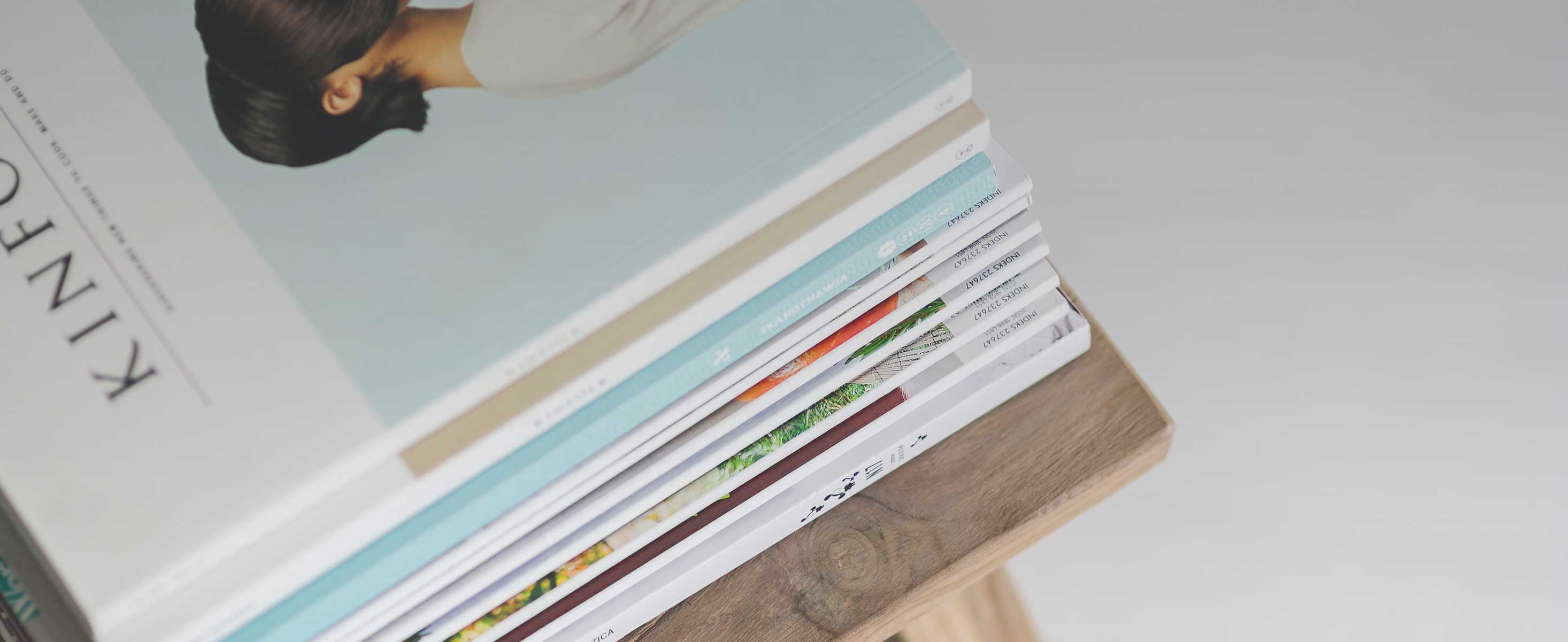
43 minute read
3.4.IPCC OWN TINKERING & TWEAKING CONFESSION
“The presence of H2O in the CO2 band (12-19µ) prevents the increase of temperature due to the saturation of the band, because the combined effect of CO2 and H2O yields an absorptivity that approaches unity, as in the black body case....the presence of H2O in these interval (12-19µ) reduces the effect of CO2 doubling, because the spectrum of CO2 plus H2O gets closer to Planck’s curve, and there is no room for larger increases in the spectrum. This saturation effect limits the temperature increase due to the increase of CO2” Adem and Garduño (1998) in the detailed presentation of the equations ruling their ATM1 computer models.
Going through the section «9.8.3 Implications of Model Evaluation for Model Projection of Future Climate» (Flato and Marotzke et al., 2013) reveals the amazing level of «tinkering» that the authors consider normal in their assessment of the ensemble of models they review. Honestly, for any computer scientist, it is simply flabbergasting. Not only do they confess that it is better if the model(s) are somehow capable of reproducing past variations, amazing as one could have expected that to be the very minimum, but they also naively indicate that when projections of previous IPCC assessments have failed to materialize it is not that serious as «these projections were not intended to be predictions over the short time scales for which observations are available to date». So basically, models are unable to make short term predictions (say a few years to one decade) but we must trust them for making good computations for the Average Mean Temperature, decades from now! Well, not that much because «longer-term climate change projections push models into conditions outside the range observed in the historical period used for evaluation». As if things were not severe enough, they confess that weighing the models, i.e. just ad-hoc tweaking to make things better match, is a reasonable practice. The tuning will be made by adjustments according to past abilities demonstrated by the models to account for past observations not knowing if this will in any case be related to their future ability to forecast anything meaningful «In some cases, the spread in climate projections can be reduced by weighting of models according to their ability to reproduce past observed climate» (Flato and Marotzke et al., 2013) and this goes as far as «the use of unequally weighted means, with the weights based on the models’ performance in simulating past variations in climate, typically using some performance metric or collection of metrics». The cherry on top of the cake is when it is written plain black on white that «Another frequently used approach is the re-calibration of model outputs to a given observed value» which means that making such sort of retro-fitting to anchor off the track computer programmes to some reference data is considered acceptable. What a mess for any computer scientists who has worked in the industry! I just could not believe it.
So models are unreliable, they fail to make any decent projections (at least IPCC honestly acknowledge it) and making weighted averages of them would improve their forecasting ability? Adjusting, tuning, parameterizing the models a posteriori to accommodate ex-post reference data points or observations that could not be properly accounted for in the first place is not a satisfactory practice. This could be somehow acceptable if the underlying physical principles were so sound that such adjustments would have no impact on the basic theories involved, but it is not the case as the computer models are supposed to help validate the AGW theory! Vicious circular reference. Let's make an astrometric analogy: let's compare the situation to an ensemble of incorrect orbits (for the same system), each unable to deliver any reliable ephemeris, and one would think that by making weighted averages of these, one would have any chance of getting an improved orbit ? Astronomers are going to laugh, indeed! This is just a spooky quackery and a feckless tampering of gimmicked models, what an outlandish and ludicrous claim to think that these computerized fantasies bear enough resemblance to reality that coercive policies could be based on them.
One should recall the very basic reasons why Gerlich and Tscheuschner (2009) dismissed climate models “It cannot be overemphasized that even if these equations292 are simplified considerably, one cannot determine numerical solutions, even for small space regions and even for small time intervals. This situation will not change in the next 1000 years regardless of the progress made in computer hardware. Therefore, global climatologists may continue to write updated research grant proposals demanding next-generation supercomputers ad infinitum. As the extremely simplified onefluid equations are unsolvable, the many-fluid equations would be more unsolvable, the equations that include the averaged equations describing the turbulence would be still more unsolvable, if “unsolvable” had a comparative”. Furthermore, these authors elaborate on the issue of boundery conditions and Gerlich and Tscheuschner (2009) state “There are serious solvability questions in the theory of non-linear partial differential equations and the shortage of numerical recipes leading to sufficient accurate results will remain in the nearer or farer future - for fundamental
292MHD-type global climatologic equations
mathematical reasons. The Navier-Stokes equations are something like the holy grail of theoretical physics, and a brute force discretization with the aid of lattices with very wide meshes leads to models, which have nothing to do with the original puzzle and thus have no predictability value. In problems involving partial differential equations the boundary condition determine the solutions much more than the differential equations themselves. The introduction of a discretization is equivalent to an introduction of artificial boundary conditions, a procedure, that is characterized in von Storch’s statement “The discretization is the model”. Thus there is simply no physical foundation of global climate computer models, for which still the chaos paradigma holds: Even in the case of a well-known deterministic dynamics nothing is predictable [201]. That discretization has neither a physical nor a mathematical basis in non-linear systems is a lesson that has been taught in the discussion of the logistic differential equation, whose continuum solutions differ fundamentally from the discrete ones [202, 203]”
For these and many more reasons, Gerlich and Tscheuschner (2009) assert “In conclusion, the derivation of statements on the CO2 induced anthropogenic global warming out of the computer simulations lies outside any science”.
The conclusion here could be borrowed from Morel (2013) “We are always making more images or "special effects" more and more disconnected from reality. This is one of the causes of the discredit to which the scientific community has exposed itself. Real scientists, like Professor Bolin, performed the measurements they needed on the ground themselves. Professor Bolin, more than any other, should have felt the danger of substituting for reality observations, numbers debited on demand by computers. He should never have let the IPCC embark on the path of "virtual reality" created by models. Scientific integrity requires that a formal distinction be maintained between the conclusions of objective observations of nature and the hypotheses illustrated by numerical simulations” (Morel, 2013)
«In sum, a strategy must recognize what is possible. In climate research and modeling, we should recognize that we are dealing with a coupled non-linear chaotic system, and therefore that the long-term prediction of future climate states is not possible. Rather the focus must be upon the prediction of the probability distribution of the system’s future possible states by the generation of ensembles of model solutions. The most we can expect to achieve is the prediction of the probability distribution of the system’s future possible states by the generation of ensembles of model solutions.» p.774. - IPCC – 2001 – TAR-14 – «Advancing Our Understanding».
The models are unreliable, they are in “disagreement” with the observations for more than 15 years, i.e. they fail to model and to predict correctly but IPCC have high confidence in them «There is hence very high confidence that the CMIP5 models show long-term GMST trends consistent with observations, despite the disagreement over the most recent 15-year period. Due to internal climate variability, in any given 15-year period the observed GMST trend sometimes lies near one end of a model ensemble, an effect that is pronounced in Box TS.3, Figure 1a, b as GMST was influenced by a very strong El Niño event in 1998.» (IPCC, 2013).
The elementary tuning of or across the models leads to discrepancies that are higher than the major effects searched for, e.g. it is amazing to see that the effect of the differences in CMIP3 PI ensemble inter-model average planetary albedo is greater than the supposedly effect of a doubling of CO 2: “We have partitioned the earth’s planetary albedo into a component due to the reflection of incoming radiation by objects in the atmosphere α P,ATMOS and a component due to reflection at the surface α P,SURF. In the global average, the vast majority (88%) of the observed planetary albedo is due to α P,ATMOS. The CMIP3 PI ensemble inter-model average planetary albedo is also primarily due to α P,ATMOS (87%). The inter-model spread in global average planetary albedo is large, corresponding to radiative differences at the top of the atmosphere (2σ = 5.5 W m-2) that exceed the radiative forcing of doubling carbon dioxide” (Donohoe and Battisti, 2011). The only thing it shows, is that we only deal with models having more than a hundred parameters to tune them to help produce what results are expected and even like that, that they keep changing their “predictions” and that the more they keep changing the less we trust them, even though they are supposed to be improved from one generation to the next!
The study from Zelinka et al. (2020) addresses how climate sensitivity is dealt with across latest CMIP6 models. It seems that the logic followed is sort of a headlong rush, always predicting more warming and trying to ever find new means of doing so. The latest arbitrary choice comes up with the representation of clouds, without any rationale to support their choices, both the water content and the areal coverage of low-level clouds decrease more strongly with greenhouse warming in the latest models, causing enhanced planetary absorption of sunlight, which provides for the long awaited amplifying feedback that ultimately results in more warming! Zelinka et al. (2020) report “Here we show that the closely related effective climate sensitivity has increased substantially in Coupled Model Inter-comparison Project phase 6 (CMIP6), with values spanning 1.8–5.6 K across 27 GCMs and exceeding 4.5 K in 10 of them. This
(statistically insignificant) increase is primarily due to stronger positive cloud feedbacks from decreasing extra-tropical low cloud coverage and albedo. Both of these are tied to the physical representation of clouds which in CMIP6 models lead to weaker responses of extra-tropical low cloud cover and water content to unforced variations in surface temperature”. So, does one can have any idea of the way clouds are being represented in these models, and why there should be more or less ? The glimpse of an answer is offered by the worthless notion of parametrized physics as Zelinka et al. (2020) add “The sensitivities of cloud properties to CCFs293 are typically estimated via multi-linear regression applied to inter-annual covariations of meteorology and clouds in the unperturbed climate. Models exhibit widely varying cloud sensitivities owing to diversity in how clouds, convection, and turbulence are represented via parameterized physics “. As Gerlich and Tscheuschner (2009) reminded us, later supported by Kramm and Dlugi (2011), this pseudo-physics of parametrized computations and sensitivities estimated by multi-linear regressions applied to whatever covariation are simply meaningless!
The only thing that these studies, investigating “forcing”, “feedbacks”, and “climate sensitivity” in abrupt “CO 2 quadrupling experiments” conducted in the latest generation of fully coupled GCMs as part of CMIP6 demonstrate, is how the modelers have completely run amok and lost any connection to physics, reality and the way the Earth's climate slowly reacts and adapts to ever changing conditions. Zelinka et al. (2020) end their paper with a glimmer of lucidity “This raises the possibility that ECS is indeed high in the real world, but it first needs to be established that CMIP6 feedbacks and forcing are in quantitative agreement with these constraints. It is possible, for example, that higher ECS in models from larger extratropical low cloud feedbacks might simply be revealing (as yet unknown) errors in other feedbacks. Such a conclusion would also need to be evaluated in light of other evidence. For example, how well do high ECS models simulate past climates or the historical record? While some high ECS models closely match the observed record (e.g., Gettelman et al., 2019), others do not (e.g., Golaz et al., 2019). Do the former models achieve their results via unreasonably large negative aerosol forcings and/or substantial pattern effects (Kiehl, 2007; Stevens et al., 2016)?” So most of the latest models are simply unable to account for past climate up to LIA, they keep nudging up fear levels for an overnight quadrupling (!) of [CO2] (why not more?) by resorting to obscure modeling techniques better called tricks or gibberish, and those that perform somehow better could find means to do so by making an unreasonable usage of aerosol to cool down the past! Science has lost its mind, but as DOE orders and fat grants flow in, one must deliver what he's been paid for. Finally, the only reasonable sentence of this comprehensive appraisal of the the latest generation of models is “Establishing the plausibility of these higher sensitivity models is imperative given their implied societal ramifications” (Zelinka et al., 2020). No doubt, politicians are on the right track, let's destroy our economies and our societies, for models that even those at the heart of their development wonder – with some honesty – what is their plausibility ! Let's remind what that means, for the Cambridge dictionary “seeming likely to be true, or able to be believed”. Highly unlikely forecasts delivered by these models must be believed! This is a religion not science any longer.
Some hinsight into the sequence of evolution of these Global Climate Models (GCMs) can be obtained by studying the way a group of authors teamed up under the leadership of Hansen starting with (Hansen et al., 1984) to slowly move the target away from any climate reconstruction objective to software platforms designed to numerically simulate the “forcing” of various components. These GCMs were certainly not able to predict climate in 1997 and at least acknowledged by then the chaotic nature of the phenomenons studied, Hansen (1997) wrote “Indeed, the climate system exemplifies "complexity," a combination of deterministic behavior and unpredictable variations ("noise" or "chaos"). Interactions connect all parts of the system, giving rise to complex dynamical patterns that never precisely repeat. The slightest alteration of initial or boundary conditions changes the developing patterns, and thus next year's weather is inherently unpredictable. This behavior results from the nonlinear fundamental equations governing the dynamics of such a system (Lorenz, 1963)”.
In the next five years, the authors focused on modeling whatever greenhouse gas catastrophe could be predicted from arbitrary increases of various “forcings”, with little regard to the influence of the clouds, the oceans and the Sun and it was pretty clear that these GCMs could not account for even the little ice age, much less the interglacial warming and the Holocene. Hansen (2002) wrote “The present simulations, carried out on a Silicon Graphics 2000 system, focus on the past 50-year period and include additional forcings and models. Some of the experiments now being carried out for 1951 to present (see Table 3) are using a version of the model reprogrammed, documented, and optimized for parallel computations but nominally with the same physics as in SI2000. The aim is to find a practical path leading to a prompt new round of experiments for longer period, 1850 – 2000, including improvements in the realism of both forcings and models”. So basically, as of 2002, these GCMs were hardly capable of accounting for the last 50 years of observations
293CCF= Cloud Controlling Factor
and mainly focused as per the authors on the inclusion of “additional forcings”. The Little Ice Age (LIA), was a remote objective, not even really considered as 1850 was set as tentative and remote mark. As long as any of these models are completely unable to account to what led to the end of the LIA and to a reversal of the conditions, how could they benefit of the slightest credibility?
As noticed by Glassman (2009) “All by themselves, the titles of the documents are revealing. The domain of the models has been changed from the climate in general to the “inter-annual and decadal climate”. In this way Hansen et al. placed the little ice age anomaly outside the domain of their GCMs. Thus the little ice age anomaly was no longer a counterexample, a disproof. The word “forcing” appears in each document title. This is a reference to an external condition Hansen et al. impose on the GCMs, and to which the GCMs must respond. The key forcing is a steadily growing and historically unprecedented increase in atmospheric CO2. “Efficacy” is a word coined by the authors to indicate how well the GCMs reproduce the greenhouse effect they want.”
In fact, the change from Global Climate Models to Global Circulation Models, acknowledges the abandonment of these authors of the goal to predict global climate and according to the objectives they set to themselves, “The accuracy and sensitivity of their models is no longer how well the models fit earth’s climate, but how well the dozens of GCM versions track one another to reproduce a certain, preconceived level of Anthropogenic Global Warming” (Glassman, 2009). It is worth noticing that in these GCMs, no part of the CO 2 concentration is a consequence of other variables (e.g. such as the temperature, accounting for the increased out-gassing of the oceans according to Henry's law) and these GCMs appear to have no provision for the respiration of CO2 by the oceans. They neither account for the uptake of CO 2 in the cold waters, nor the exhaust of CO2 from the warmed and CO2 saturated waters, nor the circulation by which the oceans redistribute through down-welling and later up-welling the CO2 from the pole to the tropics.
As of 2005, these authors started considering other important factors, such as how loosely modeled the clouds were or how the “forcings” would produce rather regional effect than global ones, Hansen et al (2005) asserting “global forcing has more relevance to regional climate change than may have been anticipated. Increasing greenhouse gases intensify the Hadley circulation in our model, increasing rainfall in the Inter-tropical Convergence Zone (ITCZ), Eastern United States, and East Asia, while intensifying dry conditions in the subtropics including the Southwest United States, the Mediterranean region, the Middle East, and an expanding Sahel. These features survive in model simulations that use all estimated forcings for the period 1880–2000”. With respect to the influence of the clouds, one will be happy to learn that until 2005 it did not dawn on these authors that they might have an important effect, (i.e. actually a regulating one) and Hansen et al (2005) write “Clouds affect the amount of sunlight absorbed by the Earth and terrestrial radiation to space. Even small imposed cloud changes can be a large climate forcing. Cloud changes due to human aerosol and gaseous emissions or natural forcings such as volcanic emissions and incoming cosmic rays are difficult to quantify because of the large natural variability of clouds, cloud feedbacks on climate that occur simultaneously with imposed cloud changes, and imprecise knowledge of the driving human and natural climate forcing agents. In the meantime, cloud forcings in climate models are probably best viewed as sensitivity studies. Various observational constraints allow rationalization of the overall magnitude of assumed cloud forcings, but these constraints are imprecise and their interpretations are debatable”. When clouds are so roughly accounted for, does tuning the software to respond mainly to hypothetical CO2 forcing make sense ?
The answer may be brought by Voosen (2016) “Climate models render as much as they can by applying the laws of physics to imaginary boxes tens of kilometers a side. But some processes, like cloud formation, are too fine-grained for that, and so modelers use "parameterizations": equations meant to approximate their effects. For years, climate scientists have tuned their parameterizations so that the model overall matches climate records. But fearing criticism by climate skeptics, they have largely kept quiet about how they tune their models, and by how much. That is now changing. By writing up tuning strategies and making them publicly available for the first time, groups hope to learn how to make their predictions more reliable—and more transparent”. The trouble is that the damage is done, the Pandora Box is now open, and one can see in it the can of worms that needs heavy parametrization to achieve what are not lackluster results but truly deceptive predictions. What is unbelievable is that these “climate models” guide regulations like the U.S. Clean Power Plan, and inform U.N. temperature projections and calculations of the social cost of carbon when they are highly unsuitable for any kind of decent prediction nor even proper rendering of past climate observations. This is well acknowledged by the disclosure of the constant tuning required by all modeling teams (Voosen, 2016), but also crystal clear after the analysis made by Curry and Webster (2011) and Curry (2016a-b, 2017). In fact, everybody knows the deception and Indeed, whether climate scientists like to admit it or not, nearly every model has been calibrated precisely to the 20th century climate records—otherwise it would have ended up in the trash. “ It’s fair to say all models have tuned it,” says Isaac Held, a scientist at the Geophysical Fluid Dynamics Laboratory, another
prominent modeling center, in Princeton, New Jersey. More importantly, claiming that “climate models”, i.e. softwares, represent the direct application of the laws of physics is also far fetched for various reasons: the first is that the equations of Henry Navier are of great interest but of little immediate usage without a computer as in most cases they cannot be solved analytically and as it has not yet been even proven whether solutions always exist in three dimensions and, if they do exist, whether they are smooth – i.e. they are infinitely differentiable at all points in the domain, and therefore this situation has led the Clay Mathematics Institute in May 2000 to make this problem one of its seven Millennium Prize problems in mathematics294. It offered a US $1,000,000 prize to the first person providing a solution for this specific statement of the problem: In three space dimensions and time, given an initial velocity field, there exists a vector velocity and a scalar pressure field, which are both smooth and globally defined, that solve the Navier–Stokes equations. Despite their relative simplicity of form, Navier-Stokes equations also have this property of generating extremely complex behaviors, apparently random and unpredictable: they indeed have, under certain conditions, explosive amplification properties of very small disturbances or errors (“chaos”) which make them unusable directly for simulating or predicting turbulent flows (“butterfly effect”) due to the too large number of scales and structures, some instability, too great sensitivity to initial data and boundary conditions, both of the flow and of the equations (DeMoor and André, 2005). Most of the mathematical difficulties linked to Navier-Stokes equations, partial differential equations with respect to time t and to position coordinates x i, have their origin in the non-linearity (with respect to the speed field) of the term representing the acceleration of the fluid particle: decomposed according to such partial derivatives, it indeed appears as the sum of a linear “trend” ∂ ⃗ u/∂ t and a quadratic nonlinear “advection” (⃗ u . gradient )⃗ u where ⃗ u is the velocity field.
Therefore, from the “basic” laws of physics as elicited by Henry Navier and George Stokes, i.e. a set of partial differential equations in space and time for a set of state variable, a lot remains to be done to accommodate them to some usage by computer programmes and Müller and von Storch (2008) remind “This requires first the discretization of the equations, both in space and time. The process of deriving the governing differential equations includes several closures through parametrizations and approximations. These equations are transformed into a discrete, finite form which allows for a digital implementation on a computer”. The closure problem is the consequence of the fact that it is impossible to represent all the processes within the system, to incorporate the surroundings and to resolve all scales; in that respect, “Example 2.1.” given by Müller and von Storch (2008) dealing with cloud formation is very revealing and shows the complexity of the issues addressed. One can easily understand that these models, although of a great complexity, have necessarily to simplify a lot the real world to cope with it and also heavily depend on the discretization techniques used. This make them suitable for meteorological forecasts, or for theoretical studies in atmospheric and oceanic sciences, but certainly not as means to make decadal or centennial temperature projections to determine calculations of the social cost of carbon, an heresy in itself. They are and remain just research instruments and were unfortunately purposely diverted from their original mission to serve as a surety means to give credence to the anthropogenic explanation promoted by IPCC (they do not search for any other!). The outcome is that policy makers relying on the information delivered by these ad-hoc models had no idea of the uncertainties embedded in these climate simulations and hence in their conclusions and the implications for their policies. The damage to science will be incommensurable as it will be difficult to explain in layman terms why one can have confidence in astrometric calculations delivering an ephemeris of Apophis for example (Figure 56), but why “climate models” were fantasies and failed to make any decent account of past or future climate states.
“It’s not just the fact that climate simulations are tuned that is problematic. It may well be that it is impossible to make long-term predictions about the climate – it’s a chaotic system after all. If that’s the case, then we are probably trying to redesign the global economy for nothing” Judith Curry (2017).
Moving away from strictly radiative (-convective) circulation models, research teams have started to consider that the Earth is a far more complex system than what is portrayed by studies focusing just on an increase in CO 2 and their alleged consequences. In that respect, the approach described by Heavens et al. (2013) is interesting as long as the model and the computer software that goes along is taken for what it is, i.e. a means to study an extremely complex system and not a means to make forecasts on which to base policies. Here is how the authors describe their effort “Studying how biological processes and climate are related requires a new type of climate model: the Earth system model (ESM). ESMs include physical processes like those in other climate models but they can also simulate the interaction between the physical climate, the biosphere, and the chemical constituents of the atmosphere and ocean. ESMs are chiefly distinguished from climate models by their ability to simulate the carbon cycle. If the sum of all CO 2 emitted into the atmosphere between 1966 and 2008 is compared with the observed level of atmospheric CO 2,
294http://www.claymath.org/millennium-problems/navier%E2%80%93stokes-equation
approximately one of out of every two CO2 molecules appears to be missing (Figure 2). This extra CO 2 has not vanished entirely. It has been incorporated into land and ocean reservoirs, often in carbon fixed by organisms during photosynthesis. Whether all of it will stay there and what proportion of future emissions will remain in the atmosphere are open questions, which have motivated the development of land model components that can predict the spatial distribution of vegetation, how its growth varies through the year, and the exchange of carbon between it and the soil. Similar model components exist to simulate the marine biosphere and chemistry”. One can sense from this excerpt the extraordinary complexity of the system modeled and the fact that whatever the progress we can make and the computing means we can allocate, we can just expect as Gerlich and Tscheuschner (2009) reminded us, well … models and one should be very cautious not taking them for the reality!
Hopefully, the role of the oceans operating as a biological carbon pump is taken now more effectively into account. Buesseler et al. (2020) observe that “Earth system models, including those used by the UN/IPCC, most often assess POC (particulate organic carbon) flux into the ocean interior at a fixed reference depth” using an idealized and empirically based flux-vs.-depth relationship, often referred to as the “Martin curve”, but observe that “We find that the fixeddepth approach underestimates BCP efficiencies when the Ez295 is shallow, and vice versa”.
Moving on to a completely different approach, probably much more realistic, is to acknowledge that the Earth Climate System is such a complicated issue with intricate variables and unknown responses that it does not make sense to try to model it the way the GCMs do and to rather follow a technique known as system identification. “The field of system identification uses statistical methods to build mathematical models of dynamical systems from measured data. A common approach is to start from measurements of the behavior of the system and the external influences (inputs to the system) and try to determine a mathematical relation between them without going into many details of what is actually happening inside the system; this approach is called system identification”296. Classical references are (Ljung and Glad, 1994) or (Isermann and Münchhof, 2011), in French one could mention, e.g. (Bako, 2008) or (Bastin, 2013).
An example of such an approach is Golyandina and Zhigljavsky (2013) where black box models applied to the energy balance of the planet directly give climatic sensitivities to the equilibrium with respect to three inputs: CO 2, solar activity and volcanic dust.
In his book, “Climate Change: Identifications and projections” de Larminat (2014) deals with the issue of climate modeling in a different way: by using proven techniques for identifying black box-type models. “Taking climate observations from throughout the millennia, the global models obtained are validated statistically and confirmed by the resulting simulations. This book thus brings constructive elements that can be reproduced by anyone adept at numerical simulation, whether an expert climatologist or not. It is accessible to any reader interested in the issues of climate change”. de Larminat (2014; 2016) uses well-known techniques in identifying industrial processes, from several historical reconstructions of temperatures (eg Moberg, Loehle, Ljungqvist, Jones & Mann) and from several series representing solar activity (Usoskin-Lean, Usoskin -timv, Be10-Lean, Be10-timv) of the last millennium and even until the year 843, without a priori assumptions. A very careful analysis of confidence intervals and confidence domains leads to the results summarized as follows:
(1) observations cannot demonstrate the anthropogenic origin of global warming; neither climate sensitivity to
CO2 nor even its sign can be said with confidence; (2) solar activity is the main factor of climate change and its role (sensitivity in °C / (W/m²)) is underestimated by a factor of 10 to 20 by the IPCC; the IPCC starts from physical considerations on the smallness of the variations of the total solar irradiance (TSI); but the black box model applied to the series of observations gives a much higher sensitivity and the solar activity explains most of the warming since the end of the Little Ice Age.
Therefore, de Larminat (2014) demonstrates very clearly that for such a complex system as the Earth's climate, system identification techniques deliver objective and convincing results such as:
• the warming period which led to the contemporary optimum is essentially due to the combined effect of solar activity and natural variability (which is found in residues, like the 60-year cycles which result from parameters which are not taken into account in this black box model);
295Ez is the sunlit euphotic zone, the layer closer to the surface that receives enough light for photosynthesis to occur. 296https://en.wikipedia.org/wiki/System_identification
• the anthropic contribution, if it exists, is not distinguished enough from the preceding effects for one to claim to see it, and certainly not with the high degree of certainty displayed by the IPCC.
The margin of error and uncertainty calculations and the hypothesis tests provide all the necessary validations from a scientific point of view. Furthermore, as reported by Veyres (2020) “a more visual demonstration of the accuracy of the results found is the agreement between the calculation results and the observations and the predictive capacity of the model; blind simulations without any information on temperatures after the year 2000 show with surprising accuracy the "plateau" observed in global warming since 2000. For these short-term predictions, state estimates by Kalman filters are used, where the state reflects the accumulation of heat in the oceans. In addition to sensitivities, the method provides a rigorous assessment of the probability that a parameter is within a certain interval, without all of these very subjective statements of "confidence" or "likelihood" or "subjective probability" that adorn each paragraph of the IPCC WG1 report and of which Rittaud (2010; 2015) has emphasized the non-scientific nature”.
All models proceed by conventional flux adjustments as explained by Kerr (1994) “In climate modeling, nearly everybody cheats a little. Although models of how the ocean and the atmosphere interact are meant to forecast the greenhouse warming of the next century, when left to their own devices they can't even get today's climate right. So researchers have tidied them up by "adjusting" the amount of heat and moisture flowing between model's atmosphere and ocean until it yields something like the present climate”. In that respect, Nakamura et al. (1994) introduced deliberately an error and demonstrated that coupled ocean-atmosphere GCMs that require adjustments in the surface fluxes of heat and freshwater to achieve some resemblance to current climate conditions do not account for the real sensitivity of the real climate. This was clearly summarized by Kerr (1994) "Mototaka Nakamura, Peter Stone, and Jochem Marotzke of the Massachusetts Institute of Technology (MIT) report that they deliberately introduced an error into a climate model, then seemingly adjusted the error away, only to find that it still hampered the model's ability to predict future climate”. Coupling the atmospheric and oceanic components was inevitable as otherwise the atmospheric software component relied totally on getting SSTs and the amount of heat released by the oceans on observation data which prevented the systems from forecasts capacities and Kerr (1994) adds that this coupling “left the job of calculating the interactions of the ocean and the atmosphere to the less-than-perfect models themselves. If the atmospheric component made more clouds than in the real world, not enough sunlight would get through to warm the ocean; if the ocean currents did not carry enough warm water poleward, high latitudes would be too cold. The result was that even when a coupled model was set up to simulate the existing climate, it would drift away to something quite unreal. In the 1989 version of the NCAR coupled model, for example, winter-time ocean temperature around ice-bound Antarctica were 4°C above zero, while the tropical ocean was as much as 4°C too cold ". It is always reassuring to think that people argue a lot about a supposed 0.6 or 0.4°C warming per century when their models are several degrees apart from basic observations.
The truth is that the best meteorological models and corresponding software simulation systems must be reminded of the reality by plugging them in actual observation data every six hours or so, otherwise they fall into the ditch and ironically during COVID-19, and because the frequency of overseas flights was considerably decreased, observations made by commercial flight were not available any longer as usual and the quality of meteorological forecasts decreased considerably. In fact most models are tweaked with fudged flux-adjustments and this up to the point, that not too far in the past Kerr (1994) stated “Actually, shove might be a better word than nudge: Adjustments have typically been at least as big as the model-calculated fluxes - in some places five times as large". Syukuro Manabe admitted though defending the practice that the Geophysical Fluid Dynamics Laboratory (GFDL) in Princeton, also did compensate underlying errors with flux-adjustments, for example as stated by Kerr "because of computational biais, the GFDL model assumed an unrealistically large amount of precipitation in high latitudes - an error he and his colleagues corrected with a moisture flux adjustment”. Amazingly, Syukuro Manabe stated “Compensating in kind for a fictitious climate feature is harmless”. I am sure that if the reader had some doubts, he feels much better now. Manabe also claimed that an increase of computer power will reduce flux-adjustments in the future, but as reminded by Browning (2020) “The total error E can be considered to be a sum of the errors: E = D + S + T + F + I“ and increasing the power of the computer does not solve the issues at stake. In a similar way to the experiment performed by Nakamura et al. (1994), Krasovskiy and Stone (1998) demonstrated that the model representation of THC in the simulators could be seriously deteriorated whenever other errors were corrected by flux-adjustments “The approximate analytic solutions are in good agreement with Marotzke’s exact numerical solutions, but show more generally how the destabilization of the thermohaline circulation depends on the sensitivity of the atmospheric transports to the meridional temperature gradient. The solutions are also used to calculate how the stability of the thermohaline circulation is changed if model errors are ‘‘corrected’’ by using conventional flux adjustments”.
Coupling convincingly the Ocean-Atmosphere circulation boxes have been and remain an on-going a challenge, but adding ice-components, land usage, vegetation, freshwater budgets and all geochemical processes requires a lot of faith to think that this will tell anything of how planet Earth will really behave. Moving from the time-scale of prediction of the meteorological systems, basically one week, to just one month or slightly more is demonstrated as a major challenge by the forecast of exceptional events such as heat waves, e.g. (Weisheimer et al., 2011; Stéfanon, 2012). Nakamura (1994) in his D.Sc. thesis studied the influence of planetary-scale flow structure on the evolution of synopticscale297 waves and how these synoptic eddies exhibit complex behavior when strong diffluence 298 in the low-frequency flow (defined as blocking), is observed and displays a strong relationship with high-frequency synoptic scale eddies. This D.Sc. work led naturally to the simulation study of the 2003 heatwave in Europe reported in Nakamura et al. (2005), for which the Atmospheric general circulation model For the Earth Simulator, AFES i.e. a massively-parallel-vector supercomputer (Ohfuchi et al., 2004) was used. Nakamura et al. (2005) explain that because of the seemingly lowfrequency nature of the dynamics behind the heatwave of 2003, it serves well as a test case for low-frequency state hind-casting and tried to “reproduce the heatwave in AFES with the observed daily SST, starting one month before the heatwave. The resolution used for this study was T639L48, truncation wave number of 639 and 48 vertical levels. There are 6 levels in the planetary boundary layer, 28 levels in the troposphere, and 14 levels in the stratosphere”.
Apart from the control run where all observed SSTs were given to the system, the results of the other runs do not appear overly encouraging. Nakamura et al. (2005) conclude that “a coarse-resolution model (perhaps even T639 used here) is unlikely to simulate the event well even if all the external forcings, including the SST, are given. This is because the model cannot adequately resolve the nonlinear processes involved in the positive feedback of high-frequency waves onto the diffluent low-frequency flow”. Furthermore, making any one month or slightly more previsions, not 2,000 years or the Holocene, supposes that the state of the art would have the “ability to forecast the SST and land surface temperatures, in addition to its ability to accurately represent the internal dynamics of the atmospheric low-frequency state. This means that such a long-range forecast model must have the atmosphere up to the top of the stratosphere, all oceans, the land surface, and perhaps the ice, interacting dynamically and thermodynamically with each other. Needless to say, the model must be able to accurately represent those second-order variables, such as the cloudiness, precipitation, and soil moisture, that are important for low-frequency forcing. Finally, but never the least, observational network must be improved to provide a reasonable initial condition to the forecast model”. One can sense from this concrete example of the prevision of heat-waves, one month or so ahead of their occurrence, why this represents a major challenge and why running models for hundreds or worse thousands of years into the future (or the past feeding them data and adjusting fluxes) does not appear too realistic nor rational. As just reported, Nakamura is a long standing expert and has always been cautious, especially as adding more and more component does not ensure more reliability, especially if the underlying processes are not well understood and represented. In Nakamura (2013) the sudden change in the reference Greenland Sea surface temperature (GSST) is interpreted as resulting from “a major change in the near-surface baroclinicity in the region, in addition to a large change in the net surface heat flux at the air–sea boundary over the Greenland Sea” and related these modifications to changes in the North Atlantic Oscillation (NAO) index. From thereof, it is stressed that without a proper understanding of the various processes in the Arctic and subArctic regions and appropriate representation in climate simulation models no short- to mid-term climate variations, not to mention longer-term climate variations or changes could be considered reliable. Perhaps, the ever more fanciful claims of the IPCC experts stating that the models are ready to reproduce the climate in all details for centuries, milleniums or are even “validated” because they account for climate events over geological times (for sure if they just read the tape backwards) have been the straw that broke the camel's back of this honest scientist when he decided to publish on the sorry state of climate science “Confessions of a climate scientist: the global warming hypothesis is an unproven hypothesis” in Nakamura (2018) with a summary given by Thomas (2019) in “A Climate Modeler Spills the Beans”. What Nakamura (2018) says is well worth reading as this is the result of 25 years of academic work beyond his MIT D.Sc. in the domain and does not exactly match the consensus that is sold to us on each and every occasion:
“The temperature forecasting models trying to deal with the intractable complexities of the climate are no better than toys or Mickey Mouse mockeries of the real world”.
“The global surface mean temperature-change data no longer have any scientific value and are nothing more than a propaganda tool to the public”.
297The synoptic scale in meteorology (also known as large scale or cyclonic scale) is a horizontal length scale of the order of 1000 kilometers or more. This corresponds to a horizontal scale typical of mid-latitude depressions (e.g., extratropical cyclones) 298Diffluence in meteorology is a widening of the pressure isolines in the direction of the wind. Diffluence corresponds to a deformation of the pressure field without any associated vertical movement.
“Climate forecasting is simply impossible, if only because future changes in solar energy output are unknowable. As to the impacts of human-caused CO2, they can’t be judged with the knowledge and technology we currently possess”.
Other gross model simplifications include: Mototaka Nakamura
• Ignorance about large and small-scale ocean dynamics • A complete lack of meaningful representations of aerosol changes that generate clouds. • Lack of understanding of drivers of ice-albedo (reflectivity) feedbacks: “Without a reasonably accurate representation, it is impossible to make any meaningful predictions of climate variations and changes in the middle and high latitudes and thus the entire planet.” • Inability to deal with water vapor elements • Arbitrary “tunings” (fudges) of key parameters that are not understood
Mototaka Nakamura
“I want to point out a simple fact that it is impossible to correctly predict even the sense or direction of a change of a system when the prediction tool lacks and/or grossly distorts important non-linear processes, feedbacks in particular, that are present in the actual system. The real or realistically-simulated climate system is far more complex than an absurdly simple system simulated by the toys that have been used for climate predictions to date, and will be insurmountably difficult for those naïve climate researchers who have zero or very limited understanding of geophysical fluid dynamics. The dynamics of the atmosphere and oceans are absolutely critical facets of the climate system if one hopes to ever make any meaningful prediction of climate variation. Solar input, absurdly, is modelled as a “never changing quantity”. It has only been several decades since we acquired an ability to accurately monitor the incoming solar energy. In these several decades only, it has varied by one to two watts per square metre. Is it reasonable to assume that it will not vary any more than that in the next hundred years or longer for forecasting purposes? I would say, No”.
Mototaka Nakamura
“Good modelling of oceans is crucial, as the slow ocean currents are transporting vast amounts of heat around the globe, making the minor atmospheric heat storage changes almost irrelevant. For example, the Gulf Stream has kept western Eurasia warm for centuries. On time scales of more than a few years, it plays a far more important role on climate than atmospheric changes. It is absolutely vital for any meaningful climate prediction to be made with a reasonably accurate representation of the state and actions of the oceans. In real oceans rather than modelled ones, just like in the atmosphere, the smaller-scale flows often tend to counteract the effects of the larger-scale flows. The models result in a grotesque distortion of the mixing and transport of momentum, heat and salt, thereby making the behaviour of the climate simulation models utterly unrealistic. Proper ocean modelling would require a tenfold improvement in spatial resolution and a vast increase in computing power, probably requiring quantum computers. If or when quantum computers can reproduce the small-scale interactions, the researchers will remain out of their depth because of their traditional simplifying of conditions”.
Mototaka Nakamura
“The models are ‘tuned’ by tinkering around with values of various parameters until the best compromise is obtained. I used to do it myself. It is a necessary and unavoidable procedure and not a problem so long as the user is aware of its ramifications and is honest about it. But it is a serious and fatal flaw if it is used for climate forecasting/prediction purposes. One set of fudges involves clouds. Ad hoc representation of clouds may be the greatest source of uncertainty in climate prediction. A profound fact is that only a very small change, so small that it cannot be measured accurately… in the global cloud characteristics can completely offset the warming effect of the doubled atmospheric CO 2. Two such characteristics are an increase in cloud area and a decrease in the average size of cloud particles”.
Mototaka Nakamura
“Accurate simulation of cloud is simply impossible in climate models since it requires calculations of processes at scales smaller than 1mm. Instead, the modellers put in their own cloud parameters. Anyone studying real cloud formation and then the treatment in climate models would be flabbergasted by the perfunctory treatment of clouds in the models. In tuning some parameters, other aspects of the model have to become extremely distorted. A large part of the forecast global warming is attributed to water vapor changes, not CO2 changes. But the fact is this: all climate simulation models perform poorly in reproducing the atmospheric water vapor and its radiative forcing observed in the current
climate. They have only a few parameters that can be used to ‘tune’ the performance of the models and (are) utterly unrealistic. Positive water vapor feedbacks from CO2 increases are artificially enforced by the modelers. They neglect other reverse feedbacks in the real world, and hence they exaggerate forecast warming. Modellers are merely trying to construct narratives that justify the use of these models for climate predictions”.
Mototaka Nakamura
“The take-home message is that all climate simulation models, even those with the best parametric representation scheme for convective motions and clouds, suffer from a very large degree of arbitrariness in the representation of processes that determine the atmospheric water vapor and cloud fields. Since the climate models are tuned arbitrarily …there is no reason to trust their predictions/forecasts. With values of parameters that are supposed to represent many complex processes being held constant, many nonlinear processes in the real climate system are absent or grossly distorted in the models. It is a delusion to believe that simulation models that lack important nonlinear processes in the real climate system can predict (even) the sense or direction of the climate change correctly”.
Mototaka Nakamura
Having read what one of the most knowledgeable scholar in the field thinks after 25 years of top-level research accomplished after his D.Sc. obtained at MIT in 1994, one may better appreciate the level of politicized science, in fact a mere advertisement for gullible laymen, that is delivered in a well designed, full of nice images prospectus marketed by the Australian Academy of Science stating “Climate models allow us to understand the causes of past climate changes, and to project climate change into the future. Together with physical principles and knowledge of past variations, models provide compelling evidence that recent changes are due to increased greenhouse gas concentrations in the atmosphere” (AAS, 2015), p. 4. If you cannot believe it, read it again and remember:
“If you tell a lie big enough and keep repeating it, people will eventually come to believe it. The lie can be maintained only for such time as the State can shield the people from the political, economic and/or military consequences of the lie. It thus becomes vitally important for the State to use all of its powers to repress dissent, for the truth is the mortal enemy of the lie, and thus by extension, the truth is the greatest enemy of the State.” Joseph Goebbels299
Finally, one important point is that the models can only be as good as the data they use.
Pierre Morel is a well known French scholar (retired), a theoretical physicist (Statistical quantum mechanics). He is the founder of the Laboratory of Dynamic Meteorology (LMD) of Paris VI University, ENS, CNRS, in 1968. Among other eminent functions, Pierre Morel was Director General of the French Space Agency in charge of science and technology (1975-1982), then Director of the International Research Program on the Global Climate (1982-1994). This is what he stated (2009) “Any climatological reconstruction, based on direct or indirect instrumental measurements, is subject to systematic interpretations and corrections of the same order of magnitude as the variations expected for average global quantities. We could not therefore find more fertile ground for controversies and quibbles of all kinds, based on more or less partisan interpretations of quantitative information necessarily crushed by specialists. The evolution of the global climate is simply too small up to now (compared to the random meteorological variations and the uncertainty of the observation data) to allow an assured diagnosis of the long-term changes, even less the identification of putative cause and effect relationships based on correlations between two or more uncertain "climate signals". In terms of interpretation of climatic signals, the intensive (and passionate) examination of global data is similar to the Rorschach test: we find what we want, it is impossible to reach a scientifically indisputable conclusion based on the sole consideration of global average quantities deduced from archived observations (a fortiori from historical or paleoclimatic reconstructions” (Morel, 2009).
299https://www.jewishvirtuallibrary.org/joseph-goebbels-on-the-quot-big-lie-quot