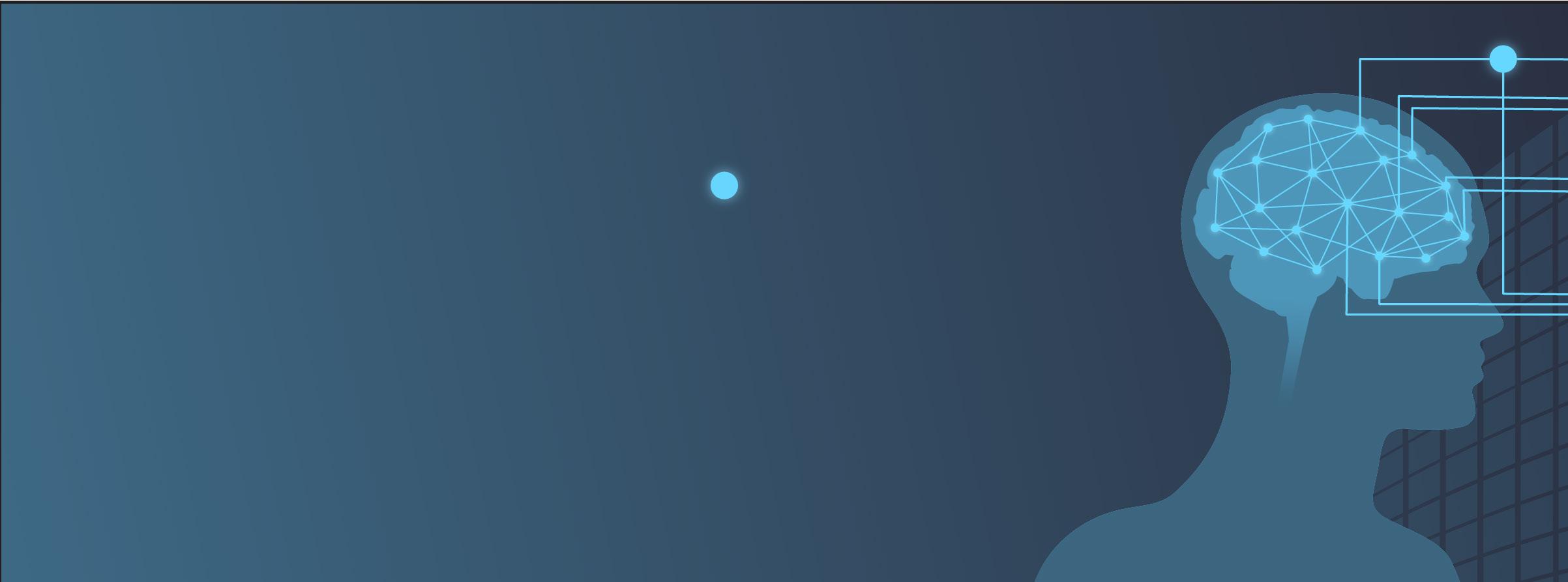
4 minute read
Revolutionizing Healthcare with AI A Quest to Improving Disease Screening and Diagnosis
By Niki Akbarian
Artificial intelligence (AI) has been integrated into various aspects of today’s society, and the medical field is no exception. In recent years, AI has shown great potential in improving disease diagnosis, prediction of treatment outcomes, equity in healthcare, and overall patient care.1 Some even say that AI can revolutionize our approach to healthcare in the future. According to Dr. Alexander Bilbily—a Radiologist and Scientist at Sunnybrook Health Sciences Centre and the Co-Founder of 16 Bit Inc.—, “[AI] is the new electricity that has just been invented, and it is starting to be used everywhere to create things that were never possible before.”
Advertisement
Dr. Bilbily’s dual passion for medicine and computer science led him to specialize in radiology after receiving his MD from the University of Toronto. During his residency training, which coincided with the beginning of the modern era of AI, Dr. Bilbily recognized the potential for AI models to improve the medical field, especially for disease screening and diagnosis. As a result, he co-founded 16 Bit, a startup with the vision of improving healthcare quality, efficiency, and equity with AI. Dr. Bilbily also directs a research lab at Sunnybrook Health Sciences Centre where he and his team work on several projects to design and implement cutting-edge screening and diagnostic tools across Canada.
One of Dr. Bilbily’s initiatives at 16 Bit uses AI to augment screening programs for osteoporosis, a condition characterized by reduced bone mineral density and bone mass. This results in changes in the architecture and strength of bones, increasing the risk of fractures.2 Although there are precise tools such as dual X-ray absorptiometry (DXA) to diagnose osteoporosis, 75% of affected individuals have never been screened. This may be due to the silent nature of the disease, as patients typically live with low bone mineral density for many years before experiencing its detrimental consequences, such as bone fracture. Thus, Dr. Bilbily and his team at 16 Bit have developed a model that would allow the screening and identification of patients susceptible to osteoporosis with X-ray images, as 80% of the population aged over 50 undergo at least one X-ray in their lifetime for various medical conditions other than osteoporosis. Although it is not possible to directly measure bone density from X-rays like you would with DXA, other features, such as bone quality and architecture that are also affected by osteoporosis but are not currently used as diagnostic measures, are captured by X-rays. Dr. Bilbily and his team at 16 Bit have trained an AI model, called Rho, which can map changes in bone architecture and other features detected by X-rays to changes in bone density as measured by DXA. In turn, Rho can extract clinical insight about the risk of each patient developing osteoporosis from X-rays and notify clinicians to run clinical fracture risk assessment and DXA to confirm the diagnosis for high-risk individuals. Similarly, Dr. Bilbily leads a project to train models that can predict patients’ cardiac risk from myocardial perfusion imaging, as well as covariates such as their age and gender.
The objective of another project that Dr. Bilbily and his team work on is to improve the quality and affordability of positron emission tomography (PET) scans. PET is an imaging modality that utilizes radioactive drugs, called radiotracers, to demonstrate the metabolic and biochemical activity of tissues and organs.3 Although PET scans are great assets in the medical field, especially for oncological, neurological, and cardiovascular applications,3 radiotracers injected for scans are expensive and associated with significant radiation exposure to patients. Hence, Dr. Bilbily and his team are interested in using machine learning (ML) methods to minimize the noise and improve the quality of imaging while using smaller doses of radiotracers. Specifically, the models created by ML would match full-dose PET scans to simulated scans that would be generated by a quarter of the required radiotracer dose. In other words, these models would take a lowdose image and turn them into images with the same quality and details as full-dose scans. If the team achieves a good model performance, it is possible to inject each patient with a quarter of the dose originally required, allowing patients to be scanned with reduced radiation exposure and at a reduced cost. One challenge, however, is to prove that the de-noised low-dose images will not miss important details, such as small metastasis. To overcome this barrier, physicians, blind to the dosage of radiotracers used, are asked to interpret the full-dose images and their de-noised low-dose match to evaluate whether it is possible to arrive at the same diagnosis using low-dose versus full-dose scans.
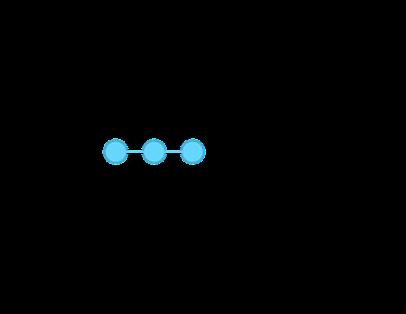
In fact, the impact of AI tools developed by Dr. Bilbily and his team has gone beyond the research setting. For instance, Rho has been Health Canada approved and has screened 18,000 patients since September 2022. Out of the thousands of individuals screened with Rho, approximately 50% were identified as at risk for osteopenia/osteoporosis, and 90% of those who had DXA after being identified as high-risk were diagnosed with the disease. It is also notable that using tools such as Rho involves no additional costs for patients as the information required for their performance comes from X-rays that are being obtained for other medical reasons. Therefore, it is essential to be transparent with patients about how AI tools are developed and how they can assist healthcare professionals in providing more efficient and precise care.
Overall, the benefits of AI for the healthcare system are numerous. Dr. Bilbily noted, “AI is no longer a nice to have. It has become a necessity to improve efficiency because our healthcare system does not seem to be sustainable in the long term. As far as I can see, AI is our best shot.” Yet, it is critical to consider the potential limitations of deploying AI in medicine. The training of AI algorithms relies heavily on large, standardized datasets which may not be readily available in the healthcare setting. Due to the unstructured nature of electronic patient records and concerns about confidentiality, datasets are usually fragmented and incomplete. Furthermore, patient populations and treatments are rapidly changing, making it challenging to generalize and externally validate AI models trained with data from a specific patient population. Thus, physicians must understand how changes in interventions and patients can impact the performance of medical AI tools. As Dr. Bilbily states, “So much value can be potentially unlocked by [AI], but at the same time, we have to be very careful with how these [tools] are implemented in medicine…we need to make sure that [AI] is used in a safe and appropriate way.” Hence, training the next generation of clinicians with sufficient understanding of both computer science and medicine is critical for appropriately leveraging AI in healthcare.
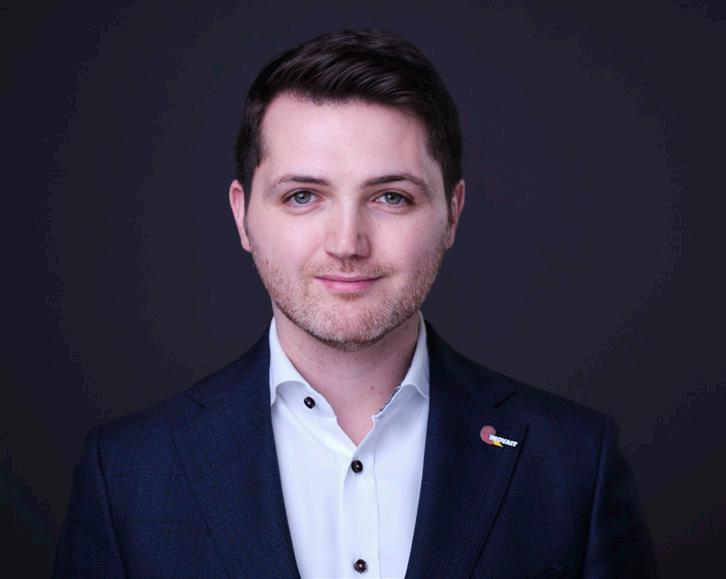
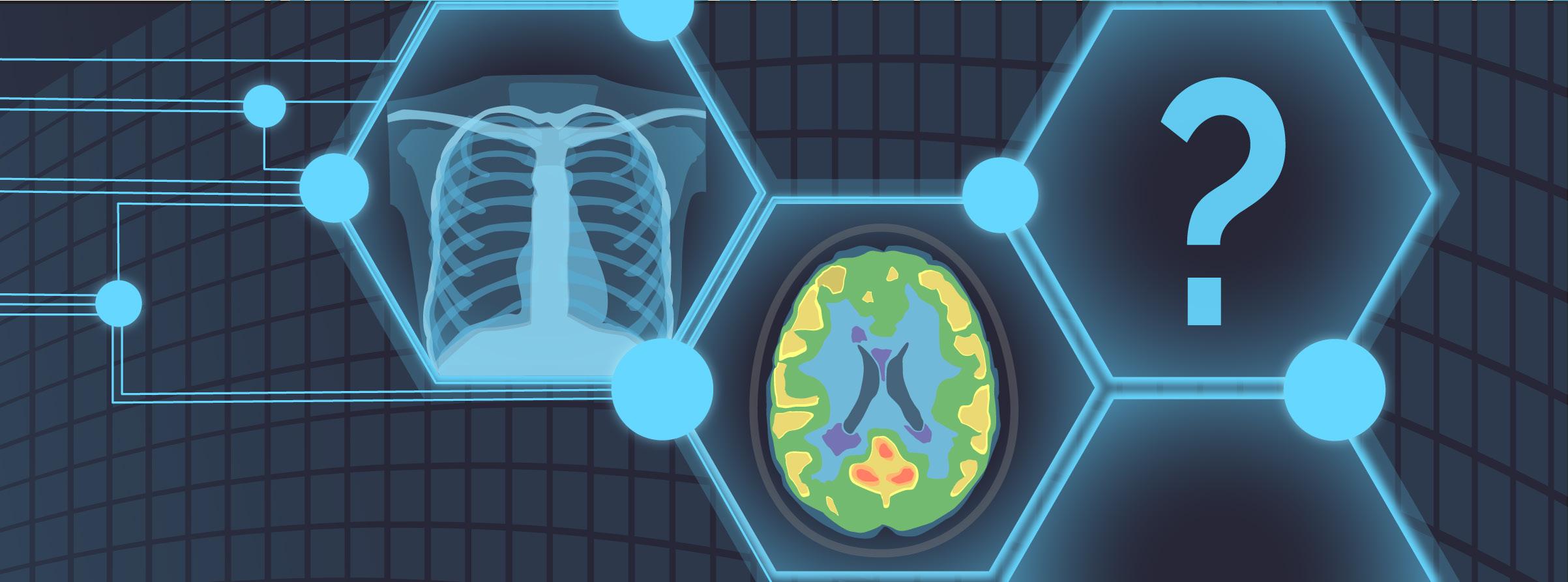