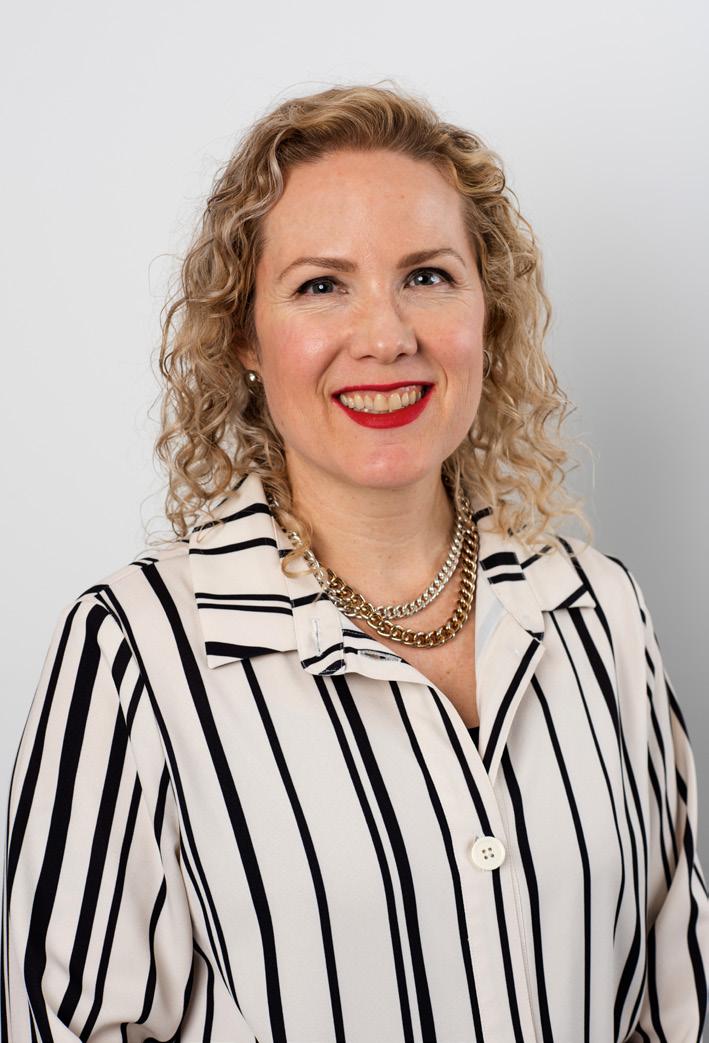
10 minute read
BANKING ON THE CHATGPT CRAZE
With announcements about new gains Generative AI models have made coming daily, the question financial institutions are left with is how to apply it safely.
For an industry that thrives on balancing risk and reward, completely ignoring this much hyped tech will not be an option forever. According to the Gartner Financial Services Business Priority Tracker Survey from February 2023, disruptors are already investing in the new technology, the biggest brands are closely monitoring the situation, and the largest group is waiting for certain improvements.
Advertisement
The wait-and-see approach seems sound with so much in flux. Starting with regulatory requirements, there’s a lot of discussion from the supra-national to industry levels about how these new generative technologies need to be regulated. With at least one country considering a complete ban and high-level technologists sounding warnings, it’s hard to know what investments might be lost if they don’t comply with coming legislation.
Even if we stick to existing laws, in the UK the FCA’s Consumer Duty regulations require explanation in decisioning and proof of compliance, neither of which are possible with today’s LLMs in charge of customer communications.
Then there’s the lingering downsides, I’ll go into a few of the big ones:
1. Hallucinations: This is the polite way to say that the responses ChatGPT and other similar predictive models generate are those of a confident liar. Even if you were to train it exclusively on your company’s data, there’s no known way to ensure it always sticks to the truth. Specific styles of prompt engineering tend to yield more truthful results, particularly after a few turns, and there may be ways to catch the bulk of hallucinations with post-processing, but there’s no known way to control the content completely on its own.
2. Black box: Because of the ways the AI trains itself, even its developers don’t always know why it’s made certain decisions or even all that it’s capable of. Equally, companies behind the big models haven’t been transparent with users about what data was used to train the models – including whether or how the data your use of it generates will be utilised. This missing information hampers risk assessment and leaves serious security questions.
3. Old news: Depending on the model, the latest information it has access to could be years old. This can lead to outdated answers and lower chances of understanding questions that incorporate new terms or ask about more recent events.
4. Lack of context: Despite their ability to communicate convincingly, these models don’t understand what they are being asked and don’t have access to the same context clues that we do, leading to confusing responses that might not be wrong per se, but that aren’t correct for the situation.
However, for all the drawbacks, the potential upsides may prove too tempting to ignore, and steps for safer implementations could reduce risk enough to make it worth it.
Content creation/adaptation for marketing is one area where generative technologies can be used in a hands-on way by people, making it safe while saving enough time and costs to be irresistible. Marketing can be customised to niche groups or completely individualised without an undue amount of effort.
This individualised approach could also be employed during onboarding to help meet regulatory requirements like the UK’s new Consumer Duty outcome of consumer understanding. Use in response creation – both to tailor the wording to the correct reading level or primary language of the individual, and to determine whether they understand the product and the implications of sign up are both valuable endeavours that aren’t feasible at scale without technology’s help. Again, use here should be as a timesaver and any responses it generates should be checked before use.
The not-quite-ready-for-prime-time tech might not be suitable for unmediated customer-facing interactions, but behind the scenes it can shine, especially in these two important areas:
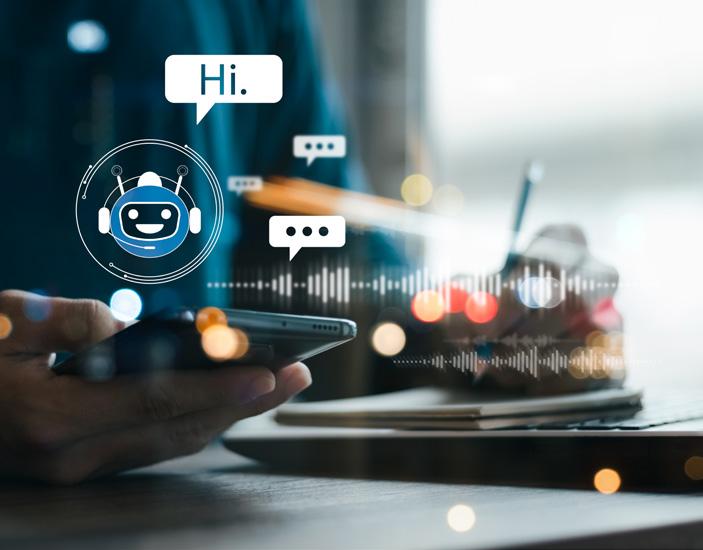
1. Fraud detection: These models have been used to determine the probability that they have been used to generate a text. In a less meta-application, they could be employed to detect other types of potential fraud.
2. Data extraction and insight: Developments in tech, including the right application of Generative AI, open a whole new realm of possibilities when it comes to extracting insight from data. Including having more data to work with. For example, when analysing old call recordings, instead of sampling only a fraction of calls manually, adding LLMs into the mix make it feasible for companies to gain full insight into what customers most frequently ask for, along with how they ask for it and the corresponding agent responses as well. By automating the bulk of categorisation and labelling work and learning the words and phrasing customers are using enables training of company NLP for better recognition. LLMs are great at figuring out alternate phrasings, to further improve recognition rates.
Whether you decide to dive right in, test the waters, or wait for the calm before investing, make sure you:
1. Explore behind-the-scenes rather than customer-facing use cases
2. Employ it alongside people to enhance their expertise or enable faster execution – don’t leave it on its own
3. Test thoroughly. Only then, can you ensure your investments are sound, make sense, and offer value without compromising security.
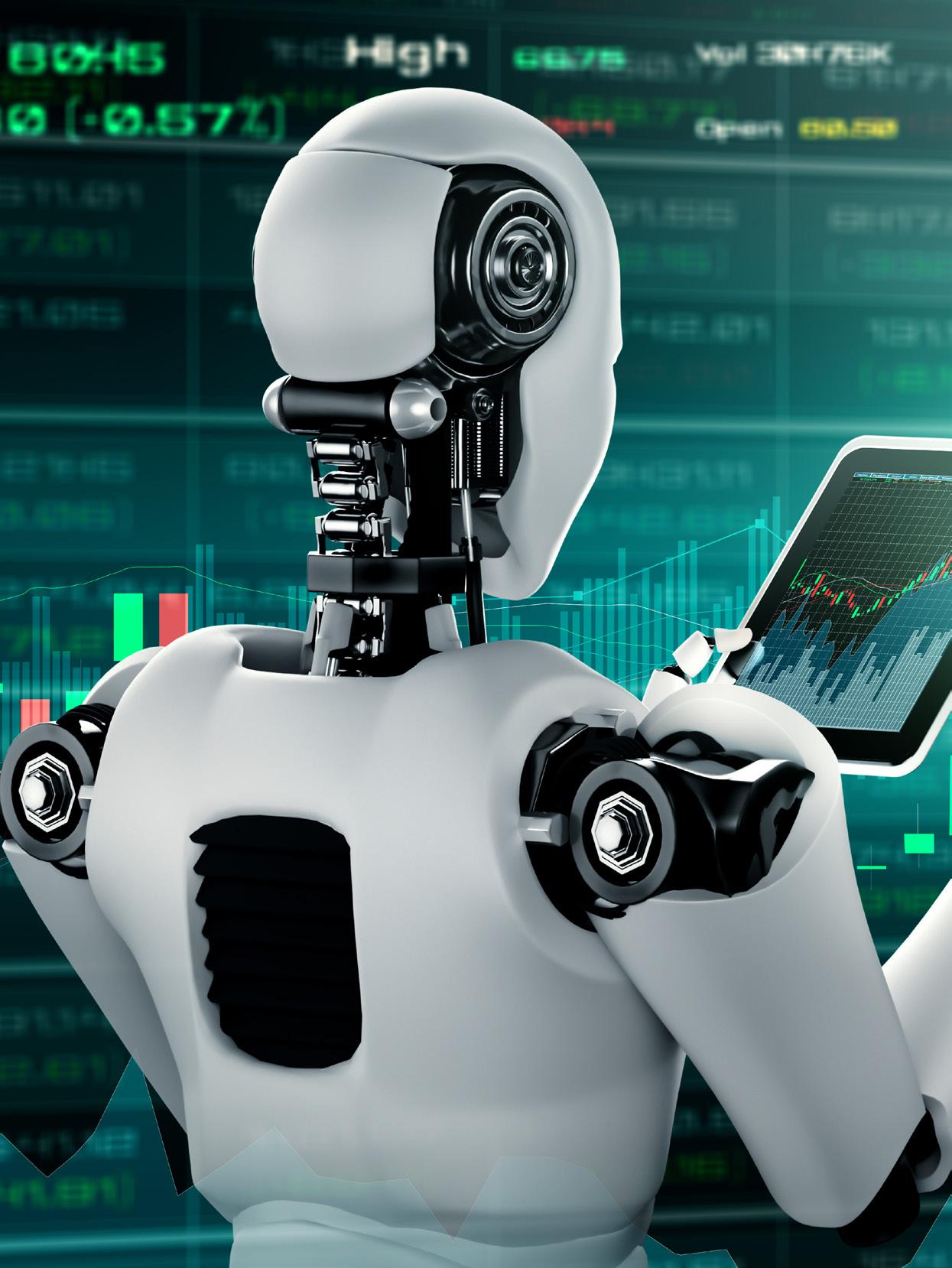
Top 5 Ai Use Cases For Quantitative Trading
In recent years, the finance industry has undergone a transformation with the advent of Artificial Intelligence (AI). Among the various areas where AI has made a significant impact, quantitative trading stands out. By utilising algorithms to analyse vast amounts of financial data and make trades based on patterns and trends, AI has revolutionised this field. While statistical methods have long been used in finance for data analysis, the growing complexity and volume of financial data have made it imperative to incorporate AI methods. These cutting-edge techniques provide more nuanced insights and a deeper understanding of the data. Here are some top AI use cases in quantitative trading that can drive higher ROI and enhance productivity.
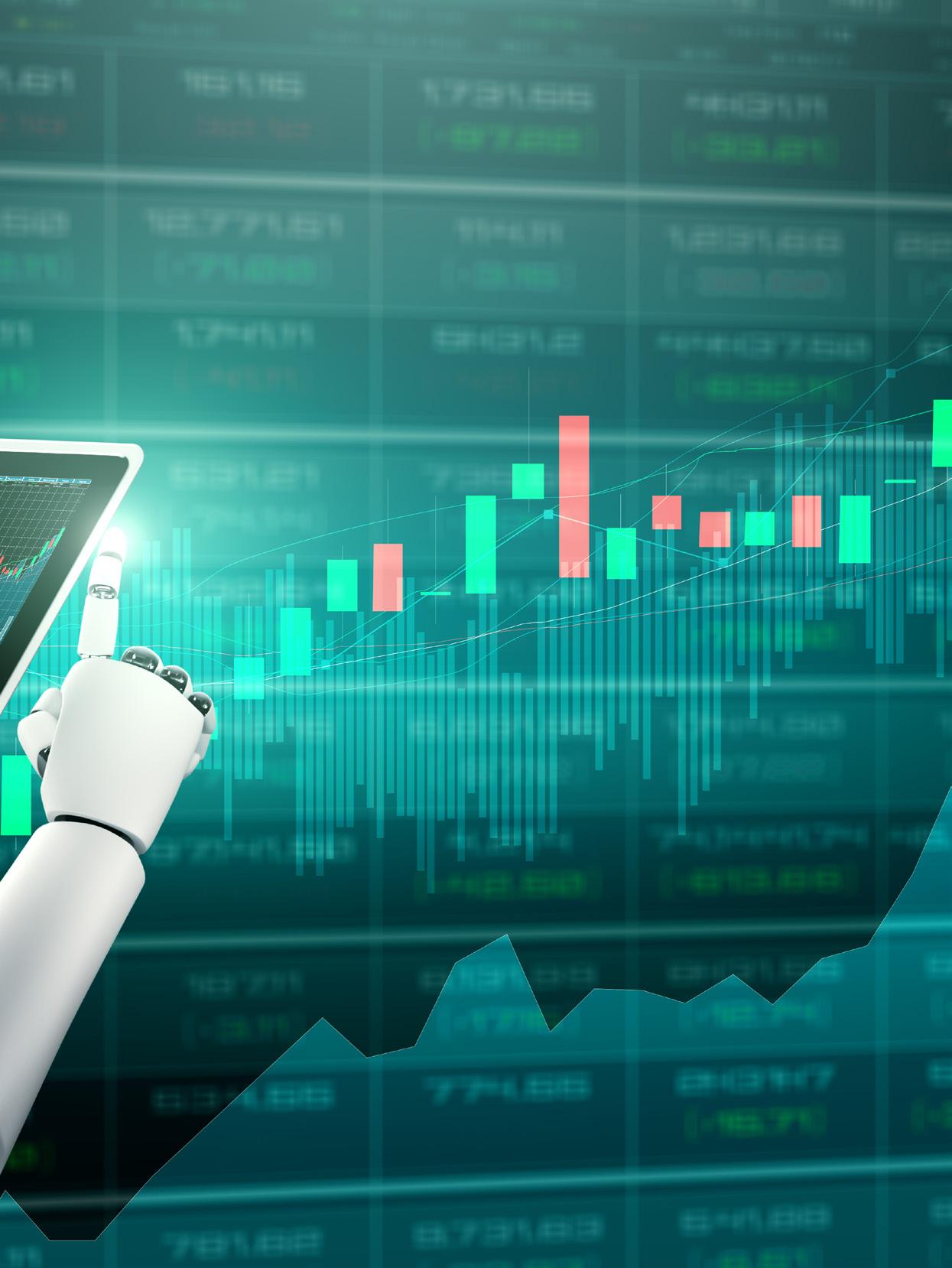
1. Financial Sentiment Analysis
Generative AI has greatly enhanced businesses' capacity to comprehend and analyse textual data, such as news and social media sentiment. Leveraging sophisticated transformer models like GPT-3, AI-powered sentiment analysis has become more precise and effective, empowering traders to make well-informed decisions based on up-to-date data in real-time.
If a trading team aims to base their trades on news and social media sentiment, they can employ AI-powered sentiment analysis to analyse various content, such as news articles and social media posts, to determine whether the sentiment is positive or negative. For instance, if the sentiment surrounding a specific company is positive, the trading team may opt to buy stock in that company. Conversely, if the sentiment is negative, they may choose to sell their holdings. By utilising AI-powered sentiment analysis, the trading team can make better-informed decisions based on real-time data.
2. Trading Pattern Recognition
Automated trading utilises AI algorithms to automatically execute trades based on predefined patterns or rules. For instance, let's say a trading team desires to make trades based on a stock's price movements between 9:30 a.m. and 10:00 a.m. daily. Using AI-powered pattern recognition, the team can identify this pattern and automatically execute trades during that time window. By automating their trading strategies, the team can save time and enhance their overall efficiency.
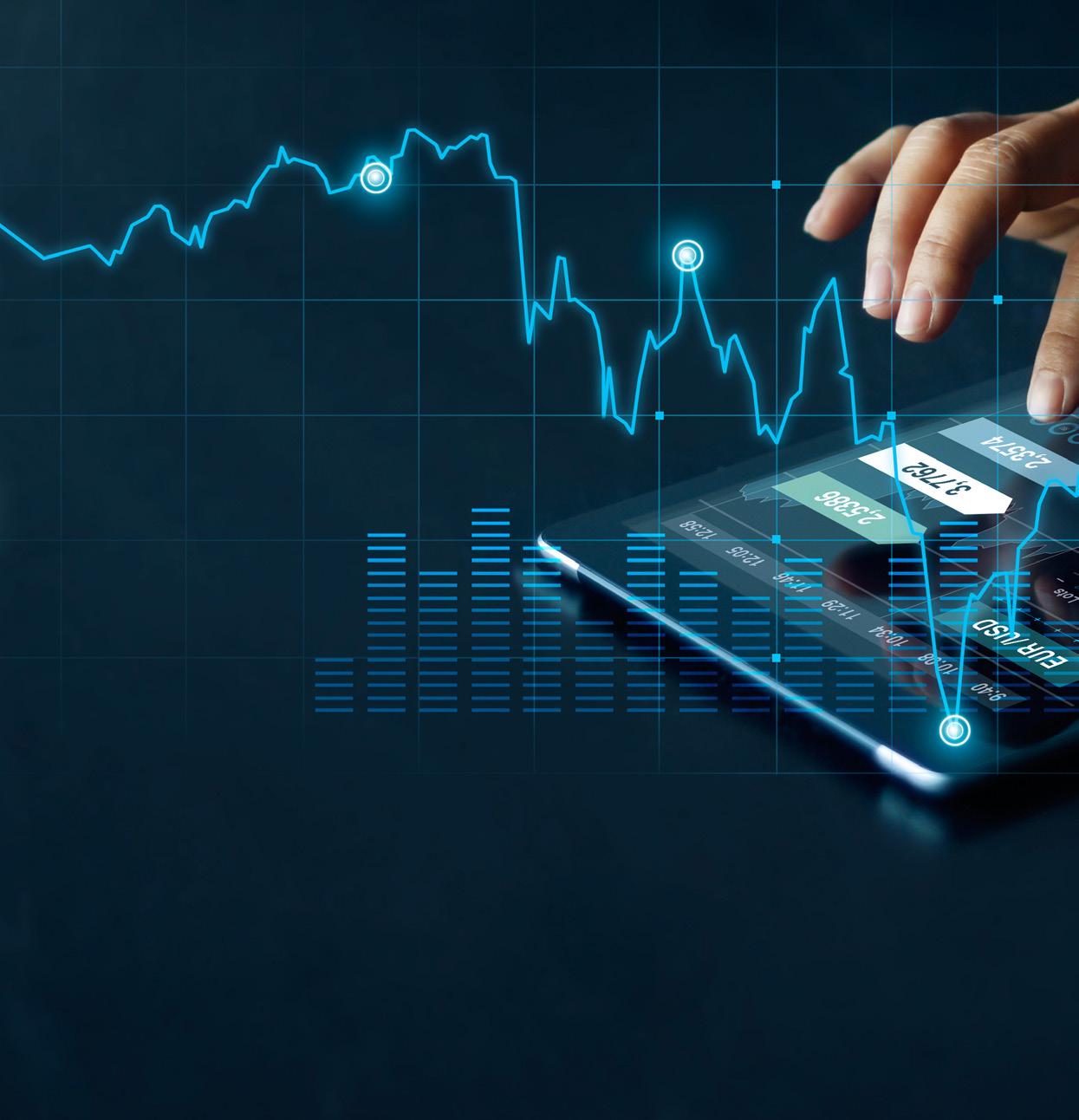
3. Accelerate Algorithmic Trading
High-frequency trading is characterised by ultrafast trades aimed at exploiting small price fluctuations. AI-powered algorithms are capable of analysing massive amounts of data and executing trades with superior speed compared to human traders. For instance, an algorithm can swiftly analyse data on a stock's price movements and automatically execute trades based on identified patterns or trends. By leveraging AI-powered algorithms, the trading team can conduct a higher volume of trades in a shorter time frame, potentially leading to increased profits.
4. Detect Market Anomalies
Challenges have arisen for trading teams in reconciling daily securities pricing, with a majority of exceptions requiring review despite not being true anomalies. To tackle this issue, trading teams can use AI-powered algorithms that utilise historical data spanning multiple years to swiftly build machine learning models. By tracking the movements of a particular stock against other securities, AI can identify anomalies and alert an analyst to review them. This approach not only minimises exceptions but also eradicates the need for manual effort, thus improving efficiency and accuracy for trading teams.
5. Risk Management
Managing risk is a significant challenge in trading, and AI-powered predictive modelling can aid traders in identifying potential risks and gauging the probability of events occurring. For instance, consider a quantitative trading team that has invested in the energy sector and seeks to anticipate the risk of a future decline in oil prices. To achieve this, the team can leverage AI-powered predictive modelling to analyse historical data on oil prices, supply, and demand. The AI algorithm can discern patterns and trends in the data, enabling it to predict the likelihood of a future drop in oil prices. Based on this prediction, the trading team can make strategic adjustments to their portfolio, such as reducing their exposure to the energy sector or diversifying investments into other sectors, to mitigate potential losses.
The bottom line
Each of these applications can have a significant impact on ROI by mitigating the risk of losses, increasing the frequency of profitable trades, and optimising returns while minimising risk. Additionally, the utilisation of AI in quantitative trading can yield cost savings and enhance productivity by automating trading strategies, expediting and streamlining trade executions, and optimising asset management. Through the integration of AI-powered tools, trading teams can make well-informed trading decisions, resulting in higher ROI with improved precision and efficiency.
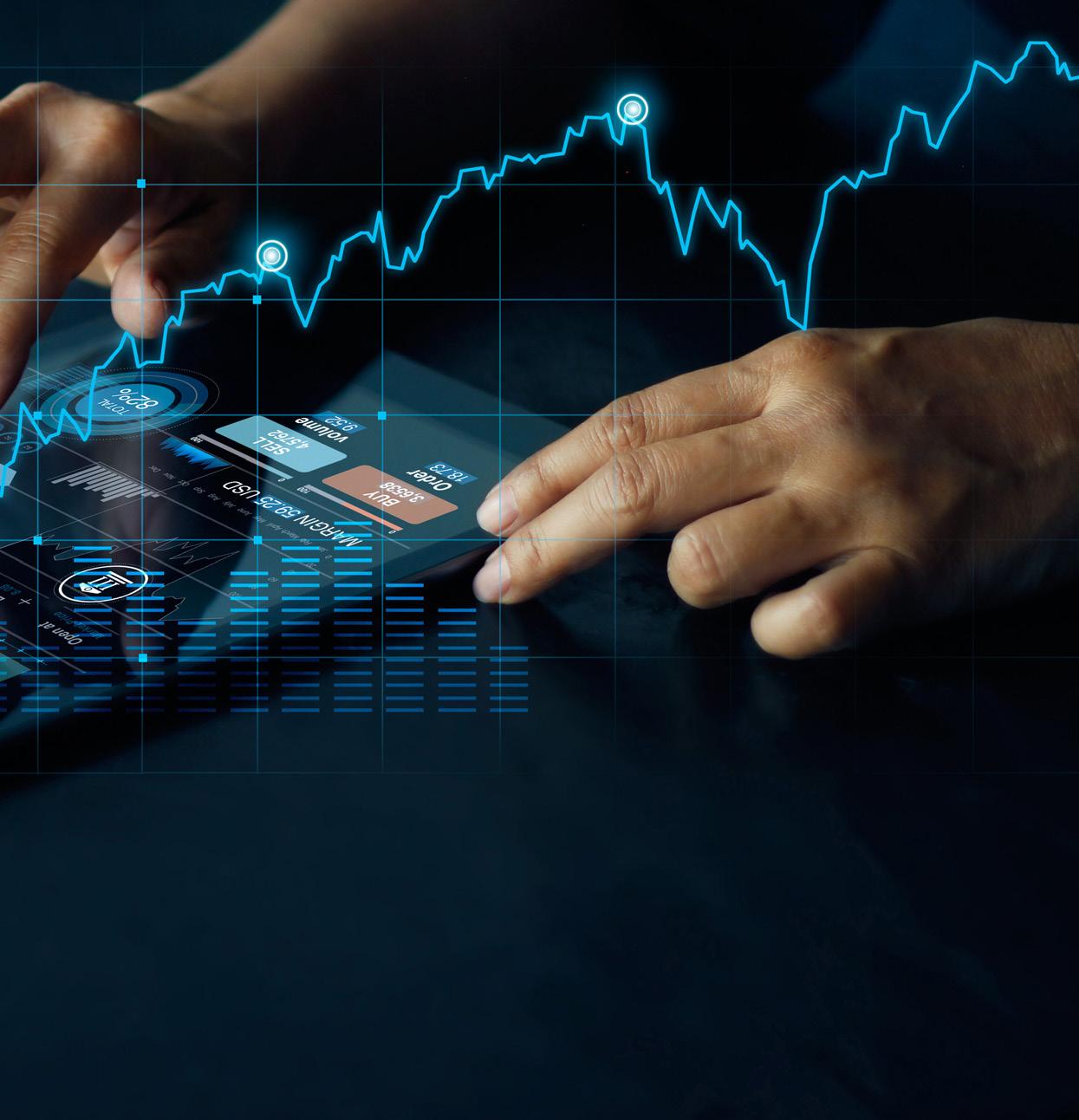
Leslie Kanthan, Co-Founder and CEO of TurinTech
Goldman Sachs has warned that the Bank of England could raise interest rates to 5% this summer as it battles to bring down inflation. The combination of rising interest rates and inflation, coupled with price hikes, has caused substantial challenges for businesses, particularly for SMEs that have been hit the hardest.
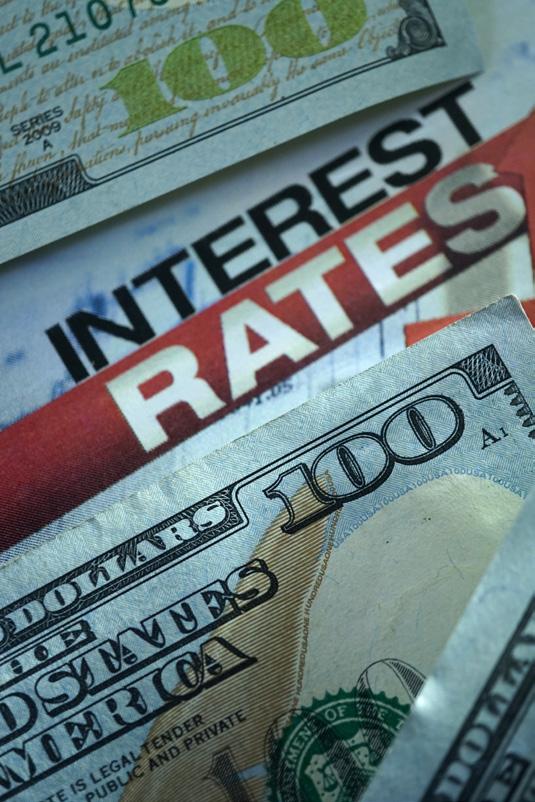
Businesses are already hurting. In December 2022, Lloyds conducted a survey with over 1,400 SME leaders and found that 82% said the rising cost of living was having a negative impact on them, with two-thirds (61%) reporting that inflationary pressures had caused their supplier service to worsen.
In this turbulent, uncertain economic climate, business plans may start to appear more decorative than decisive. To view them as such would be a mistake. A considered business plan becomes even more crucial when the future looks worrying.
So why do business plans matter and what goes into the strongest ones?
DO BUSINESS PLANS EVEN MATTER IN TURBULENT ECONOMIC TIMES?
Why should you have a business plan?
As businesses navigate unfolding economic uncertainty, having a business plan is a lifeline. A study in the New England Journal of Entrepreneurship found that entrepreneurs with a business plan are more successful than those without one. Having a well-crafted business plan can assist in goal setting, identifying potential roadblocks and the ability to navigate complex business issues as and when required.
They provide businesses with a clear roadmap to achieve their goals and objectives and is an invaluable strategic tool. It helps them to identify their strengths, weaknesses, opportunities, and threats (SWOT), a common strategic planning technique to help assess key factors that might affect your business.
In some cases, businesses will often require a business plan before they consider reaching out to investors or even receiving a loan and so it can help them secure funding by demonstrating their potential for growth and profitability - something that many are struggling with in the current climate. The benefits of having a well-versed business plan speak volumes to investors and stakeholders alike and can distinguish the business from its competition.
What makes a strong business plan?
Every business plan will be unique but all good plans will have these key things in common.
1. Clarifying the purpose and goals of your company
Firstly, an SME needs to sit down and define its purpose and business goals. The foundation of a solid strategy depends on answering the ‘why’ and ‘what’ of your business. Why does your business exist? What is its purpose? Establishing this gives your business a guiding light through challenging times and benchmark to measure all business decisions against. Similarly, objectives explain what it is your business wants to achieve. Again, establishing your main objectives in your business plan helps to give further guidance for important decision making.
2. Engage in market research
Being informed is fundamental. After deciding your business goals, the next is to conduct thorough market research to identify the target audience, competitors, and industry trends. This information will help SMEs understand their market position, customer needs, and how they can differentiate themselves from competitors.
3. Formulate a communications strategy
Your communications strategy will help you reach your target audience in the most direct and impactful way possible. You’ll need to identify the best channels to use and the appropriate methods of communication for each. Your goal might be to raise general awareness of your brand, build credibility and reputation or simply drive sales - the strategy will lay out how you’re going to achieve this.
4. Establish your financial plan
One of the most scrutinised parts of your business plan will be your financial plan. Though current economic circumstances may make predictions difficult, being vague here will only do you a disservice. Plus, all businesses will be facing uncertainty. Your financial plan should include projections for revenue, expenses, and profits, as well as an analysis of the funding required to start and grow the business and how you intend to raise it. This part is of particular interest to investors, so the more detail the better.
5. Set up an effective tech stack
It’s wise to also plan what tools and technology you intend to use to fulfil your objectives. The technology that will be of high importance to the day-to-day running of your business will be critical to establish early on, not only from a cost perspective but for their impact on security and compliance, for example. From customer relationship management tools, to a cloud-based business phone system for internal and external calls, finding the best tools for the job and defining the processes around them will become invaluable as your business grows.
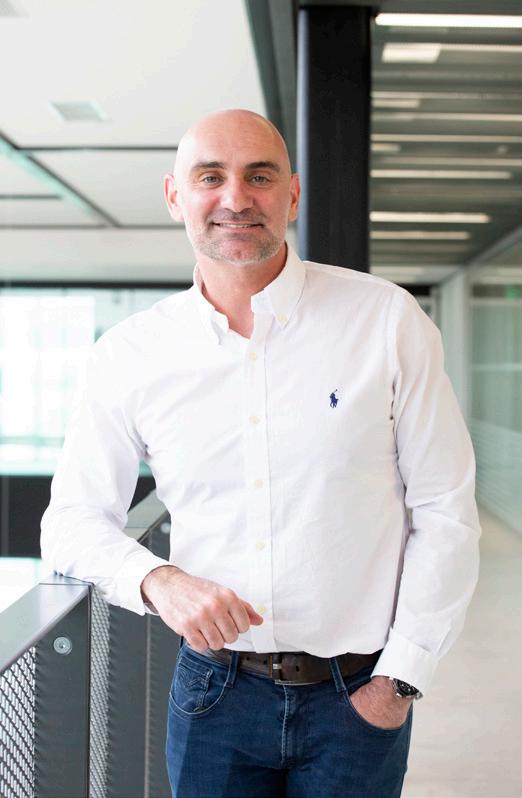
Using your business plan long term
Business plans give businesses the structure and stability they need to withstand the winds of uncertainty. A well-defined, detailed plan enables them to make fully informed decisions as well as attract new investors, raise funds and assess their own progress. Remember that business plans aren’t set in stone and will need to adapt and evolve with your business, so you should expect to continually revisit it and not just dust it off for investors.
Business plans are the bedrock of better decisions. In these economic circumstances, no organisation can afford to ignore them.
- Mike Ferguson, President, EMEA at Redpoint
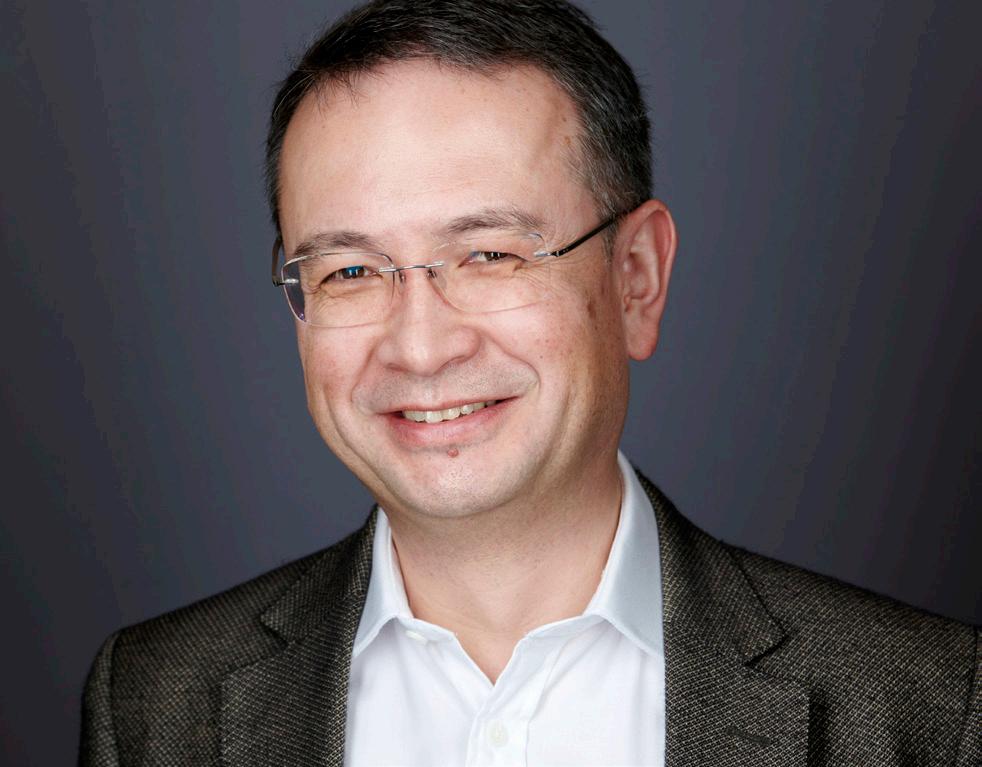