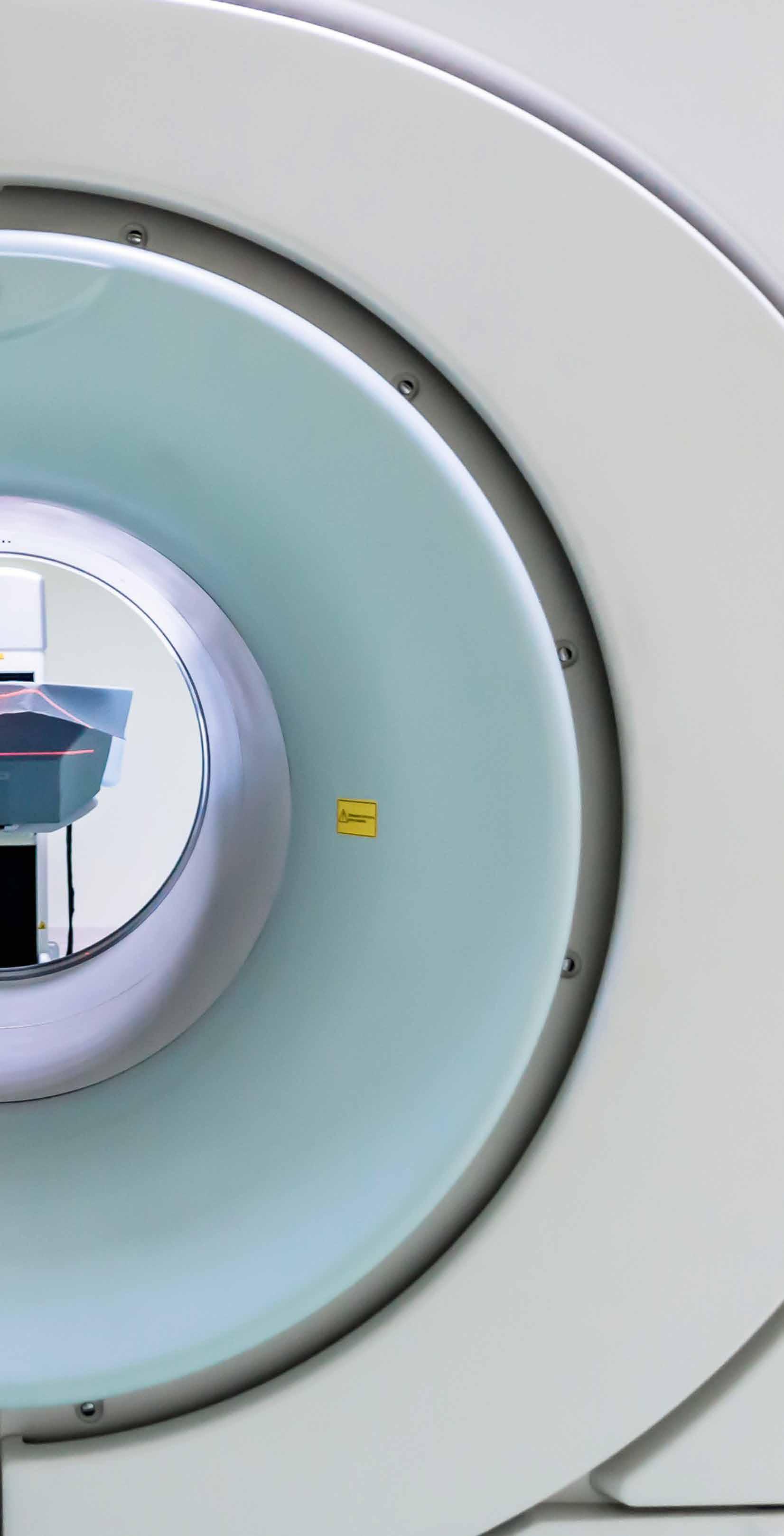
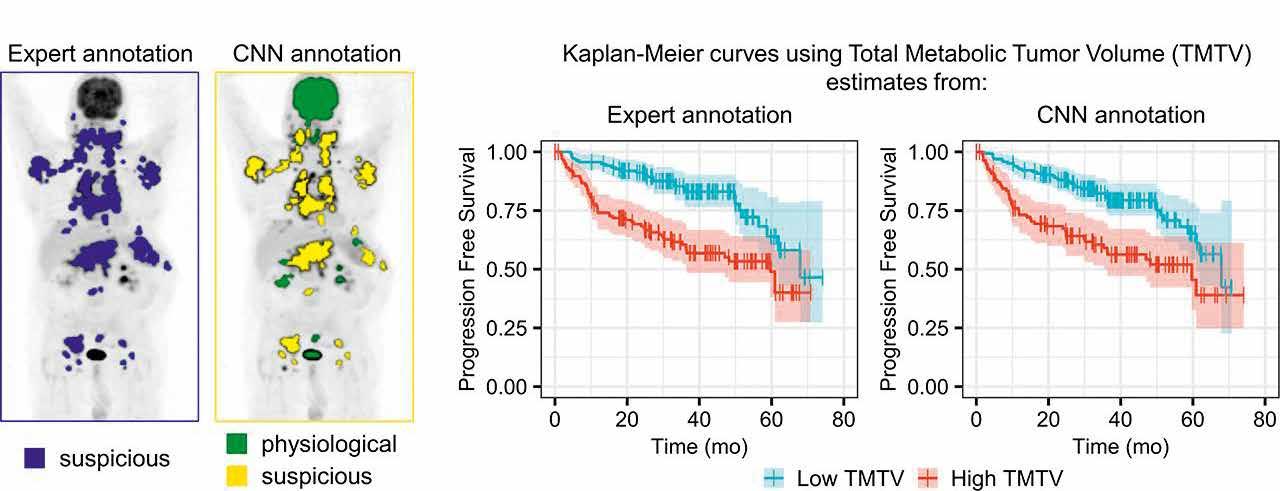
AI-driven tumour burden estimation in PET/CT scans of lymphoma patients using a convolutional neural network (CNN). The two graphs on the right show a significantly faster disease progression for patients with high total tumor volume, both when measured manually by a physician (left) and when estimated automatically with a CNN. This research was originally published in JNM. Nicolò Capobianco, Michel Meignan, Anne-Ségolène Cottereau, Laetitia Vercellino, Ludovic Sibille, Bruce Spottiswoode, Sven Zuehlsdorff, Olivier Casasnovas, Catherine Thieblemont and Irène Buvat Deep learning FDG uptake classification enables total metabolic tumor volume estimation in diffuse large B-cell lymphoma. J Nucl Med. 2021; 62 (1) 30-36. © SNMMI. https://jnm.snmjournals.org/content/62/1/30
Information for project interviews opposite: Project 1: ‘Standardized quality assurance protocols in multi-parametric imaging’ ESR: Alejandra Valladares, PI: Thomas Beyer, Co-PI: Ivo Rausch / Medical University of Vienna, Austria https://www.hybrid2020.eu/esr1.html Project 2: ‘Tumour ID cards for multi-scale characterization of tumour heterogeneity’ ESR: David Wallis, PI: Irène Buvat / Institut Curie, Paris, France https://www.hybrid2020.eu/esr9.html Project 3: ‘Improving staging through machine learning based on multiparametric imaging: a collaborative platform’ ESR: Nicolò Capobianco, PI: Sven Zuehlsdorff / Siemens Healthcare GmbH, Erlangen, Germany https://www.hybrid2020.eu/esr12.html
Healthcare Yearns for Bright Researchers of Imaging Data Project Objectives
HYBRID aims to establish the field of noninvasive disease characterization beyond the narrower vision of radiomics. Specifically, we seek to train a new generation of imaging specialists able to adopt a more inclusive approach to image analysis, that considers anatomical, functional and hybrid imaging, together with related clinical non-imaging parameters that are extracted from patients during their work-up.
Project Funding
This project has received funding from the European Union’s Horizon 2020 research and innovation programme under the Marie Skłodowska-Curie grant agreement No. 764458.
Project Partners
For full partner information, please visit: • https://www.hybrid2020.eu/partner.html
Contact Details
Thomas Beyer (scientific coordinator) Medical University Vienna QIMP Team, Center for Medical Physics and Biomedical Engineering General Hospital Vienna, 4L, Waehringer Guertel 18-20 1090 Vienna, Austria T: +43 1 40400 39890 E: thomas.beyer@meduniwien.ac.at W: https://www.hybrid2020.eu/home.html W: https://cordis.europa.eu/project/id/764458
Thomas Beyer Ivo Rausch
Thomas Beyer is a Professor at the Centre for Medical Physics and Biomedical Engineering (CMPBME), part of the Medical University of Vienna (MUV). He holds a PhD in Physics from the University of Surrey/UK and an MBA in Innovation/ Entrepreneurship from WU Vienna/AT. He is the co-developer of the first combined PET/CT system worldwide. Ivo Rausch is senior scientist in the Quantitative Imaging and Medical Physics Team at the CMPBME at MUV. His research interests relate to quantitative hybrid imaging in nuclear medicine with a special focus on PET/MRI and PET/CT.