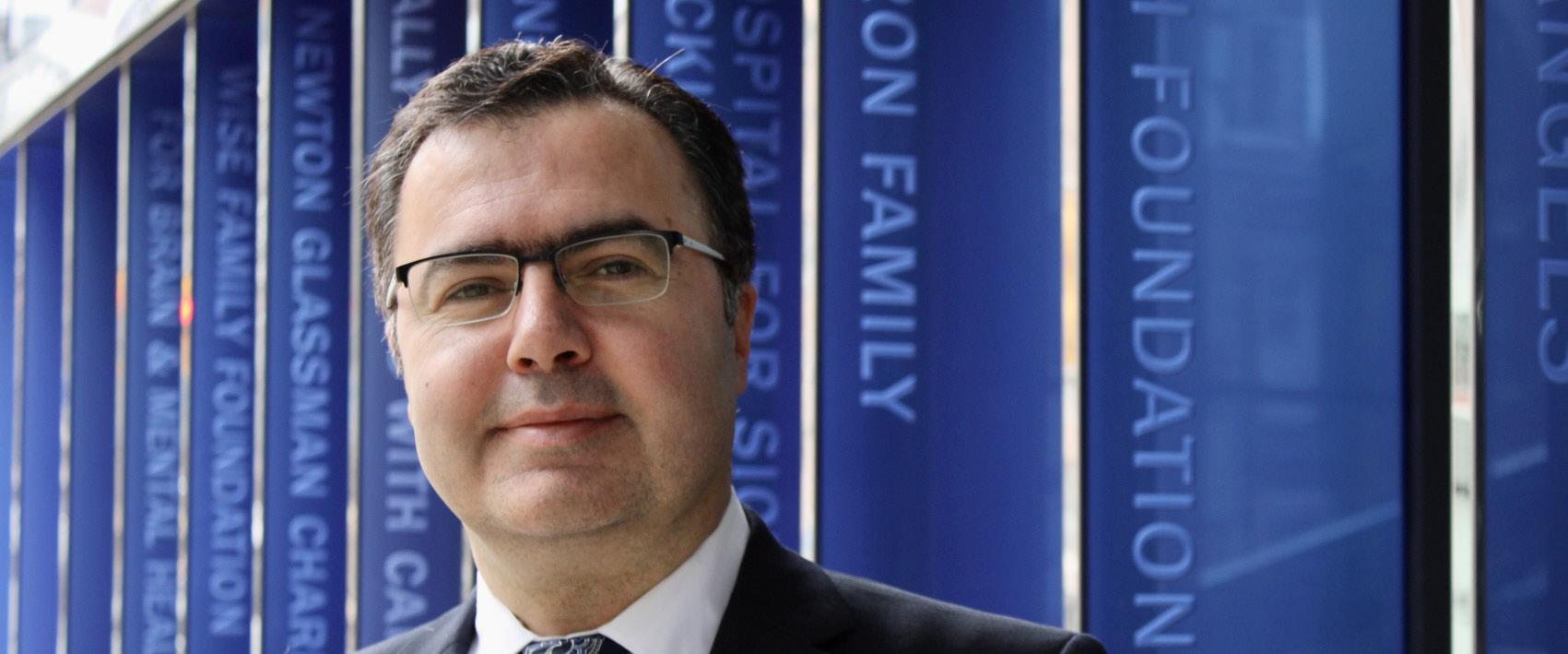
4 minute read
An Image is Worth a Thousand Diagnostic Variables
Developing AI-based solutions for precision medicine
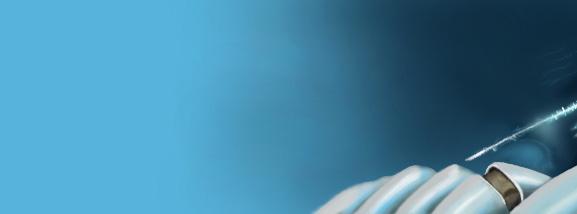
Advertisement
By Beatrix Wang
Tumours are extremely diverse, owing to the massive network of cell cycle regulators that, when perturbed, trigger formation of cancerous tissue. Clinicians and scientists are increasingly recognizing this heterogeneity and are decoding how the genetic backgrounds of tumours inform how they respond to different drugs. However, there are often barriers to actually using this genetic information to treat patients.
Nowhere are these challenges more evident than in tumours of the central nervous system, which represent a leading cause of death and morbidity for children with cancer.
“Biopsying a brain tumour is not an easy task,” says Dr. Farzad Khalvati. “It’s a very invasive procedure which may actually be harmful.” That being said, performing biopsies, a process that typically involves drilling a hole in the skull and using a needle to remove the tissue of interest, remains the gold standard for identifying the genetic factors underlying brain tumour growth. Without this information, it can be difficult to provide patients with precision medicine—treatments that target specific tumour subtypes while minimizing negative side-effects. Therefore, oncologists and neurosurgeons must carefully balance the risks and benefits of performing such a procedure.
Dr. Khalvati, a scientist at the Hospital for Sick Children and an associate professor in the Departments of Medical Imaging and Computer Science at the University of Toronto, thinks there is a faster and less invasive way to provide precise treatments for patients with paediatric low-grade gliomas (pLGGs), the most common type of brain tumour in children. For Dr. Khalvati and his lab, this solution involves the combination of artificial intelligence (AI) algorithms and medical imaging.
The premise is simple: because magnetic resonance imaging (MRI) is routinely performed for brain tumour diagnosis, there exists a great deal of information linking tumour appearance to genetic makeup. Dr. Khalvati believes that, by training AI algorithms on these MRI images and their corresponding genetic data, he can develop a program that can accurately identify the mutations driving glioma formation and growth.
This approach is promising in part because pictures are, by their very nature, dense with information that can be extracted and transformed into meaning, something that AI is particularly well-suited for. Through training, deep learning algorithms can take the data implicit within MRI scans and build predictive models using image features that help inform the underlying genetics of the tumours.
These features go beyond characteristics that are intuitive to humans, like tumour size and shape. They even go beyond more abstract radiomic features like pixel intensity, texture, and whether there is heterogeneity or homogeneity within the image. “[AI] looks at any possible information latent in the tumour region” Dr. Khalvati says. The result is thousands and even millions of potentially informative variables, which often have no concrete understandable meaning. Dr. Khalvati continues, “With AI, we are dealing with an ocean of biomarkers—candidate biomarkers—and we want to find the best model that uses these biomarkers to make predictions.”
Dr. Khalvati and his team have had significant success with their approach thus far. When looking at the two most common subtypes of pLGGs, they are currently able to correctly predict glioma type nearly 90% of the time, and the incorporation of less common subtypes yields an accuracy of roughly 80%. There is still much work to be done, but in this rapidly evolving technological landscape, Dr. Khalvati hopes that this application of AI can be deployed to clinical settings within the next five years.
That being said, before this approach can become reality, there are various challenges that need to be addressed, many of which are technical. For example, for AI-based diagnostic algorithms to be used widely, they must be generalizable across different MRI machines and settings. If such a program cannot recognize that the same tumour can look different when scanned under different conditions, then its usefulness will be limited.
One of the greatest barriers to the widespread adoption of AI-based diagnostic tools, however, is of a completely different variety. According to Dr. Khalvati, it has to do with trust. Will oncologists and neuroradiologists trust the predictions made by AI enough to adopt this tool? How do you prove to clinicians that something as intangible as AI is as accurate as concrete laboratory results? How do you make clinicians believe in AI, to the extent that they would entrust the well-being of their patients to a computer program? And how do you ensure that patients feel comfortable knowing their diagnoses have been at least partially made by AI?
The question of how to build this trust is something Dr. Khalvati and his team are also actively working on. One solution, he says, is through explainability. If the extraordinarily complex models used to predict tumour genotypes could be made more comprehensible to the clinicians using them, and if their outputs were demonstrably logical in ways that humans could follow, then clinicians would have fewer reservations about relying on them. These diagnostic decisions could then be more easily communicated to patients as well.
Another solution involves allowing for increased interaction between AI and clinicians in what is known as a ‘humanin-the-loop approach’. “I think there should be a mechanism in place where clinicians can learn from AI, and AI can also learn from the clinicians,” Dr. Khalvati says. “There should be a two-way connection.” By having clinicians and AI working alongside one another and informing one another’s decision-making, not only could clinicians correct mistakes made by AI to prevent them from happening in the future, but the AI could also potentially flag cases of human error. Such a platform could not only be beneficial for patient care but would go a long way towards establishing trust between clinicians and AI-based diagnostic tools.
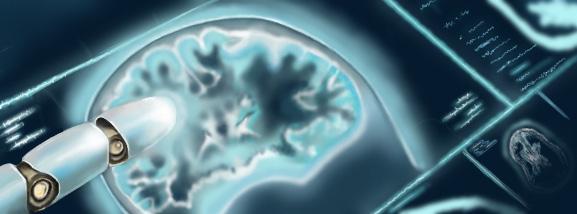
According to Dr. Khalvati, this philosophy of AI and humans working hand in hand is crucial as we move forward into a world that is increasingly reliant on AI-based tools. Right now, there are many open questions about what this world will look like and what roles AI will play in it, just as there are many problems without clear-cut solutions that need to be addressed as we push into the future. To Dr. Khalvati, it is clear that we must implement human-in-the-loop platforms that prioritize explainability as we move forward with AI-based technologies. “By always keeping [humans] in the loop, I think we are all better off,” he says. “We can learn from AI, we can adjust AI, and we can have a better understanding of how decisions are made that definitely impact our lives.” Dr. Khalvati believes that it is through making this human-centric approach a reality— wherein we work with AI, shape it, and are also informed by it—that AI can be a force for human empowerment.