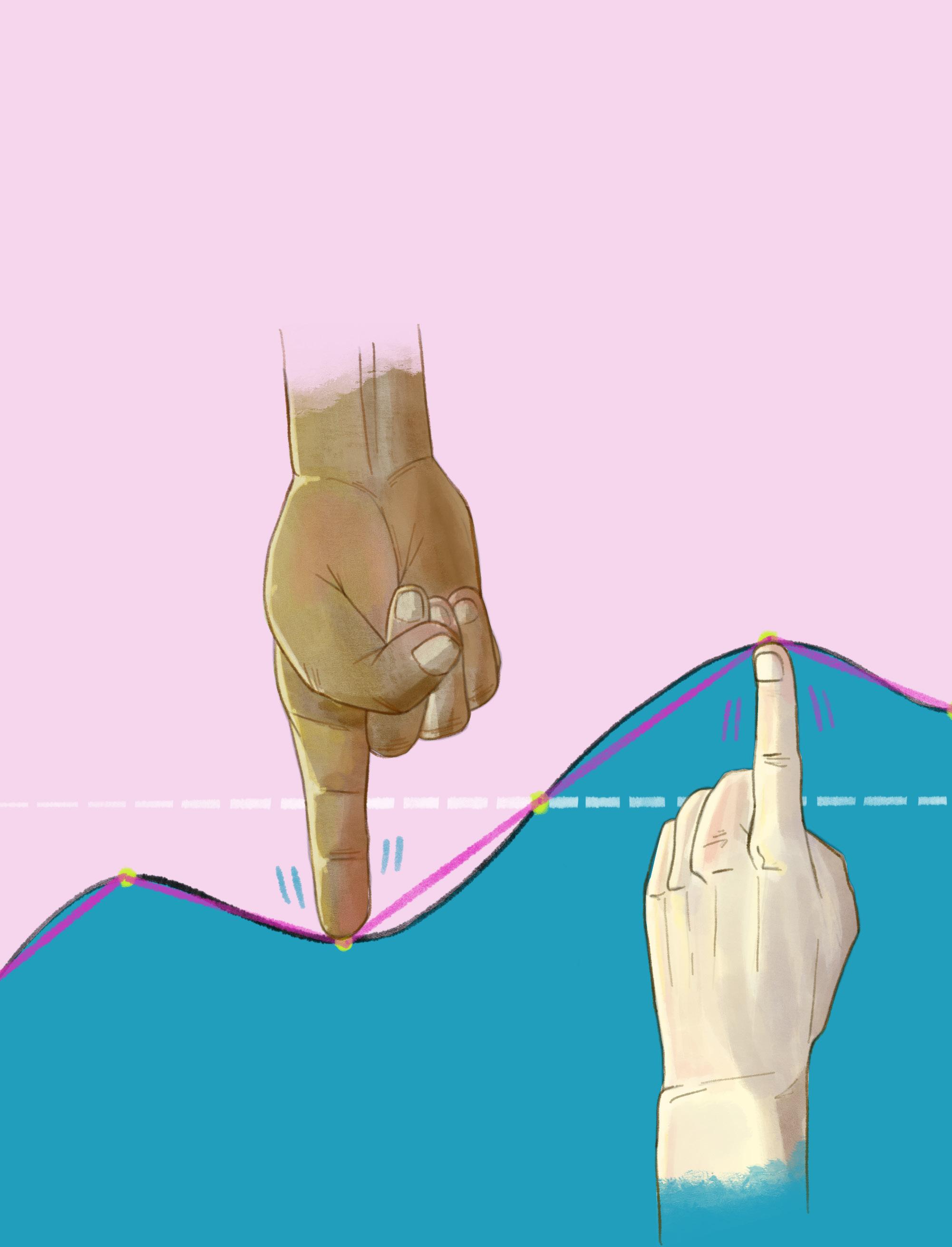
4 minute read
Diversity in Science
By Shu’ayb Simmons
Advertisement
Say, for a second, you are tasked with assessing the association between A and B across X, but C influences said relationship. This is problematic since the existence of variable C on variables A and B confuses the nature of the association— potentially affecting statistical findings and likely threatening the validity of your study. This issue becomes amplified when considering huge variables such as race, which affects many other variables. This article discusses race-related confounding variables, biomedical research validity, and possible ways to evaluate these confounds.
One, Two, Covariate, Four: Think of the Confounders
Confounders—also known as confounding variables—influence the correlation between the predictor (the independent variable) and the outcome (the dependent variable), and are not statistically accounted for.1,2,3 In other words, think of a confounder as a hidden factor distorting the predictor-outcome relationship. For any variable to be considered a confounder, it must be: 1) associated with the predictor, 2) associated with the outcome, and 3) not be an intermediary between the outcome and predictor.1 For example, let’s say you are trying to establish a relationship between the day (i.e., the predictor) and the number of Toronto Green P bikes rented (i.e., the outcome) but are not accounting for weather changes.
Here, the weather could be a potential confounder since weather and bike rentals are likely correlated. This is problematic as confounding variables can change the directionality of the effect, magnitude, and significance of the results. For this reason, the appropriate consideration of confounders is key to ensuring that your statistical model is measuring its intended metric—a concept known as validity.2 To account for confounders, biomedical researchers add them as covariates in their models. In doing so, they adjust for (or remove) the effect of that variable in the predictor-outcome relationship.
Unfortunately, the addition of covariates is not always linear (if you’ll pardon the pun), as some confounding relationships are not always explicit. In these cases, failing to consider less obvious confounders can lead to false or nonvalid results. Prominent examples of historically poorly accounted confounding variables include race and race-related variables such as socioeconomic status and demographic distribution. The complex nature of race-related confounds is primarily due to the overarching impact of race on lived experience. Race dictates social experiences such as poverty and stress.4, 5 In this manner, belonging to a particular racial group will likely correlate with specific life experiences. For example, Black communities are more likely to experience socioeconomic stress due to systemic prejudice and racism.4 The relationship between race and socioeconomic status in particular has been subject to a great deal of research due to its substantively confounding effect on biomedical health outcomes.2
Given this context, treating race as a covariate to account for race without adjusting for other race-related confounders may not always be statistically valid.6 Generally, adjusting for race has been a common move by researchers as it does not necessitate splitting the data and lowering the sample size. The severe lack of non-white biomedical data, in large part, is a substantial driving force behind this consideration. However, before treating race as a covariate, one should first establish the research question and whether adding covariates is justified. If trying to account for the effect of race, it is essential first to evaluate all potential race-related confounds, then to adjust for them as covariates in the model if this information is available. If you have enough samples, another solution would be to split the data accordingly to remove the confounding relationship—granted, this cleaves the sample size.7 In cases where this is not possible, a prospective solution is to create a new variable summarizing the correlation between the chosen confounding variables, known as a confounder summary score.3 These summary scores are then added as a covariate, helping to mitigate confounding relationships such as race and adverse past health events.8
Race-related confounders and correlated variables threaten research validity, rendering them key considerations for racial equity in biomedical research.6 As biomedical scientists, accounting for race-related confounds in our research paradigms is crucial to the robustness of our findings and achieving biomedical health equity. By doing so, we can better the healthcare received by racialized communities while actively demonstrating to subjugated communities’ that biomedicine considers their plights socially and statistically.
Stepping Forward: Biomedical Statistics, Data, & Race-Related Confounds
References
1. Greenland, S, Robins, JM (1985). Confounding and misclassification. American journal of epidemiology, 122(3), 495–506. https:// doi.org/10.1093/oxfordjournals.aje.a114131 2. Greenland, S., Morgenstern, H (2001). Confounding in health research. Annual Review of Public Health, 22(1), 189–212. https:// doi.org/10.1146/annurev.publhealth.22.1.189 3. Greenland, S. (2015). Confounder Summary score. Wiley StatsRef: Statistics Reference Online, 1–3. https://doi. org/10.1002/9781118445112.stat05133.pub2 4. Smedley, B. D. (2012). The lived experience of race and its health consequences. American Journal of Public Health, 102(5), 933–935. https://doi.org/10.2105/ajph.2011.300643 5. Brondolo, E., W-Giscombé, C L, Gianaros, PJ, et al. (2017). Stress and health disparities report. American Psychological Association. https://www.apa.org/pi/health-equity/resources/stress-report 6. Jay S. Kaufman, Richard S. Cooper, Commentary: Considerations for the use of Racial/Ethnic Classification in Etiologic Research,
American Journal of Epidemiology, Volume 154, Issue 4, 15 August 2001, Pages 291–298, https://doi.org/10.1093/aje/154.4.291 7. Pourhoseingholi, MA., Baghestani, AR., Vahedi, M. (2012). How to control confounding effects by statistical analysis. Gastroenterology and hepatology from bed to bench. https://www.ncbi.nlm.nih.gov/ pmc/articles/PMC4017459/ 8. Cadarette, SM., Gagne, J J., Solomon, DH., et al. (2010). Confounder summary scores when comparing the effects of multiple drug exposures. Pharmacoepidemiology and drug safety, 19(1), 2–9. https:// doi.org/10.1002/pds.1845
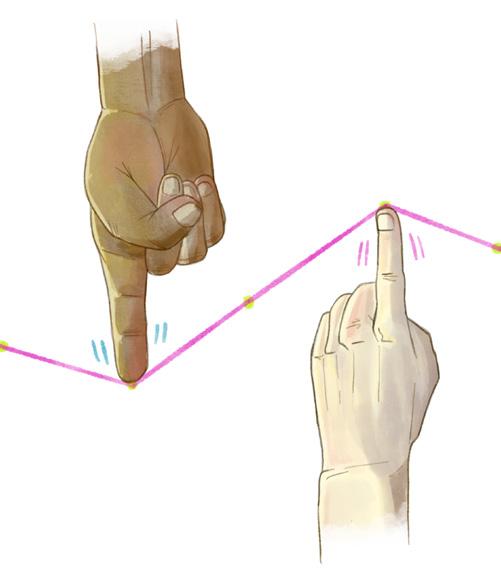