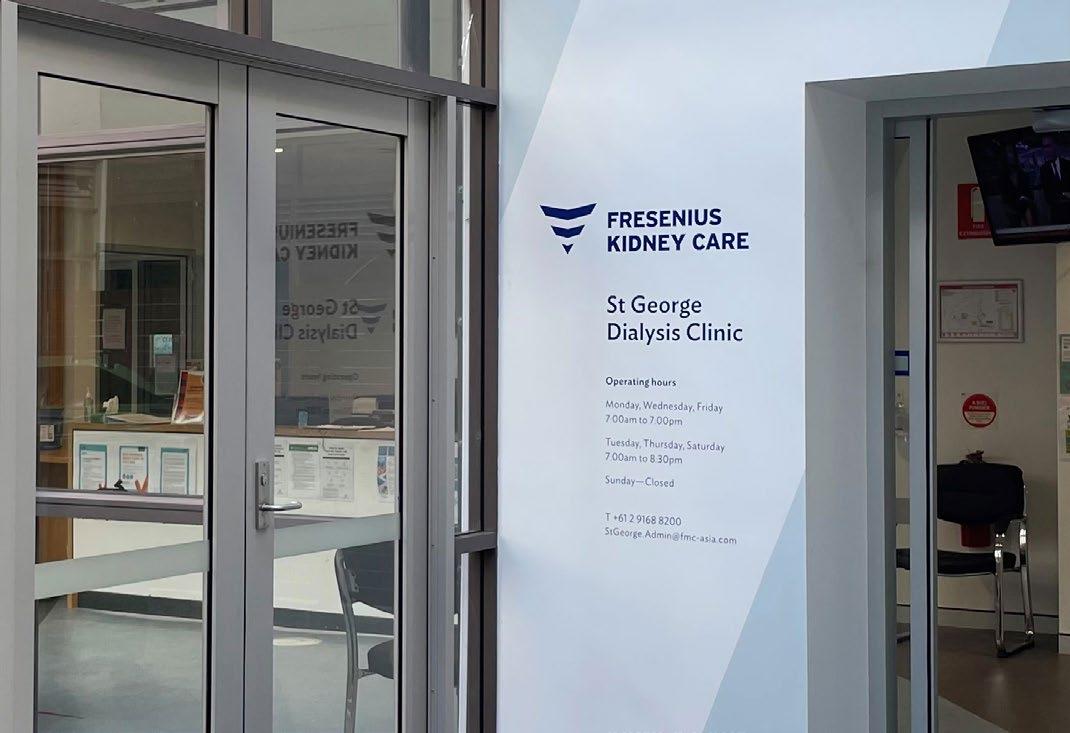
7 minute read
The Role of Big Data in the Fight Against COVID-19
Big Data
By Caitlin Monaghan, PhD, Senior Data Scientist, Applied Data Science, Biostatistics, and Epidemiology, Global Medical Office, Fresenius Medical Care and Len Usvyat, PhD, Vice President, Applied Data Science, Biostatistics, and Epidemiology, Global Medical Office, Fresenius Medical Care
Advertisement
How Big Data Analytics Have Improved Infection Predictions and Supported Patient Care during COVID-19
Worldwide, around 3.7 million patients regularly undergo dialysis, a treatment for end-stage renal disease that filters the blood. Providing dialysis creates a unique opportunity to gather unusually detailed patient data. When this data is combined with machine-learning tools that can function like brain neurons, artificial intelligence (AI) models can be created. These allow new insights to be obtained from data and to predict outcomes based on these insights.
With a wide array of global patient data sets now available, Fresenius Medical Care explains how this data and advanced modelling have been successfully used to predict patients who were infected with SARS-COV-2 virus before they tested positive for COVID-19, and how other analyses helped to identify patients who were at greatest risk of severe complications from COVID-19.
Caitlin Monaghan, PhD, and Len Usvyat, PhD, explain the value of this data for patients, clinicians, governments and businesses, especially as we have entered the post-first waves stages of COVID-19 in some countries and regions.
Fresenius Medical Care is a vertically integrated healthcare company and the world’s leading provider of dialysis products and services. The company provides dialysis to more than 346,000
RELATIONSHIP OF ARTIFICIAL INTELLIGENCE, MACHINE LEARNING, AND DEEP LEARNING
Artificial Intelligence
A program that enables computers to mimic human behaviour.
Machine Learning
Subset of AI that uses statistical methods to build programs and whose performance improves when exposed to large amounts of data.
Deep Learning
Subset of machine learning in which multilayered neural networks learn from vast amounts of data. Big Data
Big Data patients with kidney disease through its more than 4,000 clinics worldwide, as well as by offering products to partner hospitals and clinics.
Dialysis therapy gives a unique opportunity to collect data and view what happens to patients over time at a level of granularity unseen in any other healthcare environment. In North America, the company has collected significant amounts of treatment data on over 1.1 million patients, 110 million dialysis treatments, and 1.2 billion laboratory tests. This data is extraordinarily detailed, with hundreds of data elements collected per dialysis treatment, some on a per second interval. Globally, the company has access to dialysis patient data on an even larger scale, covering 1.9 million patients, 503 million dialysis treatments, 1.7 billion laboratory tests, and almost 4 billion administered medicines. Data of this magnitude and complexity is often referred to as ‘big data’ and grows continuously.
How the Data is Used
Using cutting-edge data science and machine learning methodologies, Fresenius Medical Care is able to leverage this data to help develop new benchmarks in dialysis patient care and conduct research that advances medicine. Artificial intelligence (AI) is a data science discipline that combines traditional statistical methods with sophisticated machine learning tools to gain insights and predict outcomes from big data. The company has successfully constructed a range of AI models that use its own clinical and non-clinical data from external sources. These models have predictive capabilities that provide support in making clinical decisions and help to direct patient interventions.
Modelling can be used to identify which dialysis patients are most likely to be hospitalised in the next seven days and in the next 12 months, and which patients are at greatest risk of infection. Other models predict the progression of chronic kidney disease before patients start dialysis, allowing for a much more successful transition that reduces emergency interventions. Overall, these modelling approaches allow more precise personalised care for the company’s global patient populations.
Extending this Modelling to COVID-19
Dialysis patients often have other co-existing health conditions, known as comorbidities. These increase their risk of complications from infections. During the global COVID-19 pandemic, Fresenius Medical Care has been able to use its large global databases to assist at-risk patient populations. As a rapid response to the pandemic, the company developed machine learning predictions about which counties in the U.S. and which dialysis clinics globally were likely to be affected by a new COVID-19 outbreak in the next one to two weeks. This model allowed clinicians to identify specific patients who had early changes in laboratory values or vital statistics before they tested positive for COVID-19, alerting clinicians to infections before the virus became more widespread.
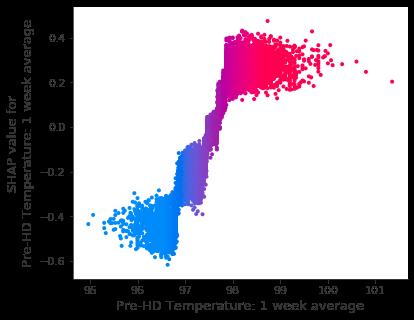
FIGURE 2 | Graph showing how patient temperatures can be used to predict the likelihood of a future positive COVID-19 test
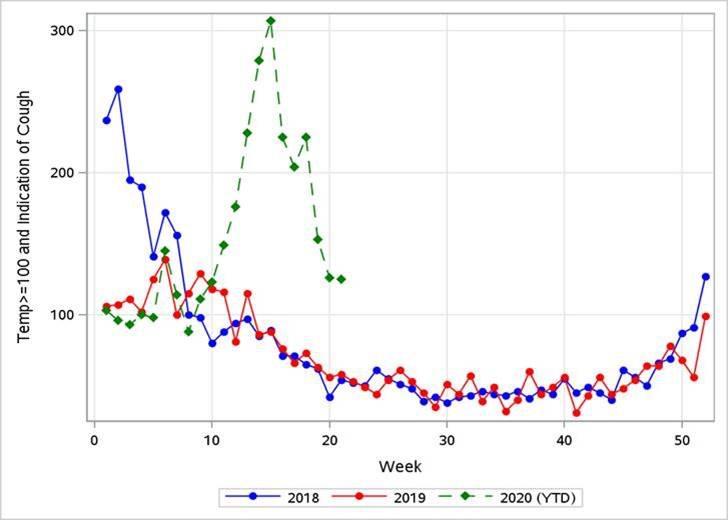
FIGURE 3 | Dialysis treatments where Fresenius Medical Care patients in North America had a fever and cough Big Data
One of the variables included in the model showed the relationship of patients’ body temperatures to the likelihood of having an infection. In the figure below, the horizontal axis shows the distribution of patient temperatures. Values on the vertical axis that are above zero show an increase in patients’ likelihood of having COVID-19, while values below zero show a decrease in that likelihood. These are known as SHAP values, an acronym for SHapley Additive exPlanations. Notably, the mean temperature for COVID-19-positive patients was only 0.2° Fahrenheit higher than the mean temperature of patients who were not diagnosed with COVID-19 (97.7° F [36.5°C] v 97.5° F [36.39°C]). This further illustrates the importance of comprehensive data-driven approaches to identify patients at risk of COVID-19: with such small differences in temperature, clinical observation is unlikely to result in screening for COVID-19, while very small changes in several patient parameters can be picked up by a computer algorithm.
Other analyses helped to identify patients who were at increased risk of severe complications if they were to acquire COVID-19. While limited data exists on which patients are likely to die or be hospitalised with this virus, realtime monitoring of data allowed for early understanding of patient characteristics that are most likely to be associated with negative outcomes.
Real-time data surveillance also helped to identify geographic areas with COVID-19 spread. The illustration below compares the number of dialysis treatments where patients had a fever and cough by week in 2018, 2019, and 2020. The graph clearly demonstrates the real-time spike in these symptoms.
These tools can be used and adjusted over time, making them extremely valuable for any future waves of COVID-19 and other pandemics.
The Value of AI in Post-COVID Healthcare
In the United States, the government supports the use of big data in improving healthcare delivery through a variety of initiatives. Dialysis is mostly funded by the federal government through the Medicare program and all providers, including Fresenius Medical Care, share data through a formal network system. The data is
de-identified to protect patient privacy. In 2019, the United States launched the American AI Initiative, the country’s national AI strategy. This directs the government to pursue investment, training, and the development of an international environment that supports AI innovation.
In the regulatory landscape, predictive modelling such as the work we have described, is categorised as Clinical Decision Support Software (CDSS). As the products of these models are suggestions, such as lists of patients at high risk, and not actual prescriptions or orders for clinical care, they are generally exempt from regulation as a medical device.
For medical innovations built on big data and automation to thrive, it is crucial to improve societal understanding and the regulatory environment for data sharing. In order to ultimately improve patient quality of life through these regulatory changes, we must actively promote innovation using data.
The COVID-19 pandemic, unprecedented in scope and severity, has also led to unprecedented uses of clinical data within and across countries. Given the current environment of uncertainty, healthcare systems are under pressure to deliver more and better care with limited resources. Future predictive models will need to be even more comprehensive and responsive, and data will be a crucial resource to fuel these models.
About us
Fresenius Medical Care is the world’s leading provider of products and services for individuals with renal diseases of which around 3.7 million patients worldwide regularly undergo dialysis treatment. Through its network of 4,110 dialysis clinics, Fresenius Medical Care provides dialysis treatments for 344,476 patients around the globe. Fresenius Medical Care is also the leading provider of dialysis products such as dialysis machines or dialyzers. Along with its core business, the Renal Care Continuum, the company focuses on expanding in complementary areas and in the field of critical care.