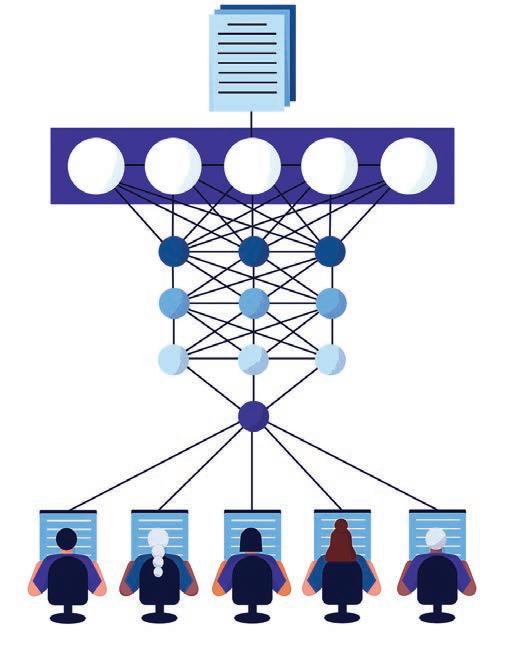
2 minute read
OF WORK
Don’t Overestimate Data and AI
Whether you call it AI, data analytics, or big data—it’s all just statistics. “The more you get into things like machine learning and AI, it’s arguably statistical calculation without statistical inference,” says Neil Hamlett (EMBA’11), data analytics team lead for ECS, which provides enterprise data-strategy support to digital transformation of the U.S. Department of Homeland Security (DHS) Cybersecurity and Infrastructure Security Agency (CISA).
Right now, there’s just a lot of hype about what data can and can’t do. Hamlett uses the hype cycle created by Gartner Consulting to explain: “The premise is that technology-driven business processes go through this maturation process, and that the stages of the hype cycle are a triggering event, which then rises to the peak of inflated expectations, and people start to figure out that there’s a lot of hype, a lot of hokum,” says Hamlett, “and then it’ll slide into the trough of disillusionment. Then it goes up on the slope of enlightenment to a plateau of productivity.”
We are in the inflated expectations phase, he says. Keep in mind—this will eventually level out.
In the meantime, get very acquainted with what you want your data or AI to do, and what it can actually do. AI can’t, for example, think creatively—we still need the human mind for that—and companies using big data can easily run into cost, maintenance, and ethics issues.
Make The Right Hires
When dealing with big data, be sure you’re hiring people who know the technology well enough to recognize its opportunities and pitfalls, but also someone who understands business strategy operations. In other words, you need someone who not only can read the data, but someone able to advise decisionmakers and understand what sort of organizational and operational reconfigurations need to happen in order to get the greatest value out of the tech.
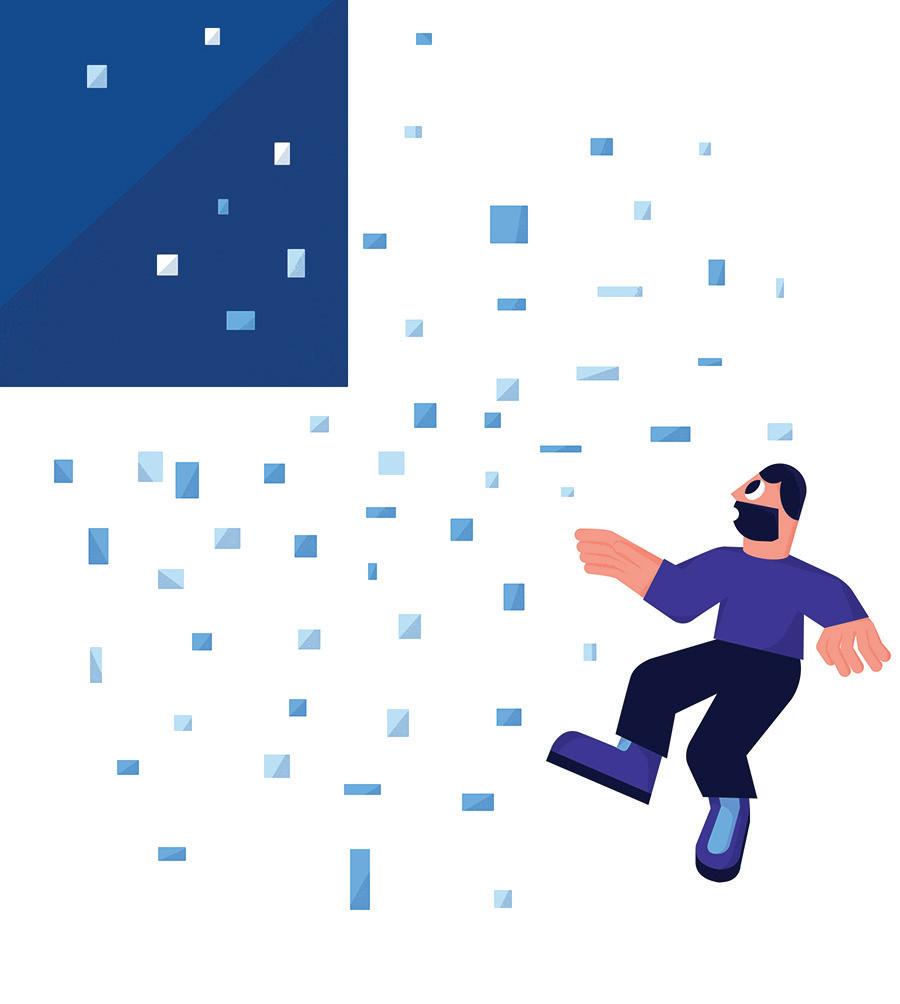
“Everybody’s going to have to be multidisciplinary,” says Hamlett. “Those are the key connections to be able to understand the opportunities and limitations with any given technology—and to be able to recognize that there are limitations.”
It’s not surprising that colleges and universities—including Georgetown—are adding data analytics majors to their curriculum and data scientists to their faculty. Schools already are educating the next generation that will help businesses bridge the data divide and translate numbers into actionable information.
MORE DATA DOESN’T MEAN BETTER OUTCOMES
We used to rely on the nightly news or stock reports to gain insights, but the truth is, some of our information is more available—and more comprehensive—than the news ever can be. “And we can report it much more quickly,” says Kristie Cole (IBP’21), co-founder of FORE, which uses open-source data to to foresee critical trends and equip its partners and clients with actionable insights to support real-time decision making, often in crises like a hurricane evacuation.
What’s more is that in an age of misinformation, data doesn’t lie. There is a catch though: data needs to be focused and refined—if it’s too broad it can be useless or worse, cause you to make bad decisions.
Hamlett and other experts argue that organizations that get value out of big data have figured out what’s important to them and how to measure it effectively. “They’re not getting value out of big data because they’re using more of it,” says Hamlett. They’re getting value, because they’re using the right data. You design the organization and your operations so you get the measurements of what matters, and you understand the limitations of your measurements.”
Another tip from Cole, if you are opting to use big data—or more of it—always compare it to historical data. “If you don’t compare new data to historical data, you may think you’ve made some discovery or uncovered some outlier that doesn’t actually exist.”