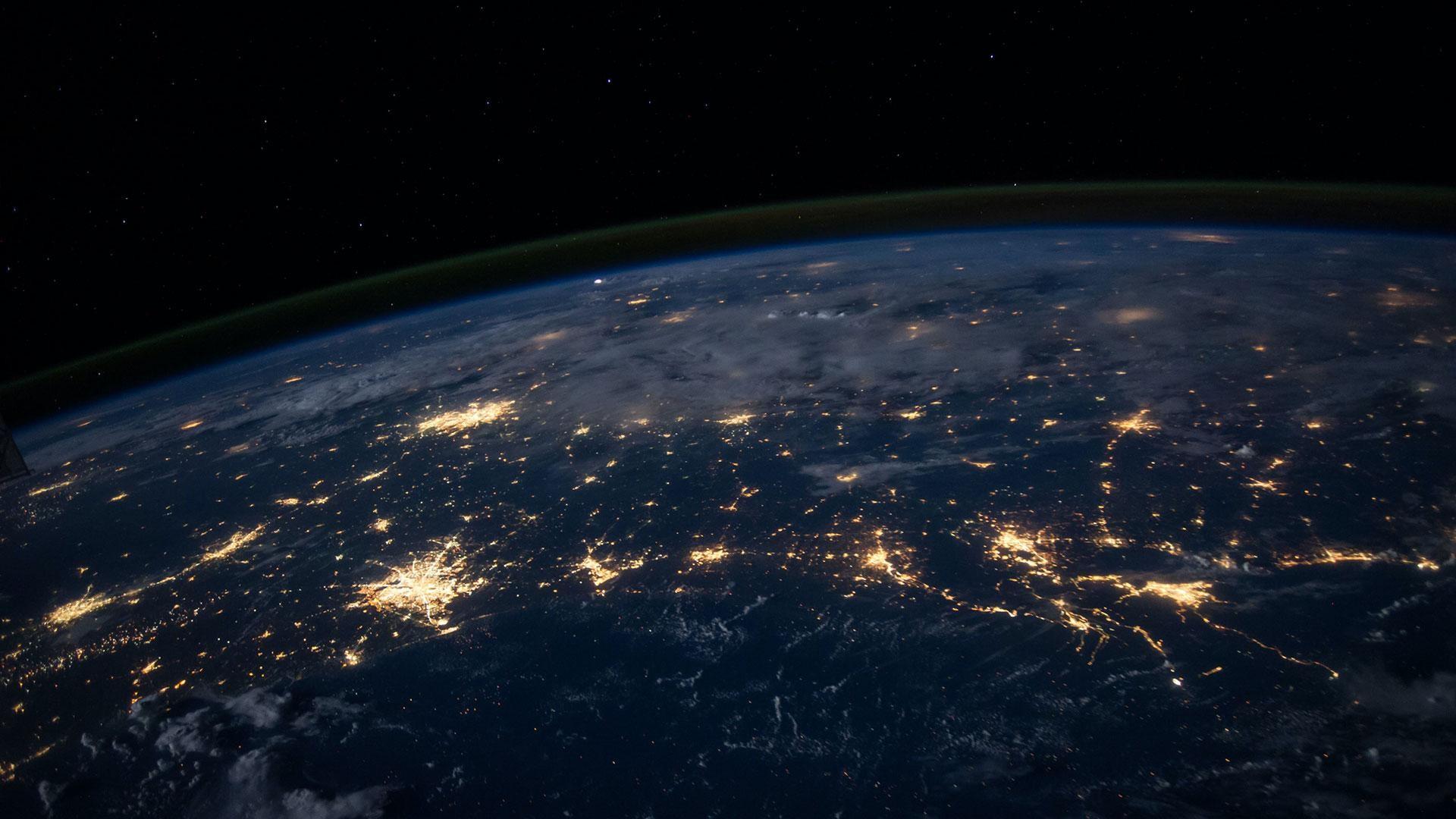

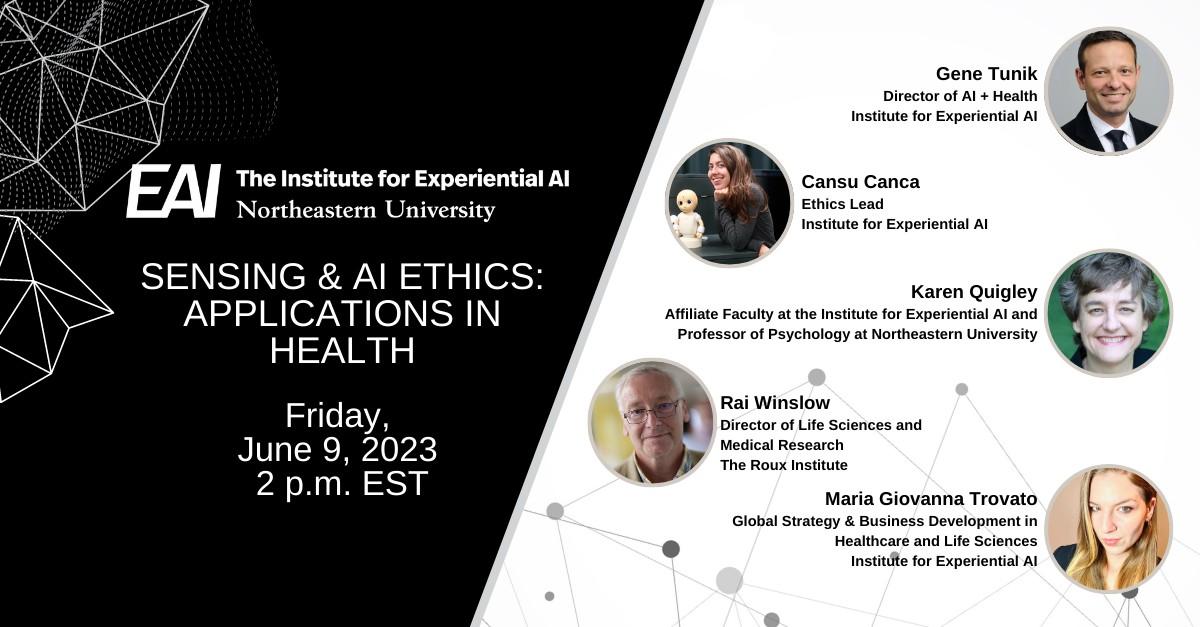
Maria Giovanna Trovato
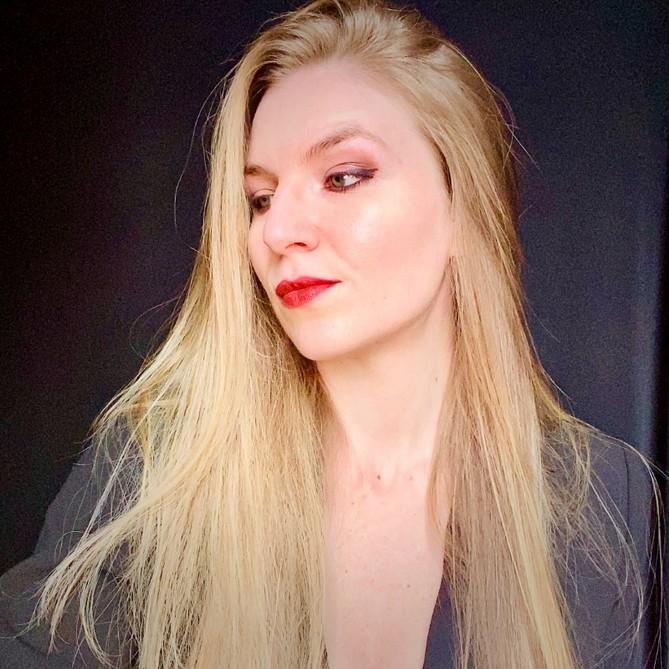
Business Lead for Health and Life Sciences at the Institute for Experiential AI, Northeastern University

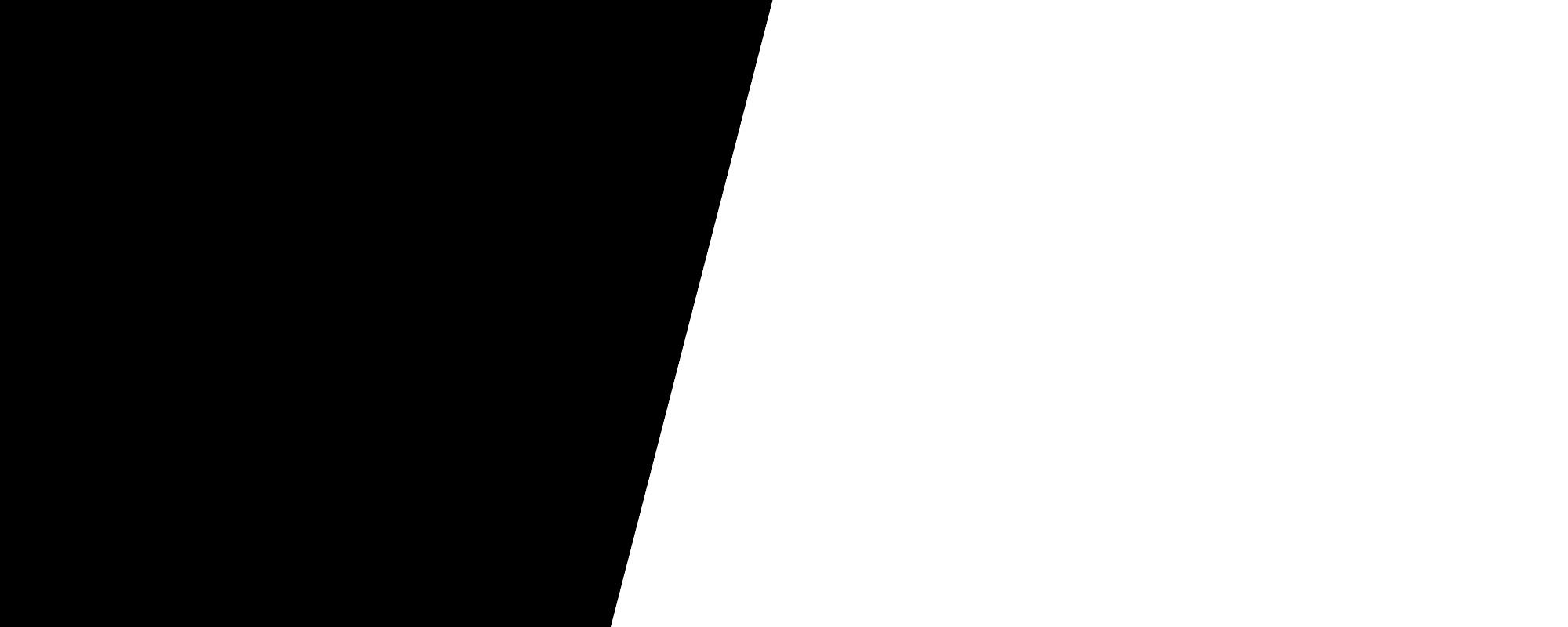
Maria Giovanna Trovato
Business Lead for Health and Life Sciences at the Institute for Experiential AI, Northeastern University
We believe the biggest breakthroughs in the art and science of AI have come—and will come—through applied projects with real-world data that generate tangible results.
The Institute for Experiential AI at Northeastern University researches and develops human-centric AI solutions that leverage machine technology to extend human intelligence.
Successful AI is not ”set it and forget it.” To create real impact, AI must prioritize the human experience and utilize a human in the loop to consistently evaluate and improve its outcomes.
The Institute for Experiential AI exists at
AI has incredible potential to transform human health.
Sensing technology will be critical to reaching that potential.
‘Sensing’ includes technologies like wearables, sleep tracking mats, and motion sensors.
Sensing technologies present incredible potential. And significant risk.
Responsible AI is crucial.
Wearable technology, also known as "wearables," is a category of electronic devices that can be worn as accessories, embedded in clothing, or implanted in the user's body. These devices enable direct interaction between sensors and the end user. They are powered by microprocessors and equipped with the capability to send and receive data via the Internet.
Revolutionizing patient care, diagnostics, and research
Remote Sensing for Health and Healthcare
Wearables and IoT
AI in Diagnostic Medicine
Drug Discovery and Development
Ethics Lead, Institute for Experiential AI Research Associate Professor in Philosophy
Co-Chair, AI Ethics Advisory Board
Director of Research, Institute for Experiential AI
Co-Chair, AI Ethics Advisory Board
Our RAI services are based on the Puzzle-Solving in Ethics (PiE) Model , developed by the AI Ethics Lab.
The PiE Model lays out four core components of AI ethics implementation: Roadmap, Strategy, Analysis, and Training.
Accelerating Health and Healthcare Innovation with Human-Centric AI
Global AI + Health practice that spans our 14 campuses spread across 3 countries and 2 continents.
The Challenge:
• Sparse data collection and in the confines of medical visits
The Solution:
• Continuous monitoring offers real-world data about individuals
• Explainable AI models needed to process and interpret large and complex data
Training
Training Data
Model Training
Fractured Hand (accuracy=.89)
Model Output
• Why does it think there’s a fracture?
• Am I missing something?
• Can I correct the error in the AI algorithm?
Fractured Hand
• Fx with displacement
• Ligament disruption
• Mechanism of injury
• Other symptoms
Training Data Explainable Model Model Explanation
● I understand why the AI prediction
● Decision was based on known input data
● I’d like to tweak the model based on learned features
• Note generation from clinicianpatient interactions
• Real-time visualization of salient data
• Visualization of Real World Evidence / Data
• Ecologically-based data labeling
• Smart sampling
• Data compression
• Auto alert to patient / clinician of adverse events
Raimond Winslow Director of Life Sciences and Medical Research
at the Roux Institute. Professor, Northeastern University College of Engineering
HEART Project: Healthcare Enabled
by AI in Real-TimeData (about patients) Models (of patients) Recommendations (for patients)
In the Cardio-Thoracic ICU (Kramer/ Sawyer/ Winslow)
Monitor Waveforms
Vital Signs
MMC Epic
Advanced prediction of negative outcomes
Feed-back to caregivers
RealTime Comput
Platform
Septic Shock/sepsis
(Median EWT 8 hours)
Liu, R., Greenstein, J. L., Fackler, J. C., Bembea, M.M., Sarma, S. V., Winslow, R. L. (2020). Spectral Clustering of Risk Score Trajectories
Stratifies Sepsis Patients by Clinical Outcome and Interventions
Received. eLife, 2020;9:e58142 DOI: 10.7554/eLife.58142
Pediatric Multiple Organ Dysfunction Syndrome
(Median EWT 37 hours)
PPV 97%
Time Relative to Threshold Crossing (hours)
SN Bose, JL Greenstein, JC Fackler, SV Sarma, RL Winslow, MM
Bembea (2021). Early prediction of multiple organ dysfunction in the pediatric care unit. Frontiers Pediatr. doi: 10.3389/fped.2021.711104
Karen S. Quigley. Ph.D.§ & Lisa Feldman Barrett, Ph.D. §
† *
Northeastern University §
Massachusetts General Hospital †
Harvard Medical School *
The Promise of Wearables for AI + Health is optimized when:
• Data is high-quality, reliable, and multimodal
• Predictive models at the person level, not the group level
• Data is accessible and comprehensible to the user
• Data is secure and protects user privacy
The Promise of Wearables for AI + Health is optimized when:
• Data is high-quality, reliable, and multimodal
• Predictive models at the person level, not the group level
• Data is accessible and comprehensible to the user
• Data is secure and protects user privacy
High-quality, reliable
• Ambulatory physiological monitoring (8 hours/day for 14 days)
• Physiologically-triggered sampling moments (6-8/day)
N = 52 (56% female; M = 22.48 years, SD = 4.38 years)
44 patterns were observed in at least 2 subjects (66%)
1/3 of patterns were unique
The Promise of Wearables for AI + Health is optimized when:
• Data is high-quality, reliable, and multimodal
• Predictive models at the person level, not the group level
• Data is accessible and comprehensible to the user
• Data is secure and protects user privacy
“Gave myself that piece of mind knowing that I don’t have sleep apnea. So, it’s nothing that I should have a concern about being, the numbers being so low. That I’m not even close to like maybe being dangerous. It’s like no, you’re really healthy.”
“It was reassuring that, though I perceive my sleep as being abnormally short, the scientific data says I’m sleeping longer than I think.”
The Promise of Wearables for AI + Health is optimized when:
• Data is high-quality, reliable, and multimodal
• Measures and Algorithms/Models guided by Theory
• Data is accessible and comprehensible to the user
• Data is secure and protects user privacy
Cansu Canca
Ethics Lead at the Institute for Experiential
AI Research Associate Professor in Philosophy
Northeastern University
AI Ethics in Sensing and Wearables: Critical Considerations
Clinician & Researcher
What is the right technology ?
What is the right thing to do?
Hospital & Healthcare system
What is the right health policy?
Clinician & Researcher
AI
What is the right technology ?
What is the right thing to do?
Hospital & Healthcare system
What is the right health policy?
Medical ethics
Public health ethics
Biomedical ethics
Research ethics
Clinician & Researcher
What is the right technology and the right use of it?
Hospital & Healthcare system
Medical ethics
Public health ethics
Biomedical ethics
Research ethics
AI / tech ethics
What is the right thing to do?
What is the right health policy?
Content / product: Developing & designing ethical technologies (AI for Good & Good
AI)
Process: Ensuring ethical processes for tech development
Implementation: Using and implementing tech ethically
Research — Development — Design — Deployment — Update — Monitoring + Procurement (full innovation cycle)
data algorithm tool/UI
practice (HCI)
Content / product: Developing & designing ethical technologies (AI for Good & Good
AI)
Process: Ensuring ethical processes for tech development
Implementation: Using and implementing tech ethically
● data ● user ● user’s environment
● others in relation transparency
● what ● how ● when ● choice? equal outcome
● fairness
● equity accuracy
● define harm
● impact
agency
● HCI
● collaborative decisionmaking
● risk allocation well-being
● impact of information
● individual (user & others)
● societal
● environmental contestability
● expert input
● user input
● data ● user ● user’s environment
● others in relation transparency
● what
● how ● when ● choice? equal outcome
● fairness
● equity accuracy
● define harm
● impact
agency
● HCI
● collaborative decisionmaking
● risk allocation
well-being
● impact of information
● individual (user & others)
● societal
● environmental contestability
● expert input
● user input
agency
● data ● user ● user’s environment
● others in relation transparency
● what ● how ● when ● choice?
● fairness
● equity accuracy
● define harm
● impact
● HCI
● collaborative decisionmaking
● risk allocation well-being
● impact of information
● individual (user & others)
● societal
● environmental contestability
● expert input
● user input
https://aiethicslab.com/big-picture/
informed consent
● online setting
● secondary use of data
● third party models
● withdraw from research
risk & benefit assessment
● minimal risk
● black box
● dual use
● externalities
private information
● re-identification
● group privacy
● data ownership
discrimination / bias
● subject selection
● unrepresentative data
● data with social bias
● proxies
informed consent
● online setting
● secondary use of data
● third party models
● withdraw from research
risk & benefit assessment
● minimal risk
● black box
● dual use
● externalities
private information
● re-identification
● group privacy
● data ownership
discrimination / bias
● subject selection
● unrepresentative data
● data with social bias
● proxies
● Canca & Eto, “AI and Human Subjects
Protection”, CITI Training webinar
● Canca, with Eto & Leong, “AI and Ethics in Human Subjects Research”, CITI Training module
● Eto, with Canca & Leong, “Regulatory
Approaches to AI in Human Subjects
Research”, CITI Training module
● Canca, “Operationalizing AI Principles”, ACM
Communications
● AI Ethics Lab, Dynamics of AI Principles
This slide is an editable slide with all your needs.
Optimize clinical workflow
Enhance accuracy
This slide is an editable slide with all your needs.
Human Centric Approac h
Reduce administrative burden
• Innovation
• Research
• Collaboration
• Ethics
Sensor technology developers
Human Centric Approach
This slide is an editable slide with all your needs.
• Innovation
• Research
• Collaboration
• Ethics
AI algorithm designers
Healthcare Professionals
“If you can dream it, we can help you build it!”
Contact Maria Giovanna at m.trovato@northeastern. edu
Or scan the QR code