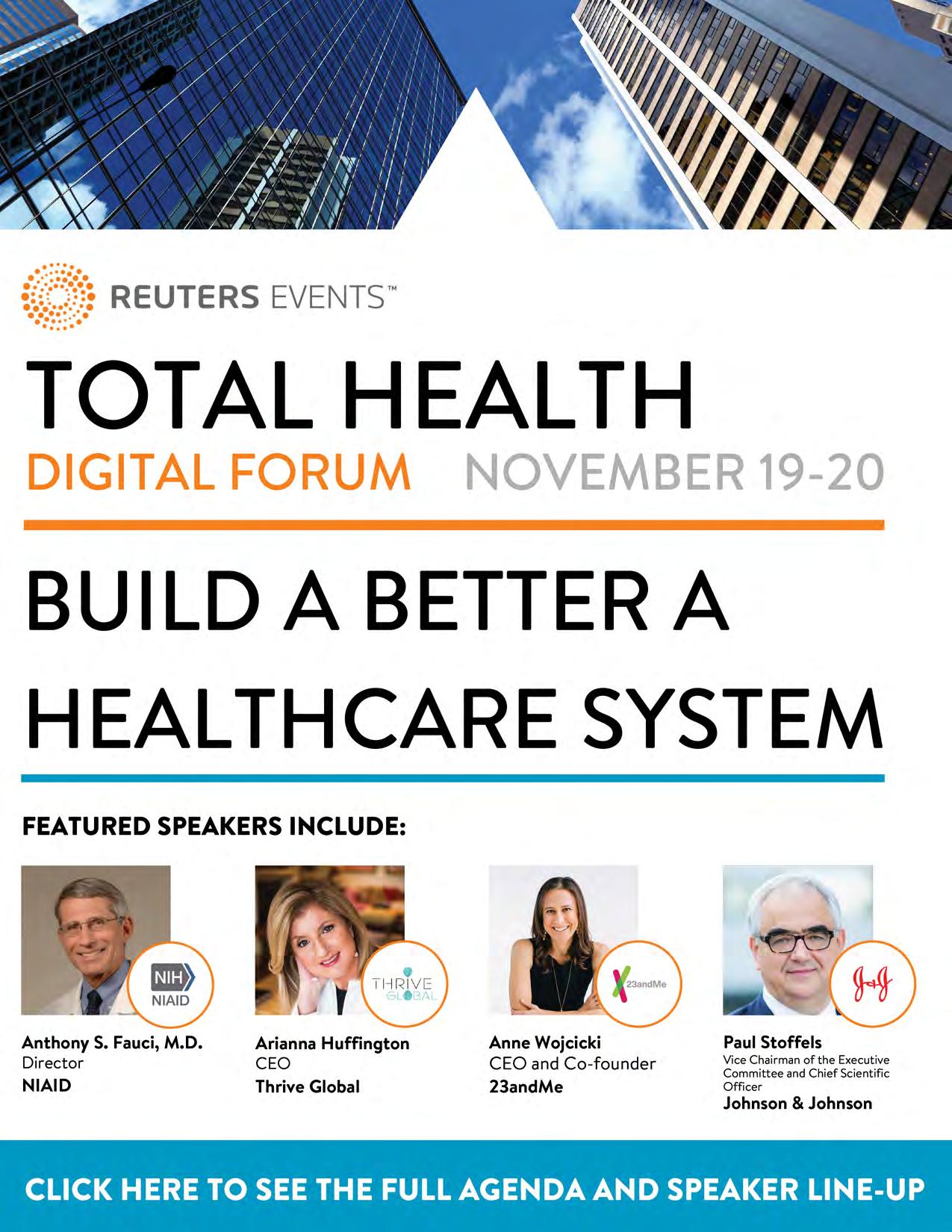
15 minute read
How Commercial Can Best Use AI: a Veeva Summit Talk
Execs from Antares, GSK and Flexion On Evolving Commercial Operations to Fully Maximize AI
Moderator:
Advertisement
BRIAN MAHONEY General Manager Veeva Andi
Speakers:
SAL PAOLOZZA Senior Director, Sales Operations Antares Pharma MADHAVI RAMAKRISHNA Global Head, Commercial Data & Analytics, Pharma Technology GSK
HAZIQ HAQUE Director, Commercial and Medical IT Flexion Therapeutics
Artificial Intelligence, AI, has been one of the key terms bandied about in the industry. But it’s a big category. What does it mean? How does it help us? What’s the relationship between AI and Big Data? The Veeva Commercial & Medical Summit, North America, is the largest commercial and medical affairs event in the life sciences industry, connecting over 1300 professionals. This year it was conducted virtually, and featured over 50 sessions, five specialized tracks, and 47 speakers. The event included this media roundtable on how commercial operations can better use artificial intelligence, conducted by Brian Mahoney, Veeva’s Andi General Manager. Healthcare Sales & Marketing is grateful to Veeva for the opportunity to feature highlights from the interview in this article.
Let’s get a little bit about GSK and what you’re doing for AI and some of your organization’s motivation, because you have a very diverse and large program. Madhavi Ramakrishna: One, from a delivery focused standpoint. And the second, of course, from an operational standpoint. There’s a huge agenda coming from the top, particularly in that we are dealing with the COVID crisis and a focus on driving top line growth, more than ever. And of course, making sure that we get the launch effectiveness right. What does that look like in terms of analytics and data? And simply going back to the basics of what do we do to build and automate the routine data insights that we consume on a day-on-day basis. That’s from a delivery standpoint. Moving on to the operational, we always talk about the people, process and technology. What does that mean in terms of advancing our agenda from the basic BI to the more advanced analytics? It’s the AI and machine learning. And then just getting into the mindset of testing and learning, leveraging our information. There are a couple of different aspects that we are touching on. One, recruiting the right level of talent. Second, making sure that we are, from a mindset standpoint, ready to operate and then capitalize on the data assets that we have probably sitting in our backyard. And of course, making sure that we are able to cohesively think of it as a heat map view of our BI services and capabilities. What does that mature product or capability look like to then be the growth and the performance? Where do we need to invest more? The first step is organizing our house, going back to the drawing board and making sure that we have some of these foundational frameworks. To move into the programs, I think there’s a huge focus around rep effectiveness. What can we do again in the areas of landing the right message on the right channel at the right time to the right customer? It’s our next best program, which essentially touches on the maturity that we need to have as an organization from a data management standpoint, to then being able to apply it to a bespoke need from a market standpoint to then delivering this program at scale. That’s just scratching the surface of what we have going on. In specialty, a lot of initiatives and agendas there, oncology and other key areas of interest where we’re talking about potentially advancing our frameworks or platforms to then leverage the real-world evidence. What does that mean? And all of this is the AI, our maturity or the advancement of our maturity in the AI space. Sal and Haziq, what were your company’s motivations for AI, and particularly how do you see AI driving and changing your business? Sal Paolozza: A year ago, we were launching a branded product in a highly generalized market. In addition, there was a lot of peer pressure around that product. What we wanted to do, and what we accomplished was really to improve our pull-through and provide the assistance to patients to get covered for the product. We were getting information from a lot of sources, some coming in daily. We used Veeva Andi’s AI to prioritize the suggestions that we were sending out to our sales force. That helped with pull through. Depending on the physician, depending on the patient, it would prioritize the suggestion allowing the representative to provide the proper assistance to the practice for pull through purposes and to help patients get access to our product. Since we’ve implemented, we’ve seen nice growth, and obviously we’ve seen a lot of consistency for our product.
Haziq Haque: I think our motivation into the AI space really fit part of our long-term vision, which was improving our business intelligence (BI) landscape. How do we service our main customer, which is our rep? How do we make their lives easier? How do we make their lives more effective? And how do we deliver data in a much better way than what we had been doing? And then we wanted to look into how do we get the insights from our other reporting assets and provide it to our reps, if we have a big dashboard, a large data set that we’re providing them? A lot of the overhead in terms of determining insights, determining key factors in their data was placed back onto them. We want to enhance their insights without drowning them in noise. That’s where that AI component really comes in and shines for us just to say, “Not only are we taking your big dataset, we’re narrowing it down into key information for you.” We’re also taking it a step further and saying, “Here’s the best insight for you today.” It really helped with that prioritization component. The Veeva CRM and Andi helped us sift through the insights.
Our long-term vision is improving our business intelligence (BI). How do we service our main customer, our rep? How do we make their lives easier and more effective? And how do we deliver data in a much better way?
Sal Paolozza: I would like to just add to that. I think the data was always available to all the reps. Obviously you launch products, you have products, you have portals, you have dashboards, you have reports, right? You have a lot of good reps, but with varying expertise when it comes to getting and analyzing information. What AI has really surfaced is getting the same level of information to all reps. This way they can act on it without them having to go in and look through all that noise, which is tons and tons of reports and portals. I think that’s where we succeeded. It’s surfacing the right information at the right time based on the right clients and patients, the demographics. Any surprises or learnings in getting AI going? What about user adoption of AI as a tool for your teams? Haziq Haque: You helped us shift some conversations internally. A big opportunity or shift that we saw was in that conversation between marketing and sales. AI became another tool set for them, another channel for them to engage reinforcement with the reps and reinforcing good behavior. The other side of this that we saw was we were able to take anecdotes from a high performing team and say, “Look, this is a great example. We’ve launched this Veeva CRM Approved Email template that’s really successful in our region. We were able to replicate that key behavior across the whole team. I think it provides a great framework for people to say, “Oh, okay. I get how this works.” It really sets up a nice framework to say, “Here’s our segments. Here’s our actions. Here’s how AI is going to improve and enhance that interactivity.” That’s been the big shift that I think we’ve seen. Madhavi, you brought up a point about changing rep behavior. How are they adapting to the idea that a machine is giving them suggestions, and what the next best action might be? And is there any pushback from your field teams? Madhavi Ramakrishna: I think this is no different than the machines telling and validating the gut, right? We are experiencing a bit of that. Vary your data suggesting and guiding you along the way. I see this as more of a change management problem and less of an AI or machine learning problem. What we have tried to do is preempt that. When we are trying to deploy these large programs, what are the showstoppers that come along the way? How do we put a structure in place to account for those little nuances? We do have the handoffs or the principle that we are going to centrally guide and establish these frameworks. And then we are going to have locally-led change management teams to push and continue to educate our reps. There’s a huge amount of investment and effort in terms of market engagement, because at the end of the day, you can have all the fantastic programs filled, but if you cannot land them in the hands of users, you’ve essentially failed or delivered a compromised solution. There’s a huge focus around market engagement, making sure that we are continuously training, up until the point of launch of all these programs. And of course, handholding post launch.
Sal Paolozza: I’ve been nodding because it’s definitely changed management. There’s a lot of training behind it and really explaining the technology and the value. But I want to pause on value. After a year of using these programs, I actually have reps and managers coming and saying, “You know what would be nice? This type of suggestion. We’d like to see these insights.” And the reason is as long as the suggestions and the insights are providing value to the representative, which they can then in turn use to bring value to their accounts, they’re happy to use it. They want to learn it. But I think the trap that you’ve got to avoid is creating more noise. We went through a period where the reps complained. “Way too many suggestions, too frequent,” and so forth. We had to come up with the right balance between the frequency, the prioritization, and the value that we’re bringing through Veeva Andi to the reps. Once we got that, now they’re demanding more insights. And I think that’s the key. Training and value, make sure you’ve trained them on how to use it, and make sure that you’re putting out valuable suggestions. I think that’s key.
Haziq Haque: We had broad support from the field almost right out of the gate. And I think there were a couple of things that we did differently, and I think in some cases — because of our size and working model — we didn’t have any pushback. We had, in fact, one of our leaders who’s helping us launch say, “This new functionality that we’re putting together and launching to the field, think of it as a virtual assistant.” We focused less on the AI piece of it as we rolled out to the field and the reps, because it makes this thing, from a user experience perspective, such a big concept to kind of wrap your head around. This idea of virtual assistant and that it’s nudging you in the right direction, really resonated, and it really helped with adoption and support for us. I think the other part was we knew our field enough to know that we had to start a little bit smaller. Partially because we were sensitive to this idea of, the reps already get all this information. How is this going to help? We limited the number and the volume of suggestions and insights that we were creating right out of the gate. And we said, “We’ll scale up later.” A combination of those things really helped us avoid any pushback. And I think to a degree with the reps working from home, what was really helpful from an adoption perspective was that they have more time to explore the function and the tools. And it’s creating good behavior now, where they can log into the iPad every day, look at these, dismiss, interact. And when their days get busier, when they start to roll out into the field, it’s a behavior that’s built in. When the adoption gets easier with reps and they get more comfortable with the technology, what kind of next steps do you want to take beyond what you have been doing with them? Haziq Haque: For us, it’s creating this environment of continuous improvement and feedback, assuring the reps that the more they put in, the more they can get out. I think that’s a key next action for us — making sure from a field perspective, whether at a home office or headquarters, they understand that. The marketing team, the head of sales, those guys get it. And now there’s this new tool for them to use. But I think from a field and at the rep level, it’s important for them to be able to come back and say, “I’ve got this great working algorithm that I’m finding great success with doctors in the field or accounts in the field.” They get to have input into the process, which is a great motivator to get feedback and continue with our program. I think next steps from an AI perspective is moving into the more advanced analytics areas. How do you take, say Veeva Data Cloud and leverage AI components there to derive insights so that we can do those longer-term research studies? That’s an area that I could foresee many of us as customers moving into. We’ve spoken a lot about building that data ecosystem as your next step. Anything to add there? I know GSK is really thinking not just about next best action, but beyond. Madhavi Ramakrishna: I think we’re going at this as an ecosystem.
We call it the integrated approach to making sure that we are not mushrooming these initiatives and not cannibalizing it. For example, we talk about digital marketing and in the same context talk about next best action. And we confuse the heck out of the folks on the other end. “What do you mean by next best action? How does this tie back to what we are doing on the digital engagement?” Making sure that we have an integrated approach to these initiatives that’ll all target the same persona is very critical. Number two is also, we have to recognize that as much as AI and machine learning is a fantastic way to do value delivery, it is also a cognitive element of your training at the end of the day. It only continues to deliver value as long as it is smarter than what a human can think of. What does that nice balance look like where we continue to build the trust and making sure that the adoption exists? And then of course it is also being applied and actioned on. That’s the critical part. Leading into the measurement, I don’t want to say it’s critical that every bit and piece is premised on AI and machine learning . Test and learn, and of course drive towards an outcome. What does that measurement framework look like? We cannot continue to put our head into the sand and not realize, “There’s no value here.” We have to quickly adjust, and course correct. What does that framework look like? What are the key KPIs that we need to track and audit? What do those simulations look like if we were to traverse them on an alternate path? Building that robustness around the platforms and tech, and second, of course, taking and tying our functional programs so we’re going at it with a very cohesive approach to try and deliver the top two or three business outcomes or value drivers.
As the suggestions and insights provide value to the rep, which they can then in turn use to bring value to their accounts, they’re happy to use it. They want to learn it.
Sal, you guys are actually launching a product during Covid. How does AI play into a launch these days? Sal Paolozza: Well actually, it plays very well into a launch. First of all, from our perspective, I think we’ve been very fortunate to have a lot of Veeva technology. That’s really helped the reps keep up their interaction with their own customers. At the same time, we’re still a small company and we’re taking on new products and we’re looking at different channels. One of the channels we’re looking at is virtual reps for some of our more mature brands. We were able to drop some virtual reps in empty territories. And they were effective from day one. They knew which customers they needed to call on, they knew what they needed to say to these customers. It’s really helped to keep up the activity in every territory during Covid, and even with empty territories. It’s been awesome. And we’re looking forward to rolling it out now to almost every brand. We’re actually working on our second brand, and we’ve got a third brand coming. And we’re looking at bringing in some of the data in and building some very interesting suggestions around it. It’s been great. Haziq Haque: I think for us, it’s a little bit of the same, but on a smaller scale. On an AI front, we’re able to execute Covid-specific insights a lot faster into the field. The Veeva CRM Approved Email template and use case had to do with a message around Covid-19. When we first started working with suggestions, it took one of our developers five weeks to turn around a suggestion before this program. To put together that sales algorithm was follow up with this approved email template. I think speed to implementation, feed of connectivity between marketing and sales execution, and then of course enabling, “This is the most important thing that you should consider today.” That AI driven prioritization — that’s value. We were able to react quickly to our business needs. And that’s as we’re wrapping up a pilot and we’re about to launch nationally, we’re able to do these types of changes. And I think that’s impactful. •