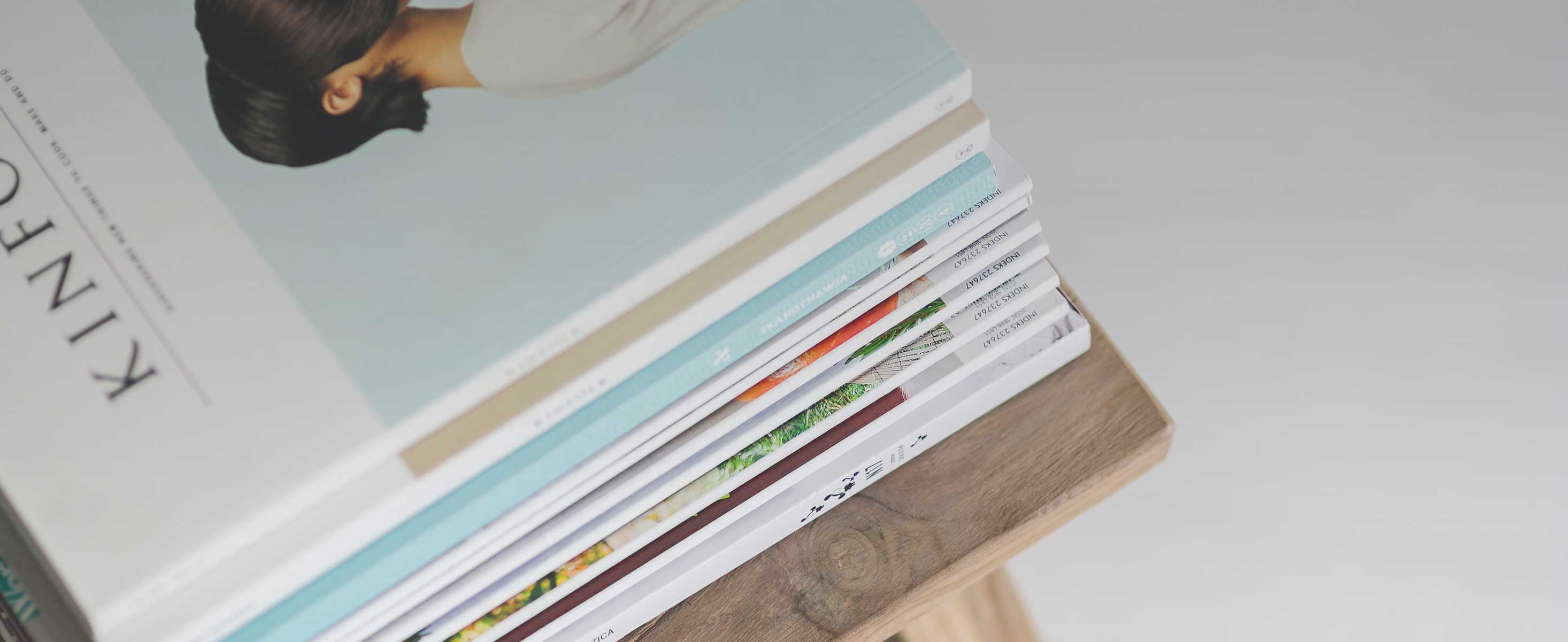
2 minute read
Scanning AI and Robotics Solutions
A robotic technician and artificial intelligence diagnostics combo shows potential to automate screening for eye disease. Roibeard Ó hÉineacháin reports
Coupling a robotically aligned optical coherence tomography (RA-OCT) system with a deep learning algorithm to classify images can enable a semiautomated approach for screening for posterior eye disease, reports Ailin (Irene) Song MD/MHSci student at Duke University School of Medicine, Durham, North Carolina, USA.
“The eye is a difficult organ to examine for non-eye doctors. We have developed a platform combining artificial intelligence and robotics to acquire and classify eye images. This is a potential platform for eye screening in settings where eye exams are otherwise challenging,” Ms Song told the ARVO 2021 Annual Meeting.
The RA-OCT system—originally developed by Drs Mark Draelos and Joseph Izatt (Duke BME) and refined by Dr Ryan McNabb— consists of a custom robotic-aligning, swept-source OCT (RA-OCT; 1040nm) system with a low mass (2.4kg) sample arm that has 70mm-diameter achromatic lenses with 3D-printed optomechanics to provide a clinically relevant 32-degree view of the retina.
When patients undergo testing with the RA-OCT system, they sit on a chair without forehead strap, chin rest, or any kind of head restraint. The system’s face- and pupil-tracking cameras triangulate the eye and pupil motion, which the robotic arm and OCT system compensate for in real-time.
To interpret the images, Ms Song and the others in Anthony Kuo’s (MD, Associate Professor of Ophthalmology at Duke University) research group used transfer learning to adapt a pre-existing convolutional neural network to train a deep learning algorithm to classify OCT images as normal or abnormal. They trained and validated their model on two publicly available OCT datasets and two of their own RA-OCT volumes. Altogether, the training and validation datasets included 87,697 OCT images, of which 59,743 were abnormal. The top-performing deep learning model had a training accuracy of 96% and a validation accuracy of 99%.
For external testing, the research group performed posterior segment imaging with the RA-OCT system in 21 patients with a broad range of retinal diseases and 22 healthy volunteers over the age of 18 at the Duke Eye Center between August and October 2020. They used the top-performing deep-learning model to distinguish between healthy and abnormal eyes.
They found that compared to clinical diagnoses, the model correctly labelled 18 out of 22 normal averaged B-scans and 18 out of 21 abnormal averaged B-scans. For pathology detection in this testing set, the model had high levels of accuracy, sensitivity, and specificity, Ms Song said.
In addition, for the correctly predicted scans, saliency maps identified the areas that contributed most to the deep learning algorithm’s predictions, which also matched the regions of greatest clinical importance, she added.
Ailin (Irene) Song: ailin.song@duke.edu
Watch the latest video content from the ESCRS and EuroTimes, FREE on the ESCRS Player
l EyeJC (ESCRS Journal Club) l Advanced Instructional Courses l Eye Contact Interviews l Video of the Month l Video Journal of Cataract, Refractive & Glaucoma Surgery l Young Ophthalmologists Videos: “My Early Surgeries”