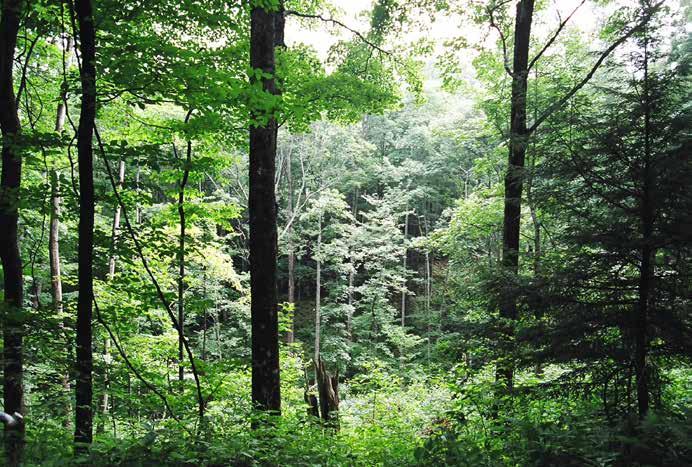
3 minute read
Biochemical Analysis of Forest Species using Hyperspectral Remote Sensing
Overview
Understanding the relationship between the biochemical properties and spectral reflectance patterns of species is one of the core components in ecosystem-related studies, providing useful insights on photosynthesis, nature and the state of plant canopies, and their response to radiation.
Traditional methods of quantifying biochemical analyses have long imposed the challenges of being both time-consuming and expensive. They also need a greater number of samples to capture the heterogeneity within vegetation patterns. However, the use of Geospatial tools like hyperspectral remote sensing for estimating vegetation area (macro scale), account carbon and biomass (meso scale), and species chemical properties (micro-scale) is one of the domain’s major technological advancements. Geospatial technologies have proved to be cost-effective in estimating plant biochemical components like chlorophyll, nitrogen, protein, and other mineral nutrients based on their spectral data.
As the interest in remote sensing for vegetation applications grows, so does the potential of hyperspectral imaging for extracting key information related to vegetation demand. The technology facilitates a detailed understanding of spectral signatures in terms of feature-specific absorption band positioning, the shape of the spectrum, spectral variability, and similarity among various types of vegetation species.
Vision: To facilitate automatic identification and quantification of various vegetation types in a region by credibly correlating spectral signatures collected on field and spectral reflectance measured using space-borne hyperspectral sensors. The integration of in-situ spectral data and space-borne hyperspectral data is to be ensured with the help of a spectral library of various surface features of interest.
Objectives
y To estimate the chlorophyll and nitrogen content of various species with integrated field and satellite-based methods. y To correlate spectral variations with that of canopy biochemical patterns under stress conditions (factors leading to a decrease in chlorophyll, and nitrogen constituents).
Stakeholders Involved
The project team from Lab for Spatial Informatics, International Institute of Information Technology, Hyderabad, Telangana, and Indian Institute of Space Technology, Thiruvanthapuram, Kerala, India. Solution and Implementation
Ground truth data generation
Æ Field work for data collection was carried out thrice: February 2017 (representing dry conditions),
September 2017 (wet conditions) May 2018 (a stress condition) to observe the behaviour of the spectrum of tree species with respect to seasonal conditions. Æ Ten species were selected based on their economic importance and dominance distribution in the study area for spectra collection from leaf samples. Æ The field spectrum was corrected statistically for dropouts and outliers in the reflectance values. Æ The wavelength values collected during both the fields were different due to using of different instruments, so the resampling technique was applied using the Gaussian technique with 6 precision, to compare spectra of two seasons. Æ The Spectral matching for the field collected data was carried out to check the discrimination between the species in different seasons. Æ The parameters obtained from field works were used for the calculation of upscaling the leaf reflectance to canopy level. Æ Field collected leaf samples were subjected to lab analysis for calculation of biochemical parameters like chlorophyll, nitrogen, cellulose, and lignin. The biochemical parameters obtained for each sample were matched with the field collected spectra for three different seasons.
CHRIS PROBA data acquisition and processing
Æ PROBA CHRIS data for three different seasons was acquired to correlate with field data. Æ Satellite data of February 2018 was acquired as an alternative/substitute data for February 2017’s data for the same fieldwork, which could not be used due to 100% cloud cover. Since this was a forest area, it was assumed there had been no changes of significance, and hence data from 2018 could be used