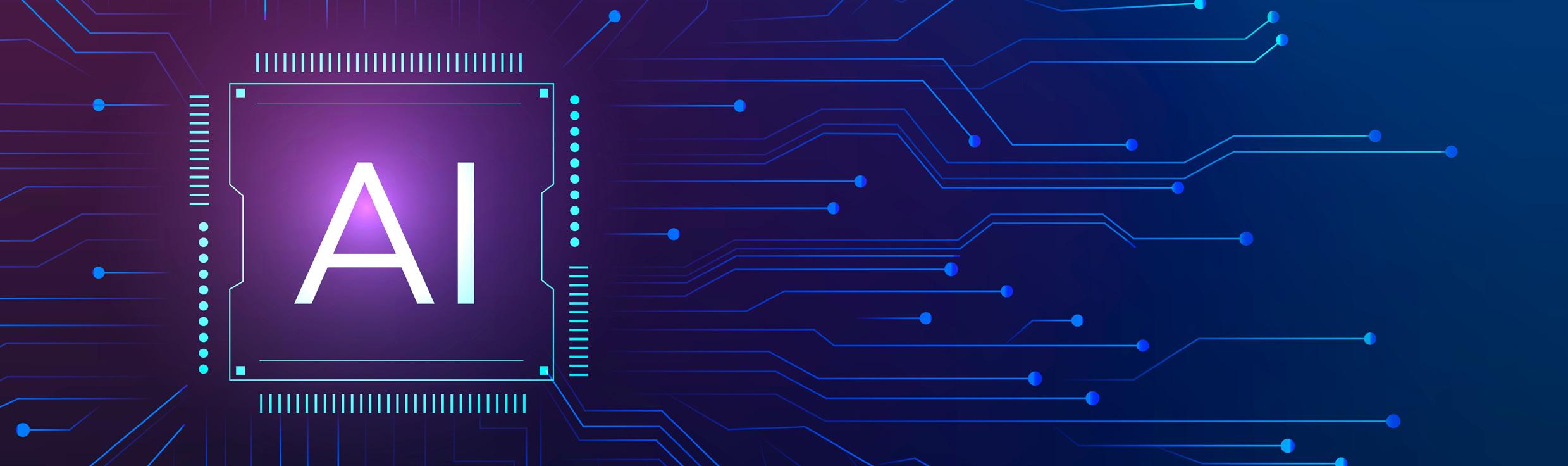
6 minute read
AI in HVAC&R- Part 1: Ethics
by IRHACE
How can AI be successfully used in HVAC&R? Arsen explores the integration of AI into the building services sector and takes a look at the importance of ethical AI practices, transparency, data privacy and mitigating biases to ensure fairness
Words by Arsen Ilhan
The advent of artificial intelligence (AI) is revolutionising various industries, including the building services sector, which encompasses heating, ventilation, air conditioning, lighting, acoustic, security, hydraulic and other integral systems within buildings. The digitalisation of these services through AI promises enhanced efficiency and sustainability. Not only limited to energy efficiency and sustainability, the integration of AI into the HVAC&R industry presents numerous opportunities for reducing costs, enabling predictive maintenance and improving user satisfaction.
According to Review of HVAC Systems History and Future Applications by DeQuante Rashon Mckoy, “With the proper data, development of AI models can, in theory, improve the overall optimisation and reduce energy consumption”. The digitalisation of buildings, driven by advancements in AI, will take the building services industry to another dimension in near future.
However, the adoption of AI in this domain brings forth a multitude of ethical considerations.
Ethical AI practices
The importance of ethical AI extends far beyond compliance; it embodies a commitment to fostering a responsible technological ecosystem. When businesses prioritise ethical use of AI, they not only mitigate risks of legal ramifications but also nurture trust, promote inclusivity and pave the way for a more ethically conscious technological landscape. This approach not only safeguards businesses from potential backlash but also nurtures enduring relationships with stakeholders based on trust, integrity and societal values.
Using AI ethically is crucial for responsible business. It goes beyond just following rules; it’s a commitment to societal values and principles. Ensuring transparency in AI decision-making processes are crucial for building trust between businesses and their stakeholders. When companies clearly communicate how their AI systems function, including the data and algorithms used, it helps establish confidence and reliability in the technology.
Mitigating biases
This openness not only mitigates concerns about potential biases or errors but also fosters a sense of accountability and ethical responsibility, which is essential for maintaining positive relationships with all stakeholders. It’s also about helping people understand why AI reaches specific conclusions. Mitigating biases within AI systems is pivotal to uphold fairness and equity. Biases, often inadvertently embedded in AI algorithms, can perpetuate discrimination. Training data can cause significant issues for AI systems in various ways. Bias in data may lead to biassed AI outputs, as seen when facial recognition systems perform poorly on individuals with darker skin tones due to biassed training images.
Data privacy and transparency
Data privacy issues arise when personal data (e.g. location of the user) is used without consent, potentially violating privacy regulations. Outdated information can result in AI producing inaccurate or irrelevant results, such as using weather data from the 1990s. The quality of data is crucial, as poor quality or mislabeled data can degrade AI performance. Lack of diversity in training data can cause AI to underperform for certain groups or scenarios.
AI systems are only as unbiased as the data they are trained on. In the building services industry, this can translate to biassed decision-making processes, affecting everything from energy distribution to security protocols. For example, an AI system trained on biassed data might disproportionately allocate heating resources or the AI might set temperatures that are uncomfortable for people in more extreme climates or those with different cultural comfort standards, resulting in occupant discomfort and dissatisfaction. It is essential to ensure that the datasets used for training AI models are diverse and representative.
Here are some examples of what constitutes diverse data:
• Geographical diversity
• Building types
• Occupant demographics
• Equipment types and ages
• Energy consumption patterns
• Maintenance record
• Indoor air quality data
• User feedback and preferences
This data diversity can be extended whether it involves design, maintenance or something else. For design applications, ensuring data diversity might include architectural styles, building materials and local climate considerations to optimise HVAC&R systems for various structures. Algorithmic bias occurs when an AI system produces results that are systematically prejudiced due to flawed assumptions in the machine learning process.
This often stems from biassed training data, which can lack diversity or represent certain groups or scenarios disproportionately. Addressing algorithmic bias involves ensuring that training datasets are diverse and representative of all relevant variables and scenarios, continuous monitoring and testing, and incorporating feedback from a wide range of users and settings. This approach helps create AI systems that are fair, accurate and effective across diverse conditions and use cases in the HVAC&R industry.
Mitigating the risks of algorithmic bias
To mitigate the risks of algorithmic bias in AI systems several comprehensive strategies are essential. First, diverse and representative data collection is crucial, involving data from various climate zones, building types and occupant demographics to ensure the model captures a wide range of scenarios and preferences. Data preprocessing and cleaning play a significant role, focusing on bias detection, normalisation and anonymisation to maintain data consistency and privacy.
During algorithm design and training, incorporating fairness constraints, ensemble methods and adversarial training can help reduce biassed outcomes. Continuous monitoring and evaluation are necessary to regularly assess the AI system’s performance and incorporate feedback loops for ongoing improvement. Transparency and explainability are also vital, ensuring that models are interpretable and decisions are clear to stakeholders. Inclusive development practices, involving diverse teams and broad stakeholder engagement, can further reduce unconscious biases.
Finally, employing data augmentation and synthetic data techniques can enhance the diversity of the training dataset, filling gaps and representing underrepresented scenarios and demographics. By integrating these strategies, AI systems in the HVAC&R industry can be developed to be more robust, fair and effective.
Ensuring fairness
One of the pivotal ethical concerns in the integration of AI into building services is the presence of bias and the imperative of ensuring fairness. AI algorithms in HVAC&R systems rely heavily on data inputs from sensors, weather forecasts and historical usage patterns to make decisions about temperature control and energy management. However, these algorithms can inadvertently perpetuate biases present in the data that has been mentioned earlier, leading to unequal treatment or outcomes across different building areas or occupant demographics. Identifying bias in HVAC&R AI involves scrutinising the data sources used for training. Providing clear information to building occupants about how the system works, what data it uses and how it ensures fairness, as well as establishing accountability mechanisms to address any biases or unfair outcomes that are identified, is essential.
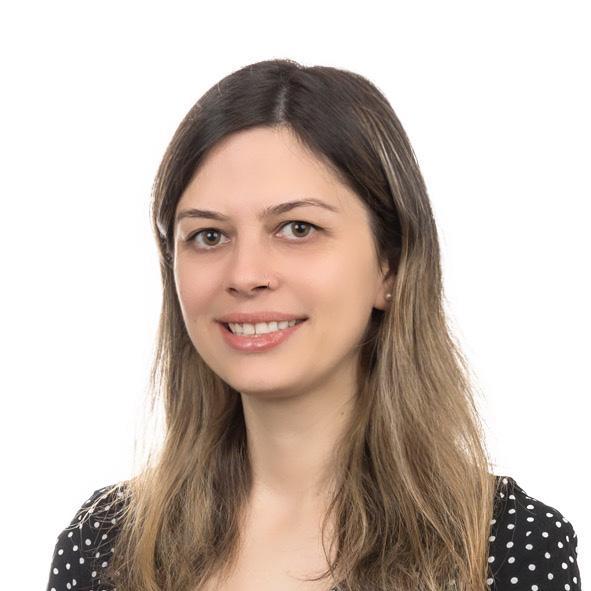
Arsen is a mechanical engineer and currently holds the position of marketing coordinator at the Women in Engineering committee. She actively advocates for increased representation of women in the industry, inspiring and encouraging them to pursue rewarding careers in engineering through Women in Engineering and Women of AIRAH. She has also been a mentor at the Royal Academy of Engineering (UK). As an AI enthusiast, she explores machine learning and its practical applications. She was recognised as a finalist for Young Engineer of the Year by CIBSE in 2023.