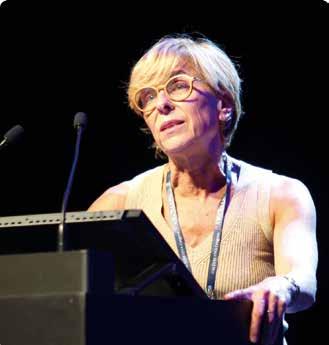
3 minute read
AI and big data hold
AI and AMD
AI’s ‘huge potential’ in AMD screening and management. Dermot McGrath reports
Artificial intelligence (AI) and big data analysis hold enormous potential to improve screening and monitoring of age-related macular degeneration (AMD) and to assist with automated detection and identification of the disease, according to Anat Loewenstein MD.
“Artificial intelligence can also help to reduce the burden of repeated visits to the clinic and provide predictability for various treatment options,” she told delegates attending the 37th Congress of the ESCRS in Paris, France.
Dr Loewenstein, Director of the Department of Ophthalmology at the Tel Aviv Medical Center, Tel Aviv, Israel, noted that the development of AI technology in the field of ophthalmology has been particularly swift and successful compared to other domains of medicine.
“Similar to radiology, ophthalmology is largely based on the visual identification of images, which lends itself well to machine learning solutions. In retinal disease, AMD and diabetic retinopathy (DR) are common diseases with elaborate imaging that allows for large training datasets to be applied,” she said.
There is an urgent need to leverage technology to help with the growing incidence of AMD worldwide, said Dr Loewenstein, noting that the introduction of anti-VEGF agents in the mid2000s radically transformed the management of neovascular AMD and greatly reduced the incidence of severe vision loss associated with the disease, she said.
With spectral domain optical coherence tomography (SD-OCT) widely available and representing the current gold standard in diagnostic imaging in the management of the most common macular diseases, the key problem for clinicians lies in processing and interpreting increasing volumes of data on a daily basis, said Dr Loewenstein.
This is where AI and machine learning applications can make a discernible difference in screening images for the development of choroidal neovascularisation and to help relieve the burden from monitoring and treatment, she said.
The potential applications of AI in AMD can be broadly divided into three categories, said Dr Loewenstein: automated classification, automated segmentation and disease prediction. In terms of classification and screening, she cited the work of Ting et al., who used almost 500,000 images from different screening initiatives to develop and evaluate a deep learning system for detection of glaucoma, AMD and DR with a specificity of over 90% for glaucoma and DR and almost 89% for AMD. Another successful example is the DeepMind AI system, which can recommend the correct referral decision – urgent, semi-urgent, routine referral or observation only – for a wide range of retinal pathologies, said Dr Loewenstein.
“Crucially, the system did not miss a single urgent case for referral in the cases of 1,000 patients, which really underscores the positive effect and potential Similar to radiology, ophthalmology is largely based on the visual identification of images Anat Loewenstein MD
Anat Loewenstein MD
for AI in screening and classification of disease,” she said.
Automated segmentation has also been successfully validated as a means to detect intraretinal fluid, subretinal fluid, drusen, blood vessels and geographic atrophy in a number of recent trials, said Dr Loewenstein.
“The performance of recent automated segmentations is comparable to that of experienced human graders,” she said.
An algorithm, Notal OCT Analyser (NOA), developed by Notal Vision with the support of Dr Loewenstein’s team in Israel, has also demonstrated its efficacy at identifying, ranking, mapping and quantifying lesion activity in the retina, she said.
Notal Vision is developing a portable OCT device designed for technician-free operation by AMD patients at home. After scanning their eyes with the device, the images are automatically uploaded to a secure server to be analysed by the NOA algorithm. If retinal fluid is detected, a report is generated by NOA and conveyed to the treating physician, helping to reduce the time from fluid onset to the next treatment. In December 2018, the device was granted breakthrough status by the FDA, and clinical studies are ongoing, including in Dr Loewenstein’s centre.
Dr Loewenstein said that such a home-based OCT device also holds rich promise in optimising emerging treatment modalities for sustained-delivery devices and long-acting formulations such as brolucizumab, faricimab, abicipar and conbercept currently undergoing phase II and phase III trials.
“With up to three months between treatments it is vital to ensure that the drug is working and treatment intervals are personalised to each patients response,” she said.