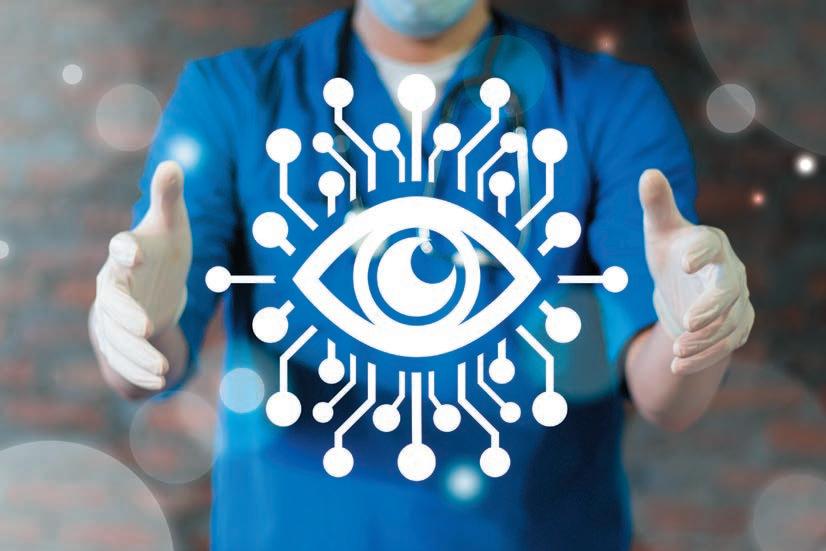
5 minute read
A Powerful New Tool in Assessing the Anterior Segment
AI giving traditional power formulae a run for the money. Roibeárd O’hÉineacháin reports
Artificial intelligence is showing increasing efficacy in diagnosing pathologies of the anterior segment of the eye and can outperform modern intraocular lens (IOL) power calculation formulae, according to Damien Gatinel MD, PhD.
“Artificial intelligence represents a fundamental change across society. It cannot be treated as a trend that will pass us by in a couple of years. Visual health is an area in which AI has some of the most promising applications due to its foundations in evidencebased, data-driven processes,” Professor Gatinel noted.
Although most of the initial research in deep learning technology in ophthalmology has focused on retinal diseases because of the widespread use of medical imaging in those conditions, recent research has revealed many potential applications in assessing the anterior segment.
Generally, AI refers to a system that uses data processing and pattern recognition to simulate cognitive functions. Machine-learning techniques can be either supervised or unsupervised. Supervised learning in ophthalmology requires experts to label individual features in clinical images to develop the AI solutions. Unsupervised learning involves using entire images without explicit labelling, and the AI itself can discover hidden patterns that may help uncover the predictive features of the pathologies under consideration.
CORNEAL DIAGNOSTICS As an illustration of supervised learning, Prof Gatinel described results from a study in which he and his associates used a convolutional neural network to develop an algorithm to distinguish between computerised corneal topography images of normal eyes from those of eyes with keratoconus and eyes that underwent previous refractive surgery. Convolutional neural networks are the current state-of-theart technology for image recognition. Inspired by biological processes, their interconnectivity patterns resemble the visual cortex.
In their study, Prof Gatinel and his team trained the network using 3,000 examinations in total—a thousand for each category. However, instead of using the coloured images, they used the actual raw numerical value constituting these maps to avoid a loss of information.
The network used the region combining the steepest curvature, the thinnest point, and the highest elevations of the anterior and posterior surface to predict keratoconus. For refractive surgery examination, the network used the region combining the flattest curvature, the thinnest point, and the highest and lowest elevations of the anterior and posterior surfaces.
In a test set of 300 Orbscans, the overall accuracy of the clustering algorithm was 99.3%. Only two examinations of the 300test set were misclassified, both of which were refractive surgery examinations. Sensitivity and specificity were 100% for KC, 100% and 99% for normal examinations, and 98% and 100% for RS examinations.i
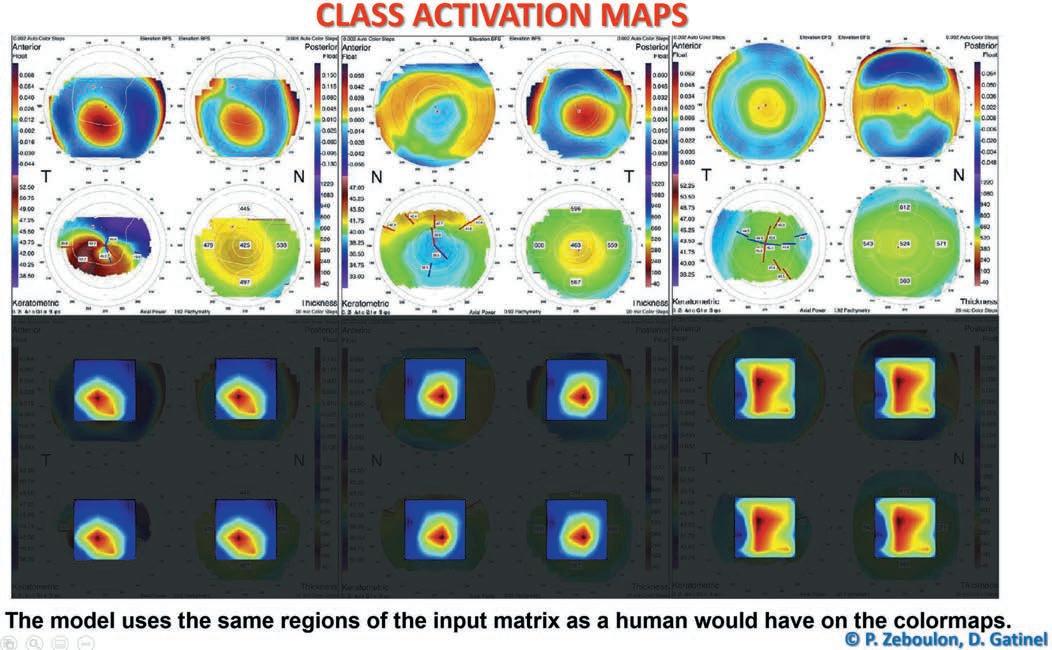
Researchers performed class activation mapping to visualise which part of the input matrix predicted each class and present graphic examples. The results are presented as a colour heatmap in which hotter colours represent the matrix elements that were the most useful to the model for making the prediction. The use of class activation mapping helps understand how the network makes its prediction. It showed that their model classified keratoconus (KC) and refractive surgery (RS) examinations by using the same regions of the input matrix as a human would on the colourmaps. The region the network used to predict the KC class combined the steepest curvature, the thinnest point, and the highest elevations of the anterior and the posterior surface. For RS examinations, the network used the region combining the flattest curvature, the thinnest point, and, respectively, the lowest and highest elevations of the anterior and posterior surface.
In another study, Prof Gatinel and his team tested the efficiency of unsupervised algorithms to extract and sort computerised corneal topography images into the same three diagnostic clusters based on data similarity with little human intervention, data cleaning, or feature selection.
The clustering algorithm analysed 7,019 usable examinations extracted from 13,705 Orbscan maps and used 248 manually labelled Orbscan maps to serve as “tracers” to help in designing the algorithm. The researchers found this method had an overall accuracy of 96.5%. The sensitivity and specificity for the three diagnostic categories were, respectively, 99.8% and 85.9% for normal eyes, 88.0% and 99.9% for keratoconus, and 93.1% for eyes that underwent corneal refractive surgery.ii
IOL POWER CALCULATION Prof Gatinel and his associates have also used an unsupervised learning AI approach to develop their Pearl-DGS IOL power calculation formula. The formula is a hybrid that relies on an optical, computational core but uses AI to determine the anatomical position of the IOL (effective lens position in a thick lens model).iii
He noted the actual physical measurement of the IOL position with OCT or other technology is not available in most data sets. Therefore, they created a formula to enable the back-calculation of the effective lens position of the principal object plane of the thick lens IOL in an operated eye, provided they had all the preoperative biometric parameters, the postoperative refraction, and the IOL’s power, geometry, and refractive index.
The results of a recently published meta-analysis, including 1,476 eyes from 14 studies, placed the Pearl-DGS first among all recent formulas in the accuracy of IOL power calculation for short eyes.iv
This reflects the prediction’s precision of the IOL position because, for these short eyes that receive high-power IOLs, the deviation from the predicted position induces a greater predictive error.
Prof Gatinel and his associates have published an open-source overview of all the details and key steps in IOL power computation with the Pearl-DGS formula.v
i P Zébulon et al. American Journal of Ophthalmology, 2020; 219: 33–39. ii P Zéboulon et al. Scientific Reports, no. 16973, https://doi.org/10.1038/ s41598-020-73902-7. iii Debellemanière G, Dubois M, Gauvin M, Wallerstein A, Brenner LF, Rampat R, Saad A, Gatinel D. “The PEARL-DGS Formula: The Development of an Open-source Machine Learning-based Thick IOL Calculation Formula”. American Journal of Ophthalmology, 2021 Dec; 232: 58–69. iv Y Luo et al. International Ophthalmology, 2022, 42(6): 1939–1956. v Gatinel et al. Translational Vision Science & Technology, 2021, 10(4): 27. doi: https://doi.org/10.1167/tvst.10.4.27.
Prof Gatinel presented his research at the Artificial Intelligence in Ophthalmology 2022 virtual meeting.
Damien Gatinel MD, PhD is head of the Anterior Segment and Refractive Surgery Department of Ophthalmology, Rothschild Foundation Hospital, Paris, France, CEROV (Centre of Expertise and Research in Optics and Vision), Paris, France. gatinel@gmail.com