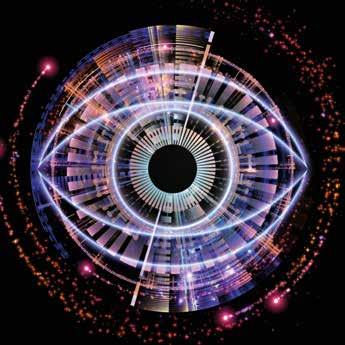
4 minute read
AI can improve the
AI and corneal diseases
Technology holds promise as diagnostic and drug development tool. Cheryl Guttman Krader reports
While retinal disease has led the way in the application of artificial intelligence (AI) in ophthalmology, there are also many opportunities for using AI technology to improve the evaluation and management of anterior segment diseases, said Jodhbir S Mehta MD, PhD. Speaking at the ESCRS 2020 Virtual meeting, Dr Mehta focused on AI applications for corneal dystrophies.
“Artificial intelligence is a platform technology that can be employed for multiple purposes in any field where there is a large dataset and good images. The promise of AI is from its capability to see things that we cannot interpret clinically,” said Dr Mehta, Distinguished Professor of Clinical Innovation, Singapore National Eye Centre, Singapore.
MULTIPLE USES Dr Mehta reported that his research group has developed and validated AI software for segmenting granular lesions in images from individuals with granular corneal dystrophy type 2.
“We know that the transforming growth factor β-induced (TGFBI) protein dystrophies are cumulative disorders in which the mutant TGFBI protein aggregates and accumulates in the cornea with time. However, patients with the same genotype can present with different phenotypes because the protein aggregates can develop in a different form depending on ethnicity, age at presentation, and other factors. In addition, patients with different genotypes can present with the same phenotype. Furthermore, patients with a TGFBI protein dystrophy who undergo transplantation are at risk for recurrence, but the risk varies depending on the underlying mutation,” Dr Mehta explained.
He proposed that the software could be used in clinical practice as a longitudinal monitoring tool in primary patients who have been diagnosed with a TGFBI-associated corneal dystrophy, and to check for protein aggregate recurrence in patients being followed after corneal transplantation. In addition, the AI algorithm could have a role in research to evaluate the effect of novel therapies for the protein aggregation disorder, such as osmolytes, chaperones and gene therapies.
Dr Mehta reported that the AI deep learning algorithm for analysing corneal images from patients with granular corneal dystrophy type 2 was developed by binarising the images into a black background with white spots representing the aggregates. The algorithm was trained and validated using a set of 806 “ideal” slit-lamp photographs from patients with granular corneal dystrophy type 2 and an internal testing set of 201 highquality slit-lamp photographs. The software was also shown to have good reproducibility when applied to images from a smaller series of cases. Next, it was applied to an external testing set of 140 “realworld” photographs that were of poorer quality but considered representative of images that might be obtained in clinical practice. In that application, the AI algorithm outperformed human raters who undertook segmenting of the stromal lesions by hand. “As expected, the software did not perform as well when analysing the ‘real-world’ slitlamp pictures as it did with the ideal images. However, our deep learning algorithm still did much better compared to the human rater values for this external dataset,” Dr Mehta said.
GUTTAE GRADING IN EYES WITH FUCHS’ Similarly, Dr Mehta and colleagues have used AI to develop an automated tool for analysing corneal guttae in eyes with Fuchs’ endothelial corneal dystrophy [Soh YQ, et al. Am J Ophthalmol. 2020 Jul 27:S0002-9394(20)30387-1]. The algorithm’s performance was validated through comparison with a manual approach to guttae identification and shown to have good agreement with the conventional Krachmer grading scale. In addition, the reproducibility of its results was demonstrated through repeated testing in a subgroup of patients.
Explaining the clinical application of this tool, Dr Mehta suggested it has the potential to improve the outcomes of transplantation surgery.
“An improved disease grading system for eyes with Fuchs’ dystrophy that is able to identify anatomic subregions of the cornea that are most affected by dense Descemet membrane guttae could potentially help guide planning of descemetorhexis zones when performing Descemet stripping only or Descemet membrane transplantation.
FAST-TRACKING THERAPEUTIC DEVELOPMENT Dr Mehta also highlighted the power of using AI as a drug discovery platform when designing therapeutics for corneal dystrophies. He discussed published work from his group that involved a conventional approach to developing pharmacotherapy for TGFBI-corneal dystrophy [Venkatraman A, et al. J Adv Res. 2020;24:529-543.]. He reviewed the steps that were used in the drug screening process for identifying candidate compounds able to bind with high affinity to the mutant protein, beginning with the need to characterise the folding and shape of the protein itself.
“The conventional approach to drug discovery is cumbersome. In fact, according to one paper, it could take 13.8 billion years to figure out all of the possible configurations of a typical protein. Artificial intelligence with deep learning could potentially enable the search for therapeutic targets for new drugs by fast-tracking the process of protein structure and binding prediction,” he predicted.