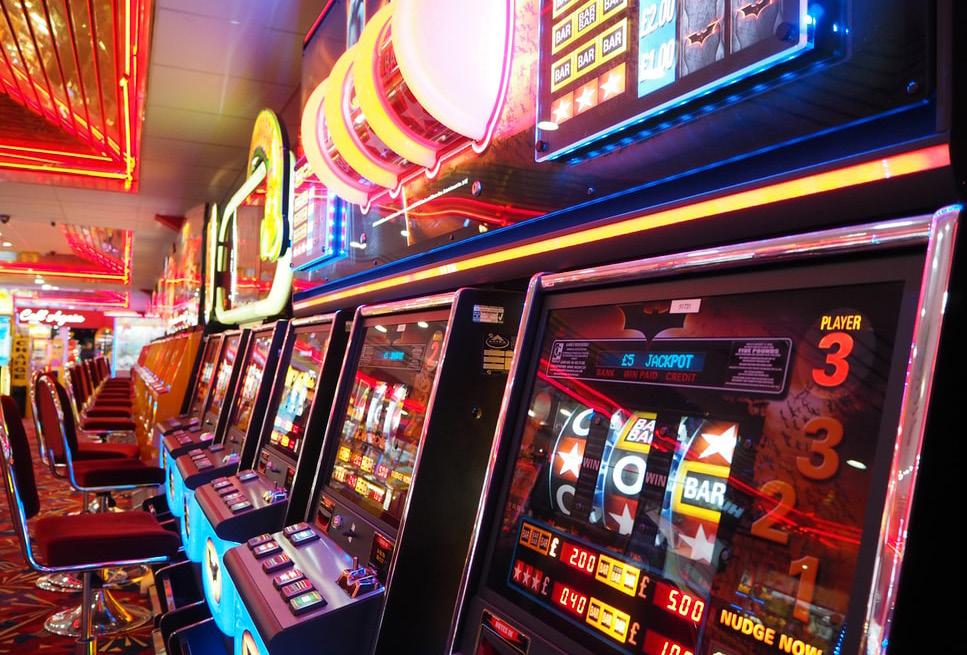
5 minute read
SLOT MANAGEMENT
Slot Management 101
How to ‘Improve’ your slots of problems!
By Andy Cosgrove
You defined, measured and analyzed your slots of problems but your boss is putting pressure on you to turn all your analysis paralysis into tangible results that add real value. It’s time to take action… or run for the hills.
In my previous article we focused on the analysis phase of Six Sigma which shows how to turn a practical problem into a statistical problem with the goal of finding a statistical solution prior to implementing a practical solution. The Improvement phase is where your dreams of keeping all your bill validators accepting every single bill all of the time and time after time. It’s crunch time, time to earn you paycheck, time to exercise your trade craft, time to walk the walk, talk the talk and prove that you and your team are the best team for the job. It may also be time for your boss to say thank you for a job well done. about the Improve phase?–Before implementing the final solution that will keep your bill validators running at an optimum acceptance rate, the improve phase starts with more advanced process modelling designed to Identify the vital few X’s (input variables) that are causing your customers to leave your establishment. Then comes experimentation which helps to optimize the X’s before simulating and validating your process improvement. Finally, and the part you’ve been waiting for, is implementing theprocess improvement prior to the Control phase which is the final phase of the Six Sigma methodology. The key deliverables of the Improvement phase are as follows: • Process modelling: regression • Advanced process modelling: MLR • Designing experiments
Process modelling: regression–Before regression comes linear correlation analysis which is used to measure the strength of the association between two variables known as X and Y, where Y represents the Output and X is the input. In casino talk, Y represents the bill validator accepting your customer’s bills and X is the dirt that makes your bill validator reject bills as a ratio of 9 out of every 10. Linear correlation analysis will determine the following:
• If the frequency of annual cleaning(X) increases and the acceptance rate (Y) remains the same, there is no correlation between cleanliness and the bill acceptance rate
• If you increase the frequency of annual cleaning (X) and the acceptance rate (Y) increases, there is a correlation
• The higher the correlation coefficient score (r), the higher the correlation between the frequency of annual cleaning and bill acceptance rate will be.
• The correlation scale starts at minus one (-1) which represents a strong negative correlation and plus one (+1) which represents a strong positive correlation.An ‘r’ score of between -0.2 and 0.2 means no significant correlation. gives the mathematical
relationship or the prediction model. The last step to proper analysis of continuous data is to
determine the regression equation. The Regression Equation can mathematically predict Y for any given X, for example, If you clean your bill validator twice a year instead of once, you should achieve a 50% better acceptance rate given the same amount of usage. Being able to predict future results based on existing sets of data (acceptance rate and cleaning frequency) will not only make your operation more efficient, but it may also make your finance department smile!
Advanced Process modelling:
MLR – MLR stands for multiple linear regression, and where simple linear regression uses 1 input (X) and one output (Y) to predict results, MLRuses multiple inputs(X’s) to predict one single output. MLRalso accounts for residuals that represent any variation that could be affecting your bill validators from operating at a higher acceptance rate. Putting it simply, MLR produces a much more accurate prediction model for moving you closer to bill validator Utopia. The following two inputs (X’s) could be used as part of your prediction model for a higher bill acceptance rate using MLR
• Input (X) number 1 =cleaning frequency
• Input (X) number 2 = number of games played
• Output (Y) = Bill acceptance rate
Designing Experiments–Now that you’ve identified the vital input X’s that are making your bill validator not accept bills it’s time to put your theories to the test by using designed experiments. The desired results of experiments are as follows:
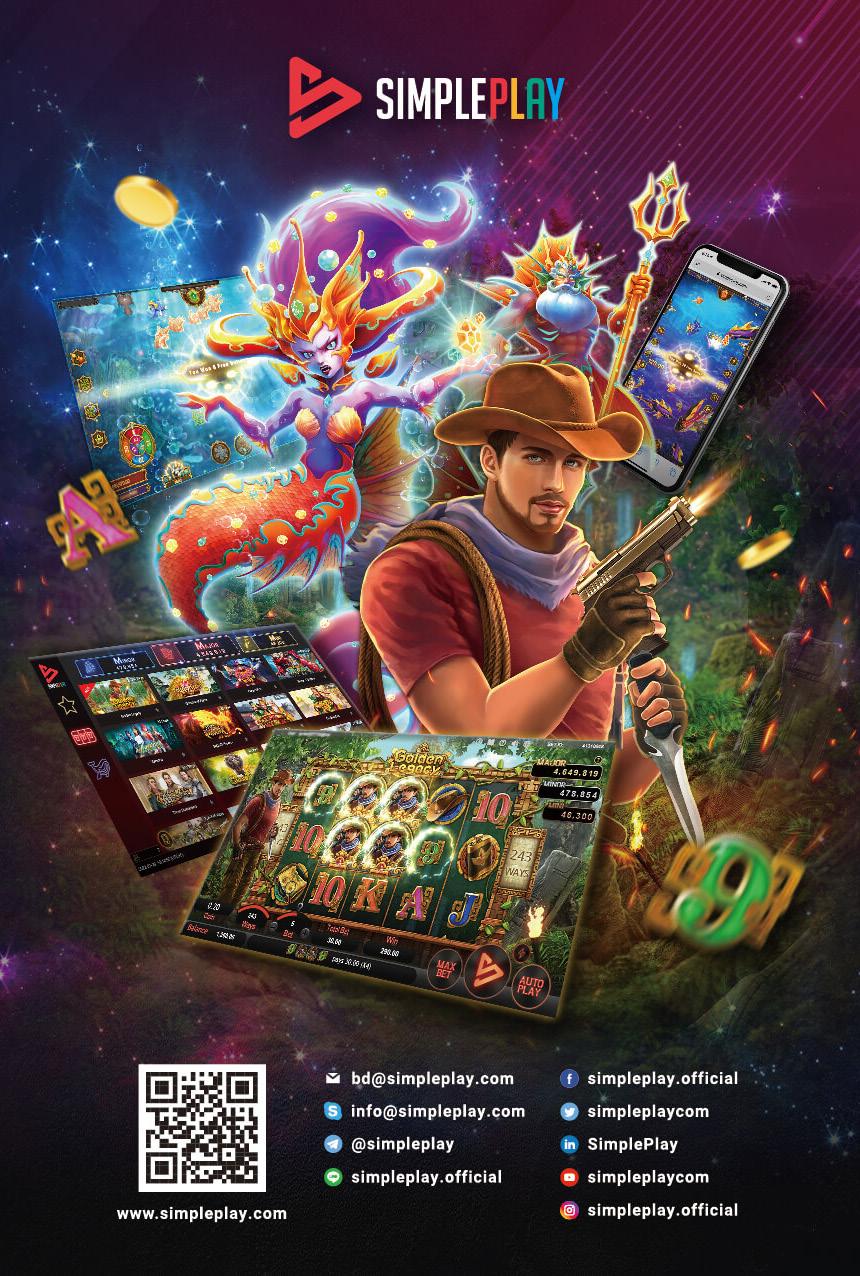
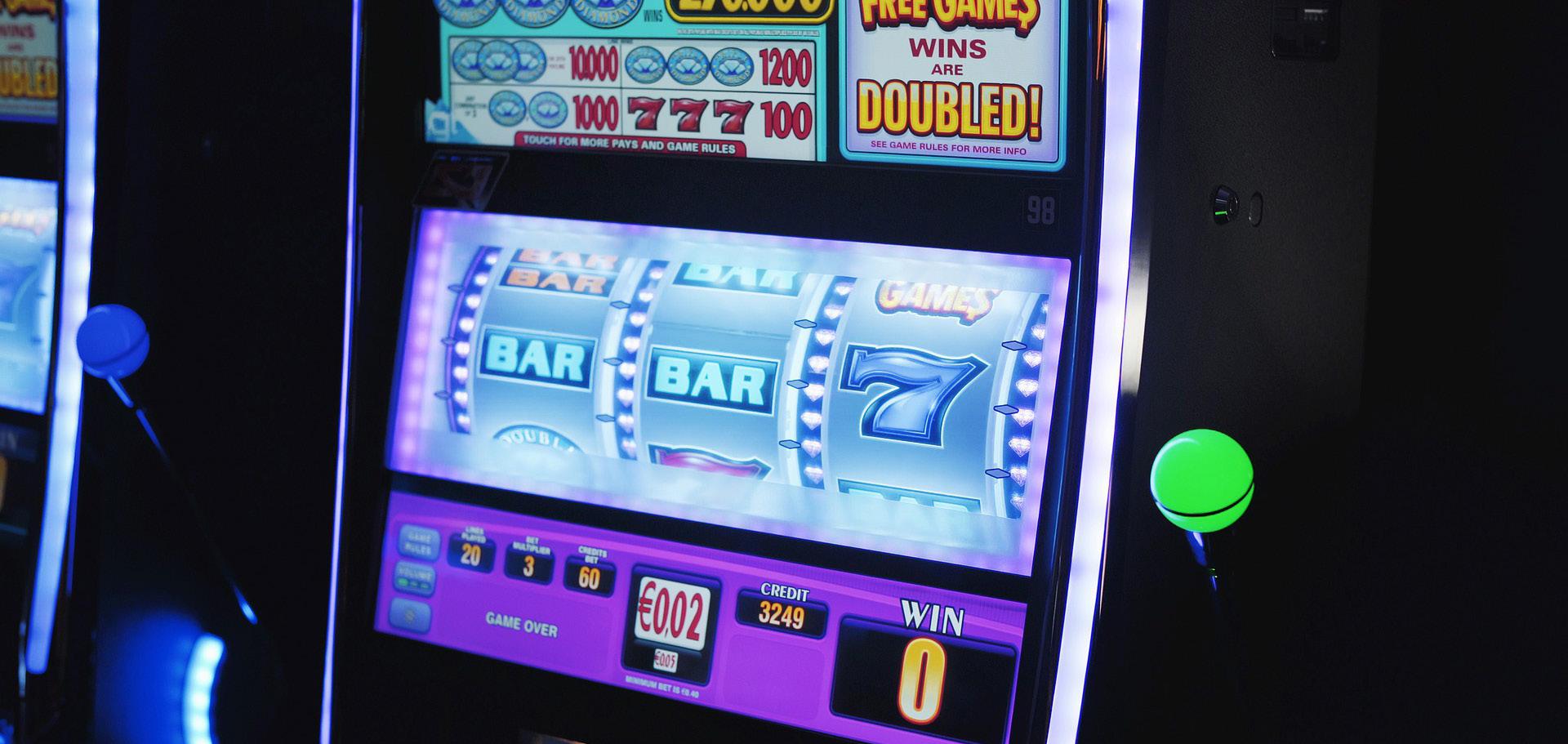
• Problem solving – to eliminate defective products or services
• Optimizing – mathematical process is desired to increase the bill acceptance rate
• Robust design – to provide consistent bill validator performance and designing processes while knowingly understanding which variables are difficult to maintain
• Screening – necessary when there is limited past process data or when statistical conclusions prevented the optimum performance of you bill validators
Design of Experiments (DOE) shows the true cause and effect relationship of the Input (X) Output (Y) of interest. The DOE Methodology includes the following:
1. Define the practical Problem (low bill acceptance rate)
2. Establish the experimental objective (improve bill acceptance rates) cleaning frequency (X))
4. Choose the levels of the Input variables (a 2 level test could involve a cleaning frequency of 1 time per month and another of 1 time per year)
5. Select the experimental design, execute the experiments and collect the data (experimental design depends on the amount of input(X) variables being tested such as cleaning frequency and games played)
6. Analyze the data and draw statistical conclusions
7. Draw practical conclusions and replicate or validate them(to minimize the affect of any unhidden bill validator headaches!)
8. Implement Solutions (success).
Graphical DOE is achieved via Interaction plots which make results much more obvious and present them in a way that even the casino manager might understand! lyze phase but Designed Experiments can be extremely effective. If you’re confident with your solution at the end of the analyze phase, then stop but if you have doubts, use designed experiments to help you over the finish line. What’s important to remember is that the sole purpose of a Six Sigma project is to eliminate your poor bill validator acceptance problems and transform it into a solution that you, your team, your customers (and maybe even the marketing manager) can be proud of. Coming next time is the Control phase which is the final phase in the Six Sigma methodology.
Andrew Cosgrove is a seasoned slot operations veteran and certified project manager with over 24 years of hands on experience in Latin America and the Caribbean. Andrew has worked on both the operator and supplier side of casino slots and is available to help you succeed and exceed customer expectations via contracted consultancy services. Andrew can be reached at andy.cosgrove@ henimgwaycasinoconsulting.com or seehttps://hemingwaycasinoconsulting.com/