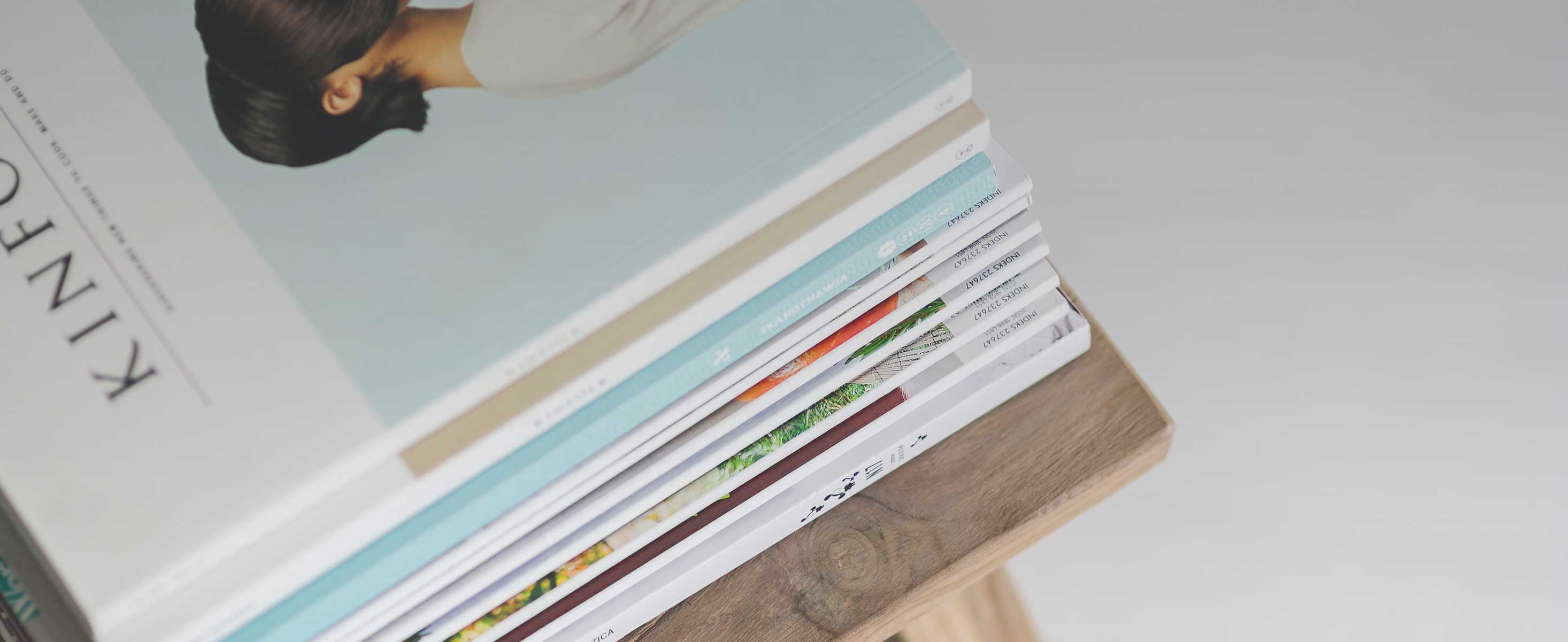
58 minute read
The Effect of Outcome Probability on Generalization in Predictive Learning Hadar Ram, Dieter Struyf, Bram Vervliet, Gal Menahem, and Nira Liberman
by Hogrefe
Hadar Ram 1
Advertisement
, Dieter Struyf 2
, Bram Vervliet 2,3
, Gal Menahem 1
, and Nira Liberman 1
1
School of Psychological Sciences, Tel Aviv University, Israel 2
Centre for the Psychology of Learning and Experimental Psychopathology, Leuven University, Belgium 3
Harvard Medical School, Massachusetts General Hospital, Boston, MA, USA
Abstract: People apply what they learn from experience not only to the experienced stimuli, but also to novel stimuli. But what determines how widely people generalize what they have learned? Using a predictive learning paradigm, we examined the hypothesis that a low (vs. high) probability of an outcome following a predicting stimulus would widen generalization. In three experiments, participants learned which stimulus predicted an outcome (S+) and which stimulus did not (S) and then indicated how much they expected the outcome after each of eight novel stimuli ranging in perceptual similarity to S+ and S. The stimuli were rings of different sizes and the outcome was a picture of a lightning bolt. As hypothesized, a lower probability of the outcome widened generalization. That is, novel stimuli that were similar to S+ (but not to S) produced expectations for the outcome that were as high as those associated with S+.
Keywords: generalization, predictive learning, partial reinforcement, learning from experience
The purpose of learning from experience is to enable prediction (De Houwer & Beckers, 2002b; Hohwy, 2013; Liberman, Trope, & Rim, 2011; Suddendorf & Corballis, 2007). When people repeatedly experience event A (e.g., gray clouds/a member of a specific social group) that is followed by outcome B (e.g., rain/the group member offers help), they learn to predict outcome B from event A (e.g., expect rain when seeing gray clouds/expect help from another member of the same group). They might learn that another event, C (e.g., white clouds/a member of a different social group), does not predict the same outcome. Prediction is useful because it enables preparing for the future (e.g., take an umbrella/choose whom to ask for help). Importantly, experience has to be generalized in order to apply to a new situation. That is, outcome predictions should be made for events that are similar but not identical to the original event A. An interesting question is that of generalization breadth, namely what determines the range of stimuli (e.g., the range of grayness/the range of group members) for which outcome B would be predicted.
The factors that affect generalization breadth have been studied mainly within the psychology of learning, but are clearly related also to central topics in social psychology, such as attitudes and stereotypes. For example, when we hear a great talk at a conference, we may not only form a positive attitude toward the speaker, but also generalize this attitude toward the speaker’s laboratory members, his/her discipline or even his/her national group, thereby changing, strengthening, or helping to create social stereotypes.
In the present article, we examine one factor that may affect generalization breadth, namely the probability that an outcome appears following a predicting stimulus. Specifically, we examine the hypothesis that low (vs. high) outcome probability (i.e., reinforcement) after a cue (S+) widens generalization. In the learning literature, this factor has been termed reinforcement rate. In what follows, we first present classic and contemporary models of learning which addressed the question of generalization as a function of reinforcement rate while also reviewing central relevant findings. We then turn to discuss why effects of reinforcement rate/outcome probability on generalization are important and elaborate why we believe that they are of interest for social psychology. Thereafter, we describe the predictive learning paradigm we use in our experiments and state our hypotheses in the more concrete terms of that paradigm.
Outcome Probability and Generalization in Classic and Contemporary Learning Models
In instrumental conditioning, when an organism is rewarded (reinforced) for a particular response in the presence of a stimulus, it is likely to exhibit the same response when encountering the same stimulus again. Continuous reinforcement occurs when reinforcement is delivered after every response of the organism to that stimulus, whereas partial reinforcement occurs when reinforcement is delivered only after some responses (Jenkins & Stanley, 1950). Both classic and more recent theories of learning predict that low probability of reinforcement (i.e., outcome probability) would give rise to wider generalization. Notable among these theories are Bayesian models of generalization (Gershman, Blei, & Niv, 2010; Gershman & Niv, 2012; Shepard, 1987; Soto, Gershman & Niv, 2014; Soto, Quintana, Pérez-Acosta, Ponce, & Vogel, 2015; Tenenbaum & Griffiths, 2001). Shepard (1987), for example, conceptualized generalization as a Bayesian inference problem: The learner experiences stimulus X (e.g., a cloud of specific grayness) with a particular consequence (e.g., rain) and assumes that stimulus X belongs to a “consequential region,” a region of stimuli that produce the same consequence. The learner’s task is to infer the probability that a novel stimulus Y (i.e., a darker cloud) belongs to the same consequential region. Generalization to Y represents the estimated probability that Y belongs to the same consequential region, given that X belongs to it. Tenenbaum and Griffiths (2001) extended Shepard’s analysis to generalization from multiple examples. They claimed that as the number of learned examples within the same consequential region increases, the learner will tend to infer a narrower consequential region and thus would exhibit narrower generalization. For example, a learner who experiences on three occasions that the clouds of grayness level 6 are followed by rain (relative to a learner who experiences it only once) will generalize less to clouds of grayness level 5. This is because learners assume that experiences are sampled randomly from the consequential region, and three similar sampled observations are indicative of a narrower region than only one observation. Thus, according to Bayesian models of generalization, a lower number of reinforcements would give rise to a wider consequential region and as a result produce wider generalization. That is, the generalization gradient to novel stimuli will be wider under partial reinforcement than under continuous reinforcement.
That low probability of reinforcement would give rise to wider generalization is also consistent with certain associative learning models. For example, elemental theories of conditioning, such as stimulus sampling theory (Atkinson
& Estes, 1963; Estes, 1950, 1959), conceptualize stimuli as set of elements. The core idea is that in each trial, only a subset of the elements of each stimulus is sampled (i.e., processed). By consequence, the same stimulus is perceived slightly differently in each trial, with a different subset of elements being processed. In reinforced trials, only the sampled elements become associated with the outcome. Generalization occurs when a new stimulus shares those elements that have been associated with the outcome (McLaren & Mackintosh, 2000; Welham & Wills, 2011). Reinforcement rate determines how the associative strength is distributed among the elements of the predictor stimulus and hence can determine the extent of generalization to a new stimulus.
Under full reinforcement, the sampling of elements is determined by their relative salience, such that the more salient elements of a predicting stimulus gain more associative strength with the outcome and each other (a process McLaren & Mackintosh, 2000 termed “unitization”: A relatively narrow set of elements gains relatively strong associative strength with the outcome). Because any novel stimulus will have a lower chance to specifically share that narrow set of elements, the generalization gradient will be narrow as well. Under partial reinforcement, on the other hand, the inherent unpredictability curbs the associative strength of the narrow set and leaves room for the other elements to gain some associative strength in occasionally reinforced trials. As a result, a larger set of elements acquires (some) associative strength with the outcome, thereby increasing the chances that a novel stimulus will share those elements. The result is a lower rate of response to generalization stimuli but wider generalization gradient.
Some studies supported the hypothesis that partial (vs. continuous) reinforcement would widen generalization in human learning. These studies, however, mainly addressed fear conditioning. For example, in some early studies (Humphreys, 1939; Wickens, Schroder, & Snide, 1954), a specific tone was paired with an electric shock and thus was the conditioned stimulus. Participants’ galvanic skin response to the sound of the tone was measured. In the generalization test phase, novel tones that differed in pitch in steps of just noticeable differences from the conditioned stimulus were presented. More generalization was found in the partial reinforcement group than in the continuous reinforcement group.
We think that the study of how outcome probability affects generalization is of great importance and relevance to how people understand their physical and social world and how
they behave in it. In our example, when rain always follows the appearance of clouds of grayness level 6 (high probability), then rain would be associated with this specific level of grayness. However, when rain only sometimes follows the appearance of clouds of grayness level 6 (low probability), then rain would probably not become associated with the specific level of grayness, but rather with a wider range of levels of gray. The learner in this example would assume a wider range of grayness values when making predictions and deciding on actions.
To use an example from the social world, when a member of a social group consistently offers help (high probability), observers would probably infer that he/she is kindhearted. However, if a member of a social group only sometimes offers help (low probability), observers would be more likely to form a milder positive evaluation that would apply to the entire social group.
Additionally, probability is viewed within Construal Level Theory (CLT; Liberman & Trope, 2008, 2014; Trope & Liberman, 2010) as a dimension of psychological distance, along with temporal, spatial, and social distances (Todorov, Goren, & Trope, 2007; Wakslak & Trope, 2009; Wakslak, Trope, Liberman, & Alony, 2006). If found, the hypothesized effect of outcome probability on generalization would open the possibility that temporal, spatial, and social distances would have similar effects. We will return to this point in the general discussion, when we also discuss how the present results may be viewed with the CLT framework.
As mentioned, while there are findings that lend initial support to our hypothesis that lower probability of an outcome after a learned stimulus will widen generalization, these findings are mainly related to the domain of human fear conditioning. We thought that because generalization is a basic process of attitude formation, it is important to examine this hypothesis also with more neutral, not fearrelated stimuli. To that end, we used a modified version of the predictive learning paradigm (Struyf, Iberico, & Vervliet, 2014), which we now turn to describe.
In the predictive learning paradigm (Struyf et al., 2014, Experiment 1), the participants’ goal is to learn which stimuli predict the appearance of an outcome. The stimuli are rings of different sizes, and the outcome is a picture of a lightning bolt. A medium ring (S+) is followed by the outcome, and a large ring (S) is never followed by the outcome. In each trial, one ring appears on the computer screen and participants indicate their prediction regarding the appearance of the lightning bolt on an 11-point rating scale, ranging from 0 (= certainly no lightning), via 5 (= uncertain), to 10 (= certainly lightning). Afterward, the ring and the scale disappear and the lightning bolt is either presented or not presented. Thus, participants learn from experience that S+ is followed by the outcome whereas S is not followed by the outcome. The paradigm includes two phases, the acquisition phase and the generalization phase. The acquisition phase presents S+ and S equally often: S+ is paired with the outcome whereas S is never paired with the outcome. At the generalization phase, eight novel rings varying in size are presented. The S+, being the middle-sized ring, is placed in the middle of the generalization dimension, whereas S, being the largest ring, is placed at the right edge. Half of the generalization rings are larger than S+ (i.e., between S+ and S). Responses to these rings are affected by both excitatory generalization from S+ (which would call for predicting the outcome) and inhibitory generalization from S (which would call for predicting no outcome). The other half of the generalization rings are smaller than S+ (i.e., on the side of S+ that is opposite to S). This allows us to test for generalization from S+ that is less influenced by generalization from S (McLaren & Mackintosh, 2002; Pearce, 1987; Spence, 1937). 1
Our hypothesis concerned these latter generalization rings.
We introduced into this paradigm a manipulation of outcome probability by varying the probability of the outcome following S+ during the acquisition phase. In the high-probability condition, this probability was 83% whereas, in the low-probability condition, it was 42%. We operationalized generalization as a tendency to predict the outcome after a generalization ring smaller than the original learned ring (S+), that is, generalization rings that are on the side of S+ that is opposite to S. As mentioned, these generalization rings reflect generalization mostly from S+ and less from S. We hypothesized that low (vs. high) probability of the outcome would cause higher predictions for rings smaller than S+ (i.e., on the side of S+ that is opposite to S). Paradigms of discrimination leaning typically confound outcome probability given S+ with contingency between stimulus and outcome, such that low outcome probability given S+ coincides with lower contingency between
1
Learning situations that include both S+ and S (often referred to as discrimination learning) tend to give rise to a peak shift effect, whereby response is most frequent not to the learned stimulus itself, but rather to a generalization stimulus next to S+, on the side opposite to S. Discussing this effect is beyond the scope of the current article (for reviews, see Honig & Urcuioli, 1981; Purtle, 1973, Spence, 1937; Struyf et al., 2014).
stimulus (S+ vs. S) and outcome (outcome vs. no outcome). Because contingency might affect learning (Allan, 1980; De Houwer & Beckers, 2002a; Shanks, 1995), we need to examine whether higher generalization might, in fact, be the result of low contingency rather than low conditional probability (of the outcome given S+). We would like to discuss this in light of the results and thus defer our answer to that question until the general discussion.
Experiment 1
Experiment 1 was designed to test how generalization is affected by outcome probability. This was achieved by using a modified version of the predictive learning paradigm described previously. In the high-probability condition, S+ was presented 12 times during the acquisition phase. Ten out of its presentations were followed by the outcome (83%). In the low-probability condition, S+ was also presented 12 times during the acquisition phase, but only five out of its presentations were followed by the outcome (42%). At the generalization phase, which was similar for both conditions, the S+, S, and eight generalization rings appeared multiple times. The S+ was reinforced in 50% of its presentation. This feature was part of the original procedure and was intended to counter extinction during generalization. We followed the suggestion of Vervliet, Iberico, Vervoort, and Baeyens (2011) and analyzed the first block of the generalization phase, which shows effects of learning that are relatively clean of extinction (but suffer from a low number of trials), and only then moved to examine the entire generalization phase. 2
We hypothesized that in the first generalization block, participants would predict the outcome following novel rings that are on the side of S+ opposite to S, in the low-probability condition more than in the high-probability condition.
Method
Participants Seventy undergraduates from Tel Aviv University participated in the experiment in return for payment. The sample size was based on previous studies with the same paradigm (Struyf et al., 2014). Participants were randomly assigned to experimental conditions. To test whether each participant learned to differentiate between S+ and S, we computed the difference between the last two S+ and the last two S trials in the acquisition phase. A lower score indicated less differentiation between S+ and S. Eleven participants were excluded because their difference score was below 1 (i.e., they failed to learn the difference between S+ and S by the end of the acquisition phase). The final sample consisted of 59 participants (M age = 23.61, 47 women) N high-probability = 30, N low-probability = 29.
Stimuli The experimental stimuli were 10 rings varying in size. The diameter of the smallest ring (R1) was 2.00 cm, and each successive ring’s diameter increased by approximately 15% (R2: 2.30 cm, R3: 2.60 cm, R4: 2.90 cm, R5: 3.20 cm, R6; 3.50 cm, R7: 3.80 cm, R8: 4.10 cm, R9: 4.40 cm, R10: 4.70 cm). The intermediate-size ring (R5) served as S+, and the largest ring (R10) served as S. 3
All the other rings served as generalization stimuli. The outcome was a drawing of a white lightning bolt on a black background. All stimuli were presented on a computer screen that was placed in front of the participants.
Procedure First, participants signed a consent form. Then, the experiment began with an instruction screen, in which participants were informed that a number of figures would appear on the screen and that some of these figures would be followed by a lightning bolt. They were told that their goal was to learn which figure would be followed by the lightning bolt.
The experimental procedure included two phases. The first phase was acquisition, in which S+ and S were each presented 12 times. The number of times that S+ was followed by the outcome changed according to the conditions: S+ was followed by the outcome in 10 of its presentations in the high-probability condition (83%), but only in five of its presentations in the low-probability condition (42%). None of the presentations of S were followed by the outcome. The generalization phase was identical in the two conditions: It consisted of six identical blocks. In each block, there were two presentations of S+ (one of which was followed by the outcome), two presentations of S, and one presentation of each of the eight generalization rings. The generalization rings and S were never followed by the outcome. Each trial started with a computer screen that said: “The next trial starts now.” Then, one stimulus was presented for 500 ms, and a rating scale appeared at the bottom of the screen, ranging from 0 (= certainly no lightning), via 5 (= uncertain), to 10 (= certainly lightning). Participants indicated their prediction on the scale by clicking on it with the
2
We will return and elaborate on the issue of extinction in the Discussion section. 3
We did not counterbalance the S to be either the smallest or the largest ring, to prevent participants form inferring a rule such as “the larger the ring the higher the probability of the outcome appearance.” Therefore, S was always the largest ring (Ghirlanda & Enquist, 2003; Struyf et al., 2014).
0 1 2 3 4 5 6 7 8 9 10 Predictions High-probability S+ Low-probability S+
High-probability S− Low-probability S−
1 2 3 4 5 6 7 8 9 10 11 12 Trials
Figure 1. Mean predictions (on a 0–10 scale) during the acquisition phase by outcome probability, trial number, and stimulus type. Error bars depict standard errors.
computer mouse. Afterward, the stimulus and the scale disappeared and the lightning bolt was either presented for 1,500 ms or was not presented at all. The inter-trial interval was always 3 s. Upon completing the task, participants responded to the following questions, which served as control variables: interest (“How interesting was the task for you?”), enjoyment (“How much did you enjoy the task?”), difficulty (“How difficult did you find the task?”), motivation (“How motivated did you feel to perform the task well?”), importance (“How important was it for you to perform the task well?”), and perceived competence (“How well do you feel that you did on the task?”) on scales that ranged from 1 (= not at all) to 7 (= very much). General mood was also assessed (“Generally, how do you feel right now?” 1 = very bad, 7 = very good), followed by eight specific emotions (“How sad/loose/tense/relaxed/nervous/happy/ joyful/depressed do you feel right now?” 1 = not at all, 7 = very much). Finally, participants answered a demographic questionnaire.
Results
The raw data (of all three experiments) including analysis script are provided in the Electronic Supplementary Materials, ESM 1–4.
Acquisition We analyzed the outcome predictions in a 12 2 2 mixed-design analysis of variance (ANOVA), with trial (12) and stimulus type (S vs. S+) as within-subject factors and outcome probability (high-probability vs. low-probability) as a between-subject factor. There was a main effect of trial, F(11, 627) = 2.77, p = .002, η 2 p = .05, and a main effect of stimulus type, F(1, 57) = 385.80, p < .001, η 2 p = .87, which showed that participants predicted the outcome more after S+ than after S. That is, participants learned to differentiate between S+ and S. An interaction between stimulus type and trial, F(11, 627) = 54.79, p < .001, η 2 p = .49, showed that the difference between the predictions for S+ and S developed over trials (Figure 1). A main effect of outcome probability, F(1, 57) = 14.06, p < .001, η 2 p = .20, was qualified by an interaction with stimulus type, F(1, 57) = 25.29, p < .001, η 2 p = .31, which indicated that participants’ predictions for S+ reflected the actual probability of its appearance, which was higher in the high-probability condition than in the low-probability condition. 4
The interaction between trial and outcome probability was not significant, F(11, 627) = 0.87, p = .57. The three-way interaction between stimulus type, trial, and outcome probability, F(11, 627) = 5.10, p < .001, η 2 p = .08, indicated that the difference in the outcome predictions between the two conditions did not exist initially, but rather emerged over trials.
Generalization Generalization is defined as giving similar conditioned response to a novel stimulus as to the learned stimulus (Shepard, 1958; for a review see Ghirlanda & Enquist, 2003). In other words, we were interested in examining the response to the novel stimuli relative to the response to the original, learned stimulus. We thus computed the difference between the predictions given to each stimulus presented during the generalization phase to the predictions given to S+ at the last two trials in the acquisition phase (for a similar conceptualization of generalization, see
Predictions minus last two S+ trials -9 -8 -7 -6 -5 -4 -3 -2 -1 0 1 Low-probability High-probability
R1 R2 R3 R4 S+ R6 R7 R8 R9 SStimuli
Figure 2. Mean generalization scores for all stimuli during the first generalization block by outcome probability. Error bars depict standard errors.
Blough, 1975; Fazio, Shook, & Eiser, 2004; Hovland, 1937). A zero difference score indicated that the prediction for a novel stimulus was similar to S+. A lower difference score indicated lower predictions of the outcome and thus lower generalization. We used the same dependent variable in this and all subsequent studies in this paper. We analyzed generalization scores in a 10 2 mixeddesign ANOVA with stimuli (10: R1, R2, R3, R4, S+, R6, R7, R8, R9, S) as a within-subject factor and outcome probability (high-probability vs. low-probability) as a between-subject factor. As explained above, we first analyzed the first block of generalization and then moved to examine the entire generalization phase (see Vervliet et al., 2011 for a similar procedure).
First Generalization Block A main effect of stimuli, F(9, 513) = 41.5, p < .001, η 2 p = .42, indicated that the outcome was predicted after some stimuli more than after other stimuli. A main effect of outcome probability, F(1, 57) = 10.07, p = .002, η 2 p = .15, indicated that generalization scores in the low-probability condition were higher (M = 2.99, SD = 1.98) than in the highprobability condition (M = 4.49, SD = 1.64) (see Figure 2). There was no interaction between stimuli and outcome probability, F(9, 513) = 1.69, p = .09. To examine our hypothesis, we analyzed the generalization scores only for the generalization stimuli located on the side of S+ opposite to S (i.e., rings smaller than S+). We conducted a 4 2 mixed-design ANOVA with similarity to S+ (four levels, from most similar to S+ to least similar to S+), as within-subject factor and outcome probability (high-probability vs. low-probability) as a between-subject factor. The hypothesized effect of outcome probability, F(1, 57) = 6.64, p = .013, η 2 p = .10, indicated that generalization scores in the low-probability condition (M = 1.39, SD = 2.93) were higher than in the high-probability condition (M = 3.17, SD = 2.35). A main effect of similarity to
S+, F(3, 171) = 5.56, p = .001, η 2 p = .09, showed that generalization scores varied across the different generalization stimuli. The interaction between similarity and outcome probability was not significant F(3, 171) = 1.77, p = .150.
All Generalization Blocks We repeated the analysis for all six generalization blocks. The 10 stimuli (R1, R2, R3, R4, S+, R6, R7, R8, R9, S) 2 outcome probability (high-probability vs. low-probability) ANOVA revealed a significant main effect of outcome probability, F(1, 57) = 15.56, p < .001, η 2 p = .21, demonstrating that generalization scores in the low-probability condition were higher (M = 4.12, SD = 2.20) than in the highprobability condition (M = 6.10, SD = 1.62). A significant main effect of stimuli, F(9, 513) = 70.55, p < .001, η 2 p = .55, indicated that the outcome was predicted after some stimuli more than after other stimuli. There was no interaction between stimuli and outcome probability, F(9, 513) = 0.70, p = .700 (Figure 3). To examine our hypothesis, we analyzed the generalization scores only for the generalization stimuli located on the side of S+ opposite to S. We conducted a 4 2 mixed-design ANOVA with similarity to S+ (four levels, from most similar to S+ to least similar to S+), as withinsubject factor and outcome probability (high-probability vs. low-probability) as a between-subject factor. In line with our hypothesis, a significant effect of outcome probability, F(1, 57) = 12.41, p = .001, η 2 p = .18, indicated that generalization scores in the low-probability condition were higher (M = 3.49, SD = 2.71) than in the high-probability condition (M = 5.61, SD = 1.84). A main effect of similarity, F(3, 171) = 45.56, p < .001, η 2 p = .44, showed that generalization scores for these generalization stimuli decreased as the similarity to S+ decreased. The interaction between similarity and outcome probability was not significant F(3, 171) = 1.73, p = .160.
Predictions minus last two S+ trials -9 -8 -7 -6 -5 -4 -3 -2 -1 0 1 Low-probability High-probability
Mean R1 Mean R2 Mean R3 Mean R4 Mean S+ Mean R6 Mean R7 Mean R8 Mean R9 Mean SStimuli
Figure 3. Mean generalization scores for all stimuli during all generalization blocks by outcome probability. Error bars depict standard errors.
There were no significant differences between the two outcome probability conditions in any of the mood measures or other measures. 5
Discussion
Experiment 1 examined the effect of outcome probability on generalization by manipulating the probability of the outcome after a predicting stimulus. Participants’ learning accurately reflected the experimental conditions, such that in the high-probability condition, participants predicted the outcome after S+ with more certainty than participants in the low-probability condition. Importantly, the generalization results were consistent with our hypothesis. Participants in the low-probability condition showed wider generalization than participants in the high-probability condition, by making predictions more similar to S+ after seeing a novel predictor that was similar to S+ (but not to S). These results were apparent not only in the first block, but also when we analyzed all of the generalization blocks.
Note that in this Experiment, the high-probability and low-probability conditions did not only differ in the probability of the outcome appearance after S+, but also differed in the number of pairings between S+ and the outcome. In Experiment 2, we controlled for this possible confound by including two low-probability conditions: one in which, similar to Experiment 1, the overall number of acquisition trials in the low-probability condition was similar to the high-probability condition (but naturally, the number of pairings between S+ and the outcome was lower), and one in which the number of pairings between S+ and the outcome was equated between the low-probability and the high-probability conditions (as a consequence, there were more acquisition trials in this low-probability condition than in the high-probability condition).
Experiment 2
We replicated Experiment 1 but included an additional lowprobability condition that equated the number of pairings of S+ and the outcome between the low-probability and the high-probability conditions. This new condition, which we term the “low-probability-long” condition, had twice the number of acquisition trials as the high-probability and the original low-probability conditions. In this “low-probability-long” condition, the overall number of pairings of S+ and the outcome matched the high-probability condition. However, the probability of the outcome appearance following S+ was still low (42%) and thus matched the original low-probability condition in Experiment 1. We term the original low-probability condition that is similar to that of Experiment 1 the “low-probability-short” condition. As in Experiment 1, we hypothesized that low outcome probability will widen generalization.
5
Although some directional differences emerged, they did not survive a Bonferroni correction for multiple comparisons. Moreover, such differences did not emerge in Experiments 2 and 3 and will not be discussed any further. ESM 5 presents a full report of the descriptive and inferential statistics of these measures. Importantly, when these measures were entered as covariates, they did not reduce the effect of outcome probability, nor did they interact with outcome probability, neither in the analysis of the first generalization block nor in the analysis of all blocks.
0 1 2 3 4 5 6 7 8 9 10
1 2 3 4 5 6 7 8 9 10 11 12 13 14 15 16 17 18 19 20 21 22 23 24 Predictions Trials Low-probability-long S+ Low-probability-long S− Low-probability-short S+ Low-probability-short S–High-probability S+ High-probability S−
Figure 4. Mean predictions (on a 0–10 scale) during the acquisition phase by outcome probability, trial number, and stimulus type. Error bars depict standard errors.
Method
Participants Ninety-one undergraduates from Tel Aviv University participated in the experiment voluntarily. The sample size was chosen according to previous studies with the same paradigm (Struyf et al., 2014) as well as according to Experiment 1. Participants were randomly assigned to one of the three conditions. One participant was excluded because he did not finish the task, and another participant was excluded because she wrote down the stimuli and their outcomes for herself during the task. As in Experiment 1, to test whether each participant learned to differentiate between S+ and S, we computed the difference between the last two S+ trials and the last two S trials in the acquisition phase. Twenty participants were excluded because their difference score was below 1. The final sample consisted of 69 participants (M age = 25.41, 40 women) N high-probability = 25, N low-probability-short = 23, N low-probabilitylong = 21:
Procedure As in Experiment 1, participants first signed a consent form and read the task instructions on the computer screen. During acquisition, S+ and S were each presented 12 times in the high-probability and low-probability-short conditions and 24 times in the low-probability-long condition. S+ was followed by the outcome 10 times in the high-probability condition (83%) and in the low-probability-long condition (42%) but only five times in the low-probability-short condition (42%). S was never followed by the outcome. The generalization phase was identical for all three conditions and was similar to Experiment 1. After completing the task, participants responded to the same control and demographic questions as in Experiment 1.
Results
Acquisition We analyzed outcome predictions in a 12 2 3 mixeddesign ANOVA with trial (12) and stimulus type (S vs. S+) as within-subject factors and outcome probability (high-probability vs. low-probability-short vs. low-probability-long) as a between-subject factor. Since the low-probability-long condition had 24 trials, only the first 12 trials of this condition were analyzed. A main effect of stimulus type, F(1, 66) = 352.95, p < .001, η 2 p = .84, showed that participants learned to differentiate between S+ and S, namely they predicted the outcome more after S+ than after S. An interaction between stimulus type and trial, F(11, 726) = 32.01, p < .001, η 2 p = .33, showed that the difference between the predictions to S+ and S developed over trials. A main effect of outcome probability, F(2, 66) = 8.64, p < .001, η 2 p = .21, was qualified by an interaction with stimulus type, F(2, 66) = 8.13, p = .001, η 2 p = .20, which indicated that participants’ predictions accurately reflected the experimental condition. Specifically, predictions were higher in the high-probability condition than in the two low-probability conditions. Indeed, a simple effect analysis revealed that for S+ predictions were higher in the high-probability condition than in the lowprobability-short condition, p < .001, and the low-probability-long condition, p = .001, which did not differ from each other, p = .190 (Figure 4). An interaction between outcome probability and trials, F(22, 726) = 2.37, p < .001, η 2 p = .67, indicated that the differences in predictions between the three outcome probability conditions emerged over trials. The three-way interaction between stimulus type, trial, and outcome probability was not significant, F(22, 726) = 1.30, p = .160. We were also interested in comparing performance at the end of the acquisition phase between the three outcome
R1 R2 R3 R4 S+ R6 R7 R8 R9 SStimuli
Figure 5. Mean generalization scores for all stimuli during the first generalization block by outcome probability. Error bars depict standard errors.
probability conditions. Therefore, we analyzed the mean outcome predictions in the last two S+ trials and the last two S trials in a 2 3 mixed-design ANOVA with stimulus type (S vs. S+) as a within-subject factor and outcome probability (high-probability vs. low-probability-short vs. low-probability-long) as a between-subject factor. A main effect of stimulus type, F(1, 66) = 575.00, p < .001, η 2 p = .90, indicated that participants learned to differentiate between S+ and S. A significant effect of outcome probability, F(2, 66) = 11.49, p < .001, η 2 p = .26, was qualified by an interaction between stimulus type and outcome probability, F(2, 66) = 4.77, p = .012, η 2 p = .13, which suggested that at the end of the acquisition phase, the difference between conditions in actual probability of outcomes was reflected in participants’ predictions. Indeed, a simple effect analysis revealed that for S+ predictions were higher in the high-probability condition than in the low-probability-short condition, p = .007, and the lowprobability-long condition, p < .001, which did not differ from each other, p = .160.
Generalization First Generalization Block As in Experiment 1, generalization scores were computed by subtracting participants’ predictions for the last two S+ trials in the acquisition phase from the predictions in the generalization phase. First, we analyzed these generalization scores in a 10 3 mixed-design ANOVA with stimuli (10: R1, R2, R3, R4, S+, R6, R7, R8, R9, S) as a withinsubject factor and outcome probability (high-probability vs. low-probability-short vs. low-probability-long) as a between-subject factor. There was no effect of outcome probability, F(2, 66) = 0.49, p = .620. A significant effect of stimuli, F(9, 594) = 30.4, p < .001, η 2 p = .31, was qualified
by an interaction between stimuli and outcome probability, F(18, 594) = 1.7, p = .035, η 2 p = .05. This interaction indicated that generalization scores in the two low-probability conditions were higher than in the high-probability condition only for stimuli smaller than S+ (on the side of S+ opposite to S) but not for stimuli larger than S (located between S+ and S) (Figure 5). To examine our hypothesis, we analyzed the generalization scores only for the generalization stimuli located on the side of S+ that is opposite to S. We conducted 4 3 mixed-design ANOVA with similarity to S+ (four levels, from most similar to S+ to least similar to S+), as a withinsubject factor and outcome probability (high-probability vs. low-probability-short vs. low-probability-long) as a between-subject factor. There was a main effect of similarity to S+, F(3, 198) = 4.51, p = .004, η 2 p = .06, which indicated that generalization scores varied across the different generalization stimuli. There was a significant effect of outcome probability, F(2, 66) = 5.19, p = .008, η 2 p = .14. Consistent with our hypothesis, a planned contrast analysis confirmed that generalization was higher in the two lowprobability conditions (M = 0.85, SD = 2.92) than in the high-probability condition (M = 2.86, SD = 2.38) F(1, 66) = 9.38, p = .003. Also as predicted, there was no significant difference between the two low-probability conditions F(1, 66) = 1.18, p = .280. The interaction between similarity and outcome probability was not significant, F(6, 198) = 0.33, p = .920.
All Generalization Blocks As in Experiment 1, we repeated the generalization analyses for all six generalization blocks. Specifically, we analyzed the generalization scores in a 10 3 mixeddesign ANOVA with stimuli (10: R1, R2, R3, R4, S+, R6,
Figure 6. Mean generalization scores for all stimuli during all generalization blocks by outcome probability. Error bars depict standard errors.
R7, R8, R9, S) as a within-subject factor and outcome probability (high-probability vs. low-probability-short vs. low-probability-long) as a between-subject factor. A significant main effect of stimuli, F(9, 594) = 60.60, p < .001, η 2 p = .48, indicated that the outcome was predicted after some stimuli more than after others. A main effect of outcome probability, F(2, 66) = 3.44, p = .038, η 2 p = .09, indicated that generalization scores in the two low-probability conditions were higher than in the high-probability condition for all stimuli. There was no interaction between stimuli and outcome probability, F(18, 594) = 1.29, p = .190 (Figure 6). To examine our hypothesis, we analyzed the generalization scores only for the generalization stimuli which were located on the side of S+ opposite to S. We conducted a 4 3 mixed-design ANOVA with similarity to S+ (four levels, from most similar to S+ to least similar to S+), as within-subject factor and outcome probability (highprobability vs. low-probability-short vs. low-probabilitylong) as a between-subject factor. As before, there was a main effect of similarity, F(3, 198) = 28.78, p < .001, η 2 p = .30, which indicated that generalization scores for these generalization stimuli varied. The effect of outcome probability was significant, F(2, 66) = 4.68, p = .013, η 2 p = .12. A planned contrast analysis between the two low-probability conditions and the high-probability condition indicated that, as hypothesized, the generalization scores in the two low-probability conditions were higher (M = 2.86, SD = 2.34) than the generalization scores in the high-probability condition (M = 4.68, SD = 2.49), F(1, 66) = 8.2, p = .006, with no difference between the two low-probability condition, F(1, 66) = 1.33, p = .250. The interaction between similarity and outcome probability was not significant F(6, 198) = 0.71, p = .640.
There were no significant differences between the three conditions in any of the mood measures or any of the control measures. Table 2 in ESM 5 presents the complete descriptive and inferential statistics for these measures.
Discussion
Experiment 2 replicated Experiment 1 with another lowprobability condition. In both low-probability conditions, the probability of the outcome after S+ was lower (42%) compared to the high-probability condition (83%). However, in the low-probability-short condition, the number of pairings between S+ and the outcome was half that of the high-probability condition (but the overall number of trials was similar), whereas, in the low-probability-long condition, it was similar to the high-probability condition (but the overall number of trials was doubled). Importantly, these two low-probability conditions yielded very similar results, at both acquisition and generalization. At acquisition, participants gave higher predictions for S+ in the highprobability condition than in the two low-probability conditions, accurately reflecting the higher probability of the outcome appearance in the high-probability condition. At generalization, participants in both low-probability conditions, compared to those in the high-probability condition, generalized more broadly, by making predictions that were more similar to what they predicted for S+ for novel rings that were located next to S+ on the side opposite to S. There was no difference between the two low-probability conditions. These results suggest that reduced probability of the outcome appearance, rather than reduced number of reinforcements, is responsible for broadening generalization. These results are consistent with our hypothesis and fully replicate Experiment 1.
Given the current design of Experiments 1 and 2, our hypothesis concerned only part of the generalization stimuli, namely only the novel stimuli that are not located between S+ and S. To investigate the effect of outcome probability on the entire generalization gradient, we conducted Experiment 3, in which S differed from S+ by shape rather than by size.
Experiment 3
Experiment 3 replicated Experiment 1, but we replaced S with a square the same size as S+. Thus, the relevant dimension to learning was the shape of the figure whereas the relevant dimension to generalization was the size of the ring. As a result, the inhibitory generalization from S to the novel rings no longer existed. Generalization for novel rings should be made only from the S+ (i.e., excitatory generalization), regardless of side, that is, regardless of whether the new ring was larger or smaller than S+ (Spence, 1937; McLaren & Mackintosh, 2002). As in Experiments 1 and 2, we hypothesized that outcome probability (low probability) would widen generalization. Unlike Experiments 1 and 2, the setup of Experiment 3 allowed us to examine the entire generalization gradient. We thus hypothesized that in the low-probability condition more than in the highprobability condition, participants would give higher predictions for the outcome following novel generalization rings, whether they were smaller or larger than S+.
Method
Participants One hundred undergraduates from Tel Aviv University participated in the experiment in return for payment (N = 86) or credit points (N = 12). Because we introduced an important change to the paradigm, we increased the sample size according to the recommendation of Ledgerwood (2015) for experiments with unknown effects. We planned to recruit 50 participants per experimental condition. Participants were randomly assigned to one of the two conditions. One participant was excluded because he did not finish the task, and another participant was excluded because he answered a phone call during the task. As in the previous experiments, to test whether each participant learned to differentiate between S+ and S, we computed the difference between the last two S+ trials and the last two S trials in the acquisition phase. Twelve participants were excluded because their difference score was below 1. The final sample consisted of 86 participants (M age = 24.19, 58 women) N high-probability = 43, N low-probability = 43. 33
Stimuli and Procedure The experimental procedure and stimuli were similar to Experiment 1, except that S was a square similar in size to S+ (a 3.20 cm rib compared to a circle with a 3.20 cm diameter in Experiment 1).
Results
Acquisition We analyzed the outcome predictions in a 12 2 2 mixeddesign ANOVA with trial (12) and stimulus type (S vs. S+) as within-subject factors and outcome probability (highprobability vs. low-probability) as a between-subject factor. There was a main effect of trial, F(11, 924) = 4.63, p < .001, η 2
p=.05. A main effect of stimulus type, F(1, 84) = 652.81, p < .001, η 2 p = .89, showed that participants predicted the outcome more after S+ than after S; that is, participants learned to differentiate between S+ and S. An interaction between stimulus type and trial, F(11, 924) = 55.26, p < .001, η 2 p = .40, showed that the difference between the predictions to S+ and S developed over trials. A main effect of outcome probability, F(1, 84) = 14.57, p < .001, η 2 p = .15, was qualified by an interaction between outcome probability and stimulus type, F(1, 84) = 27.69, p < .001, η 2 p = .25, which indicated that as could be expected, participants in the high-probability condition, predicted the outcome after S+ with more certainty than participants in the low-probability condition. A significant interaction between trial and outcome probability, F(11, 924) = 1.96, p = .030, η 2 p = .02, indicated that the difference in the predictions between the two outcome probability conditions increased over trials. The three-way interaction between stimulus type, trial, and outcome probability was not significant, F(11, 924) = 1.64, p = .082 (Figure 7).
Generalization First Generalization Block As in the previous experiments, generalization scores were computed by subtracting participants’ predictions for the last two S+ trials in the acquisition phase from the predictions in the generalization phase. We first analyzed these generalization scores in a 10 2 mixed-design ANOVA with stimuli (10: R1, R2, R3, R4, S+, R6, R7, R8, R9, S) as a within-subject factor and outcome probability (highprobability vs. low-probability) as a between-subject factor. There was an effect of outcome probability, F(1, 84) = 12.17, p = .001, η 2 p = .13, demonstrating that generalization scores in the low-probability condition were higher (M = 2.12, SD = 3.45) than in the high-probability condition (M = 4.02, SD = 3.71). A significant effect of stimuli, F(9, 756) = 22.02, p < .001, η 2 p = .21, indicated that the outcome was predicted more after some stimuli than others
Low-probability S− High-probability S−
1 2 3 4 5 6 7 8 9 10 11 12 Trials
Figure 7. Mean predictions (on a 0–10 scale) during the acquisition phase by outcome probability, trial number, and stimulus type. Error bars depict standard errors.
R1 R2 R3 R4 S+ R6 R7 R8 R9 SStimuli
Figure 8. Mean generalization scores for all stimuli during the first generalization block by outcome probability. Error bars depict standard errors.
(Figure 8). The interaction between stimuli and outcome probability was not significant, F(9, 594) = 1.7, p = .080. To examine our hypothesis, we analyzed the generalization scores in a 2 4 2 mixed-design ANOVA with both side of the gradient (bigger than S+ vs. smaller than S+) and similarity to S+ (four levels, from most similar to S+ to least similar to S+), as within-subject factors and outcome probability (high-probability vs. low-probability) as a betweensubject factor. The results revealed a main effect of outcome probability, F(1, 84) = 11.84, p < .001, η 2 p = .12, and no effect of side of the gradient, F(1, 84) = 0.03, p = .860, indicating that as hypothesized, generalization scores in the
low-probability condition (M = 1.55, SD = 3.23) were higher than in the high-probability condition (M = 3.34, SD = 3.50) along the entire generalization gradient, that is, for stimuli both smaller and larger than S+. A main effect of similarity to S+, F(3, 252) = 7.57, p < .001, η 2 p = .08, indicated that generalization scores varied across the different generalization stimuli. All other interactions were not significant; side of the gradient outcome probability, F(1, 84) = 1.20, p = .280, outcome probability similarity to S+, F(3, 252) = 1.11, p = .350, side of the gradient similarity to S+, F(3, 252) = 1.39, p = .250, side of the gradient outcome probability similarity to S+, F(3, 252) = 2.06, p = .110.
Figure 9. Mean generalization scores for all stimuli during all generalization blocks by outcome probability. Error bars depict standard errors.
All Generalization Blocks We repeated the analyses for all six generalization blocks. A 10 (stimuli: R1, R2, R3, R4, S+, R6, R7, R8, R9, S) 2 outcome probability (high-probability vs. low-probability) ANOVA revealed a significant main effect of outcome probability, F(1, 84) = 22.90, p < .001, η 2 p = .21, demonstrating more generalization in the low-probability condition (M = 3.42, SD = 2.64) than in the high-probability condition (M = 5.67, SD = 2.63). An effect of stimuli, F(9, 756) = 45.37, p < .001, η 2 p = .35, was qualified by a marginally significant interaction between stimuli and outcome probability, F(9, 756) = 1.85, p = .056, η 2 p = .02, which indicated that the effect of outcome probability was stronger as similarity to S+ decreased (Figure 9). To examine our hypothesis, we analyzed the generalization scores in a 2 4 2 mixed-design ANOVA with both side of the gradient (opposite to S vs. between S+ and S) and similarity to S+ (four levels, from most similar to S+ to least similar to S+) as within-subject factors and outcome probability (high-probability vs. low-probability) as a between-subject factor. The results revealed a main effect of outcome probability, F(1, 84) = 22.66, p < .001, η 2 p = .21, and no effect of side of the gradient F(1, 84) = 2.04, p = .16, indicating that as hypothesized, generalization scores in the low-probability condition (M = 2.91, SD = 2.35) were higher than in the high-probability condition (M = 4.95, SD = 2.39) along the entire generalization gradient. There was a main effect of similarity to S+, F(3, 252) = 44.18, p < .001, η 2 p = .34, which was qualified by an interaction between similarity to S+ and side of the gradient, F(3, 252) = 5.98, p = .001, η 2 p = .07, indicating lower generalization scores for stimuli less similar to S+, especially for stimuli that were larger than S+. All other interactions were not significant; Side of the gradient outcome probability, F(1, 84) = 2.04, p = .070, Outcome probability similarity to S+,
F(3, 252) = 2.00, p = .110, Side of the gradient outcome probability similarity to S+, F(3, 252)=.69, p = .560. There were no significant differences between the two conditions in any of the mood measures or any of the control measures. Table 3 in ESM 5 presents the complete descriptive and inferential statistics for these measures.
Discussion
Experiment 3 replicated Experiment 1 with S as a stimulus from a different category. During training, the relevant dimension was the shape (a ring as S+ vs. a square as S) whereas, during generalization, the relevant dimension was the size of the ring, as all the generalization stimuli were rings of varying size. Because S did not belong to the generalization dimension, there was no inhibitory generalization from S to novel stimuli, and generalization to novel stimuli, both smaller and larger than S+, was only made from S+ (McLaren & Mackintosh, 2002; Spence, 1937). As in Experiments 1 and 2, during acquisition, participants learned to predict the outcome appearance according to the experimental conditions. In the high-probability condition, participants predicted the outcome after S+ with more certainty than in the low-probability condition. Most importantly, during generalization, generalization was higher along the entire generalization gradient for participants in the low-probability condition, compared to those in the high-probability condition. These results are consistent with our hypothesis.
General Discussion
Three experiments examined the hypothesis that low probability of an outcome following a cue would widen generalization. This hypothesis is consistent with associative and
Bayesian theories of learning and generalization (Blough, 1975; Gershman & Niv, 2012; Shepard, 1987; Soto et al., 2014, 2015; Tenenbaum & Griffiths, 2001). To test our hypothesis, we used a predictive learning paradigm. Experiment 1 introduced two conditions: the highprobability condition and the low-probability condition, which matched the high-probability condition in the number of acquisition trials. We found wider generalization in the low-probability condition than in the high-probability condition. Experiment 2 extended Experiment 1 by adding a second low-probability condition, in which the number of times the outcome followed the predicting stimulus S+ was similar to the high-probability condition, but the outcome probability was still as low as in the original low-probability condition. Experiment 2 replicated Experiment 1 and further supported our hypothesis that low outcome probability increases generalization. Furthermore, there were no differences between the two low-probability conditions in the generalization phase, suggesting that the probability of the outcome after the cue rather than the number of their pairings widens generalization. In Experiment 1 and 2, the relevant dimension to both learning and generalization was the same, namely the size of the ring. Specifically, S+ which predicted the appearance of the outcome was a medium ring, whereas S which predicted the absence of the outcome was a large ring. The generalization stimuli were rings of different sizes, such that half of them were between S+ and S and thus could be affected by both generalization from S+ and generalization from S. The other half of the generalization stimuli were placed on the side of S+ which was opposite to S. Because these latter generalization stimuli were less influenced by generalization from S, our hypothesis concerned primarily these stimuli. Experiment 3 allowed us to examine the effect of outcome probability on generalization for all of the generalization stimuli by using S from a different category (i.e., a square) that was not supposed to affect generalization (McLaren & Mackintosh, 2002; Spence, 1937; Vervliet et al., 2011). In line with our hypothesis, generalization was wider in the low-probability condition across the entire generalization gradient.
As mentioned in the introduction, our studies confound low outcome probability given S+ with low contingency. Because low contingency might result in more similar predictions for S+ and S, it could have also led participants to make more similar predictions for all stimuli, including not only S+ and S, but also the generalization stimuli. Was it the case, then, that higher generalization in the low-probability conditions was actually caused by low contingency? We believe that several aspects of our results make this interpretation unlikely.
First, more similar predictions for S+ and S (less discrimination) should have been manifested not only in lower (more regressive) predictions for S+, but also in higher (more regressive) predictions for S. In other words, in the high-probability/contingency conditions there is stronger anti-correlation between S and the outcome, compared to the low-probability/contingency conditions. As a result, outcome predictions following S should have been more similar to S+ in the low-probability/contingency conditions (i.e., weaker anti-correlation) than in the high-probability/contingency conditions (i.e., stronger anticorrelation). In all three experiments, however, predictions for S are similar between the high and the low-probability/contingency conditions, both at the end of learning and in the first generalization block. 6 Second, if responses to generalization stimuli were a result of the relatively low contingency, then they should have seemed more similar not only to S+ but also to S in the low-probability conditions (compared to the highprobability conditions). This, however, was not the case for the novel stimuli which were the focus of our predictions (stimuli left to S+ in Experiments 1 and 2, and all novel stimuli in Experiment 3). These stimuli actually yielded responses less similar to S in the low-probability conditions than in the high-probability conditions.
Future studies should examine if behavior would differ depending on whether people are asked to predict the outcome (as in our experiments) as opposed to indicate whether the stimulus caused the outcome. Potentially, the latter question would be more sensitive to contingency than the former, which we anticipate to be more reflective of conditional probability. For example, consider an experimental design in which S+ is always followed by the outcome, but the outcome also occurs in between S+ presentations, thus weakening the contingency between S + and the outcome. Potentially, this would affect causal judgments (participants will be less convinced that S+ is the cause of the outcome) more than predictive judgment (participants will not be less convinced that the outcome would follow S+).
Generalization and Extinction
The Partial Reinforcements Extinction Effect (PREE, Atkinson, Atkinson, Smith, Bem, & Nolen-Hoeksema, 1995;
Baron & Kalsher, 2000; Hartman & Grant, 1960; Grant & Schipper, 1952) refers to the finding that a partial reinforcement schedule produces more resistance to extinction than continuous reinforcement. It is likely that PREE occurs because the difference in reinforcement rates between acquisition and extinction is less abrupt in partial reinforcement than in continuous reinforcement (Capaldi, 1966). Importantly, resistance to extinction can be viewed as an instance of wider generalization across contexts: The conditioned response or the conditioned stimulus in the context of acquisition is perceived as similar to a conditioned response or stimulus in the context of extinction. In this view, the PREE could be seen as reflecting broader generalization in partial compared to continuous reinforcement. This is, of course, consistent with our hypothesis in the present paper. Can the reverse hold? Namely can PREE explain our results? We think that this is not the case. First, we primarily relied on analyzing the first generalization block, in which extinction effects have not yet emerged (note that in both conditions, there were non-reinforced trials during acquisition). Second, we examined generalization to novel stimuli with systematic variation in similarity to the conditioned stimuli, which is different to generalization to the same conditioned stimulus in a different context, as is the case in PREE.
Implications and Future Directions
Construal Level Theory (CLT; Liberman & Trope, 2008, 2014; Trope & Liberman, 2010) addresses the question of how human beings mentally travel along four dimensions of psychological distance: plan for the future and remember the past (i.e., temporal distance), think about spatially remote places (i.e., spatial distance), consider other peoples’ points of view (i.e., social distance), and think about both likely and less likely, improbable situations (i.e., hypothetically). Because outcome probability corresponds to distance 7
between the predictor and the outcome on the dimension of hypotheticality, hypothesis regarding its effect on generalization can be derived from CLT.
Indeed, psychological distance is related to generalization in a fundamental way, as any act of prediction involves a tradeoff between accuracy and applicability (Liberman et al., 2011). For example, if I experienced rain with clouds of grayness level 6, then the prediction of rain only with that specific level of grayness is likely to be accurate, but is unlikely to apply to many situations. In contrast, predict37
ing rain for any level of grayness would apply to many situations, but is less likely to be accurate. Because psychological distance increases uncertainty, the learned stimulus needs to be categorized widely to be applicable. “Clouds of grayness level 6” might be too specific category to apply across different times, places, perspectives, and less likely situations. In other words, the variability inherent in distancing calls for using broader generalization to maintain applicability.
We see merit in the fact that learning theories and a social-psychological theory converge on a similar prediction. The experiments we presented here fit both frameworks, but future research could move to manipulations of distance that would be within the realm of CLT but outside the realm of traditional learning experiments. For example, we could introduce temporal distance by telling participants that the test phase will follow the training phase immediately versus much later. To the best of our knowledge, such a manipulation has never been used in learning experiments (perhaps because it would be difficult to implement with animals) and thus moves us more into the socialcognitive domain and to the important question of how communicated top-down information interacts with experience-based learning. Finding similar results of enhanced generalization with more distance with such paradigms would speak to the robustness of the effect of psychological distance on generalization.
Conclusions
Three experiments demonstrated that low probability of an outcome after a cue widens generalization. Understanding what affects generalization breadth is important in most basic sub-fields of psychology –learning, cognition, social psychology, and decision making. Needless to say, it is also important in many applied fields, such as education, work, organizational behavior, and public policy. In some real-life situations, such as in stereotyping and prejudice, policy makers would be mostly interested in narrowing generalization. In other situations, however, such as school learning and personnel development, authorities might primarily seek to enhance generalization. In both cases, better understanding the factors that affect generalization is crucial. We hope that the present paper made a modest step in that direction.
7
Notably, in Construal Level Theory (Liberman & Trope, 2008), psychological distance encompasses social, spatial, and temporal distances as well as hypotheticality and refers to the extent of divergence from the direct experience of me here and now. However, in classic learning theories (see Shepard, 1958b), “distance” refers to perceptual similarity, namely distance between stimuli that are represented as points in a continuous metric of a psychological space.
Electronic Supplementary Materials
The electronic supplementary material is available with the online version of the article at https://doi.org/10.1027/ 1618-3169/a000429
ESM 1. Data (.sav) Raw data of Experiment 1. ESM 2. Data (.sav) Conditions 3–2 Experiment ESM 3. Data (.sav) Experiment 3 probability square. ESM 4. Data (.sps) Analysis scripts. ESM 5. Tables and Figures (.docx) Tables of descriptive and inferential statistics for the control variables in Experiments 1–3. Figures of participants’ predictions for all stimuli in Experiments 1–3.
References
Allan, L. G. (1980). A note on measurement of contingency between two binary variables in judgment tasks. Bulletin of the Psychonomic Society, 15, 147–149. https://doi.org/ 10.3758/BF03334492 Atkinson, R. C., & Estes, W. K. (1963). Stimulus sampling theory. In R. D. Luce, R. R. Bush, & E. Galanter (Eds.), Handbook of mathematical psychology (Vol. 2, pp. 121 –268). New York, NY: Wiley. Atkinson, R. L., Atkinson, R. C., Smith, E. E., Bem, D. J., & NolenHoeksema, S. (1995). Introduction to psychology (10th ed.). Orlando, FL: Harcourt College. Baron, R. A., & Kalsher, M. J. (2000). Psychology (5th ed.). Boston, MA: Allyn & Bacon. Blough, D. S. (1975). Steady state data and a quantitative model of operant generalization and discrimination. Journal of Experimental Psychology: Animal Behavior Processes, 1 , 3. https:// doi.org/10.1037/0097-7403.1.1.3 Capaldi, E. J. (1966). Partial reinforcement: A hypothesis of sequential effects. Psychological Review, 73, 459. https://doi. org/10.1037/h0023684 De Houwer, J., & Beckers, T. (2002a). A review of recent developments in research and theories on human contingency learning. The Quarterly Journal of Experimental Psychology: Section B, 55, 289–310. https://doi.org/10.1080/02724990244000034 De Houwer, J., & Beckers, T. (2002b). Higher-order retrospective revaluation in human causal learning. The Quarterly Journal of Experimental Psychology: Section B, 55, 137–151. https://doi. org/10.1080/02724990143000216 Estes, W. K. (1950). Toward a statistical theory of learning. Psychological Review, 57, 94. https://doi.org/10.1037/h0058559 Estes, W. K. (1959). The statistical approach to learning theory. In S. Koch (Ed.), Psychology: A study of a science (Vol. 2, pp. 380–491). New York, NY: McGraw-Hill. Fazio, R. H., Eiser, J. R., & Shook, N. J. (2004). Attitude formation through exploration: Valence asymmetries. Journal of Personality and Social Psychology, 87, 293. https://doi.org/10.1037/ 0022-3514.87.3.293 Gershman, S. J., Blei, D. M., & Niv, Y. (2010). Context, learning, and extinction. Psychological Review, 117, 197. https://doi.org/ 10.1037/a0017808 Gershman, S. J., & Niv, Y. (2012). Exploring a latent cause theory of classical conditioning. Learning & Behavior, 40, 255–268. https://doi.org.10.3758/s13420-012-0080-8 Ghirlanda, S., & Enquist, M. (2003). A century of generalization. Animal Behaviour, 66, 15–36. https://doi.org/10.1006/anbe. 2003.2174 Grant, D. A., & Schipper, L. M. (1952). The acquisition and extinction of conditioned eyelid responses as a function of the percentage of fixed-ratio random reinforcement. Journal of Experimental Psychology, 43, 313. https://doi.org/10.1037/ h0057186 Hartman, T. F., & Grant, D. A. (1960). Effect of intermittent reinforcement on acquisition, extinction, and spontaneous recovery of the conditioned eyelid response. Journal of Experimental Psychology, 60, 89. https://doi.org/10.1037/h0039832 Hohwy, J. (2013). The predictive mind. Oxford, UK: Oxford University Press. Honig, W. K., & Urcuioli, P. J. (1981). The legacy of Guttman and Kalish (1956): 25 years of research on stimulus generalization. Journal of the Experimental Analysis of Behavior, 36, 405–445. https://doi.org/10.1901/jeab.1981.36-405 Hovland, C. I. (1937). The generalization of conditioned responses. IV. The effects of varying amounts of reinforcement upon the degree of generalization of conditioned responses. Journal of Experimental Psychology, 21 , 261. https://doi.org/10.1037/ h0061938 Humphreys, L. G. (1939). Generalization as a function of method of reinforcement. Journal of Experimental Psychology, 25, 361. https://doi.org/10.1037/h0057941 Jenkins, W. O., & Stanley, J. C. Jr (1950). Partial reinforcement: A review and critique. Psychological Bulletin, 47, 193. https://doi. org/10.1037/h0060772 Ledgerwood, A. (2015). Practical and painless: Five easy strategies to transition your lab. Talk presented in a symposium on best practices at the annual conference of the Society for Personality and Social Psychology, Long Beach, CA. Liberman, N., & Trope, Y. (2008). The psychology of transcending the here and now. Science, 1201 , 322–1205. https://doi.org/ 10.1126/science.1161958 Liberman, N., & Trope, Y. (2014). Traversing psychological distance. Trends in Cognitive Sciences, 18, 364–369. https://doi. org/10.1016/j.tics.2014.03.001 Liberman, N., Trope, Y., & Rim, S. (2011). Prediction: A construal level perspective. In M. Bar (Ed.), Prediction in the brain: Using the past to generate the future (pp. 144–158). New York, NY: Oxford University Press. McLaren, I. P. L., & Mackintosh, N. J. (2000). An elemental model of associative learning: I. Latent inhibition and perceptual learning. Animal Learning & Behavior, 28, 211 –246. https://doi. org/10.3758/BF03200258 McLaren, I. P. L., & Mackintosh, N. J. (2002). Associative learning and elemental representation: II. Generalization and discrimination. Animal Learning & Behavior, 30, 177–200. https://doi. org/10.3758/BF03192828 Purtle, R. B. (1973). Peak shift: A review. Psychological Bulletin, 80, 408. https://doi.org/10.1037/h0035233 Pearce, J. M. (1987). A model for stimulus generalization in Pavlovian conditioning. Psychological Review, 94, 61. https:// doi.org/10.1037/0033-295X.94.1.61 Shanks, D. R. (1995). The psychology of associative learning. Cambridge, UK: Cambridge University Press. Shepard, R. N. (1958). Stimulus and response generalization: Tests of a model relating generalization to distance in psychological space. Journal of Experimental Psychology, 55, 509. https://doi.org/10.1037/h0042354
Shepard, R. N. (1987). Toward a universal law of generalization for psychological science. Science, 237, 1317–1323. https://doi. org/10.1126/science.3629243 Soto, F. A., Gershman, S. J., & Niv, Y. (2014). Explaining compound generalization in associative and causal learning through rational principles of dimensional generalization. Psychological Review, 121 , 526. https://doi.org/10.1037/a0037018 Soto, F. A., Quintana, G. R., Pérez-Acosta, A. M., Ponce, F. P., & Vogel, E. H. (2015). Why are some dimensions integral? Testing two hypotheses through causal learning experiments. Cognition, 143, 163–177. https://doi.org/10.1016/j.cognition.2015.07.001 Spence, K. W. (1937). The differential response in animals to stimuli varying within a single dimension. Psychological Review, 44, 430. https://doi.org/10.1037/h0062885 Struyf, D., Iberico, C., & Vervliet, B. (2014). Increasing predictive estimations without further learning: the peak-shift effect. Experimental Psychology, 61 , 134–141. https://doi.org/ 10.1027/1618-3169/a000233 Suddendorf, T., & Corballis, M. C. (2007). The evolution of foresight: What is mental time travel, and is it unique to humans? Behavioral and Brain Sciences, 30, 299–313. https:// doi.org/10.1017/S0140525X07001975 Tenenbaum, J. B., & Griffiths, T. L. (2001). Generalization, similarity, and Bayesian inference. Behavioral and Brain Sciences, 24, 629–640. https://doi.org/10.1017/S0140525X01000061 Todorov, A., Goren, A., & Trope, Y. (2007). Probability as a psychological distance: Construal and preferences. Journal of Experimental Social Psychology, 43, 473–482. https://doi.org/ 10.1016/j.jesp.2006.04.002 Trope, Y., & Liberman, N. (2010). Construal-level theory of psychological distance. Psychological Review, 117, 440. https://doi.org/10.1037/a0018963 Vervliet, B., Iberico, C., Vervoort, E., & Baeyens, F. (2011). Generalization gradients in human predictive learning: Effects of discrimination training and within-subjects testing. Learning and Motivation, 42, 210–220. https://doi.org/10.1016/j.lmot. 2011.03.004 Wakslak, C., & Trope, Y. (2009). The effect of construal level on subjective probability estimates. Psychological Science, 20, 52–58. https://doi.org/10.1111/j.1467-9280.2008.02250.x Wakslak, C. J., Trope, Y., Liberman, N., & Alony, R. (2006). Seeing the forest when entry is unlikely: Probability and the mental representation of events. Journal of Experimental Psychology: General, 135, 641. https://doi.org/10.1037/0096-3445.135.4.641 39
Welham, A. K., & Wills, A. J. (2011). Unitization, similarity, and overt attention in categorization and exposure. Memory & Cognition, 39, 1518. https://doi.org/10.3758/s13421-011-0124-x Wickens, D. D., Schroder, H. M., & Snide, J. D. (1954). Primary stimulus generalization of the GSR under two conditions. Journal of Experimental Psychology, 47, 52. https://doi.org/ 10.1037/h0053617
History Received September 17, 2018 Revision received August 7, 2018 Accepted August 16, 2018 Published online February 19, 2019
Acknowledgment The data reported in this manuscript were presented at a conference (European Social Cognition Network Transfer of Knowledge Conference, Lisbon, Portugal during July 2016).
Open Data Raw data, conditions, analysis scripts, and additional materials are available in the Electronic Supplementary Materials, ESM 1 –5.
Funding This work was supported by the I-CORE Program of the Planning and Budgeting Committee and the Israel Science Foundation (Grant 51/11) and by a Center for Excellence grant from the University of Leuven –KU Leuven (PF/10/005).
ORCID Hadar Ram
https://orcid.org/0000-0003-0079-9425
Hadar Ram School of Psychological Sciences Tel Aviv University PO Box 39040 Tel Aviv 69978 Israel ramhadar5@gmail.com