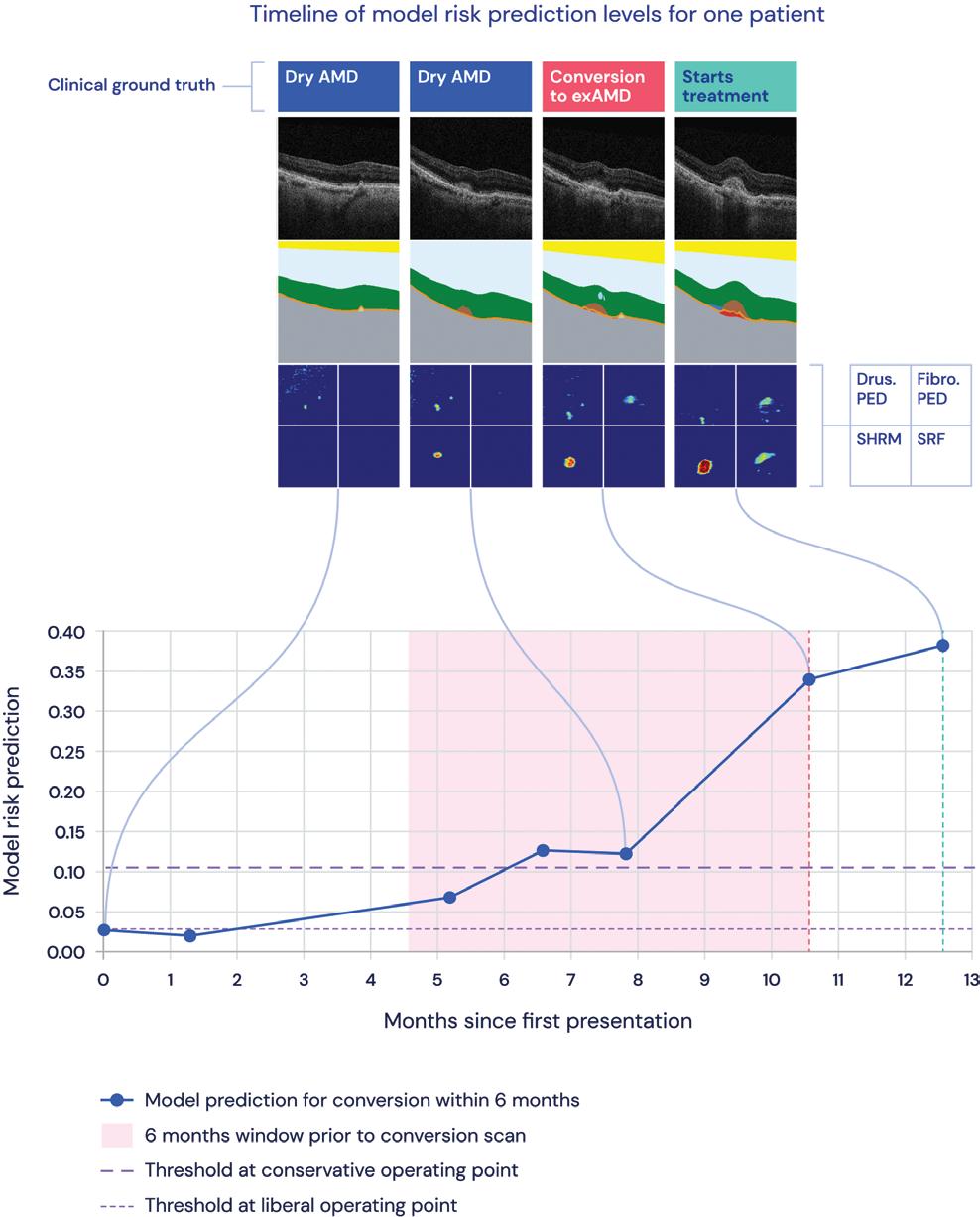
3 minute read
Using AI to predict
Using AI to predict AMD progression
AI system detects imminent conversion to exudative AMD in fellow eyes. Roibeard Ó hÉineacháin reports
Anew artificial intelligence system can predict with a high degree of accuracy the likelihood of conversion of the fellow eye in patients with unilateral exudative age-related macular degeneration (exAMD), a new study published in the journal Nature Medicine suggests.
The AI system consists of two complementary components: first predicting conversion to exAMD based on an interpretable tissue segmentation of the OCT and, second, making a prediction based on the raw OCT itself. The former adapts a two-stage architecture trained on a subset of manually segmented scans. This first segments 13 relevant tissue types and subsequently applies a classification network adapted to predict the risk of conversion to exAMD within the next six months.
The system also interprets the raw OCT Data using a twostage network with a model trained for the same task to take into account imaging features that occur in the conversion to exAWMD that are not yet captured by the segmentation model, such as reticular pseudodrusen and reflectivity of tissues.
The study’s authors trained and tested the system using a retrospective, consecutive cohort of 2,795 patients across seven different sites who were first diagnosed with exAMD between June 2012 and June 2017, achieving a per-volumetricscan sensitivity of 80% at 55% specificity, and 34% sensitivity at 90% specificity within a clinically actionable six-month time window. This level of performance corresponds to true positives in 78% and 41% of individual eyes, and false positives in 56% and 17% of individual eyes.
To compare the accuracy of the testing to human performance, three retinal specialists and three optometrists trained in medical retina were asked to predict the likelihood of fellow eye conversion to exAMD using individual and sequential scans from a randomised subset of patients. They found that system performed better than five experts (all three retinal specialists, two optometrists) and matched one (an optometrist) for the single scan task.
Furthermore, even when the experts additionally had access to each patient’s previous OCT scans, fundus images and additional clinical information, the AI system still outperformed two retinal specialists and all three optometrists an about the same as retinal specialist.
Courtesy of DeepMind
Example scans from a patient over 13 months of regular monitoring, showing raw OCT scans, the anatomical segmentations output by the AI system and a top-down view of the segmentations
“This AI system overcomes substantial interobserver variability in expert predictions, performing better than five out of six experts, and demonstrates the potential of using AI to predict disease progression,” the authors concluded.
Speaking to EuroTimes, Pearse Keane MD said: “In this paper, we use artificial intelligence (AI) to create an early warning system for ‘wet’ AMD in the ‘good’ eye of patients. In a subset of these patients, our system can predict the imminent development of wet AMD with high specificity (i.e., within a six-month time period). As a next step, we are planning how this can be used in AI-enabled clinical trials of preventative therapies.
“By treating eyes at high risk early, such trials may lead to better outcomes for patients, or even prevent development of wet AMD in the first place,” he added. The full article, “Predicting conversion to wet age-related macular degeneration using deep learning”, J Yim et al., Nat Med (2020), is available at https://www.nature.com/articles/ s41591-020-0867-7.