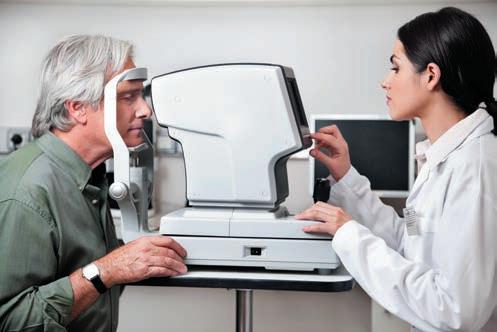
2 minute read
AI and Visual Fields
Deep learning programme beat clinical practice in detecting VF worsening. Howard Larkin reports
Adeep-learning programme developed at the Wilmer Eye Institute was more accurate in detecting worsening of visual fields in glaucoma patients than ophthalmologists evaluating the same cases in day-to-day clinical practice, a new study concludes.
In evaluating a mean of about 12 visual fields taken over about the same number of years in 871 eyes of 836 test patients, the programme correctly detected progression with a receiver operating characteristic (ROC) area under the curve (AUC) of 0.94, with a sensitivity of 0.93 and specificity of 0.99. By contrast, clinicians correctly noted progression in electronic medical records with a ROC AUC of 0.64, a sensitivity of 0.63, and specificity of 0.65.
“Overall, the deep learning model performs quite well and actually outperforms the clinicians in a non-controlled setting,” noted Jasdeep S Sabharwal MD, PhD.
CONSENSUS MEASURE The deep learning programme was trained with visual field tests performed in 6,964 eyes of 4,655 glaucoma patients seen at the Wilmer Eye Institute in Baltimore, Maryland, USA, with a mean of 11.95 fields per eye. It was validated on 870 eyes in 833 patients.
Because of low agreement among individual measures of VF progression, the study used three event-based mechanisms— AGIS, CIGTS, and GPA—and three trend-based methods—VFI slope, MD slope, and PLR slope—for training the deep learning programme. If four of the six indicators agreed, the case scored as progressing. Using four measures ensured the inclusion of at least one event-based and one trend-based measure, though trend-based measures generally show greater agreement.
“There is a lot of variation, and that is why there is difficulty finding a reference standard for these deep learning algorithms. So, as others have proposed, we used a consensus,” Dr Sabharwal explained.
The study also compared clinician assessment of progression at the last appointment, though its agreement with other measures was generally low.
The machine learning model synthesized 52 points measuring total deviation and eight global metric vectors for all visual fields of each eye to make its prediction. This data was processed using a long, short-term memory training architecture—which processes not only single data points but multiple time points in a sequence to generate a prediction.
“The output was a probability of worsening,” Dr Sabharwal said.
He foresees the programme as having direct clinical use. “Once you train the algorithm with a large set of data, it can give you a prediction for a series of visual fields it has not been trained on.”
The programme could serve as an additional piece of data with current VF printouts, Dr Sabharwal said. He currently prints out MD and VFI slopes, and GPA data, and finds them helpful in assessing cases, especially where progression is not obvious.
“Each different point helps add to it.”
The study was presented at the American Glaucoma Society 2022 annual meeting in Nashville, Tennessee, USA.
Jasdeep S Sabharwal MD, PhD is an ophthalmology resident at Wilmer Eye Institute, Johns Hopkins Medicine, Baltimore, Maryland, USA. jsab@jhmi.edu
Ready when you are.
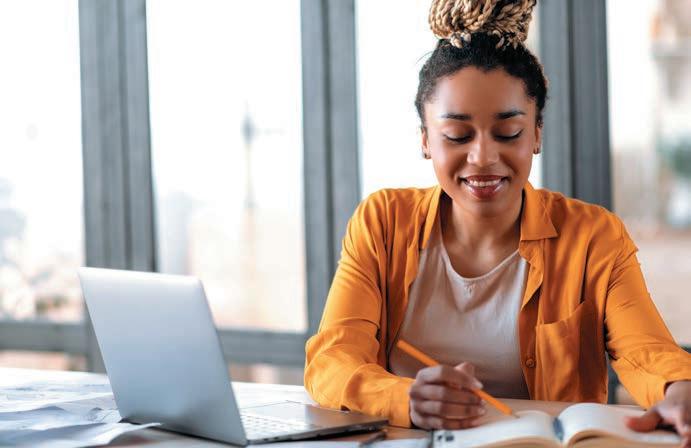
Continue your education all year with our range of online resources. Visit education.escrs.org