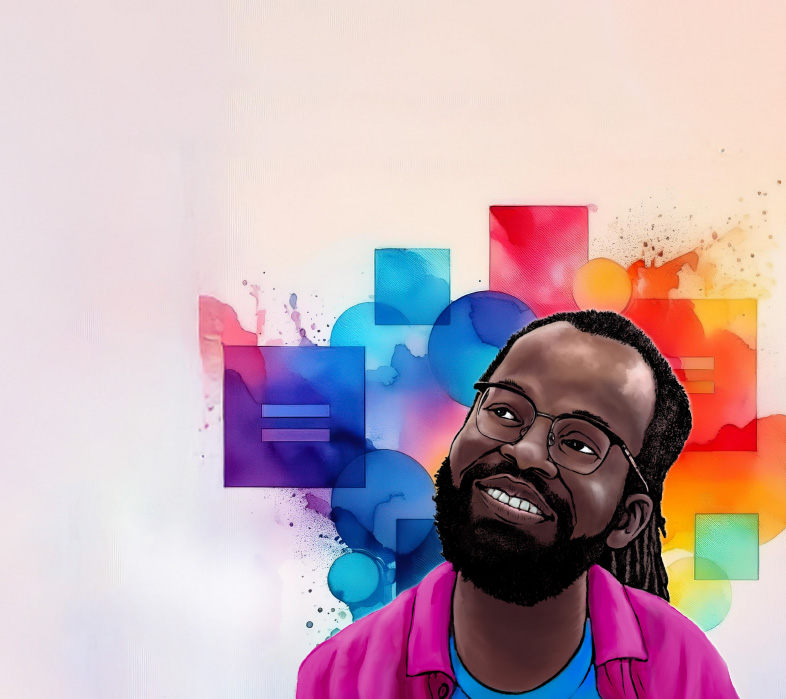
5 minute read
Trailblazing in an Ethical AI Landscape
The trials of building responsible, fair, and robust models
Artificial intelligence’s fine line between potential and peril put the powerful technology’s developers on slippery footing. It presents an unparalleled opportunity to solve some grand societal challenges, but missteps and a lack of care can perpetuate harm to significant segments of society.
Emmanuel Klu (CS ’13) works on the front line of building AI systems as a responsible and society-centered AI research engineer at Google Research. He says that this work includes asking many questions, anticipating pitfalls, and developing systems that people can trust and rely on.
“I spend a lot of my time working in two buckets: building AI systems responsibly and leveraging AI for social impact,” Klu says. “Our research is driven by solving problems that matter in society. For example, we work on language inclusion in AI models and leveraging AI to solve food insecurity.”
The first step of building AI models is to deeply understand the problem that you intend to solve. It is critical to be familiar with the various factors that influence the behaviors or outcomes that you’re modeling and to engage stakeholders with the requisite expertise for the problem domain. This helps to frame the AI task appropriately, identify potential pitfalls, and minimize unintended outcomes.
“Once the problem is correctly identified, the next important step is all about data,” Klu says. “The concept of data-centric AI comes to mind here: that AI will only be as good as its data. This means that to build models responsibly, we need to make sure the data being used supports that goal.”
Determining what represents good data really depends on the task at hand, but the principles underpinning responsible AI are consistent.
A few of these principles include privacy, fairness, and robustness.
“We need to protect privacy by making sure data doesn’t include anything that can identify an individual,” Klu says. “We also need to understand the bias present in the data and how that impacts fairness of outcomes. For robustness, we assess whether the data is representative enough to support all possible scenarios in a practical setting.”
One project that Klu worked on aimed to reduce unintended bias in language models, specifically in toxicity detection. The goal was to ensure that toxicity results were not disproportionately impacting marginalized communities. The key to this project was the development of a rich taxonomy for identity and to build a repository of context associated with identity terms. By collaborating with affected communities, this collected knowledge was leveraged to evaluate language models and to understand performance across various identity groups. Quantifying the extent of the problem and intervening to address it required carefully crafted data.
Leveraging AI for decision making is a tricky topic, especially as AI gets deployed in critical domains such as health care. One major challenge is understanding long term impacts of interventions—technological or otherwise—in society.
Klu says that he spends a fair amount of his time on systems dynamics, a discipline commonly used in business and engineering operations, as an approach to better understanding and addressing complex societal problems.
It allows him to explore possible feedback loops that technology creates. For example, how technology changes human behavior, the unexpected ways in which humans may use technology, and how that use will lead to the evolution of these models.
“We need to better understand how human-computer interactions might evolve and how best to build today to mitigate harms that may take time to show up,” he says.
This expands his research at Google beyond the technical aspects of building AI models and into the social sciences. “We call AI development a ‘sociotechnical’ process,” Klu says. “This naturally means it must be multidisciplinary.”
“For example, beyond our technical approaches to evaluating a model, we also pull in a lot of people to try to use it, break it, or highlight issues,” he adds.
This “red team” may include ethicists, social scientists, user experience specialists, and psychologists—professionals who don’t need to have technical experience—to help AI researchers develop models that are fair, robust, safe, and trustworthy.
Klu says his introduction to AI research started at Illinois Tech—but he opted to temporarily leave the research field to join Google as a software engineer immediately after graduating in 2013.
He started at Google by building a platform for designing and managing big data pipelines for enterprises, and he later worked as a site reliability engineer in Google Cloud, where his focus shifted to systems modeling and reliability while he helped the platform expand its geographic footprint.
But he wanted to use his skills to work on social issues and moved into Google’s AI division.
“I found a research team working on solving systemic and societal problems,” he says. “Although I didn’t have much direct experience building AI, I knew systems, data, and scale. I was able to bring my systems thinking into that context.”
He continues, “I like to believe I found my way back to AI at the right time, as it started to experience its boom in society. My role allows me to channel my hopes for a more just, fair, equitable, and sustainable society into my work. I think appreciating and understanding the complexity of society is only the first step towards that.
“I am excited to see how we can get to better understand AI and leverage it for impact.”