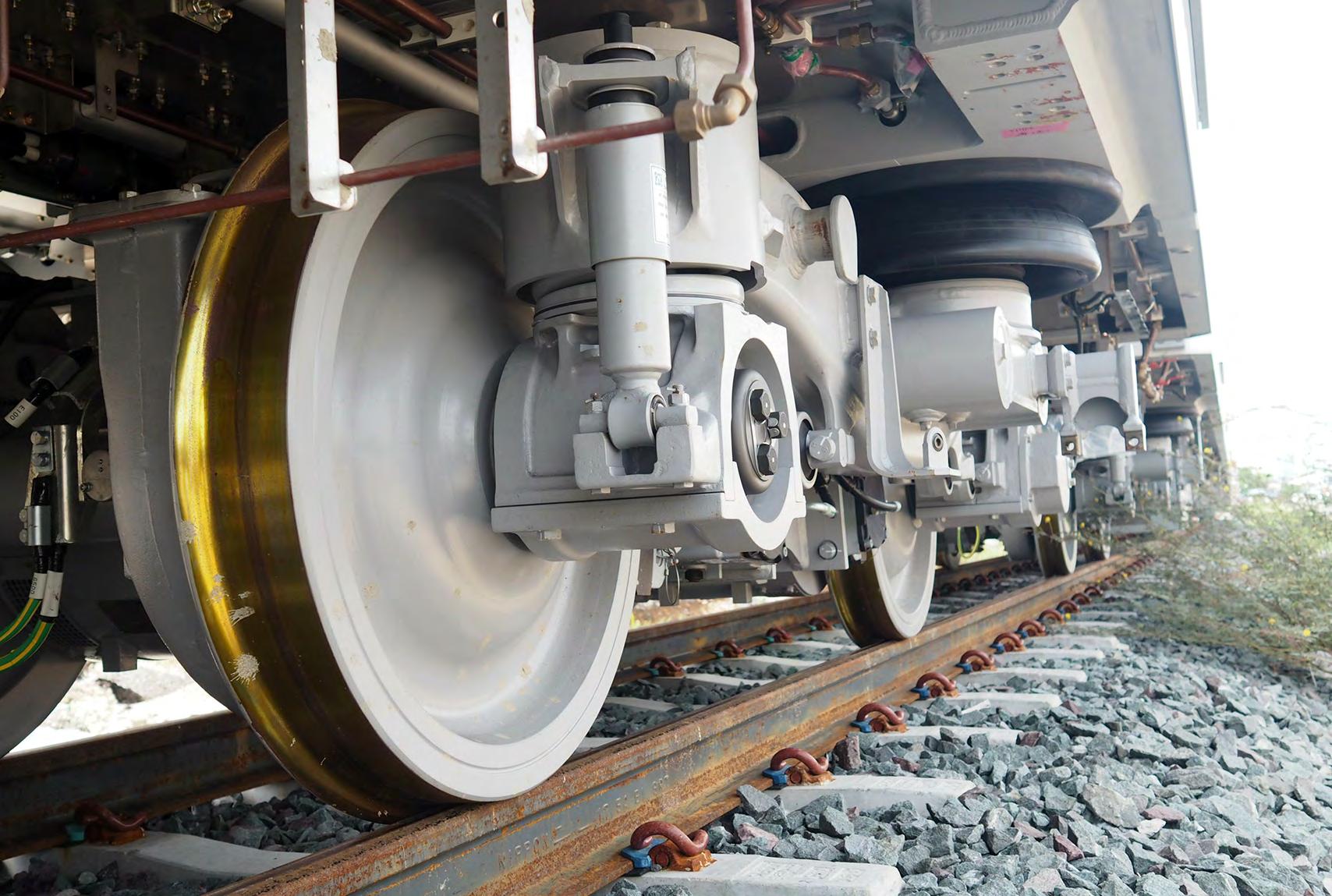
5 minute read
Predictive maintenance: Safety and cost-effectiveness enhancement in sustainable railway services
Dr.-Ing Ekkarut Viyanit
In the new era of mobility and logistics, a rail system will play a crucial role in land transportation of passengers and goods due to its more superior safety, cost competitiveness and environmental friendliness compared to other transportation modes. According to Thailand’s vision to become a regional railway network hub in South-East Asia by 2027, it is very important to prepare the existing and upcoming railway systems with sufficient quality in comparison with neighbouring countries. It is widely agreed that a modernised maintenance management system is one of the most effective and efficient tools for ensuring the reliability, availability, maintainability and safety of railway services in all perspectives.
Advertisement
Due to the large role played by maintenance in the life-cycle cost of railway assets, the National Science and Technology Development Agency (NSTDA), Thailand is conducting research and development in the field of predictive maintenance corresponding to technical challenges in the railway business nationwide. It is believed that an efficient and effective maintenance strategy can improve the quality of railway services at an optimal cost throughout a railway life cycle.
CHALLENGES AND BENEFITS OF PREDICTIVE MAINTENANCE IN RAILWAY APPLICATIONS
A conventional approach by maintenance in railway assets, e.g., rolling stock, infrastructure, signaling system etc., has mainly relied on periodical maintenance, i.e., time-based or mileage-based maintenance. Based on experiences of many railway operators worldwide, it is realised that periodical maintenance should be replaced by further developed maintenance technology in order to address current constraints in dealing with improved ecosystem sustainability and cost competitiveness. Due to technological knowledge enhancement, together with developing advanced devices and systems for monitoring and diagnosis, predictive maintenance has been developed and sub-categorized under condition-based maintenance (CBM) as defined by EN13306 (CEN, 2001).
In fact, predictive maintenance is classified as a means of data-driven decisions based on algorithms and carried out at an appropriate time prior to the occurrence of a failure event as shown in Figure 1. Currently, it is very challenging for a technical crew to execute the just-in-time maintenance shortly ahead of the failure event. Therefore, predictive maintenance actions need to have comprehensive and more precise data for failure prediction as well as for maintenance planning and scheduling. Figure 2 illustrates the typical architecture of data-driven predictive maintenance applicable to railway assets.
Data monitoring serves as a fundamental element of predictive maintenance, followed by data deposition, data analytics and data insight, respectively. For railway assets, the data-driven predictive maintenance reveals its capability to ensure the high quality of services through performing just-in-time maintenance, minimising unexpected equipment downtime and avoiding catastrophic failure of assets (Schmidt et al., 2014). In addition, total cost saving is also highlighted as a significant outcome of preventive maintenance (Zhang et al., 2021).
RESEARCH AND DEVELOPMENT ON PREDICTIVE MAINTENANCE
As a part of its role as the national science and technology development agency, NSTDA aimed to develop a platform of data-driven predictive maintenance technology which is applicable to the rail transport sector. In the case of rolling stock, for example, structural health monitoring (SHM) in association with data-driven predictive maintenance of a bogie is an active research and development (R&D) topic conducted at NSTDA. This is because bogies are recognized as a crucial component at the heart of railway dynamics. Figure 3 indicates an R&D framework regarding data-driven predictive maintenance of railway vehicle bogies. At the fundamental level, it is very necessary to employ various on-board sensor-based monitoring techniques to acquire data from the bogie. For example, vibration transducers are used to acquire vibration behaviors occurring on the bogie during service. Strain gauges are sometimes required if stress-strain distribution of a bogie’s structure is needed for engineering reliability assessment according to a demand of predictive maintenance. In some cases, a novel technique using ultrasonic guided waves is also employed for real-time monitoring of fatigue cracks in the bogie frame (Yan et al., (2019).
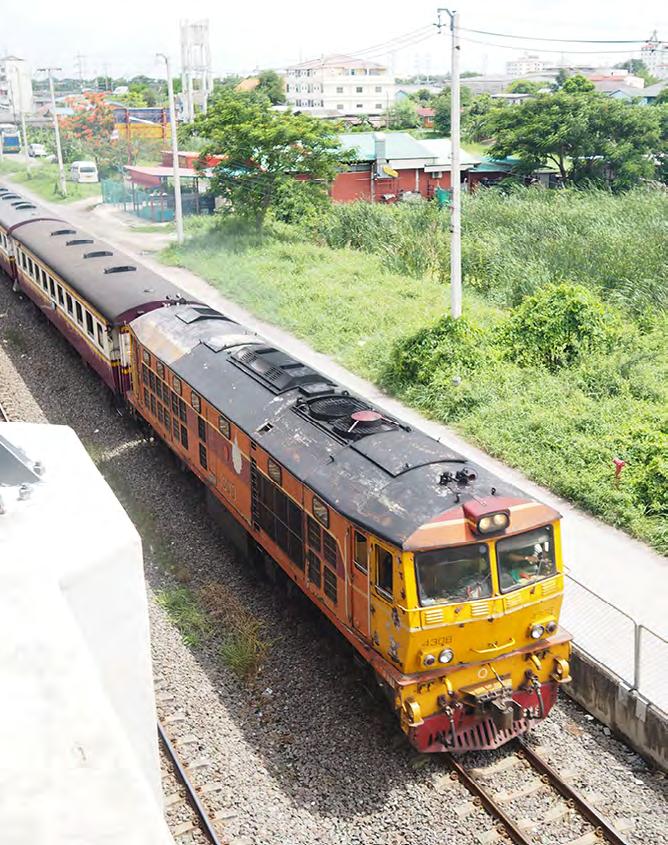
In addition to on-board data monitoring, in-field inspection data including non-destructive testing (NDT) should also be included as part of data monitoring. After that, data management is carried out to ensure that the quality of data is sufficient for data analytics. In the process of data analytics, a technology of digital twin serves as an enabling tool to gain an insight into technical information from various scenarios in addition to the actual service condition of railway vehicles. With accurate data and powerful algorithm in data analytics, effective and efficient predictive maintenance can eventually be made to enhance the safety and quality of railway services to be as expected.
Figure 1. Maintenance classification and predictive maintenance concept
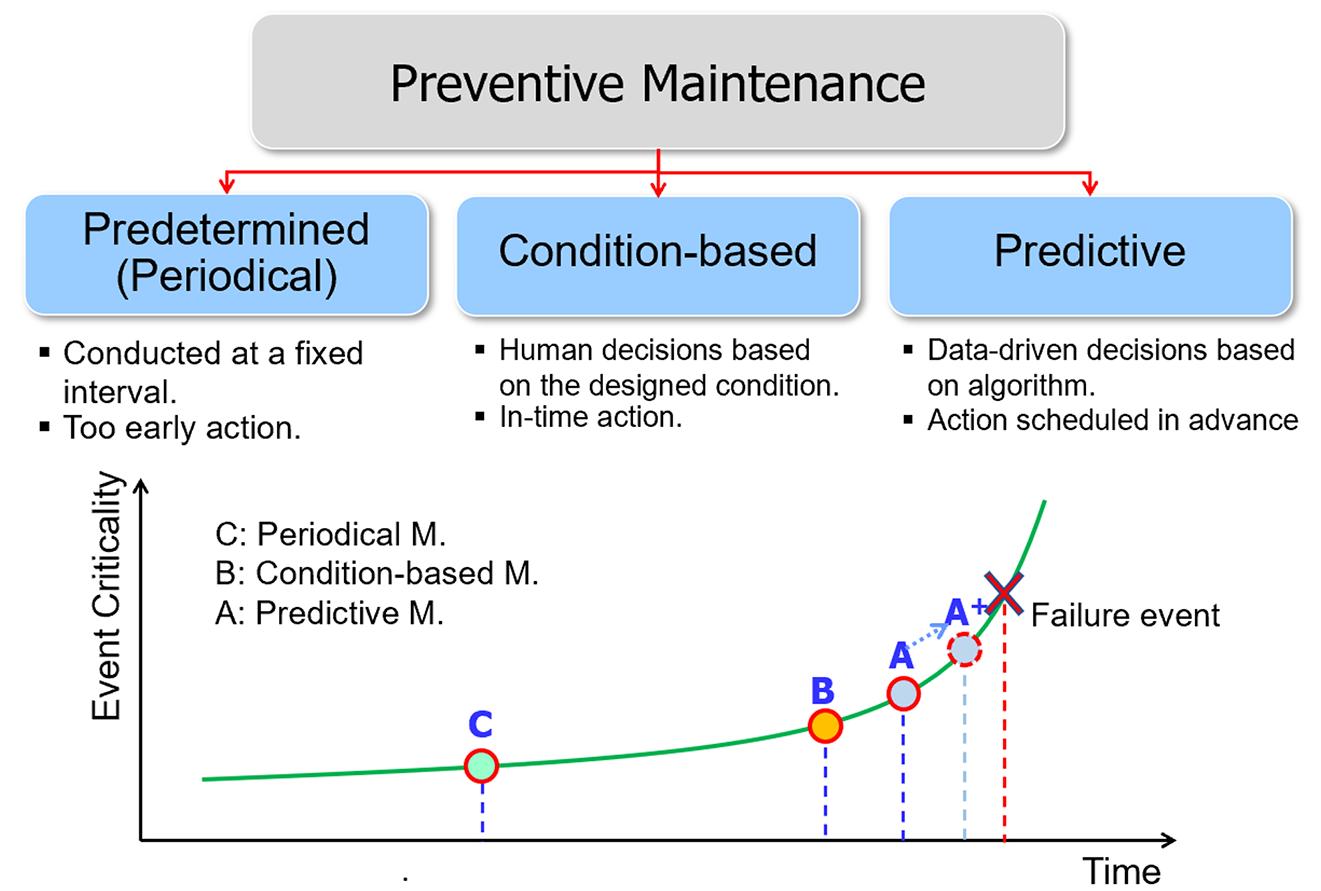
Source: Schmidt, B. et al., 2014, Next generation condition-based predictive maintenance.
Figure 2. Architecture of data-driven predictive maintenance
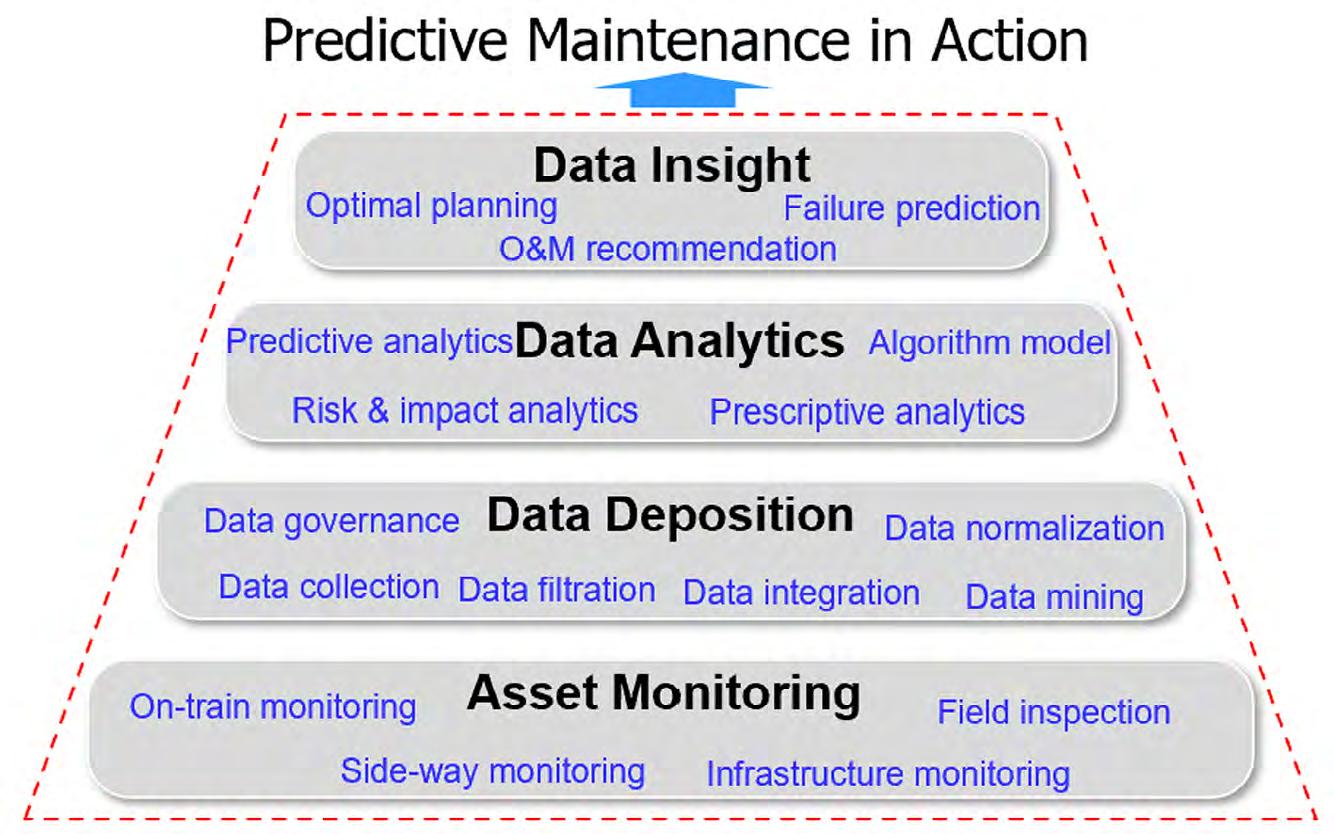
Zhang, C. et al., 2021. Scheme design of railway predictive maintenance based on IoT and AI technology.
Figure 3. Research and development on data-driven predictive maintenance for railway assets (rolling stock)

Contact details:
Dr.-Ing. Ekkarut Viyanit, Principal Researcher and Director, Rail and Modern Transports Research Center (RMT), National Science and Technology Development Agency (NSTDA), ekkarut.viy@nstda.or.th
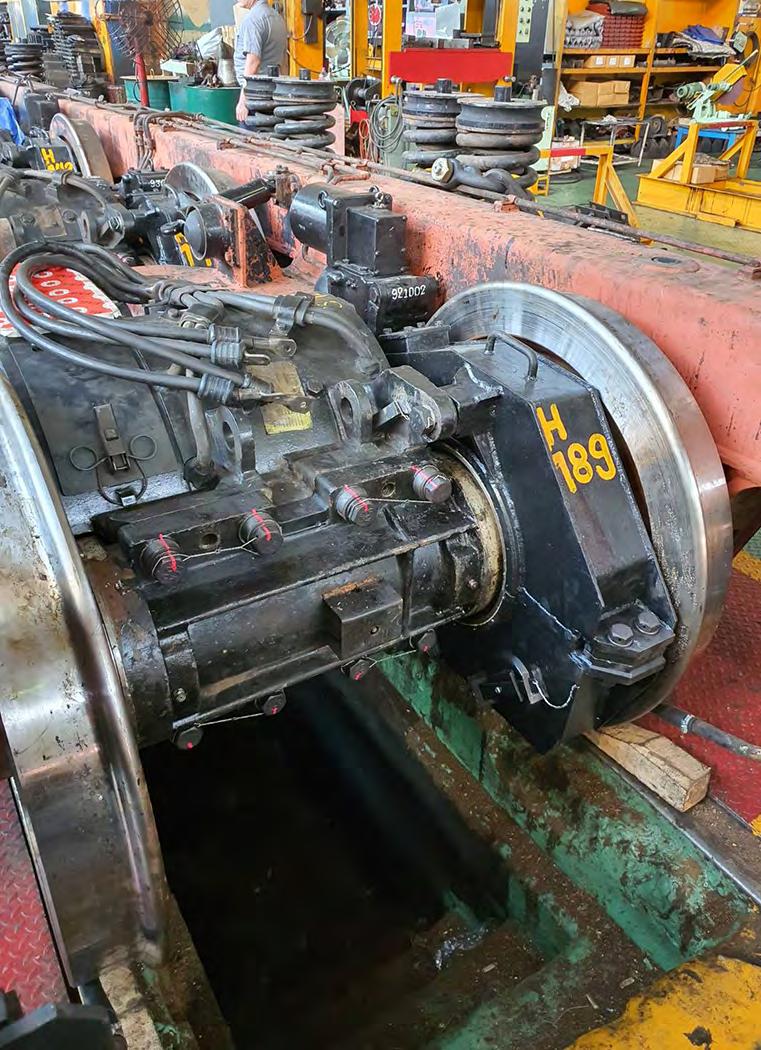
References
CEN (2001). Maintenance terminology. European Standard EN13306.
Schmidt, B. et al. (2014). Next generation conditionbased predictive maintenance. Available at https://www.researchgate.net/publication/ 318775487_Next_Generation_Condition_ Based_Predictive_Maintenance/citation/ download
Zhang, C. et al. (2021). Scheme design of railway predictive maintenance based on IoT and AI technology, Proceedings of the 7th Annual International Conference on Social Science and Contemporary Humanity Development (SSCHD 2021).
Yan, J. et al. (2019). Active monitoring of fatigue crack in the weld zone of bogie frames using ultrasonic guided waves. Sensors. 19. 3372. 10.3390/s19153372.