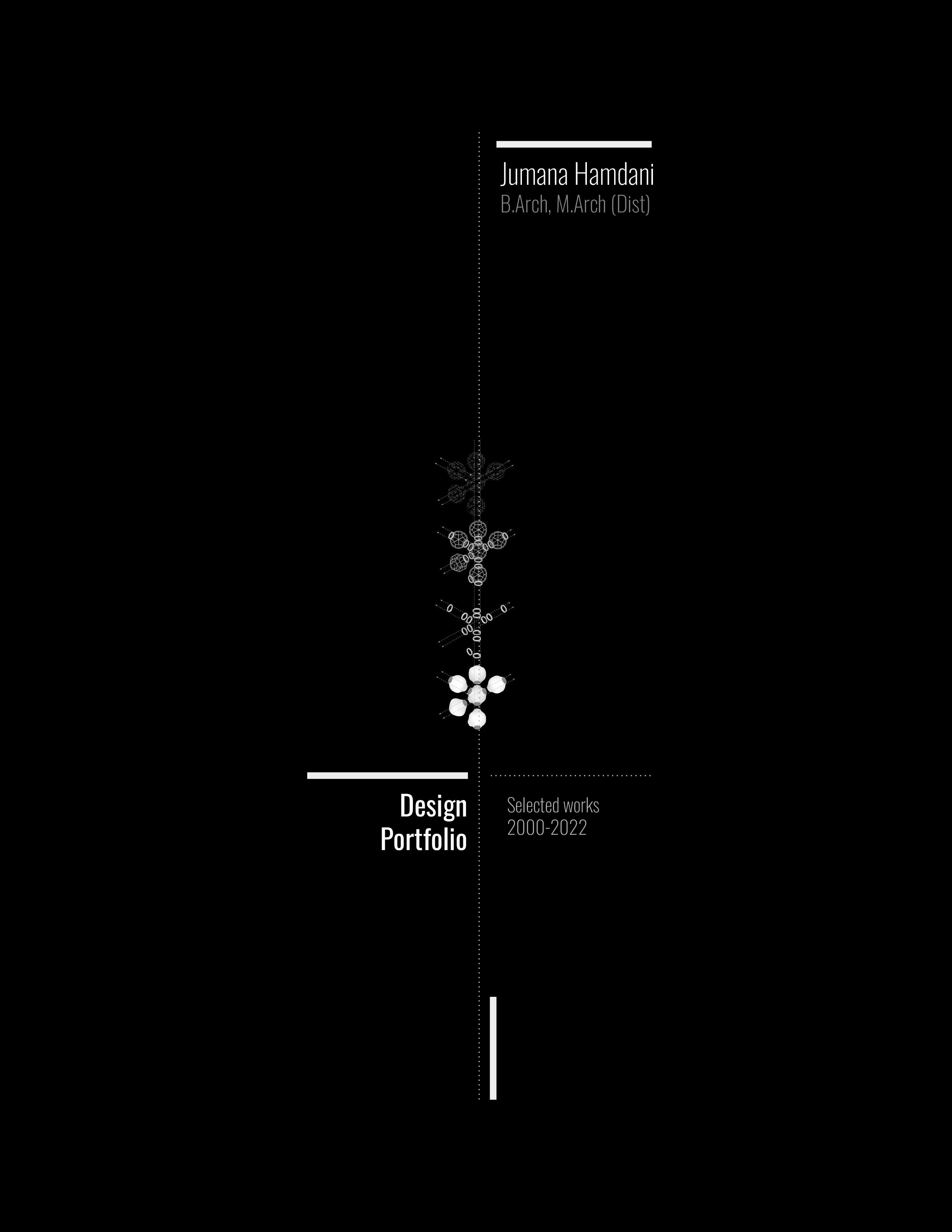
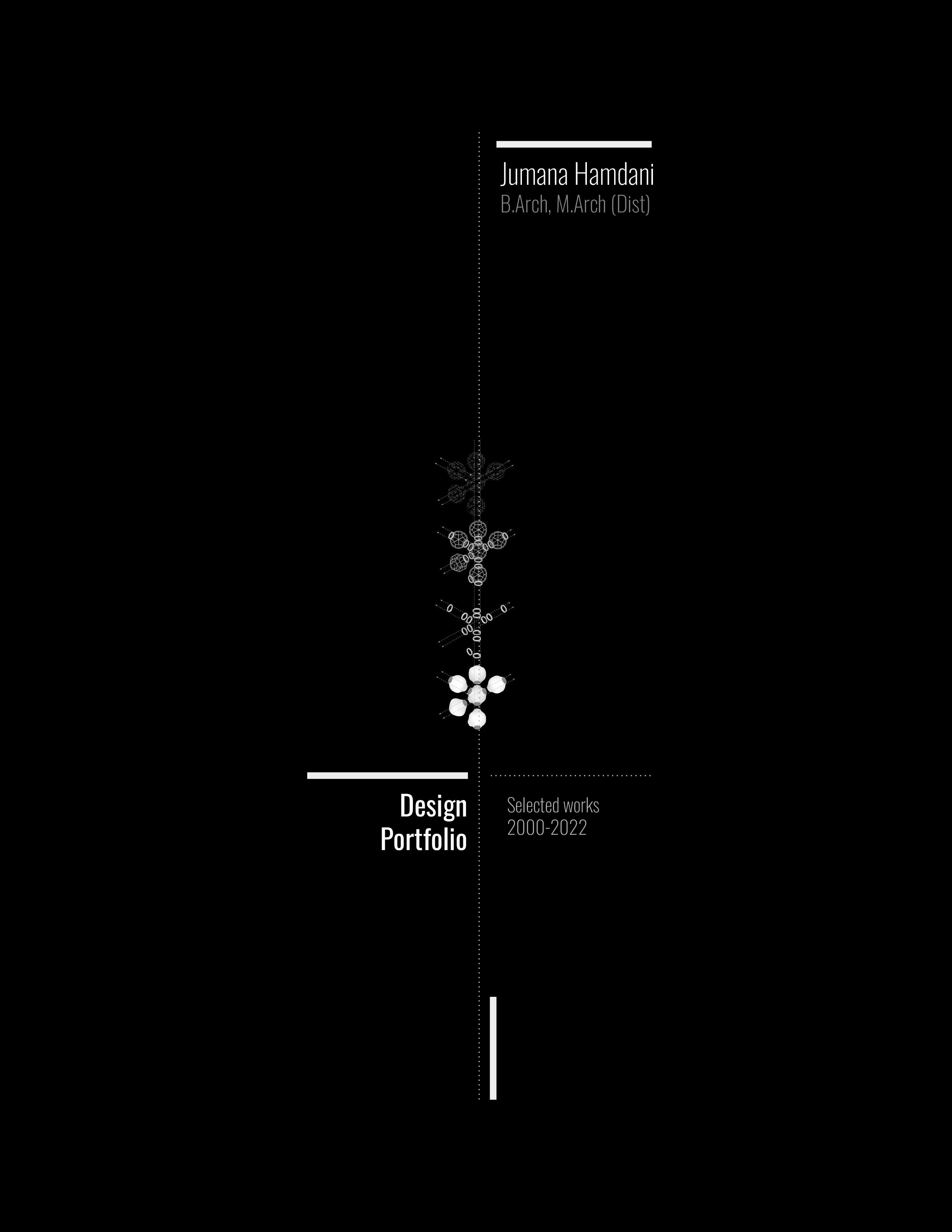
TABLE OF CONTENTS
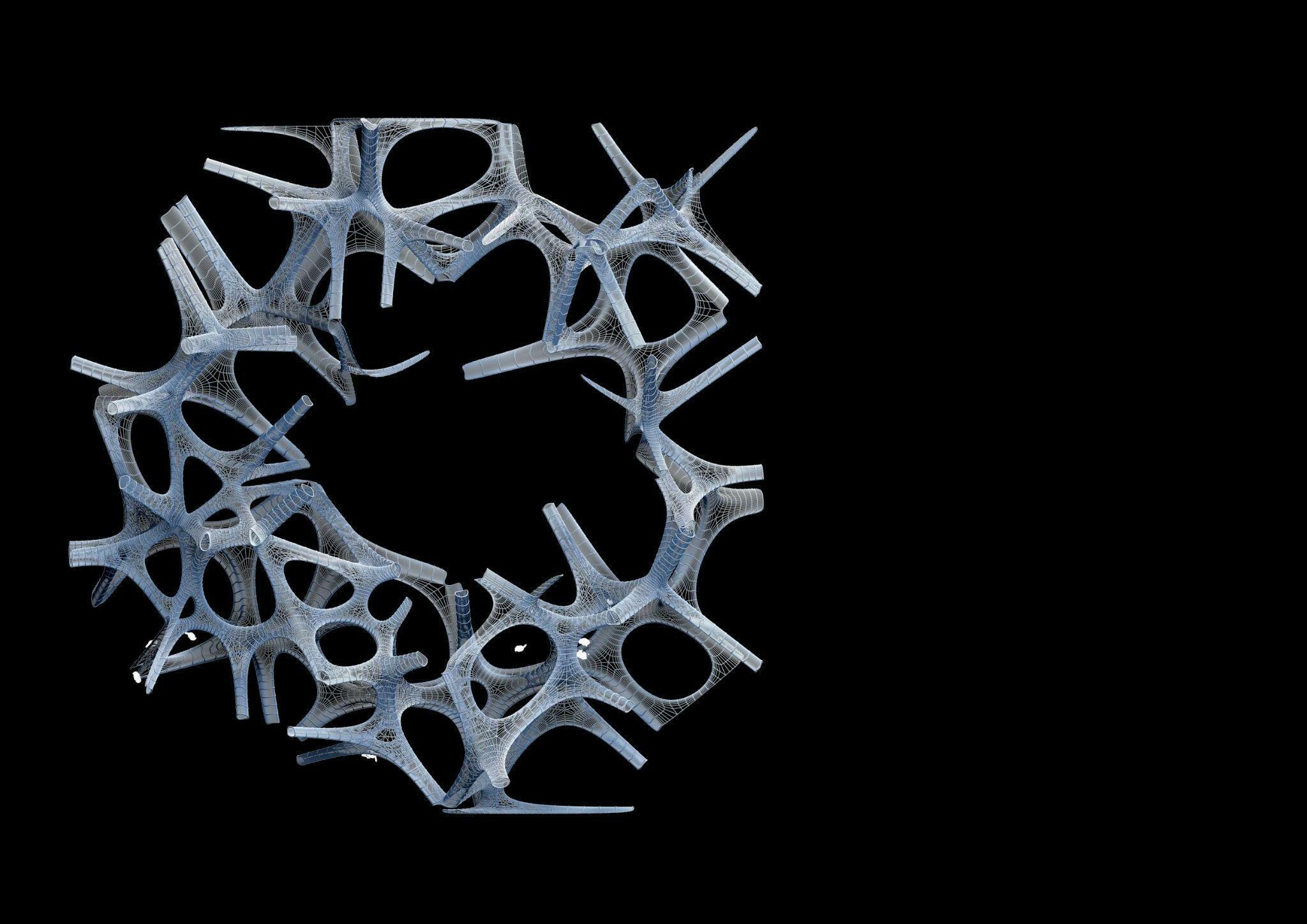
05 Walking Courtyards Karlskrona, Sweden, 2022 06 Walk 21 Seoul Conference Seoul, South Korea, 2020 07 Salt Pavilion Uyuni, Bolivia, 2021 08 Espejo Project Uyuni, Bolivia, 2021 09 Wings of Change Competition San Jose, USA 10 Future City Baghdad, Iraq, 2000 11 Mörrum Conference Center Karlshamn, Sweden,2009 12 Arab Open University Amman, Jordan, 2006 13 Emblem Structure Dubai, UAE, 2007 14 London Environmental Analysis London, UK, 2021 15 What’s My Plaza For? Madrid, Spain, 2022 16 Depth City 2022 17 Game of Green Vienna, Austria, 2022 18 Subway City Munich, Stockholm, Prague, 2022 19 Northern Light Pavilion Lapland, 2022 20 Nebulae: Orbital Space Habitat Lower Earth Orbit, 2022 21 Fuzzy Concept Exhibition Ronneby, Sweden, 2012 22
Sketchbook 23 Research Trip Valldaura Self-Sufficient Labs - IAAC, Barcelona, Spain, 2022
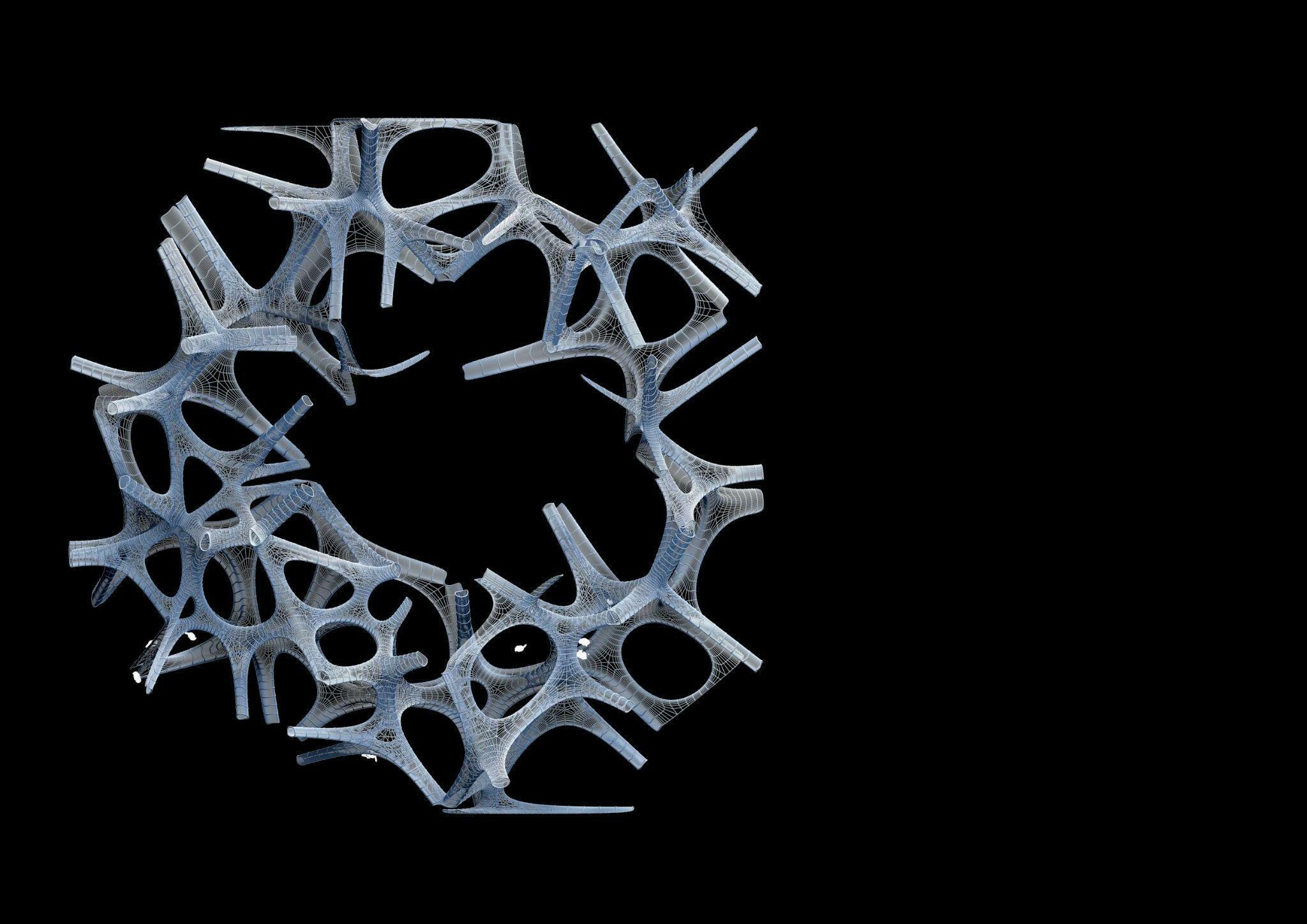
PROFILE 01
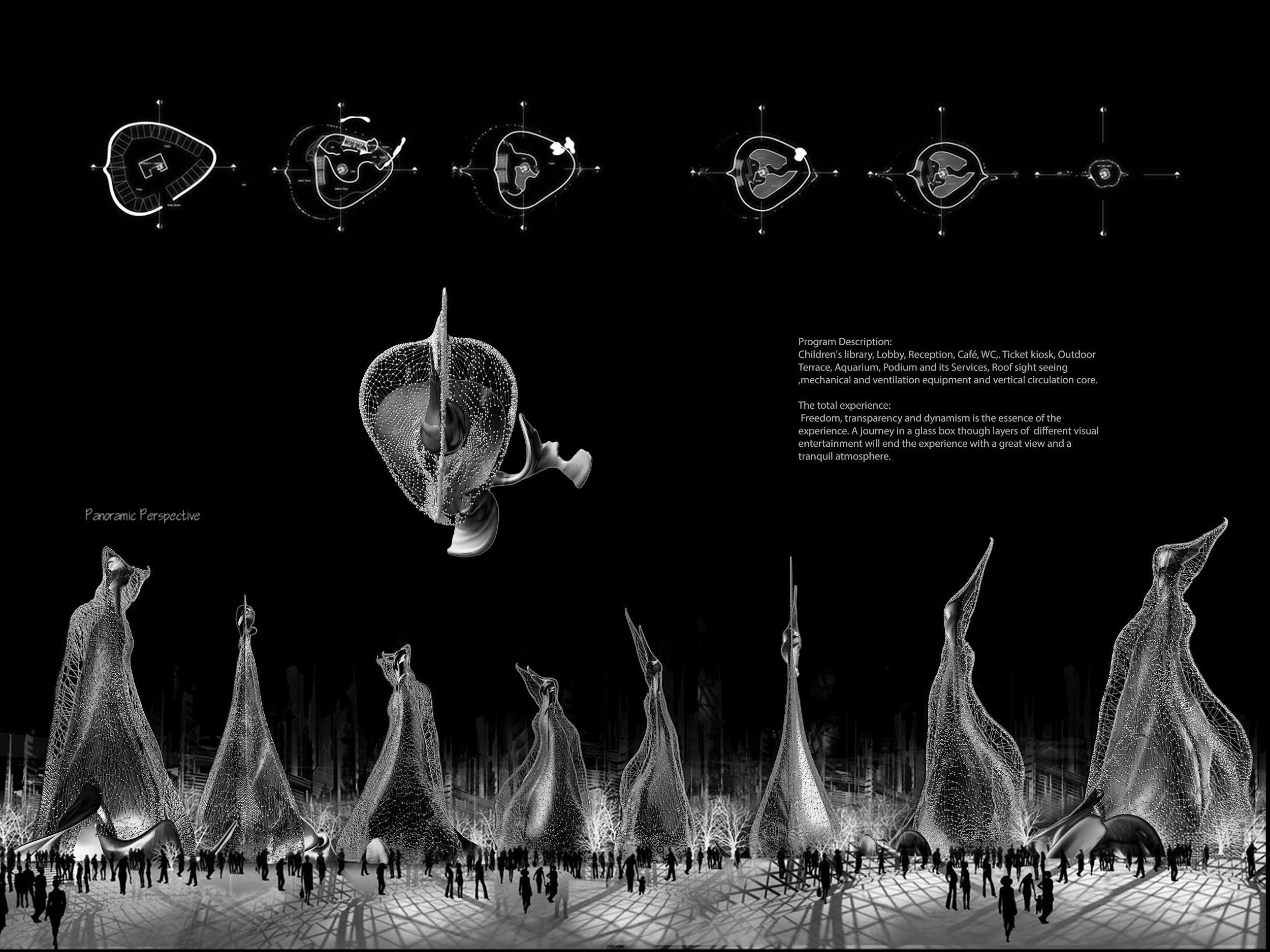
Jumana Hamdani
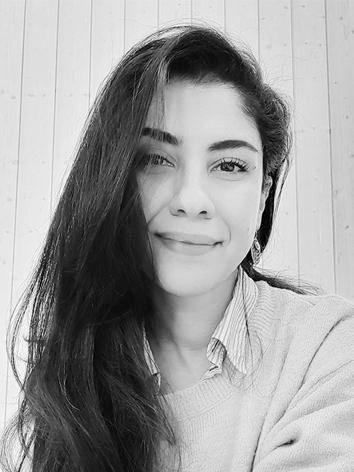
B.Arch, M.Arch (Dist)
Architect SAR/MSA and educator at The Swedish School of Planning, BTH. Founder of Jumana Hamdani Architects based in Karlshamn, Sweden. Holds a Master's degree in Advanced Computation for Architecture and Design (Dist) from The Institute for Advanced Architecture of Catalonia in Barcelona and has been a designer of several public and private projects in Asia, Africa, and Europe for the past two decades. Worked in the field of Architectural Computational Design, BIM and Parametric Modelling, Space Habitat Architecture, Urban Analytics, Spatial Networks, Environmental and Structural Design Analysis, developing AI Methods, and Machine Learning Strategies for resilient neighbourhoods. A member of the Swedish Architecture Association and the international list of the German Architectural Association of Baden-Württemberg, AKBW.
WORK MAP
Space Habitat in LEO
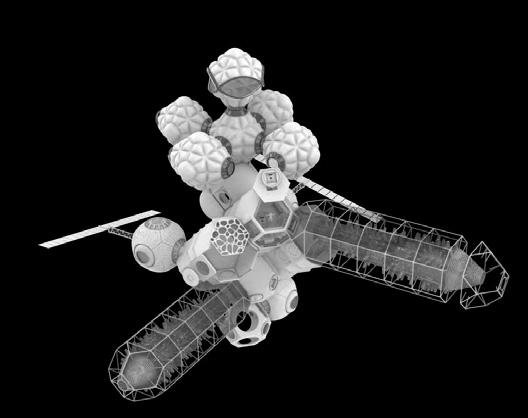
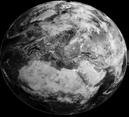
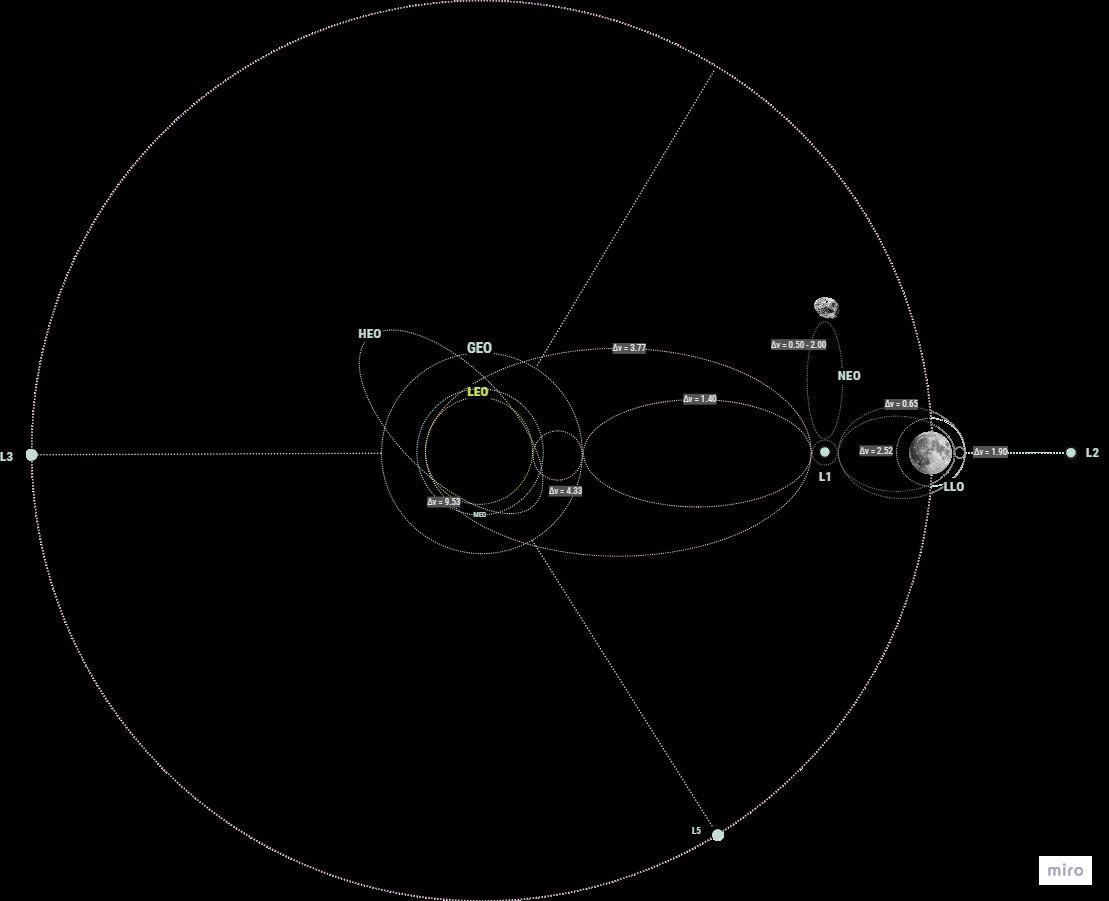
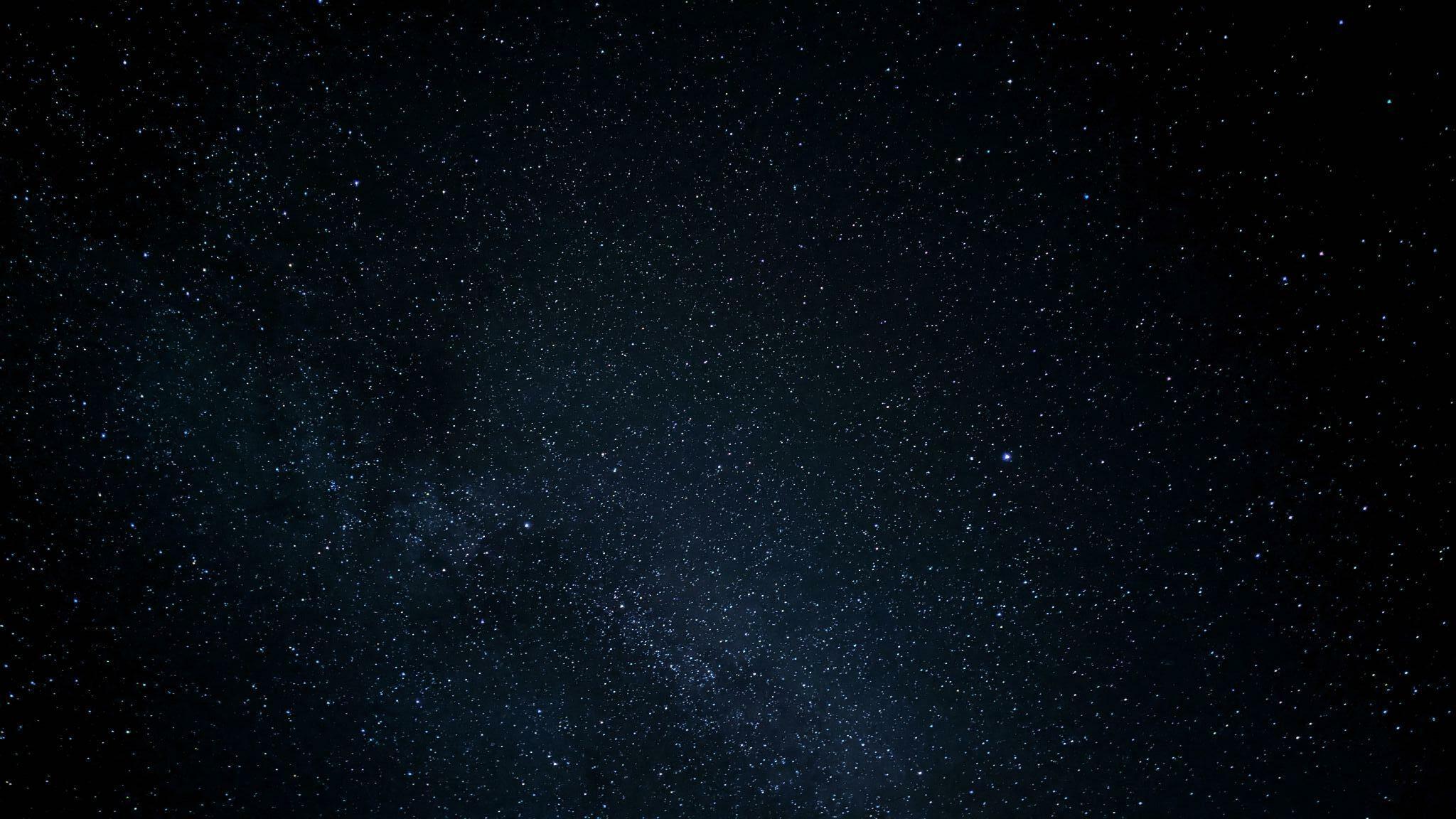
FOREST PAVILION
03Date:2021-2022
Location: Karlshamn, Ronneby
Project Type: commissioned by Region Blekinge, & Kuturrådet
Tools: Rhino 3D, ABB Robot
The project was commissioned to Jumana Hamdani Architects by Region Blekinge/ Konst i Blekinge and Kuturrådet to collaborate with Sculptur AB fabrication firm in Karlshamn using ABB Robot that reaches up to 3m height to 3D print with sustainable materials.
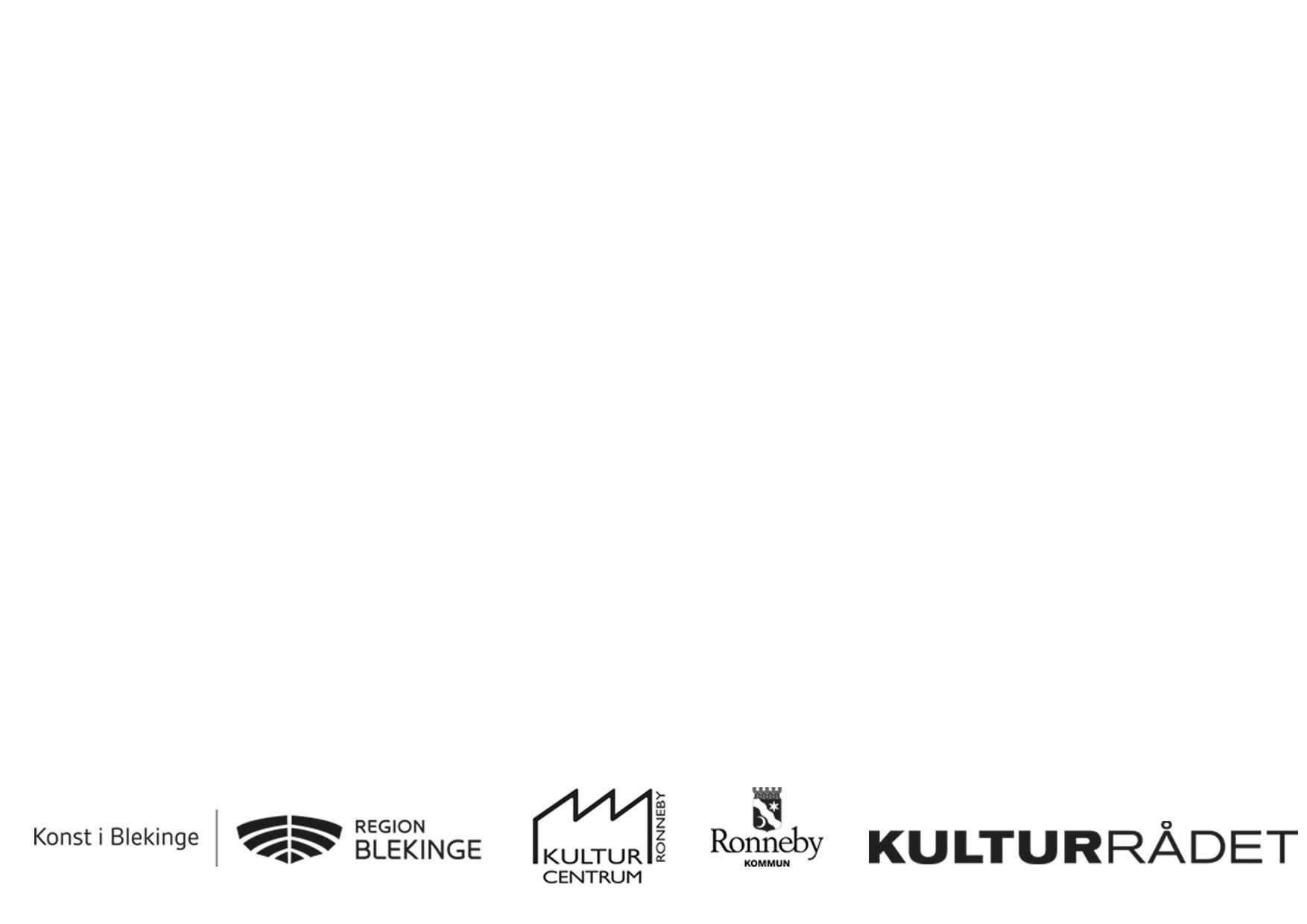
The Forest Pavilion is an experimental public art project testing 3D printing with recycled material made of wood fibers combined with bioplastic PLA. The project aims to rewild cities by creating a new urban structure that has similarities to the surrounding landscape of Blekinge, resembling trees.
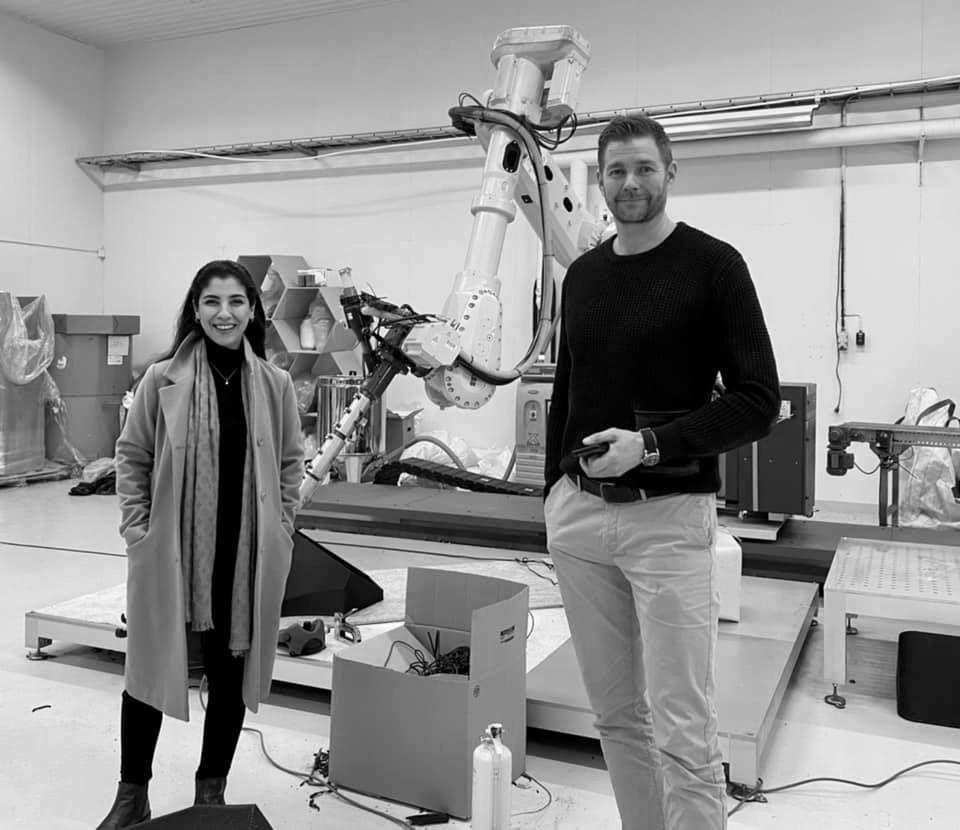
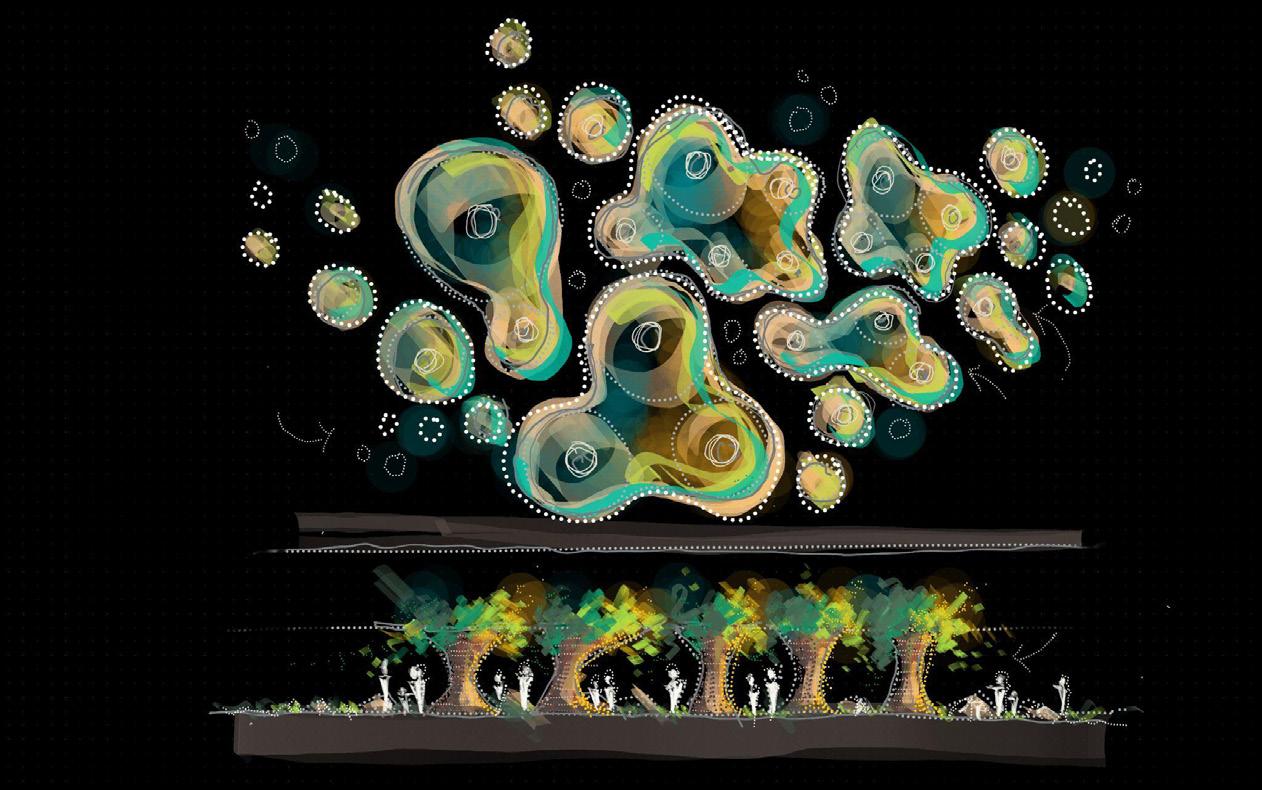
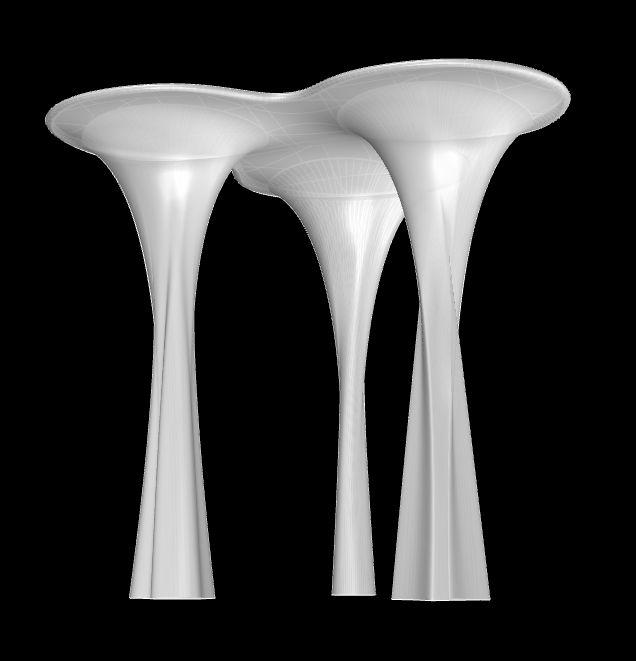
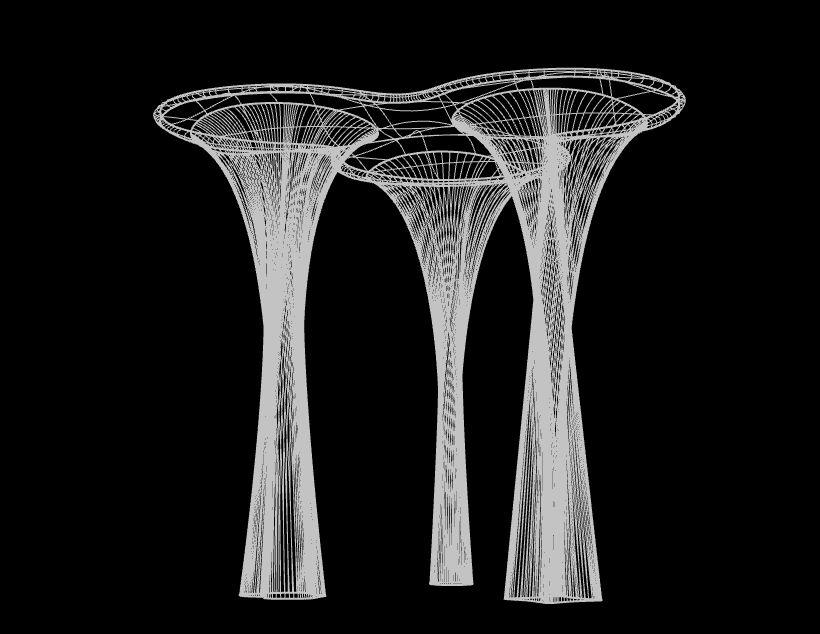
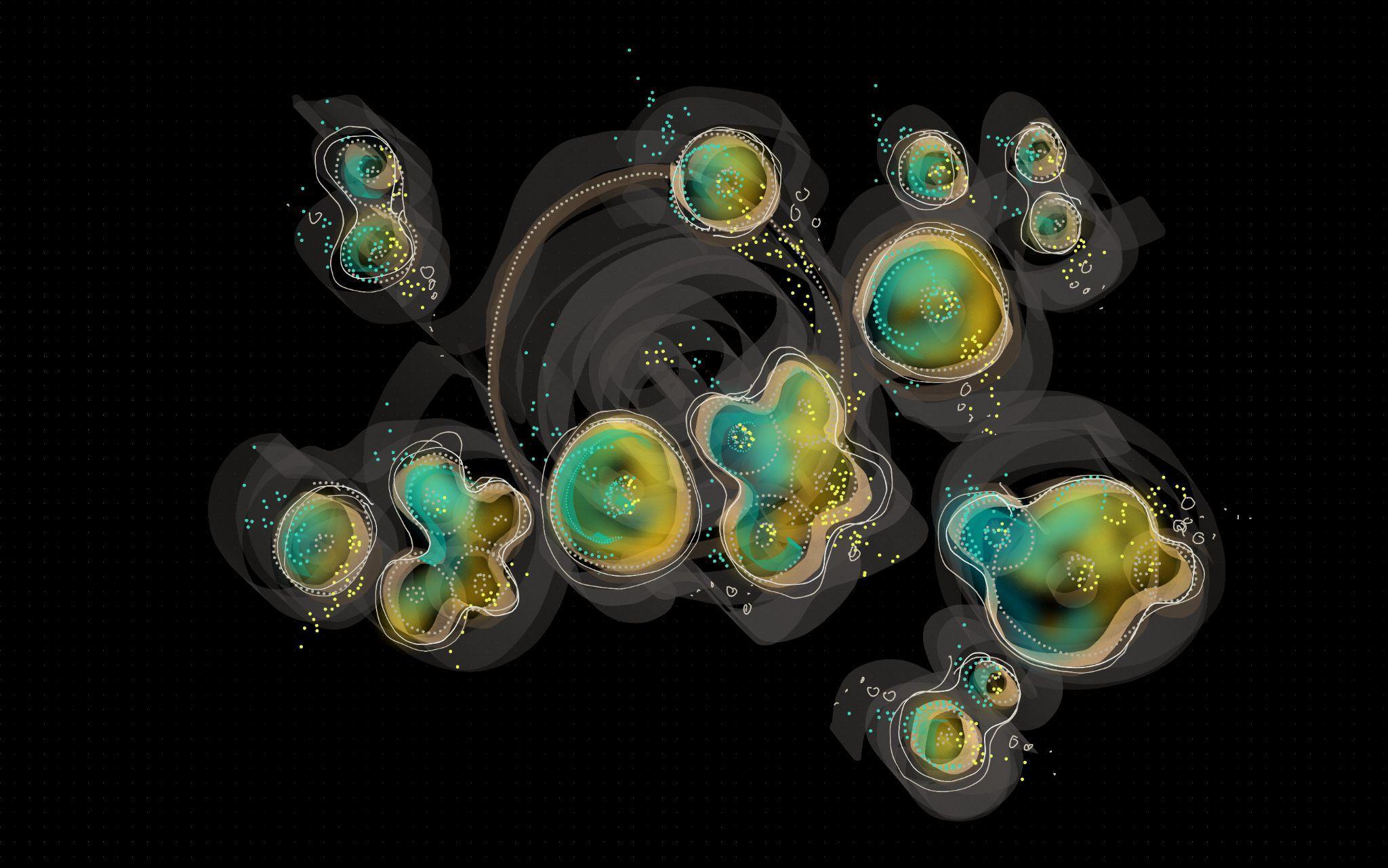
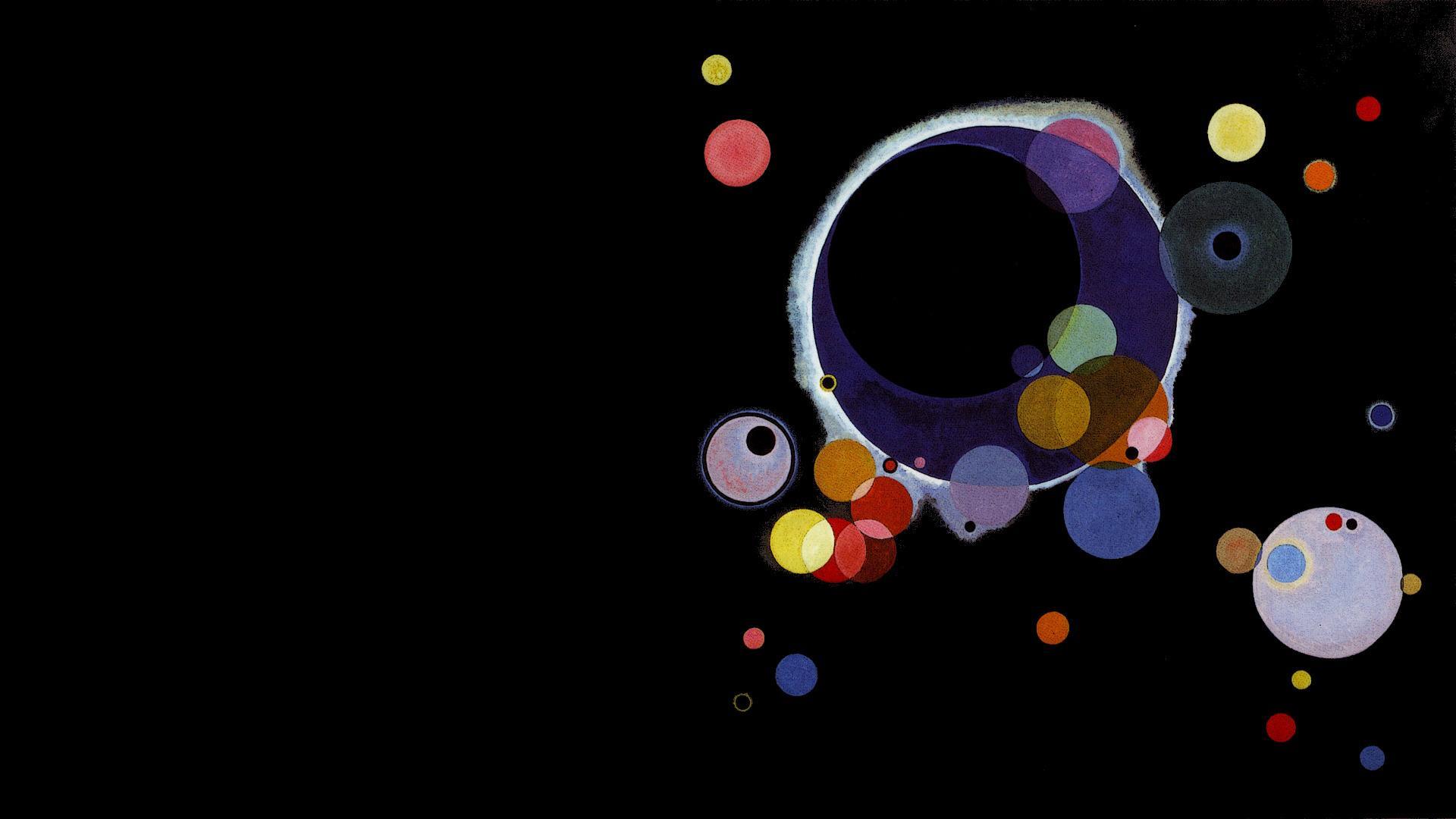
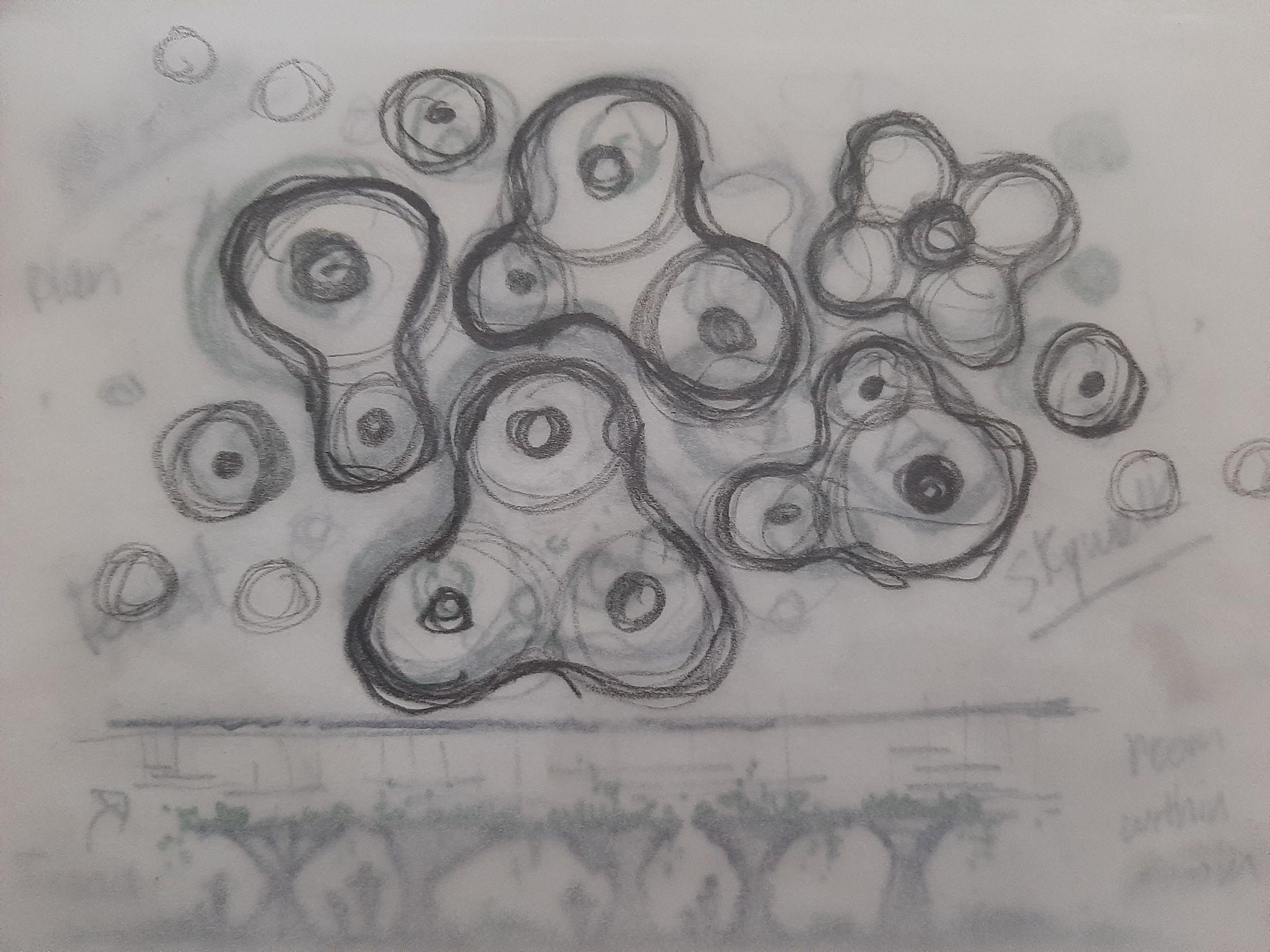
INITIAL CONCEPT
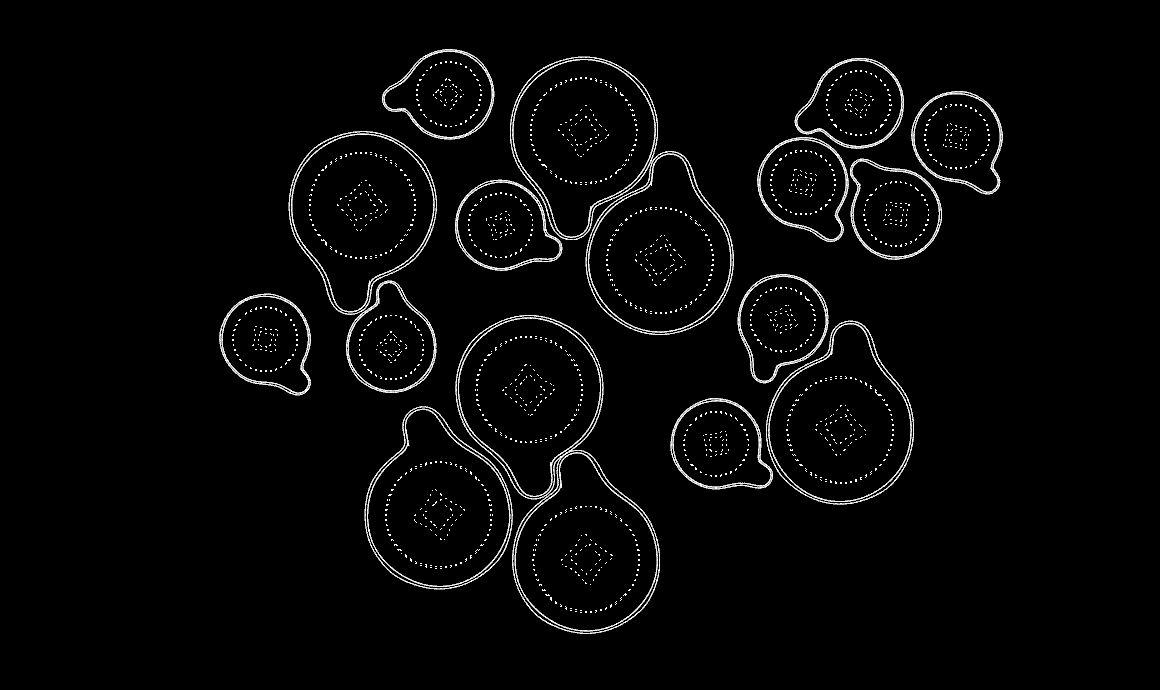
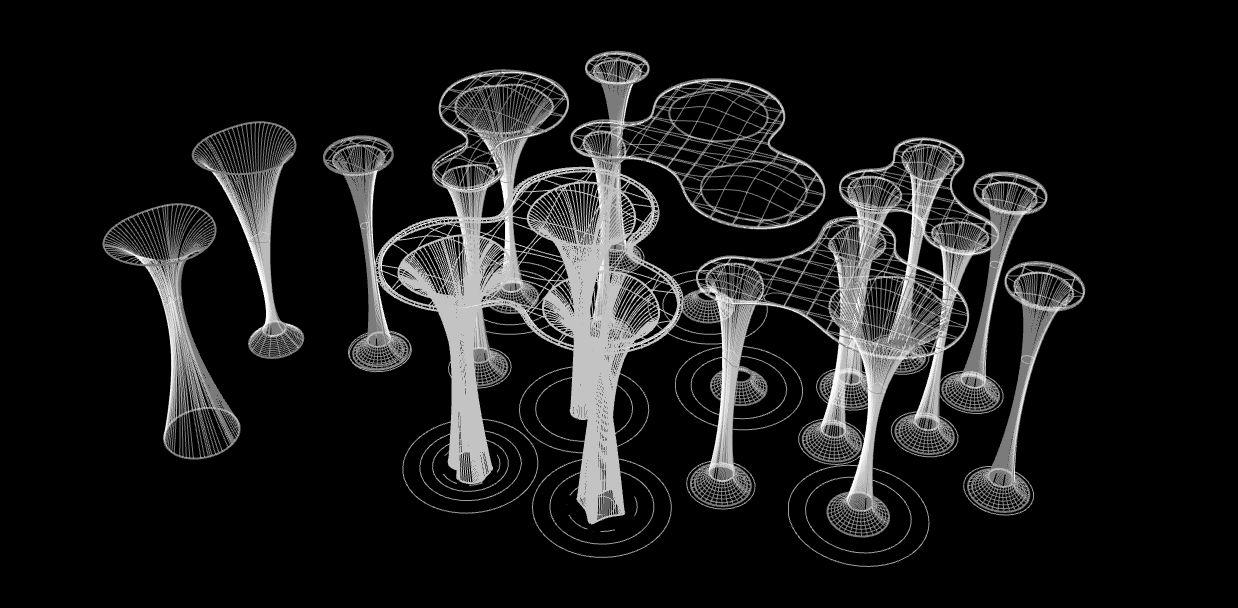
Eco-systems/Rewilding Forest Pavilion
Growing cities and the need to build more at a low cost, and the limited resources on our planet, are all factors pushing us to find more solutions towards sustainable ways of living, building, and reusing the existing constructions.
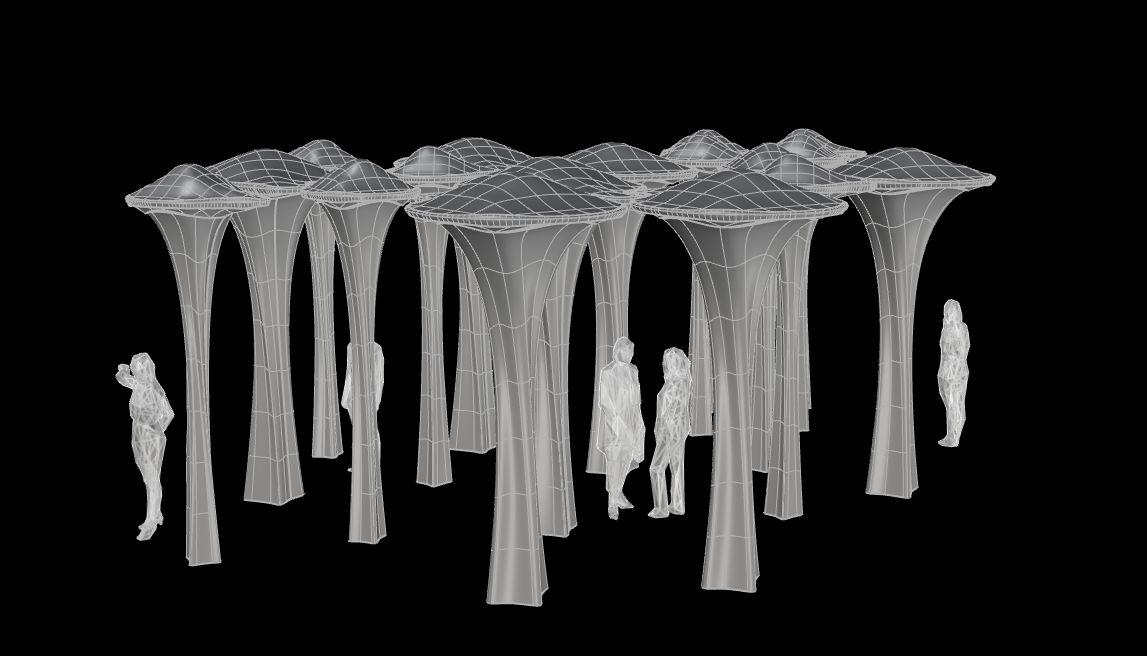
FOREST PAVILION
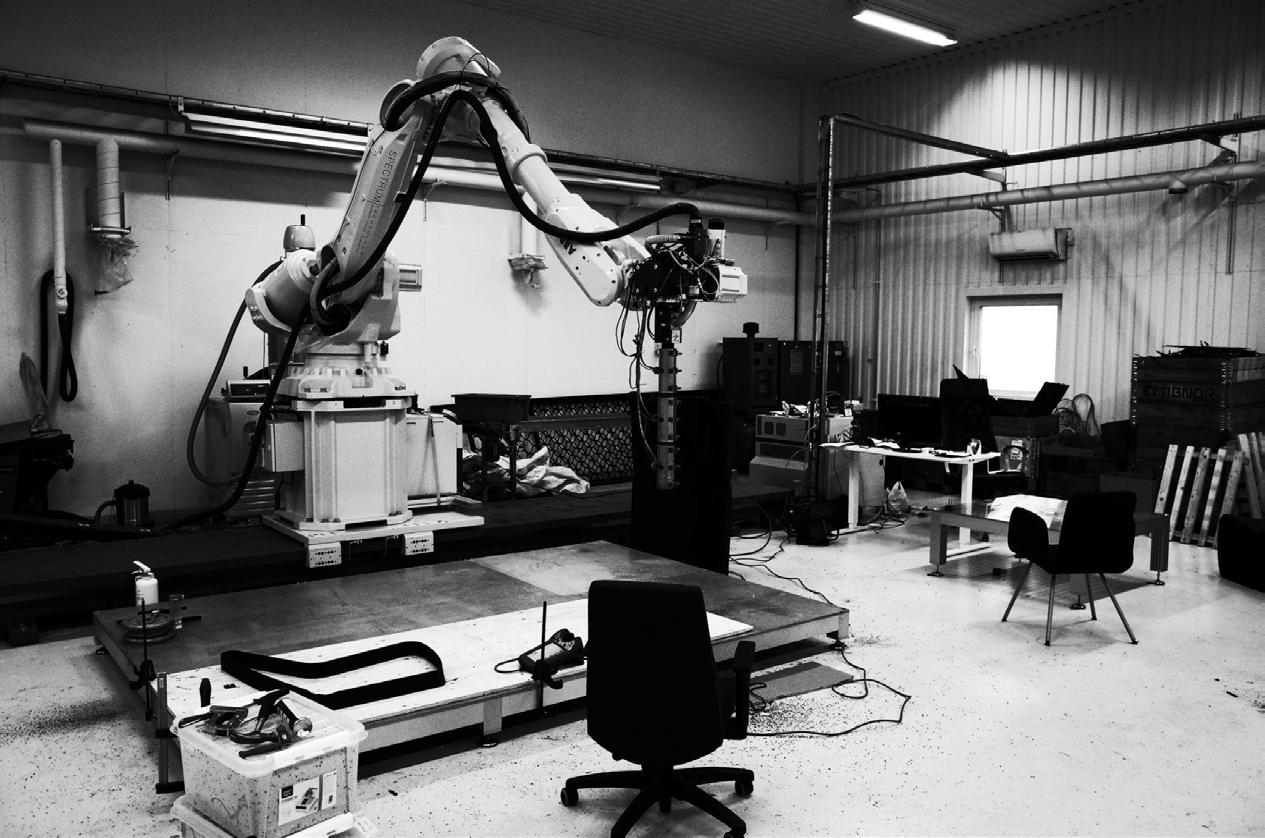
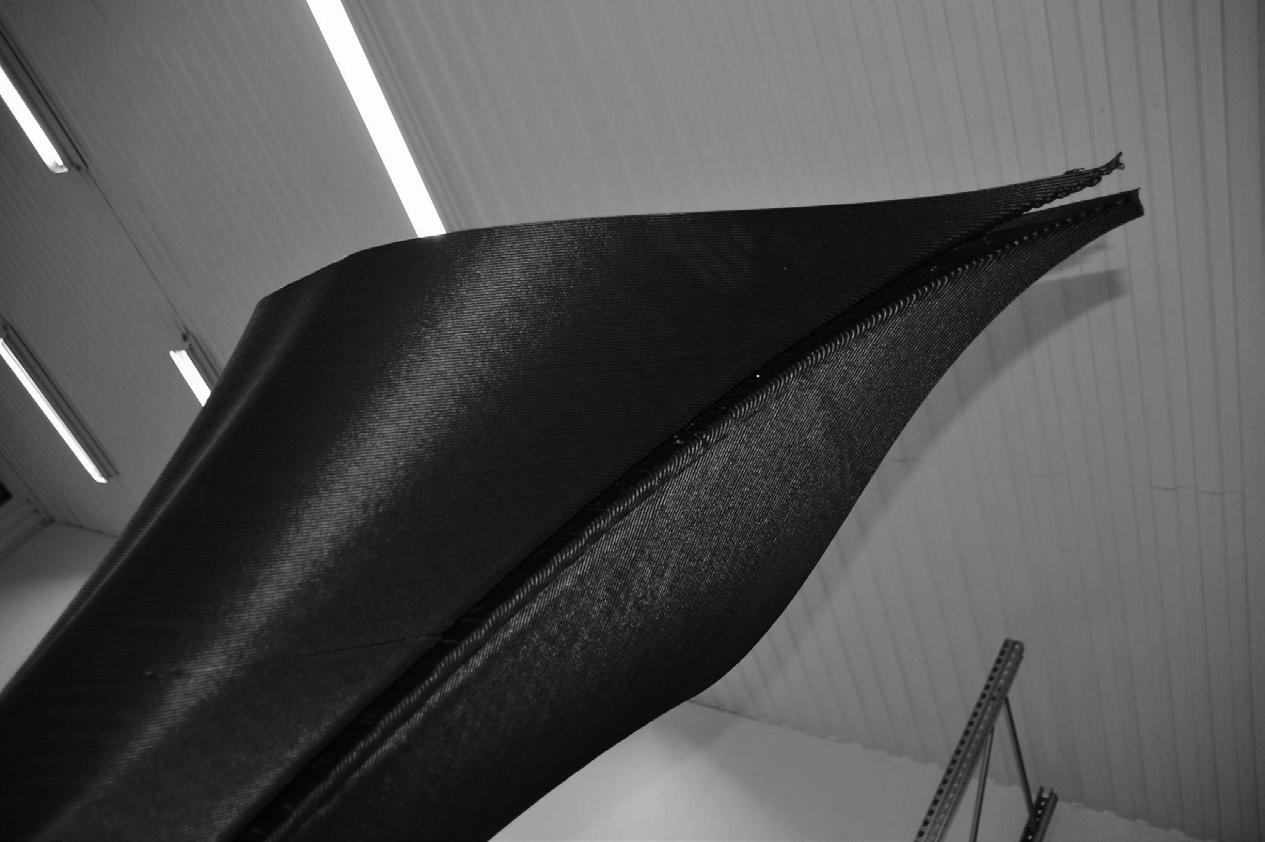
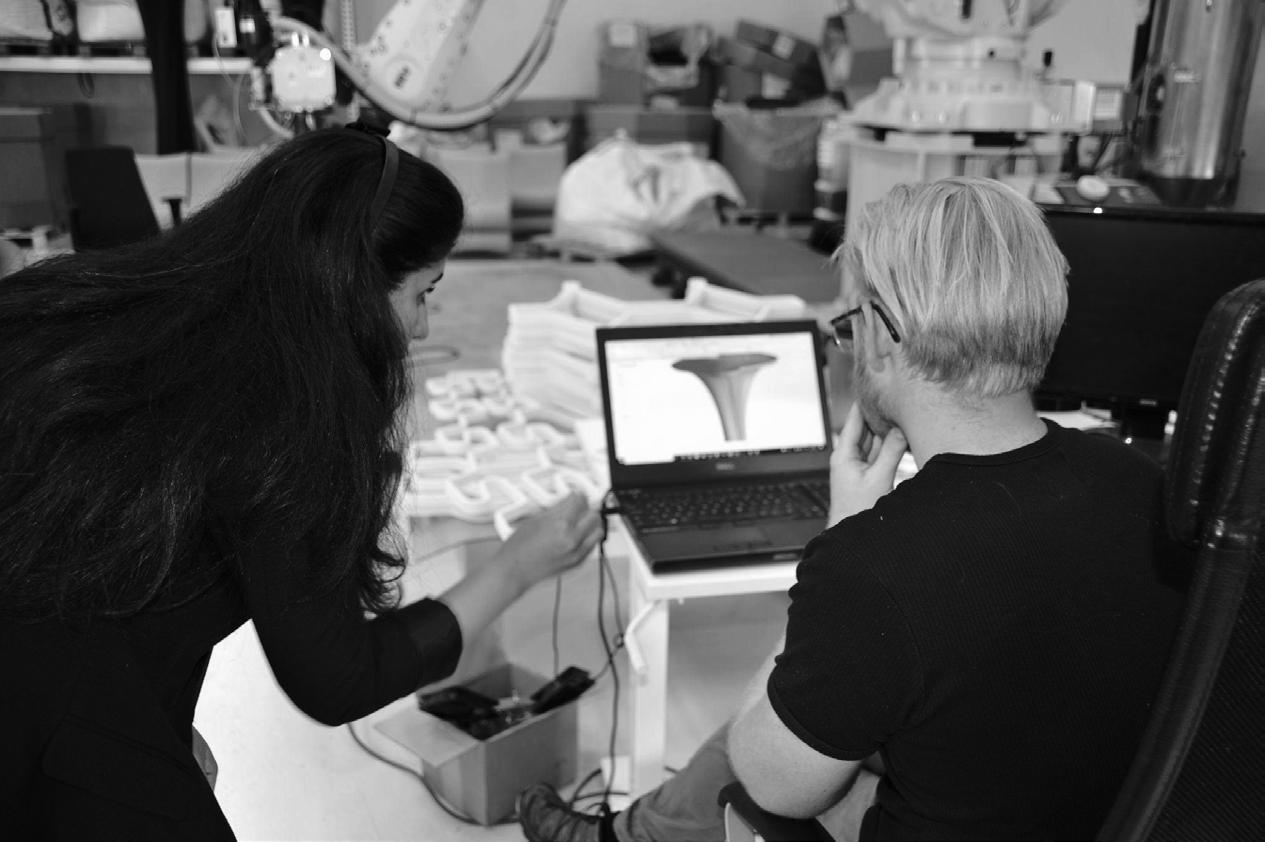
DESIGN IN PROCESS
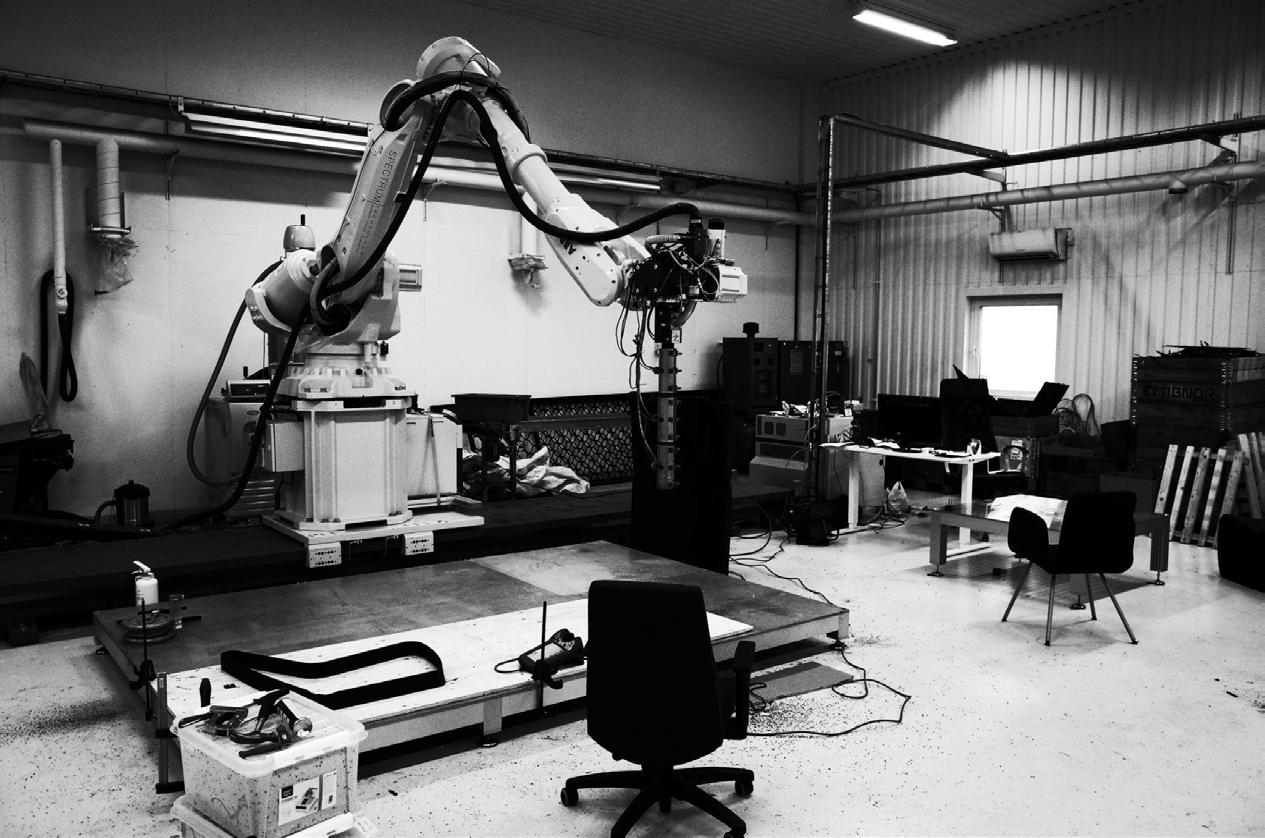
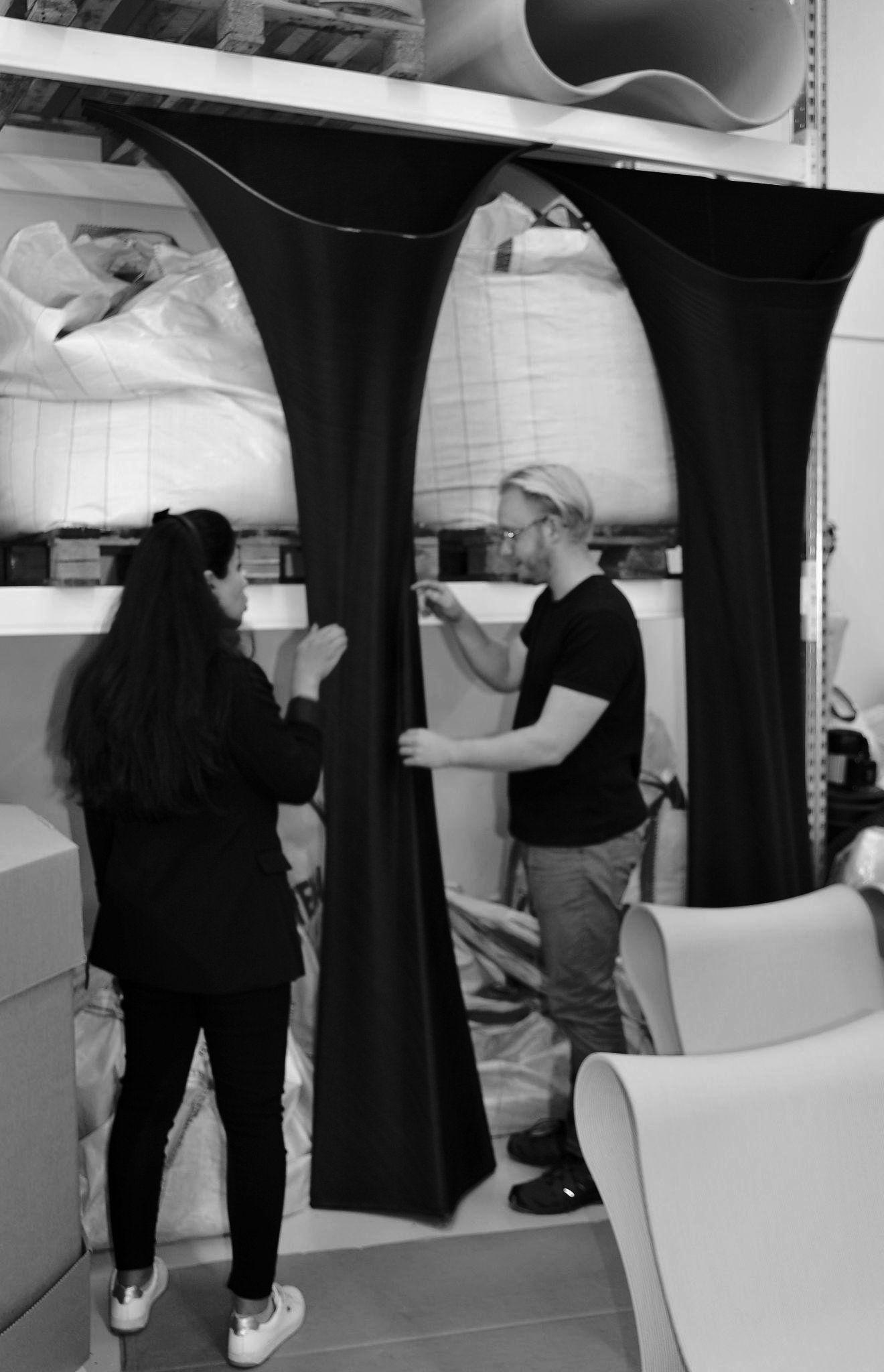
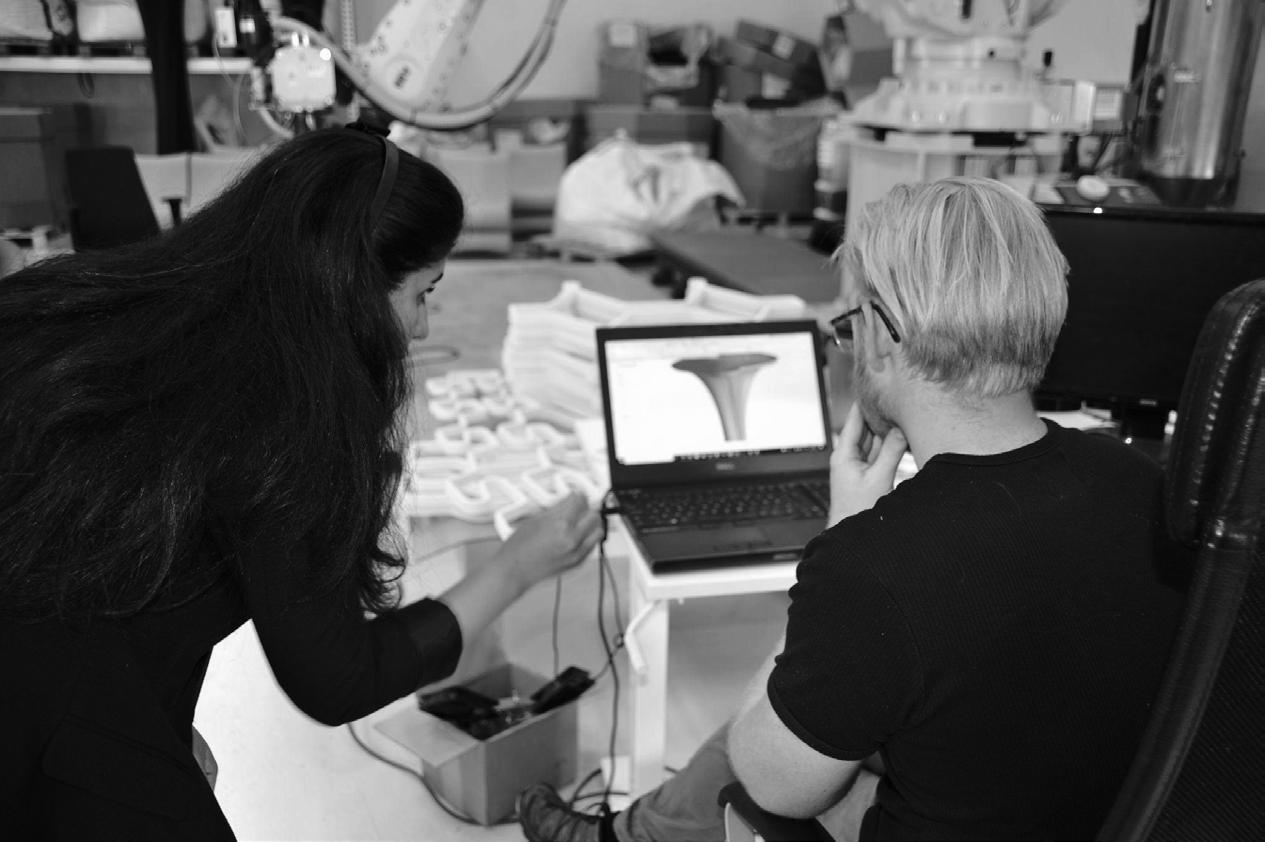
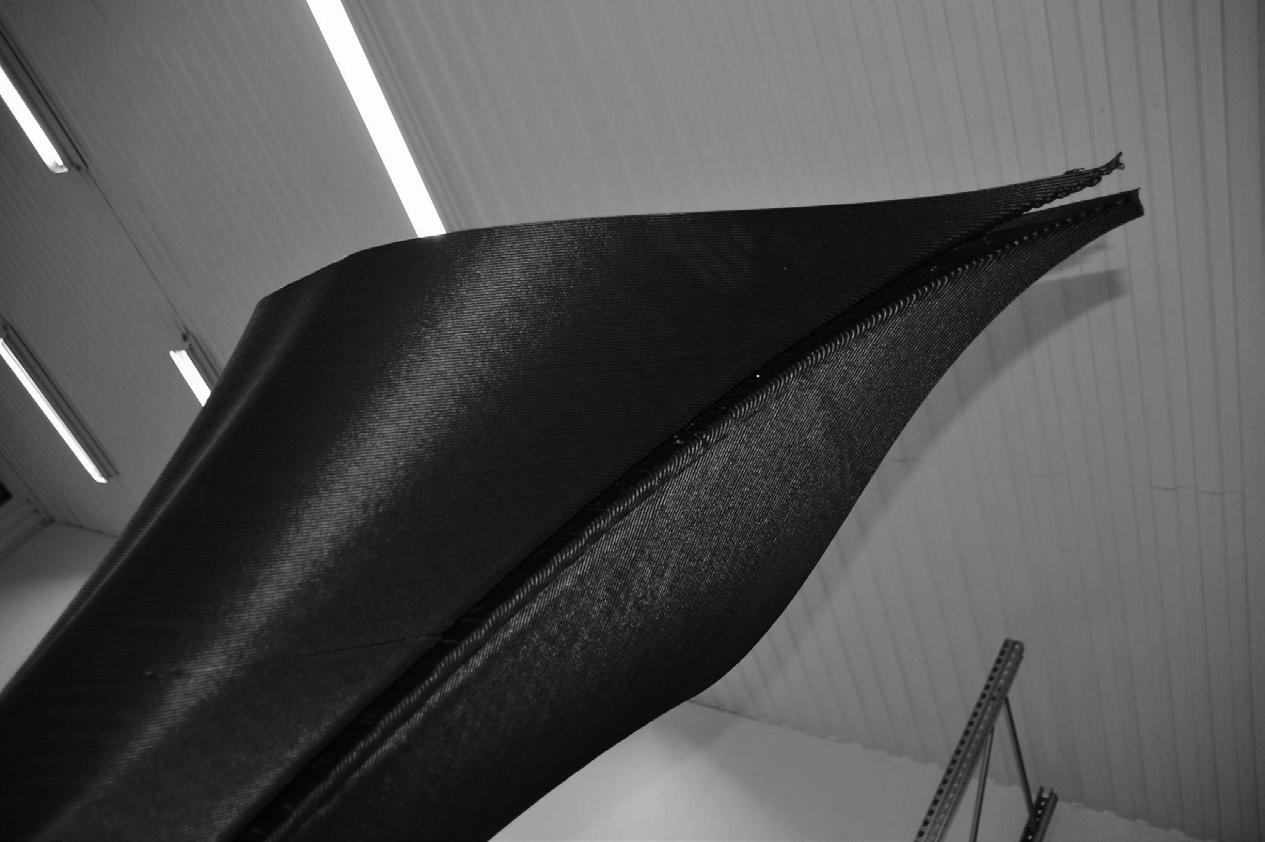
FOREST PAVILION
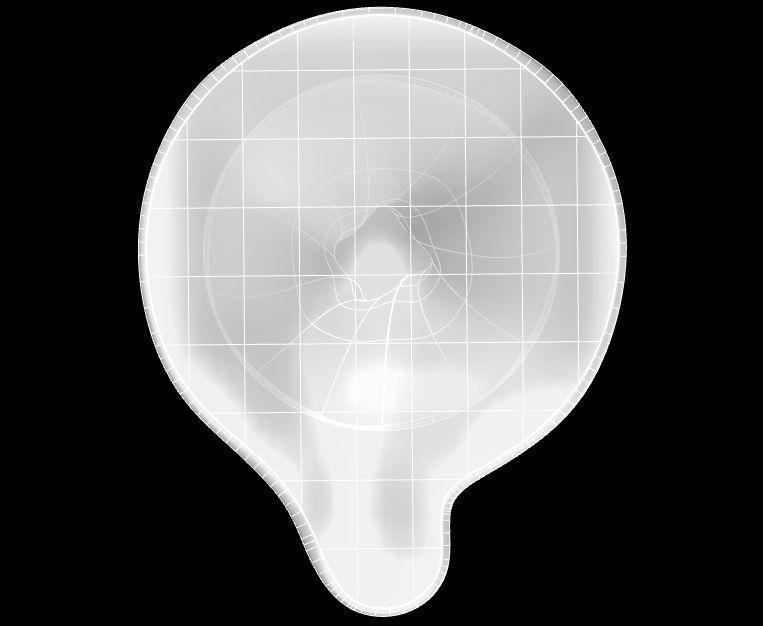

FABRICATION
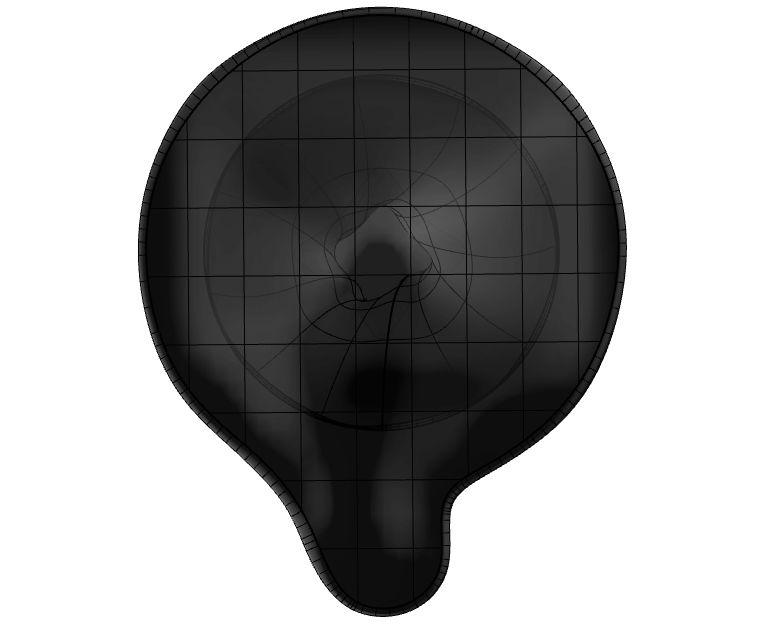
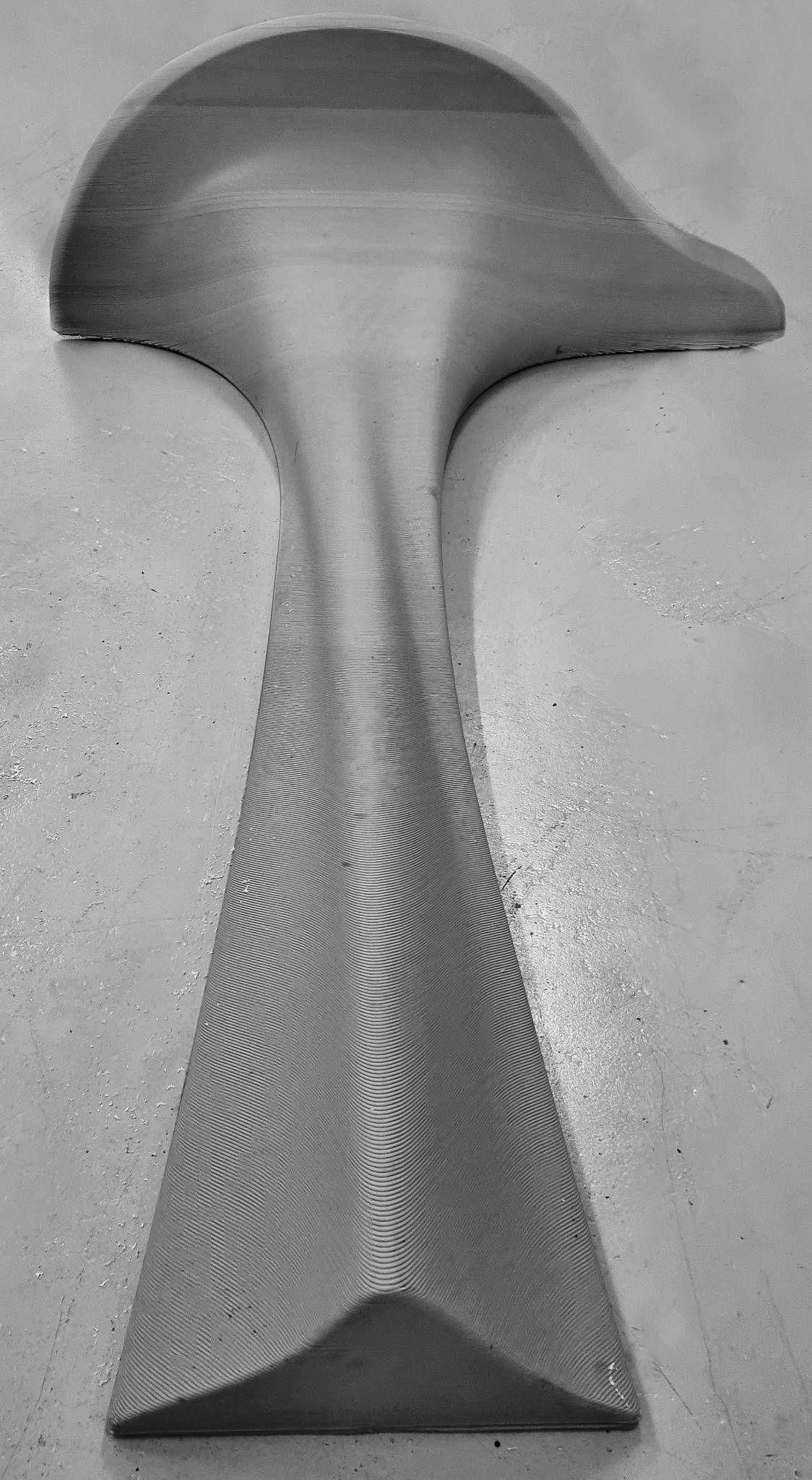
WOOD FIBRE
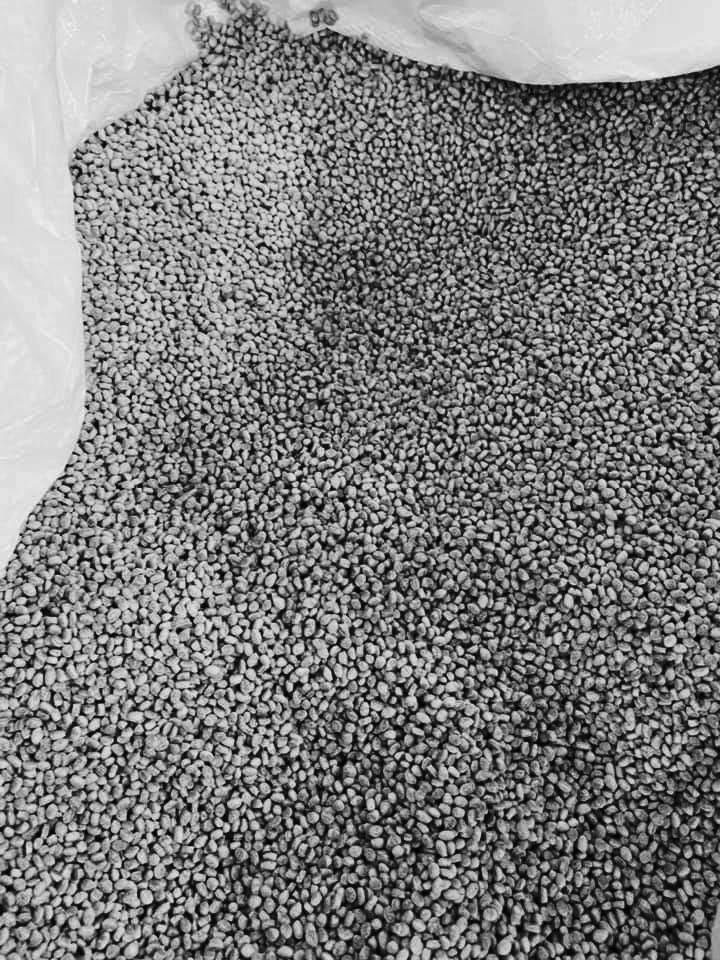
FOREST PAVILION
3D PRINTING MODEL
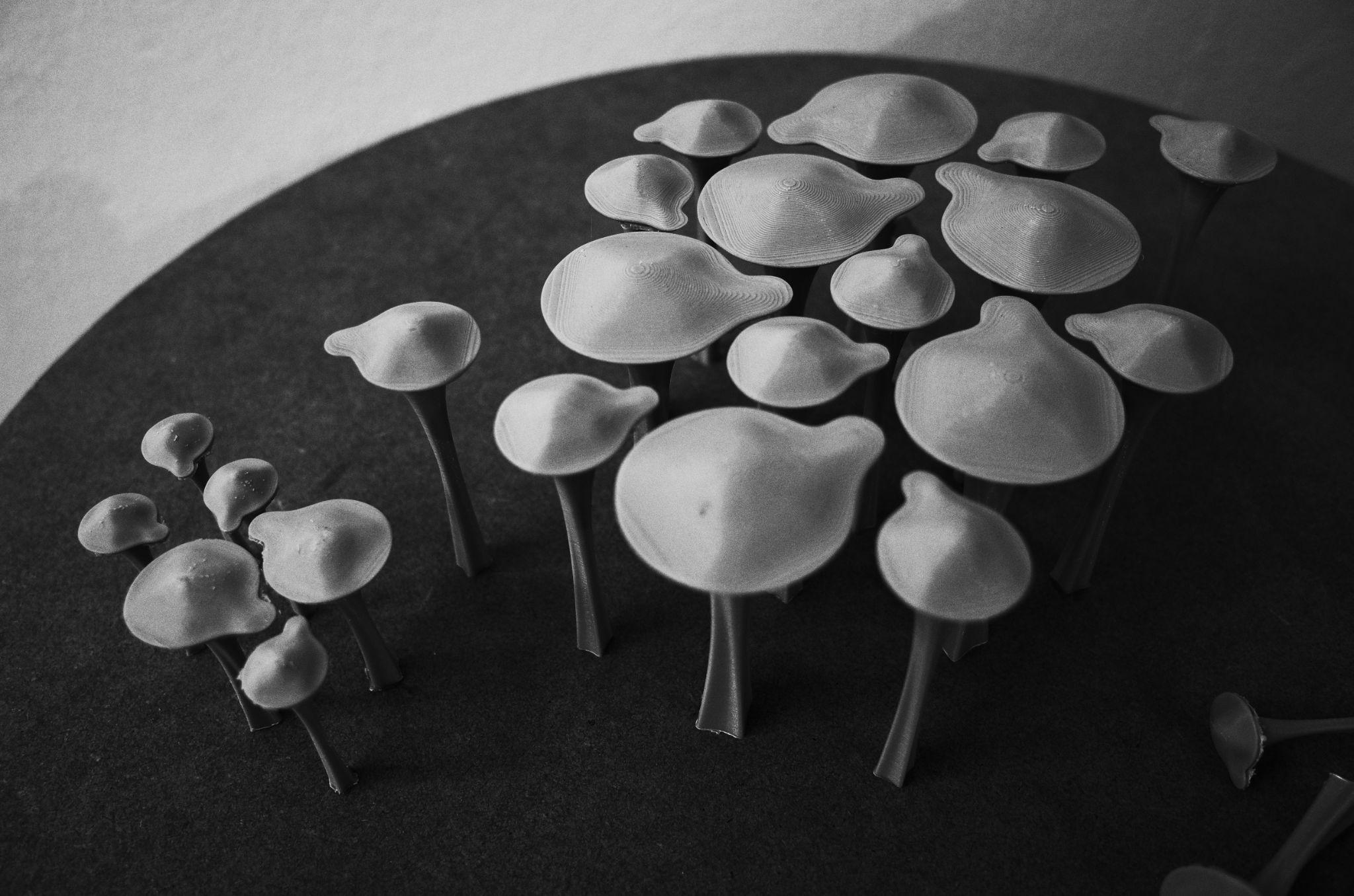
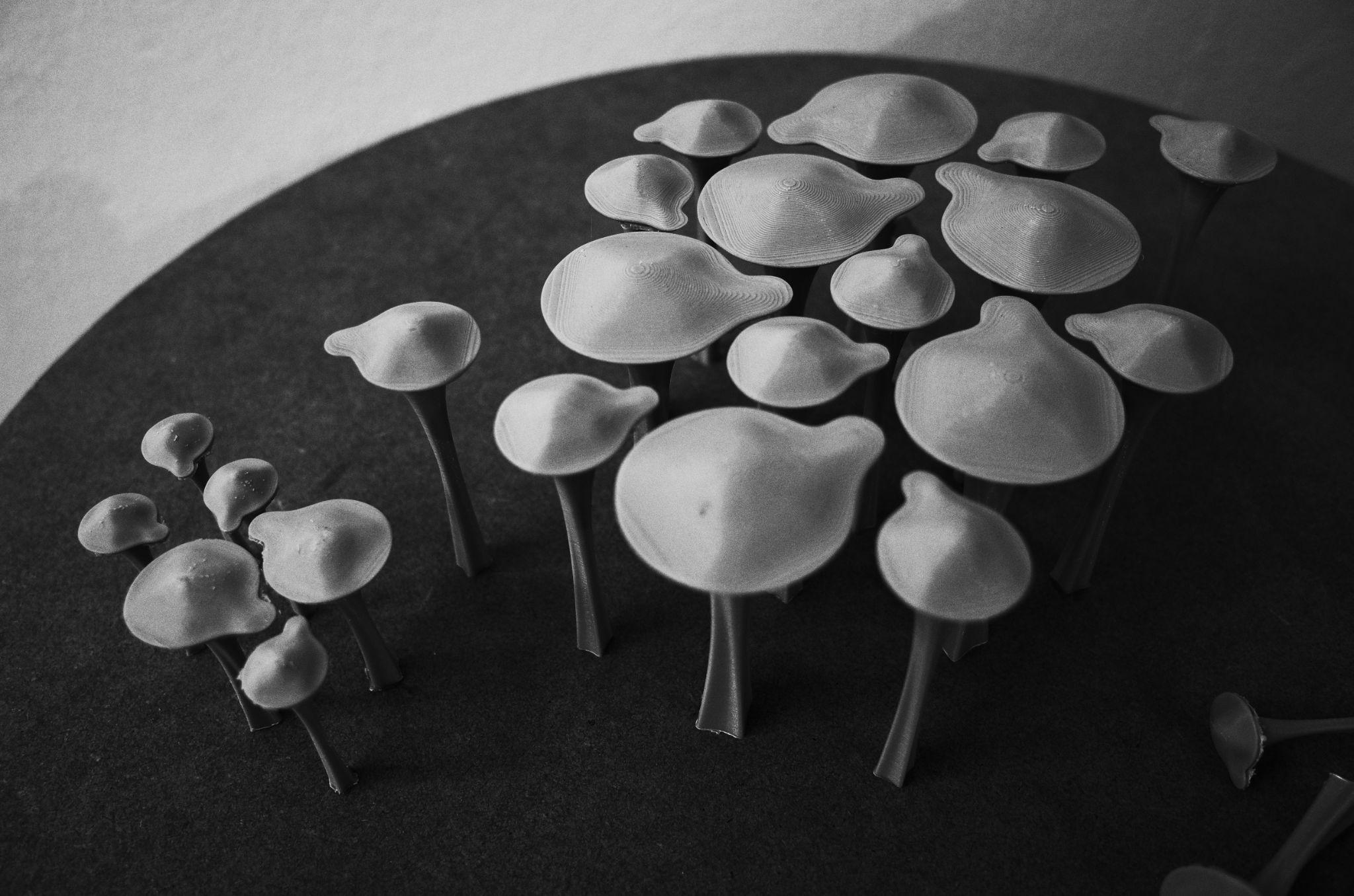
FOREST PAVILION
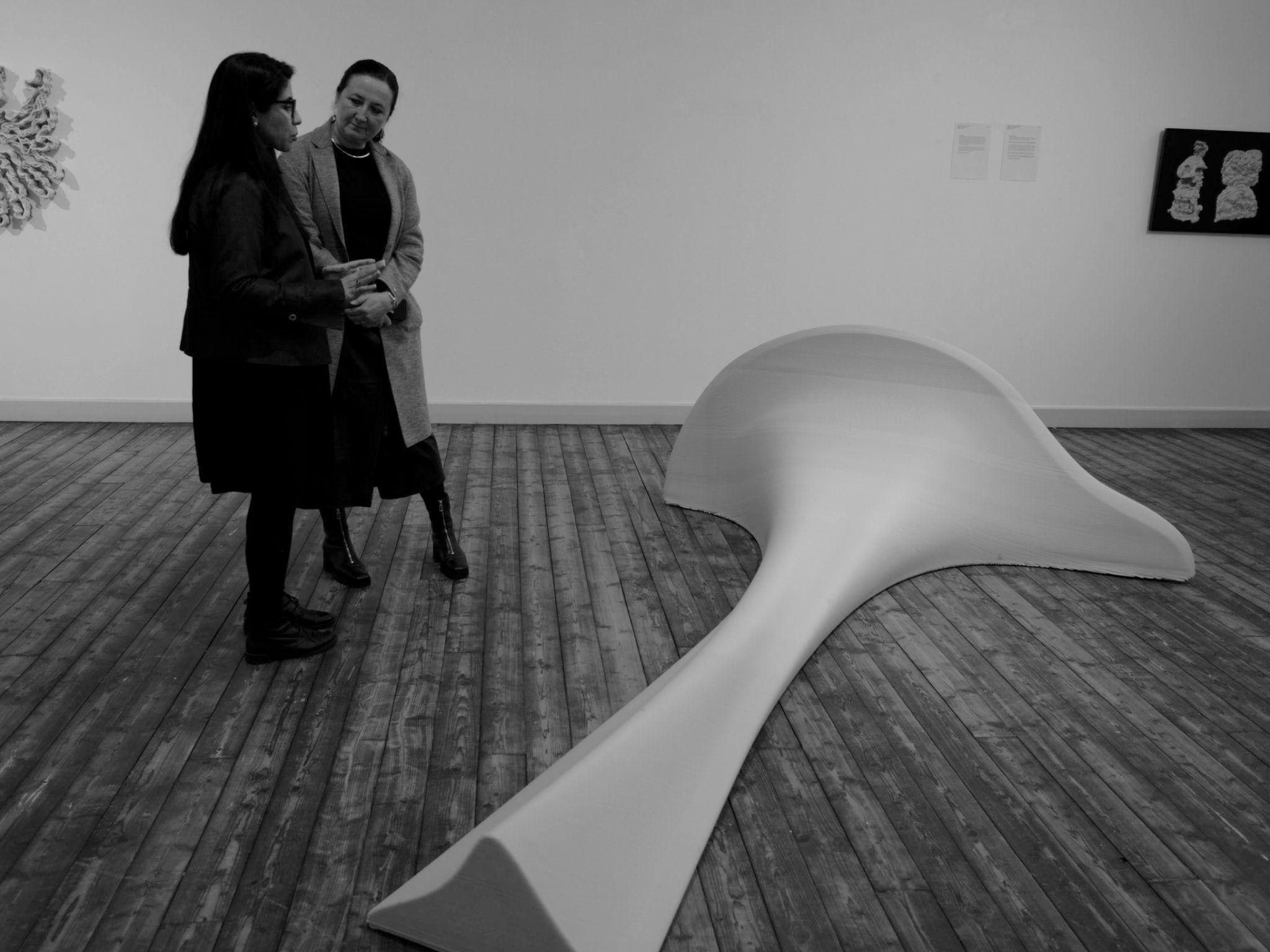
EXHIBITION
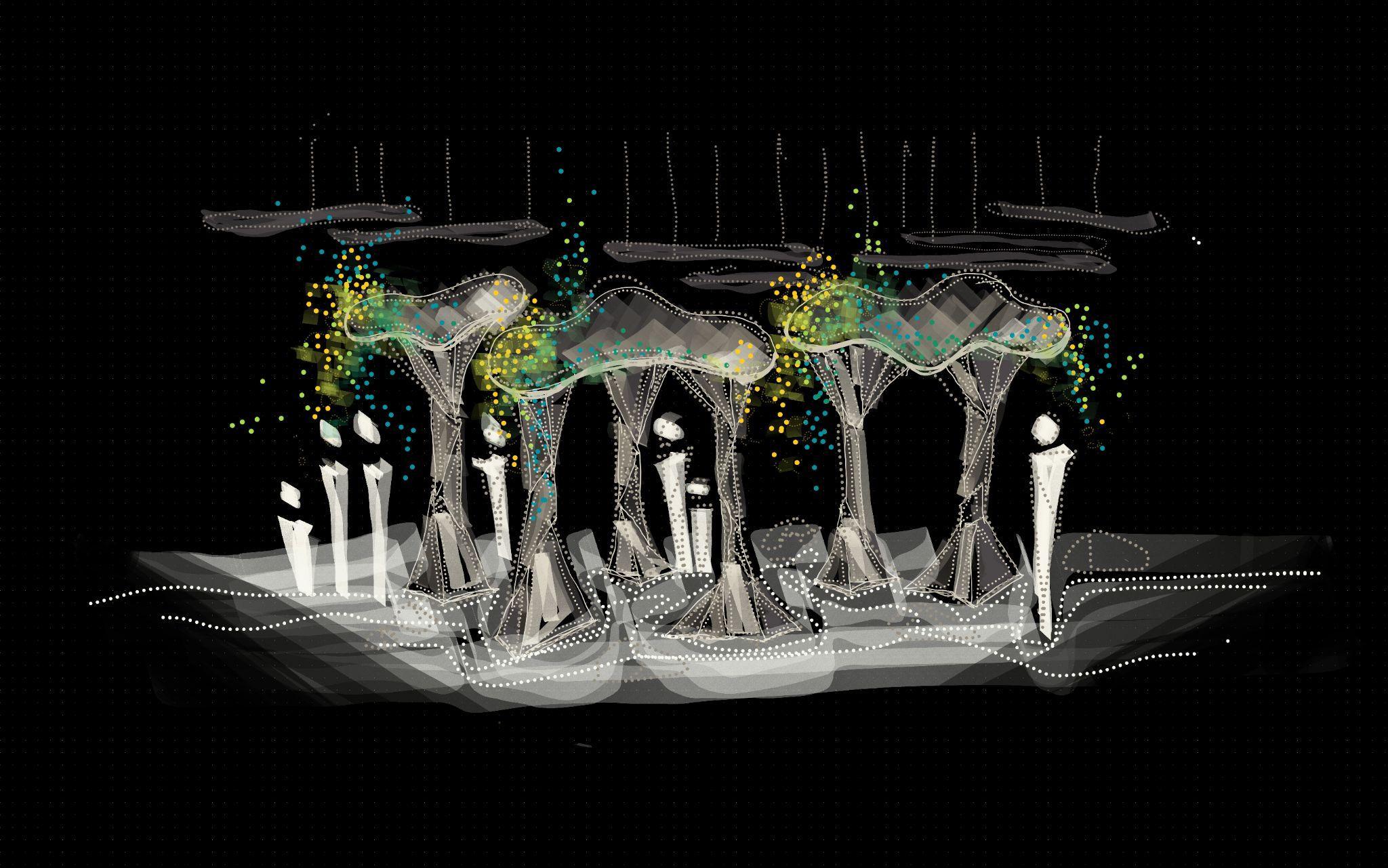
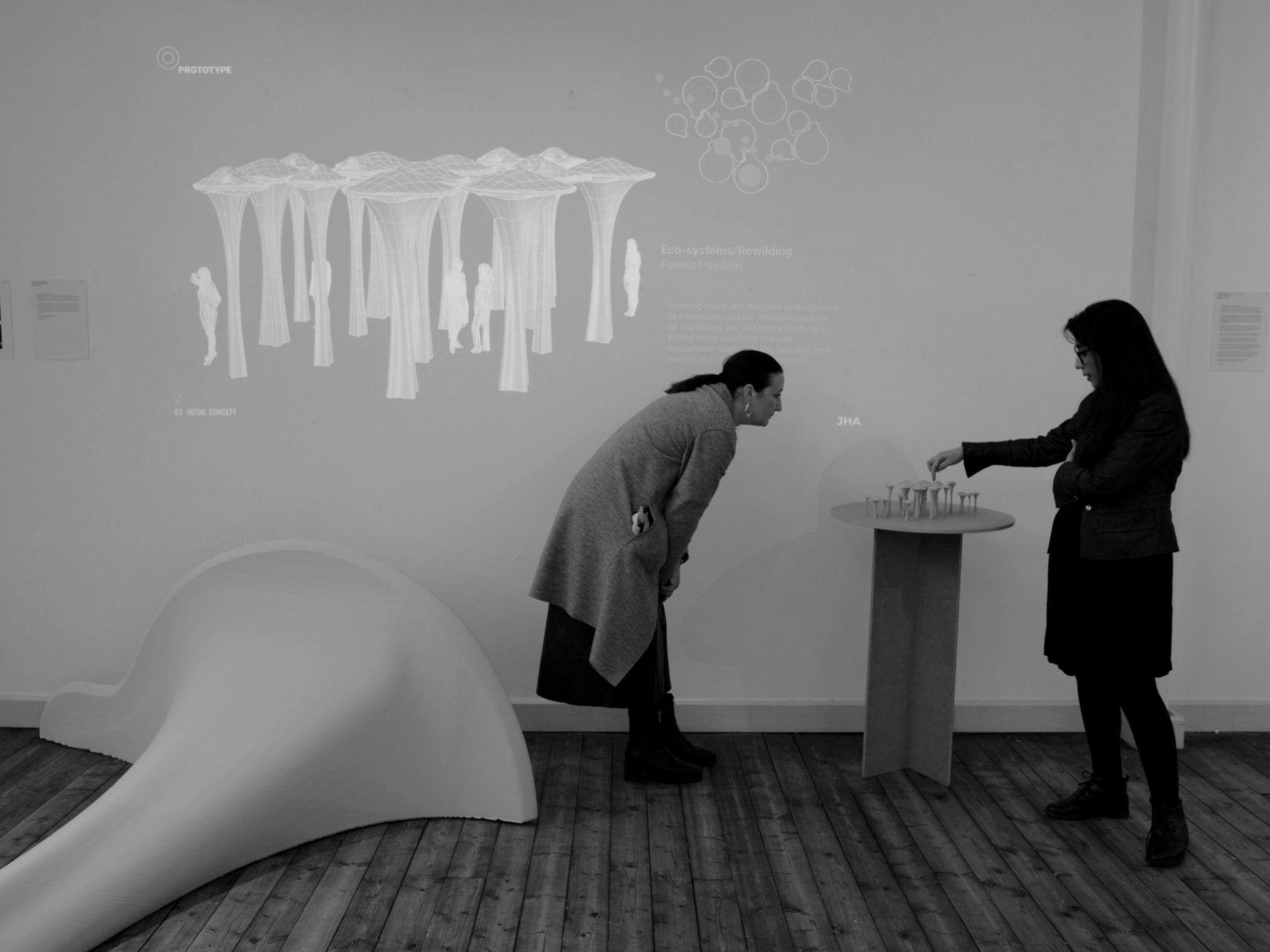
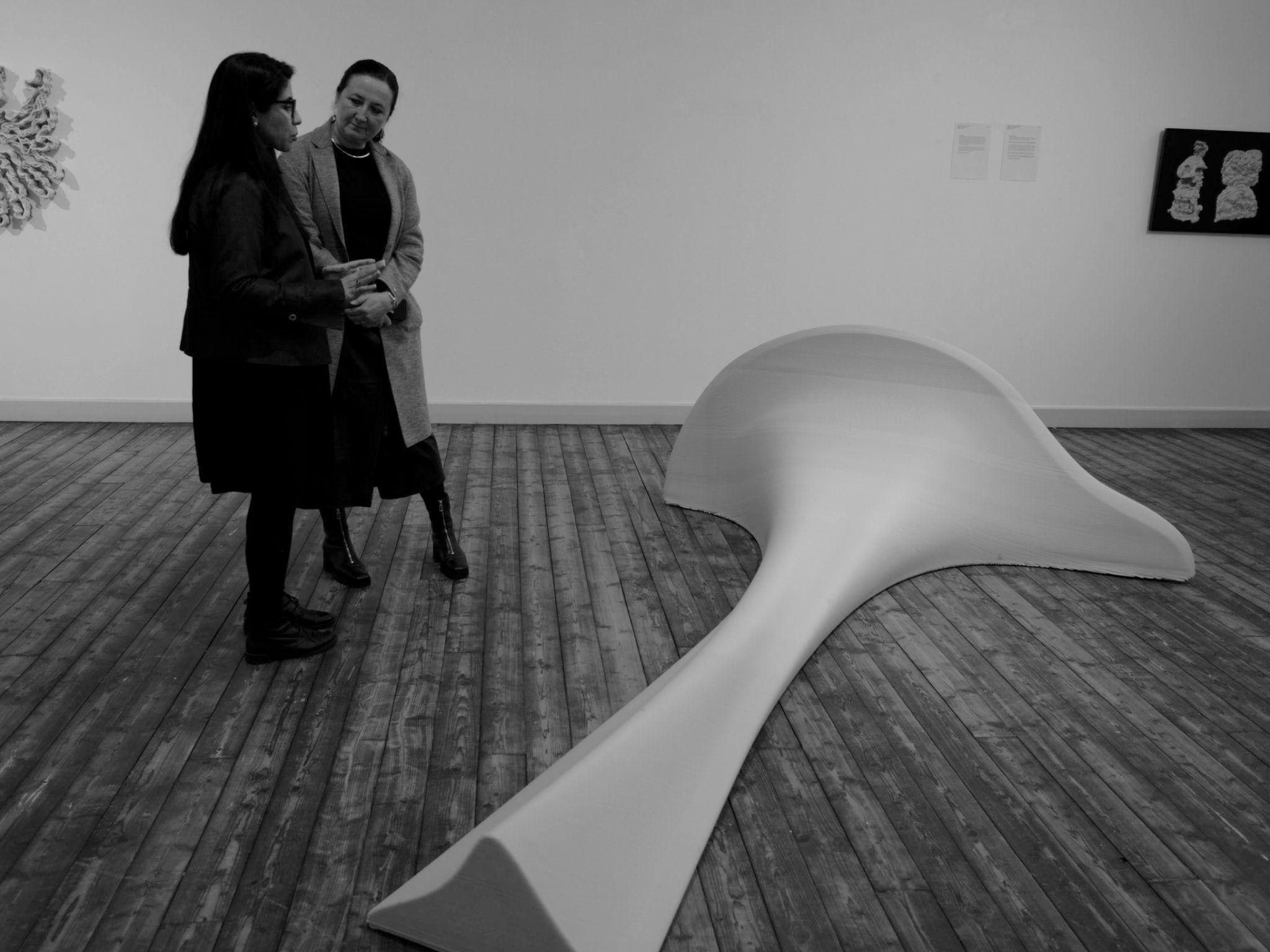
IAAC SCHOLARSHIP

04 Date: 2021 Location: Barcelona, Spain Proposal Type: Won MaCAD Competition Scholarship at IAAC Tools: Rhino, Grasshopper, 3D Max, Photoshop
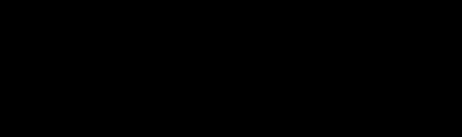
Non-Anthropocentric Structures: Creating Spaces for Wildlife using 3D Printed Waste
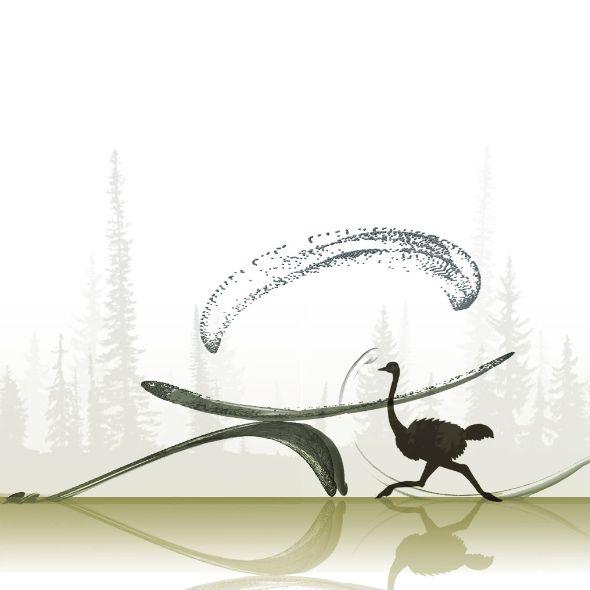
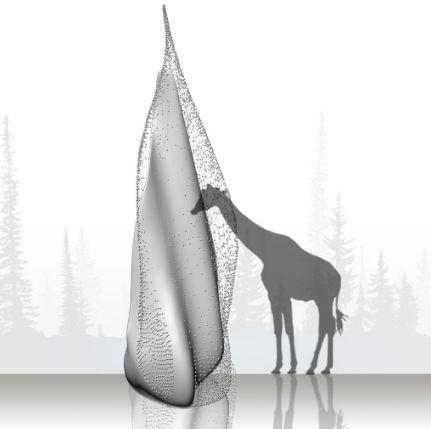
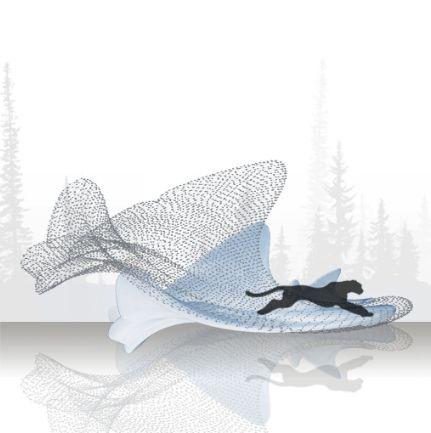
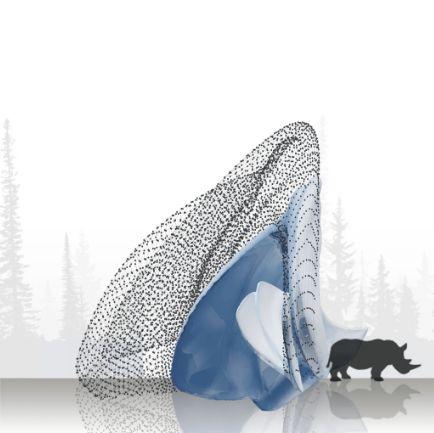
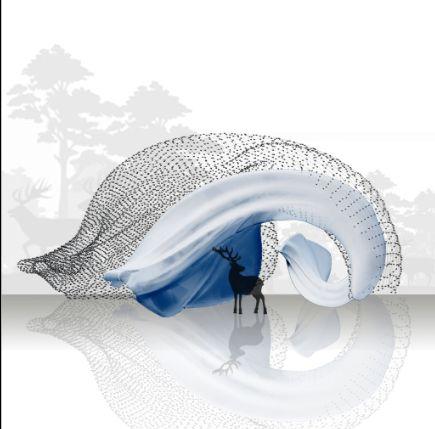
Shifting the design thinking towards non-anthropocentrism can save so many species endangered with their surrounding environments. Using 3D printing as an indispensable smart construction technology can help us for example recyclinging the waste in the oceans and turning it into self-sustainable structures that can provide habitats for wildlife on land or above and below water. Also,The use of 3D printing features impact on notable labour, energy saving and material cost benefits, sustainability development support in the construction sector.
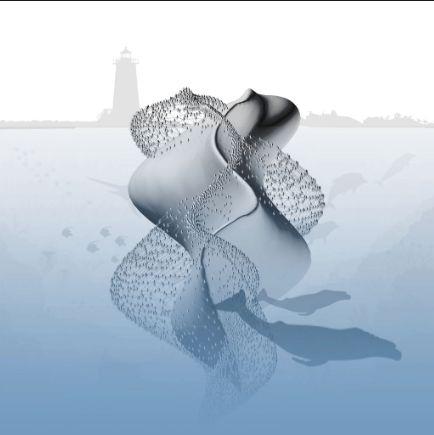
WALKING COURTYARDS
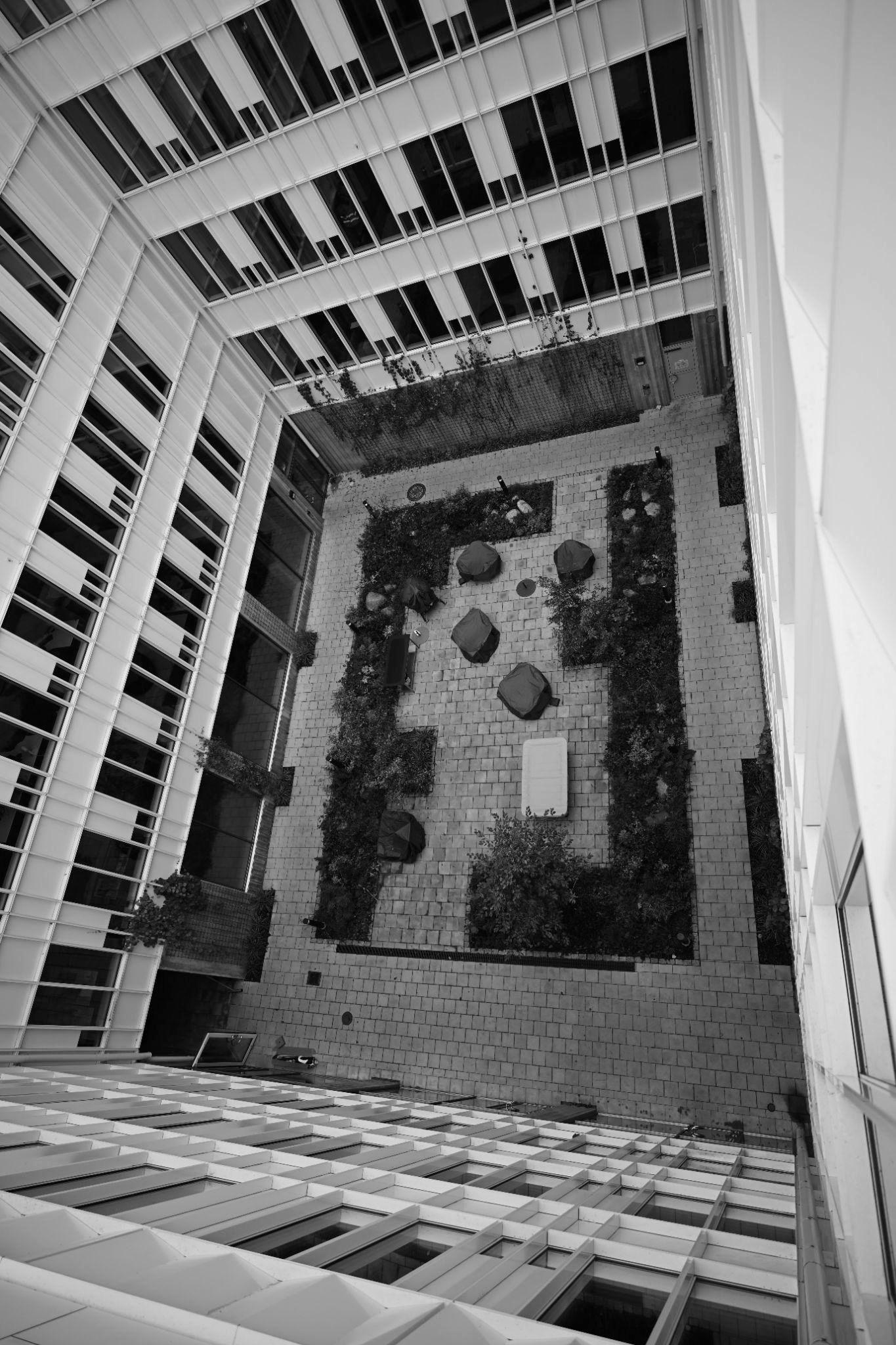
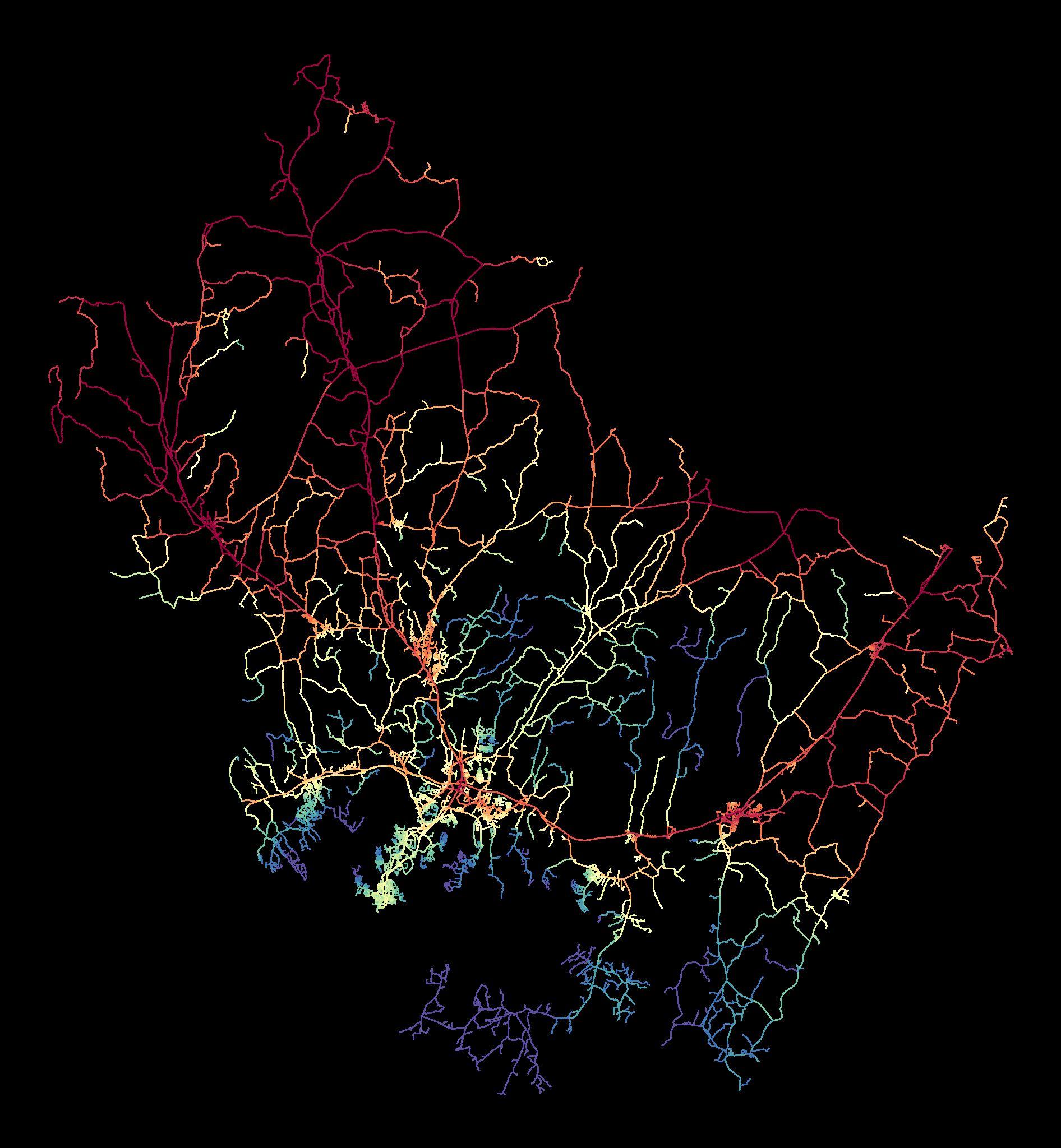
05 Date: 2022
Courtyards are traditional architectural features that go back to ancient times. They exist worldwide in different forms and for various social and environmental reasons. In this we will focus on two case studies, one in the north of Europe in Karlskrona, Sweden, and one in the south in Athens, Greece. The comparative research aspects would be aesthetics, social, and thermal comfort for both courtyards. The analysis of the courtyards would serve as a prelude to our walk in town through an itinerary of the courtyards on our way.
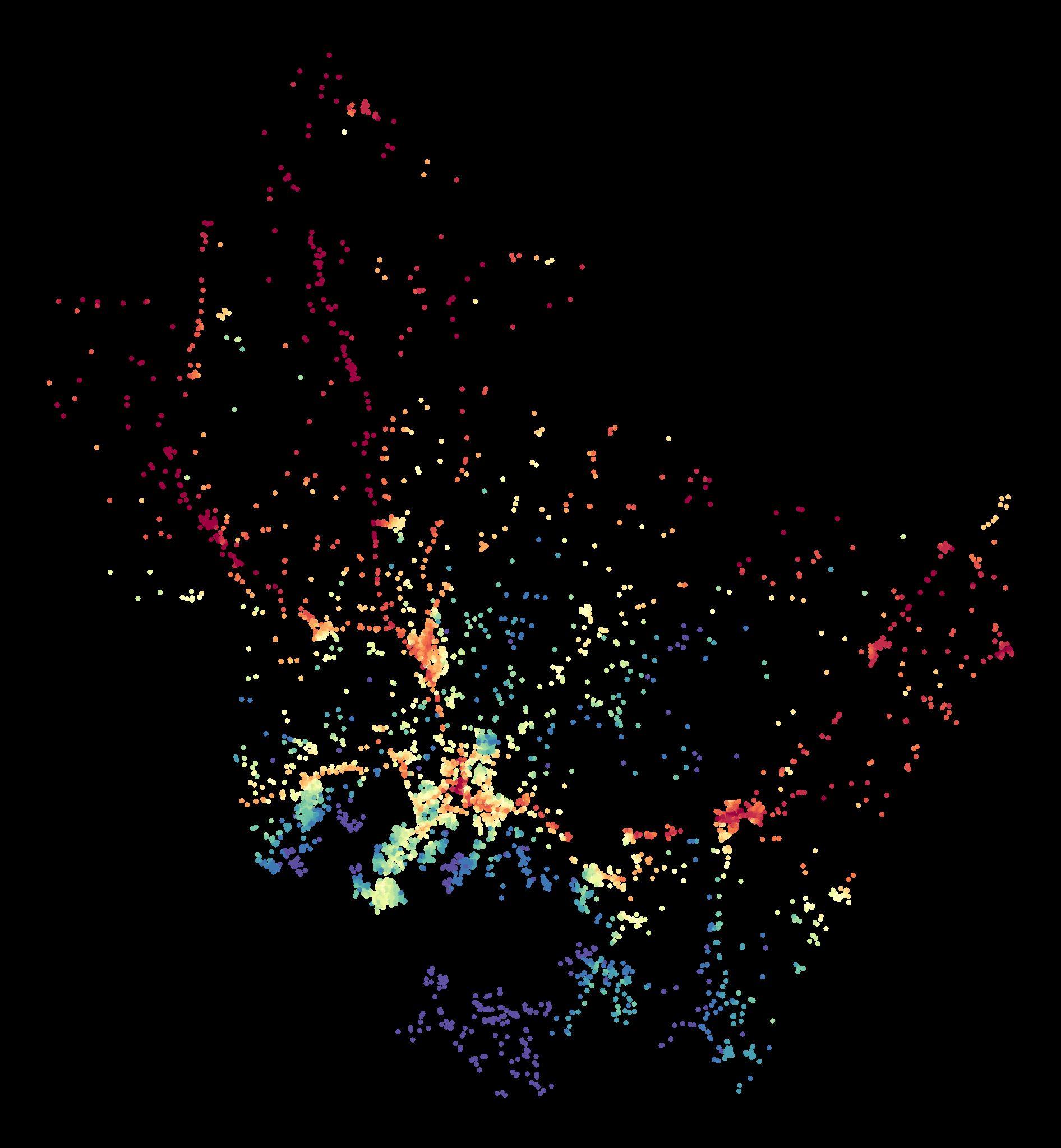
WORLD TRADE CENTER KARLSKRONA
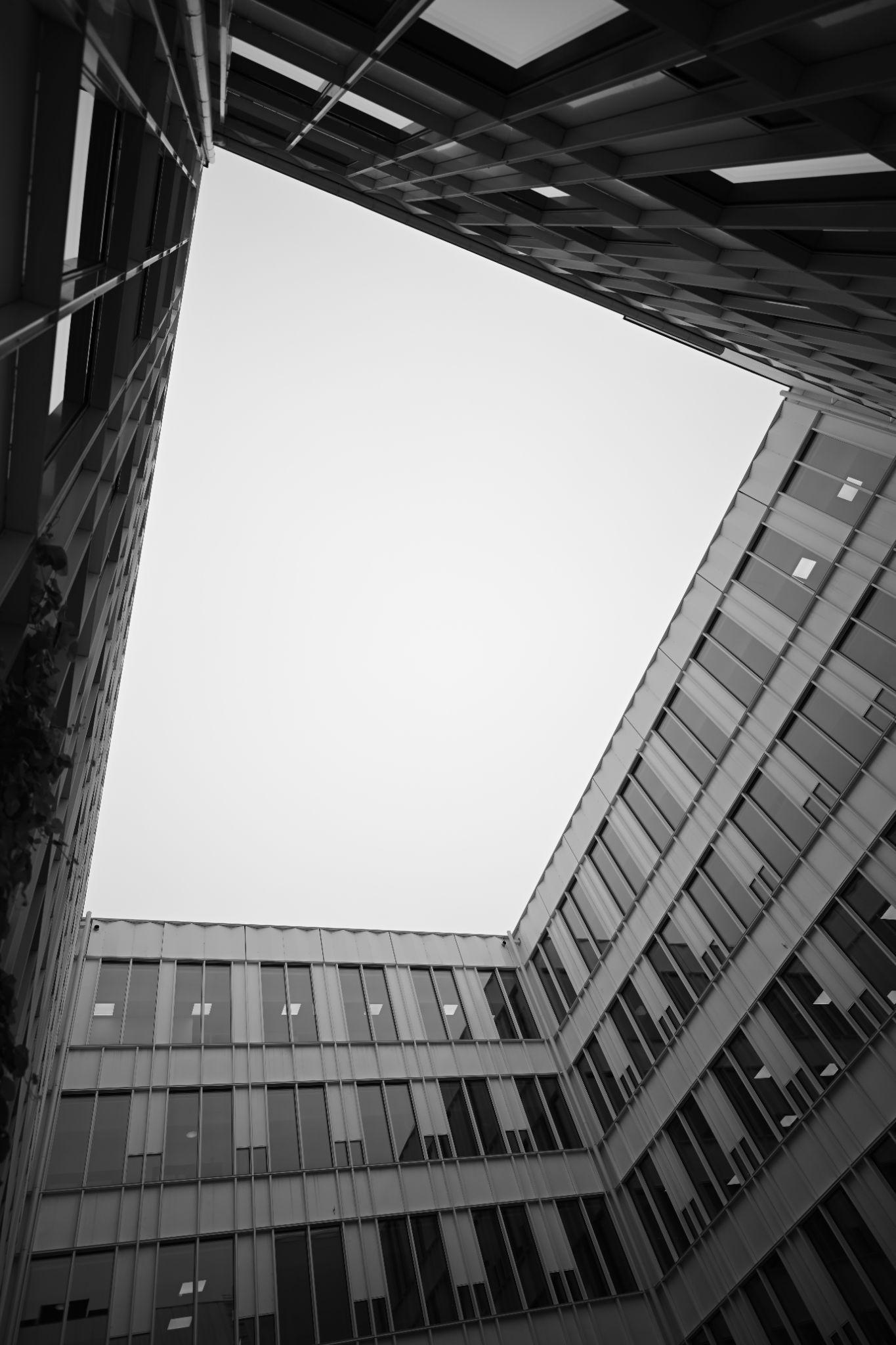
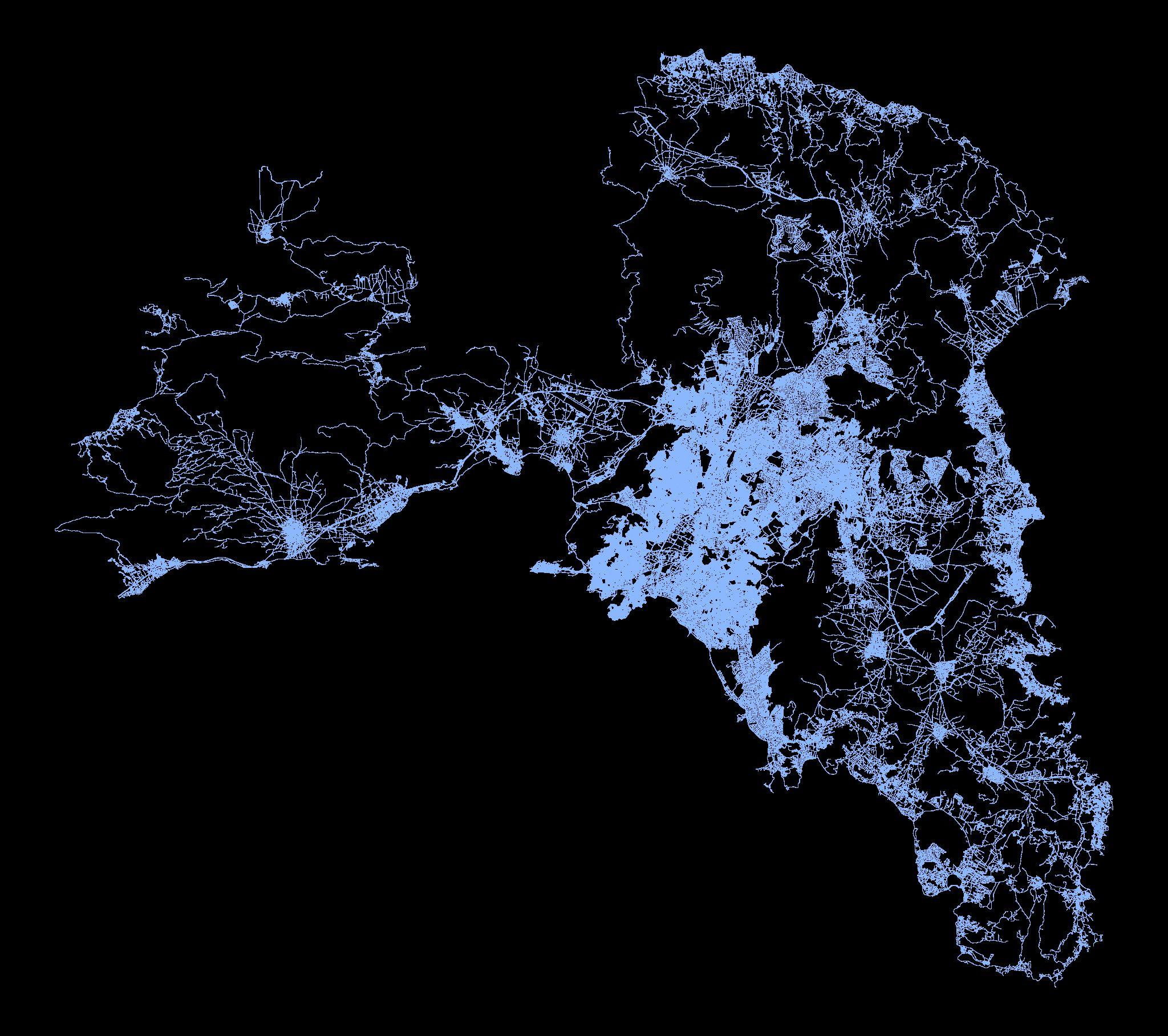
WALKING COURTYARDS
Karlskrona Network Analysis
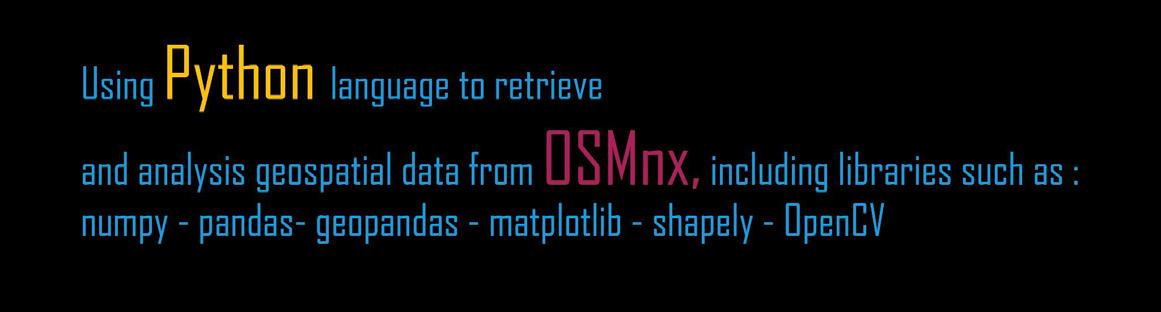
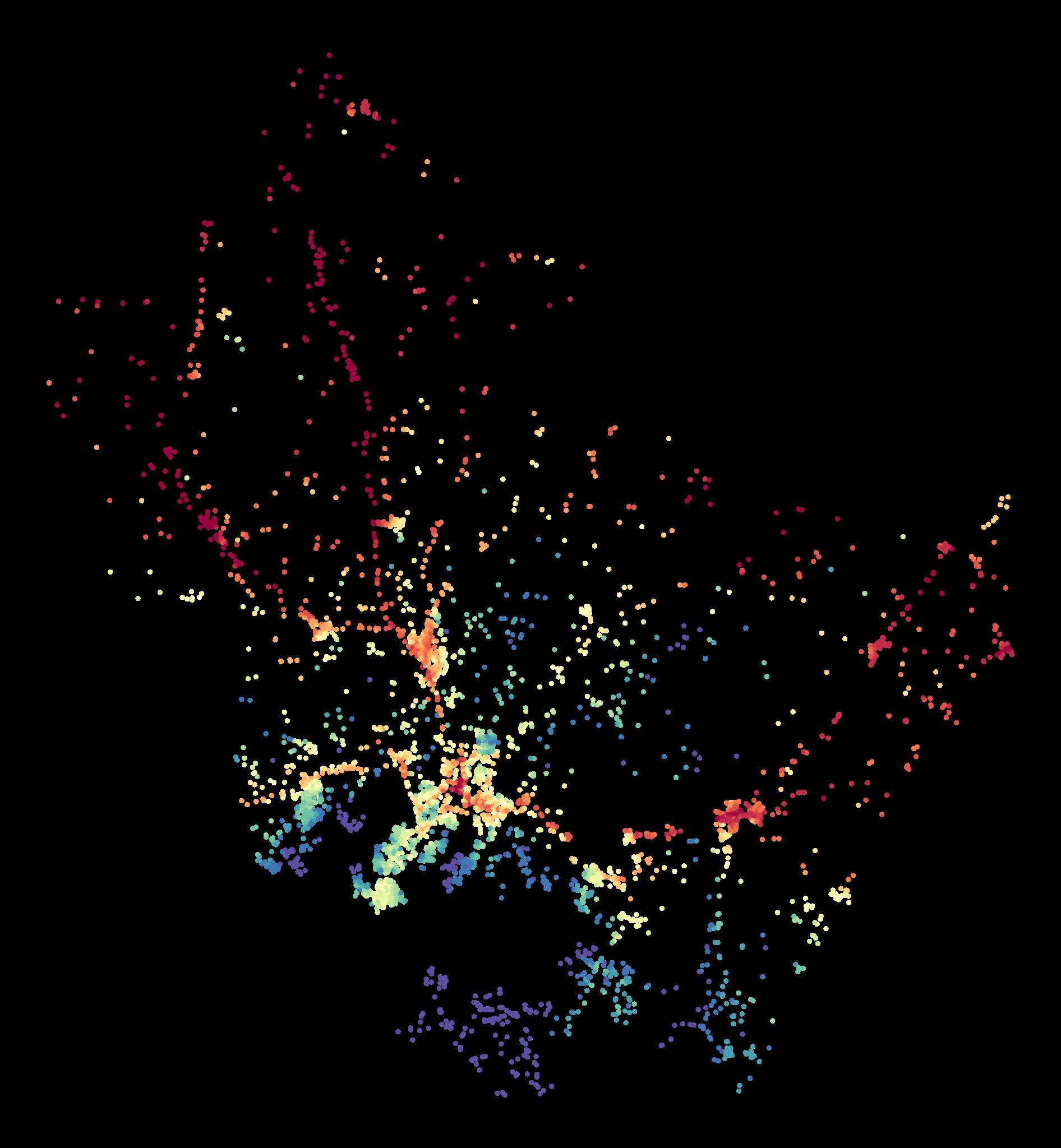
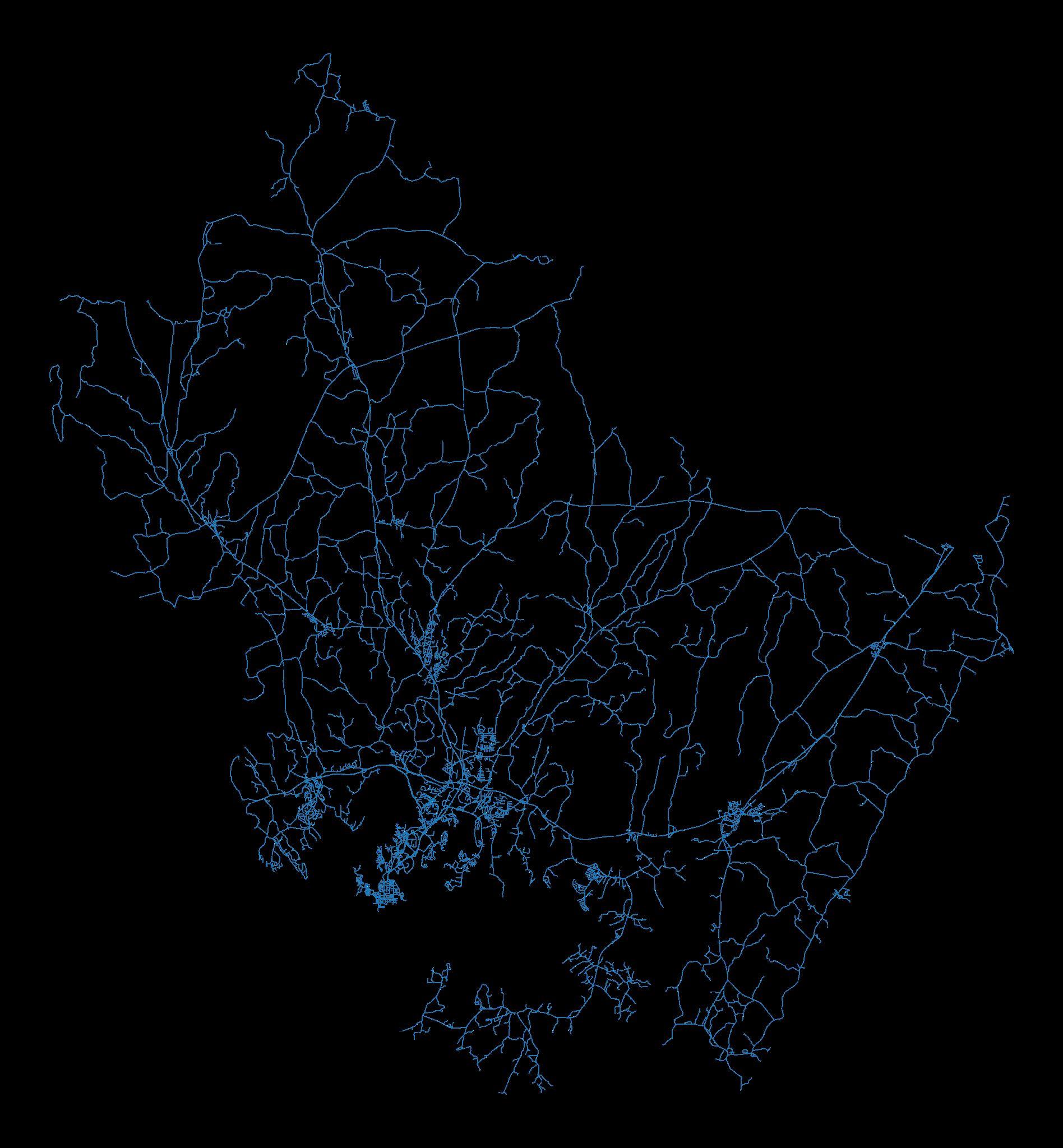
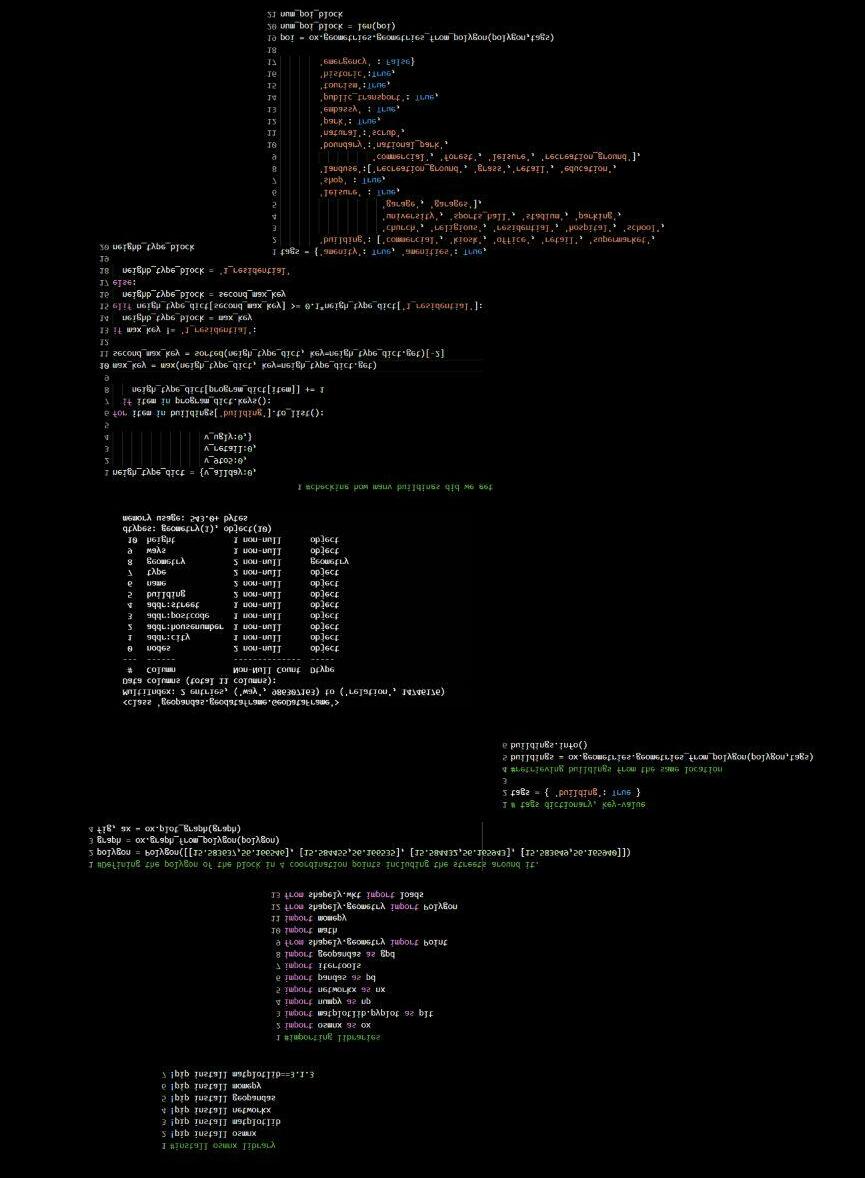
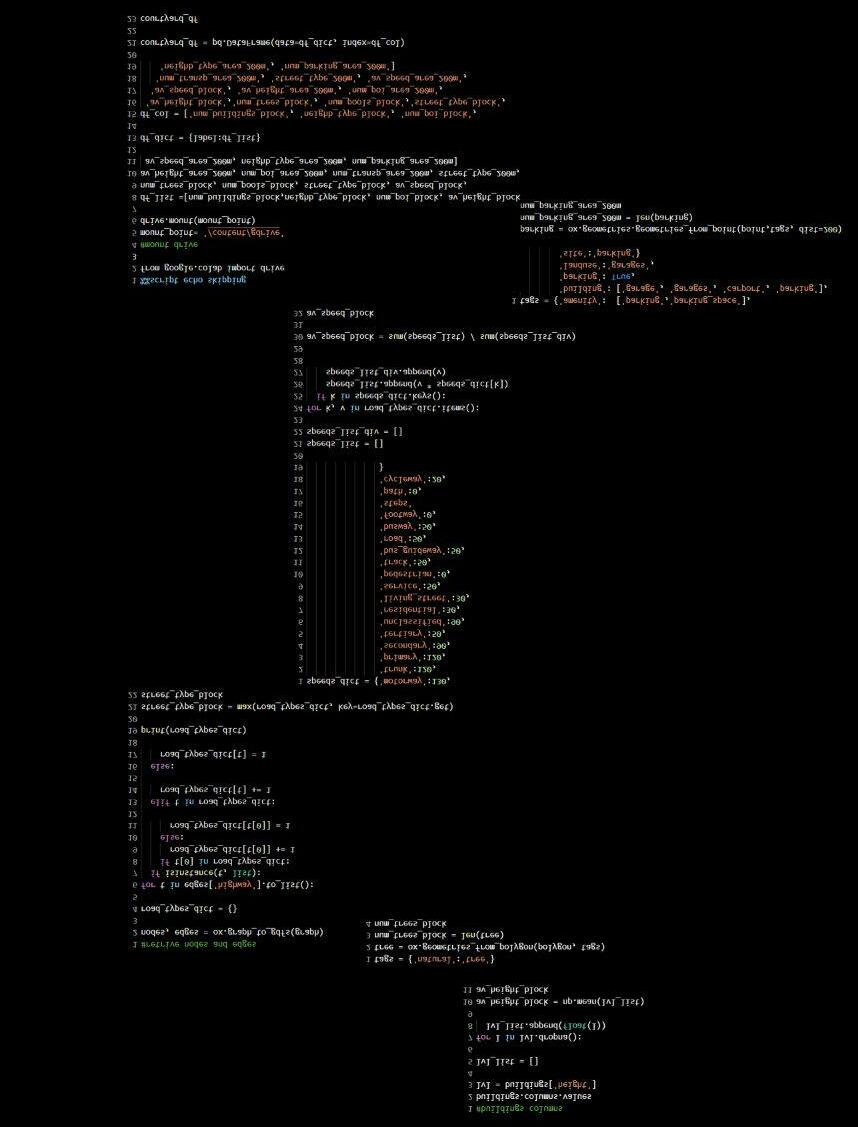
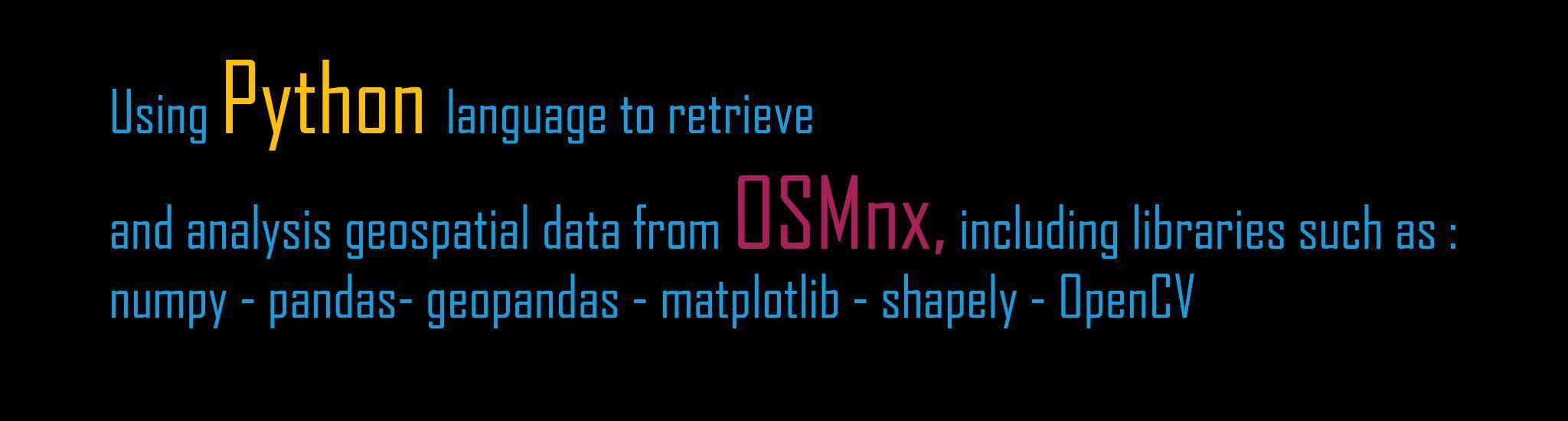
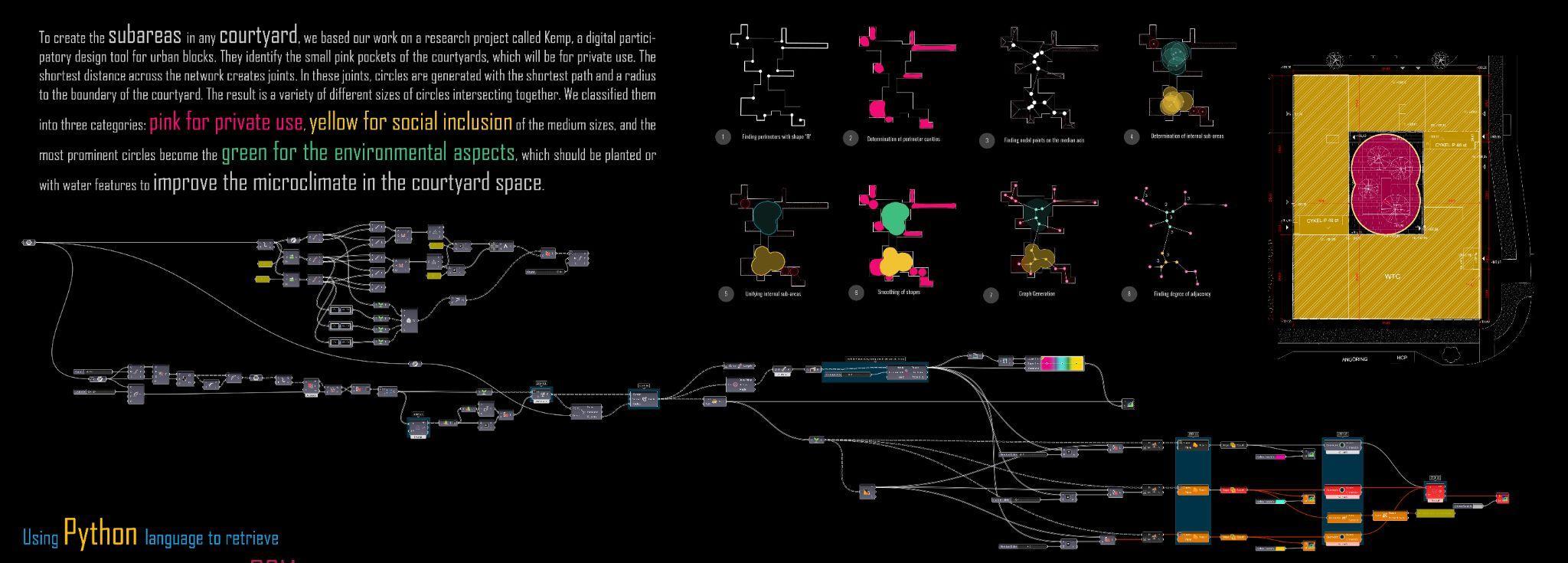
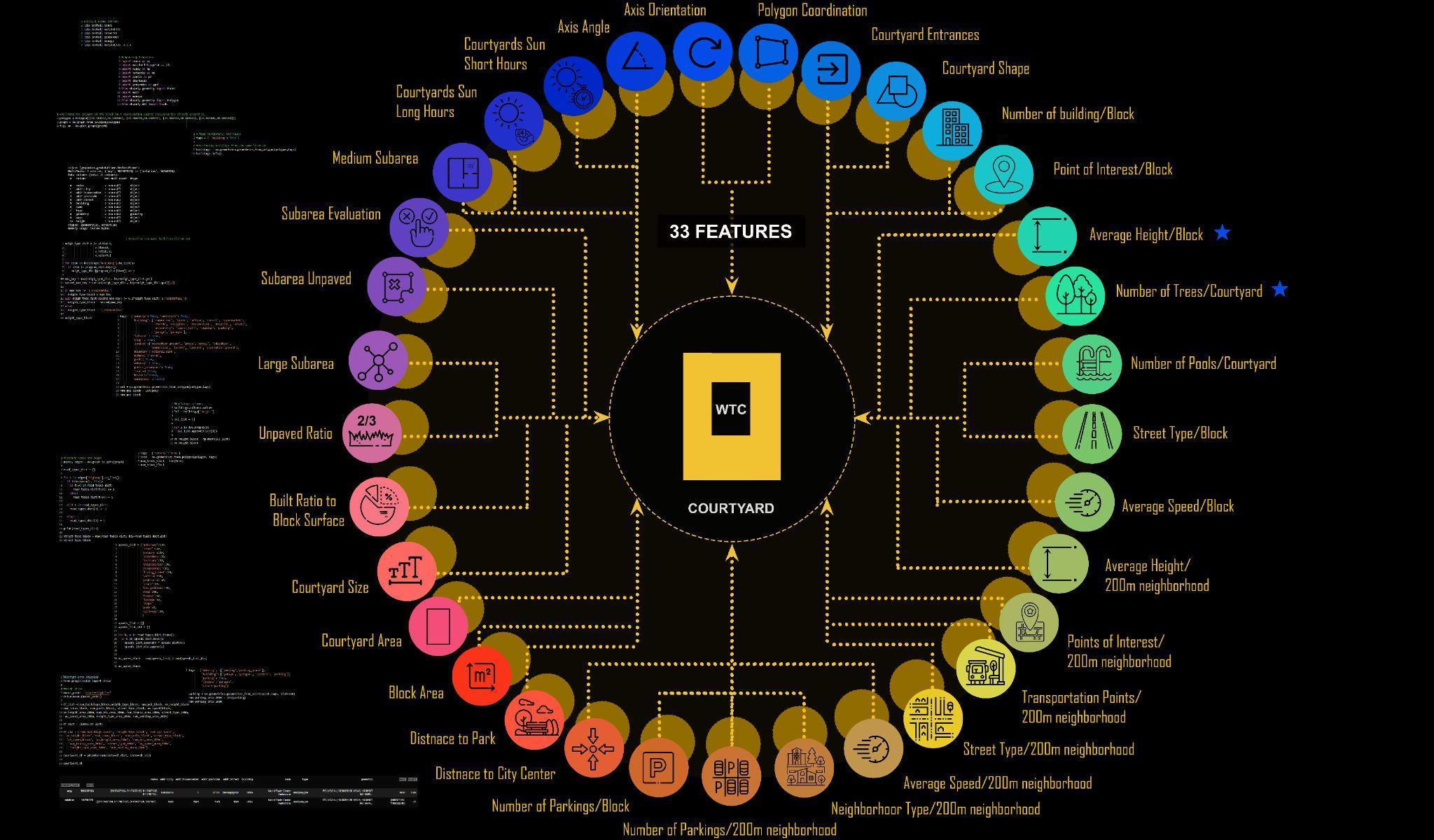
Straightness
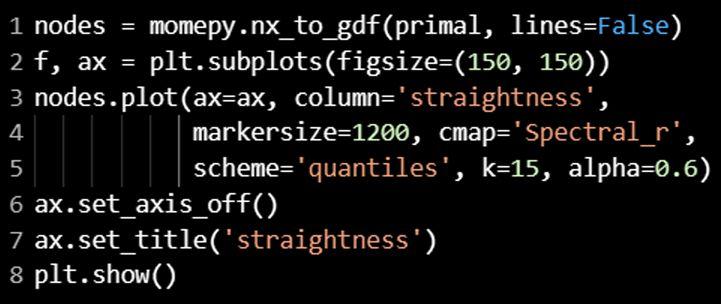
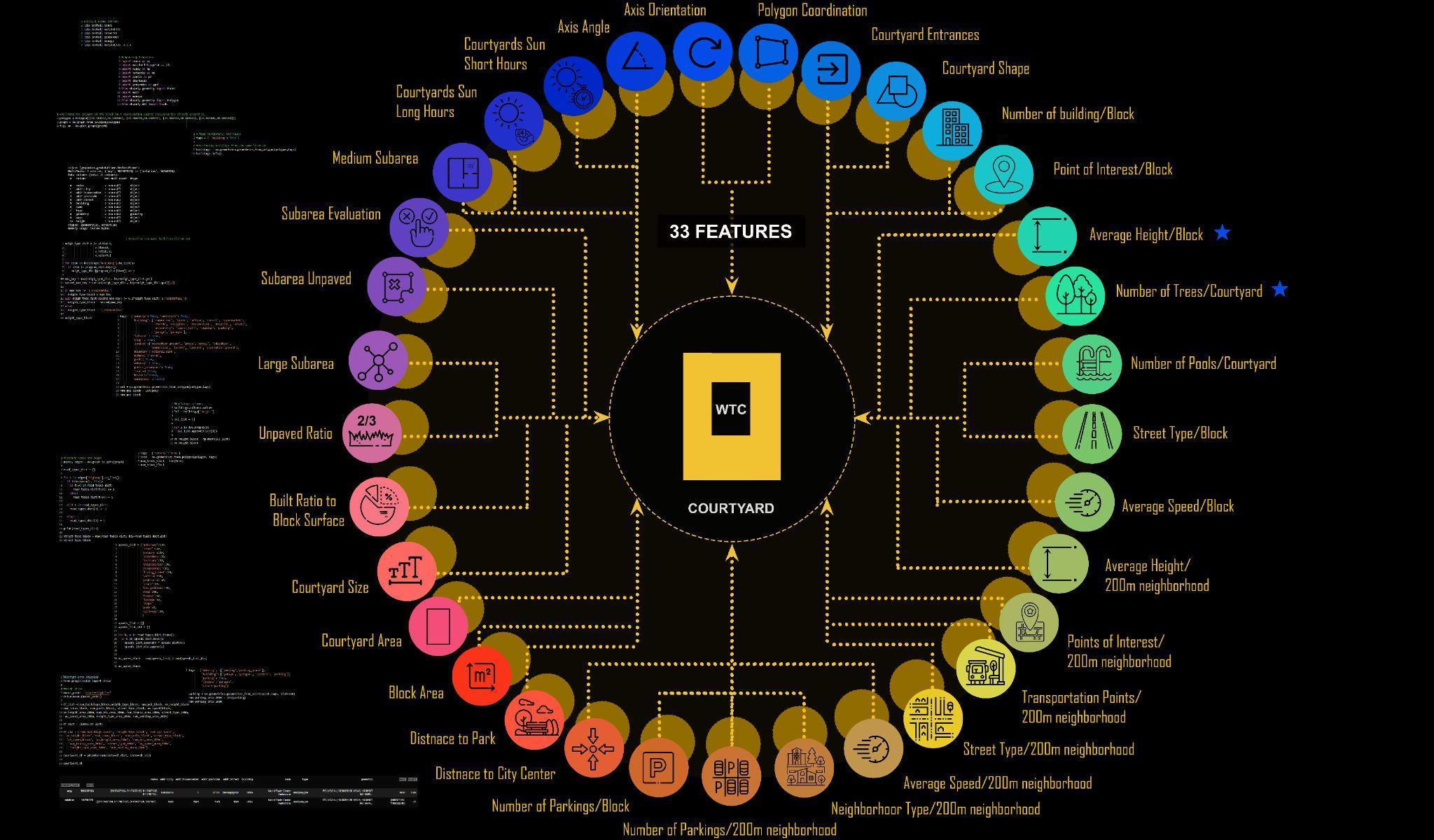
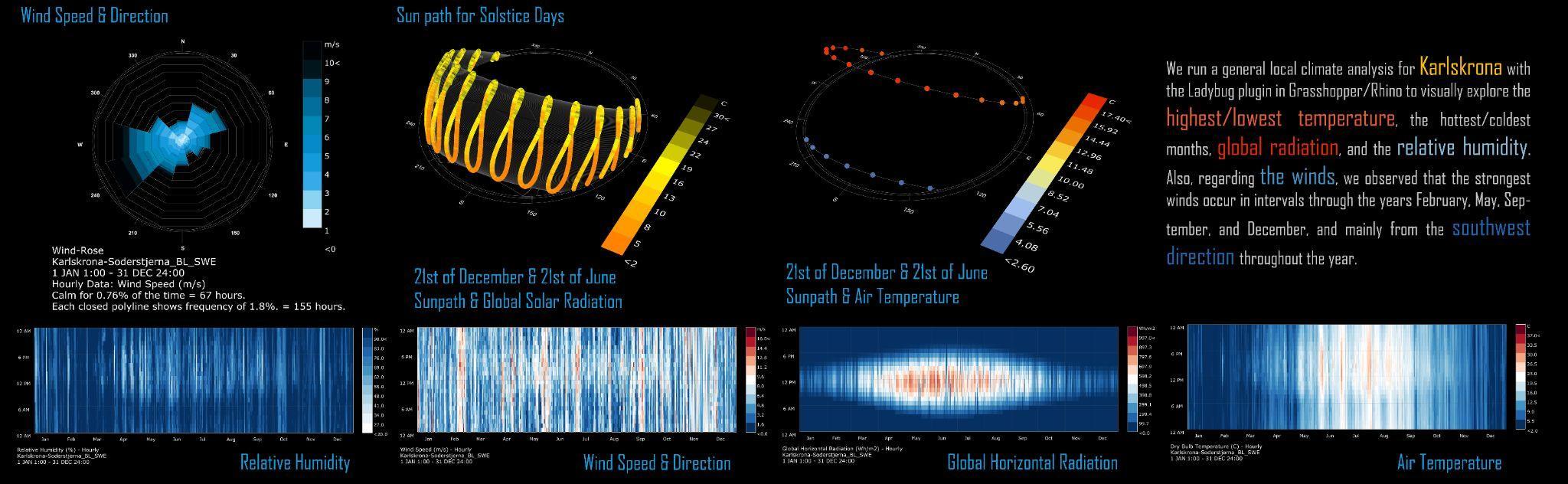
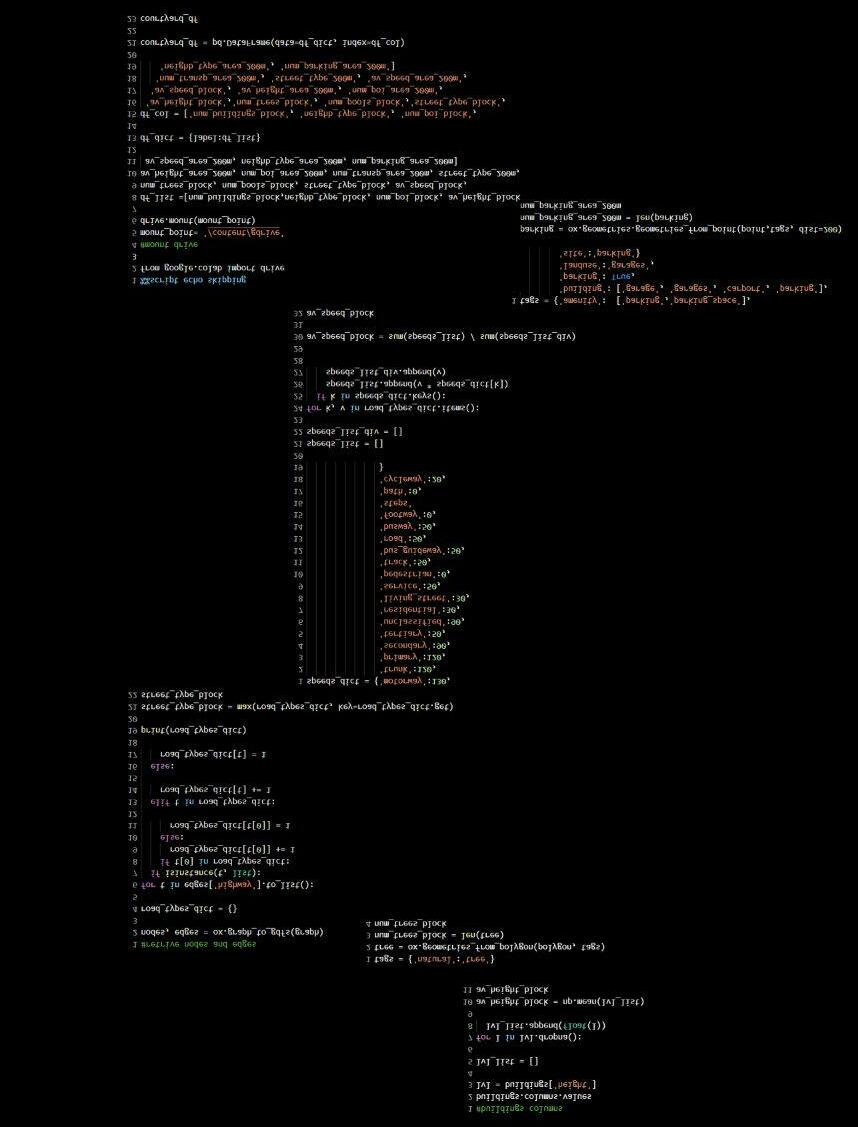
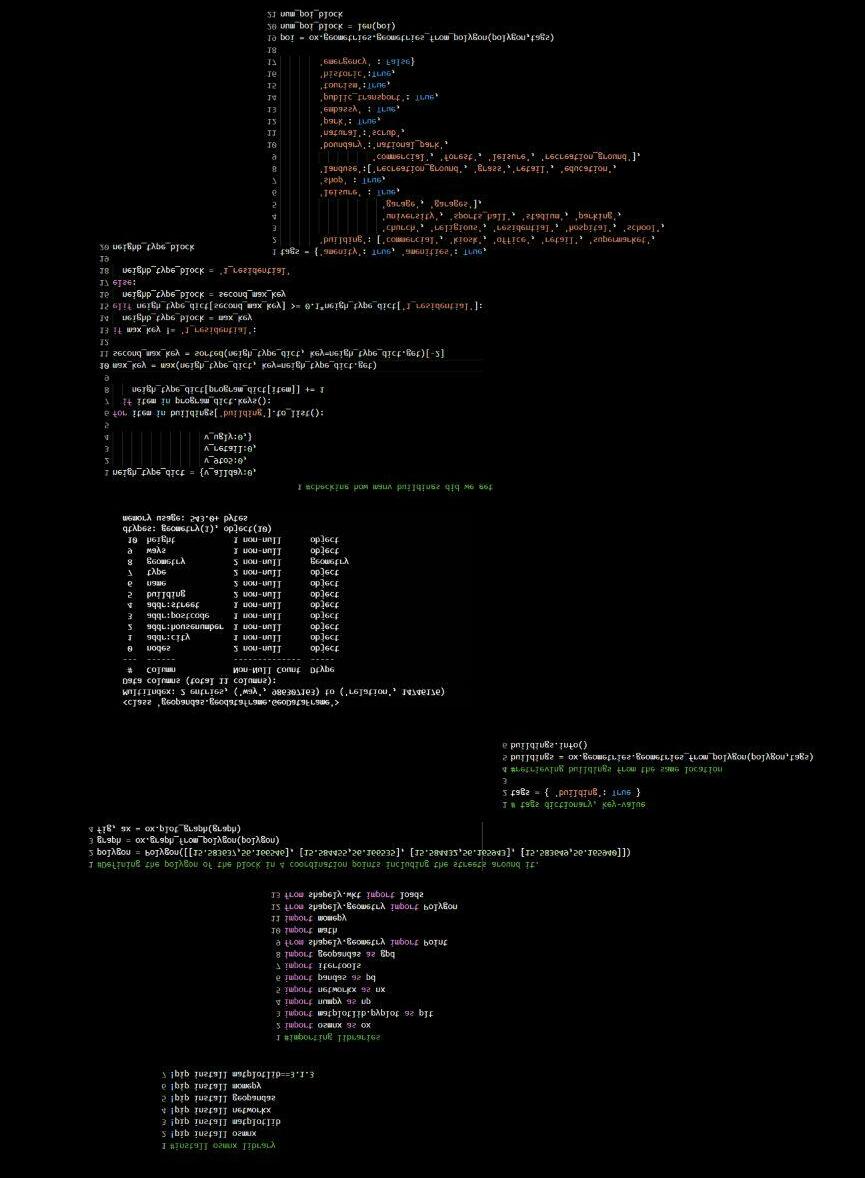
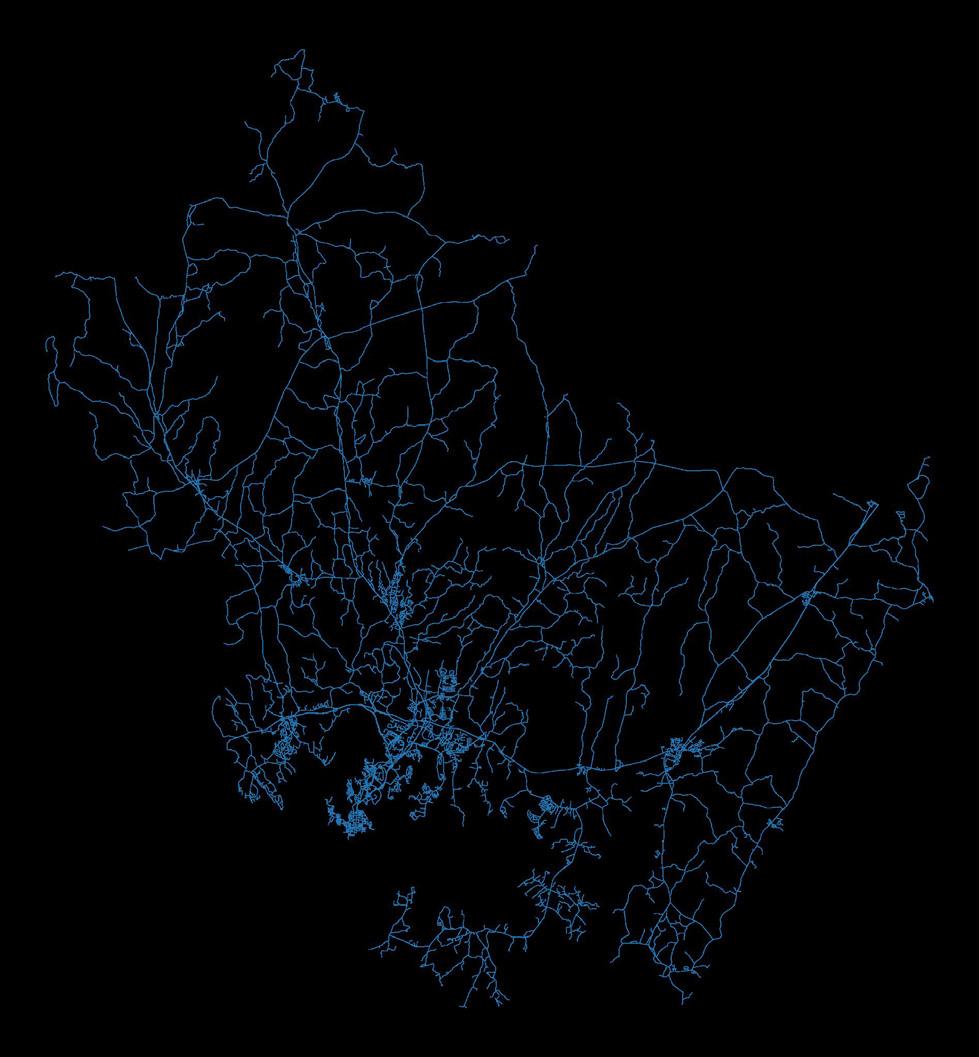
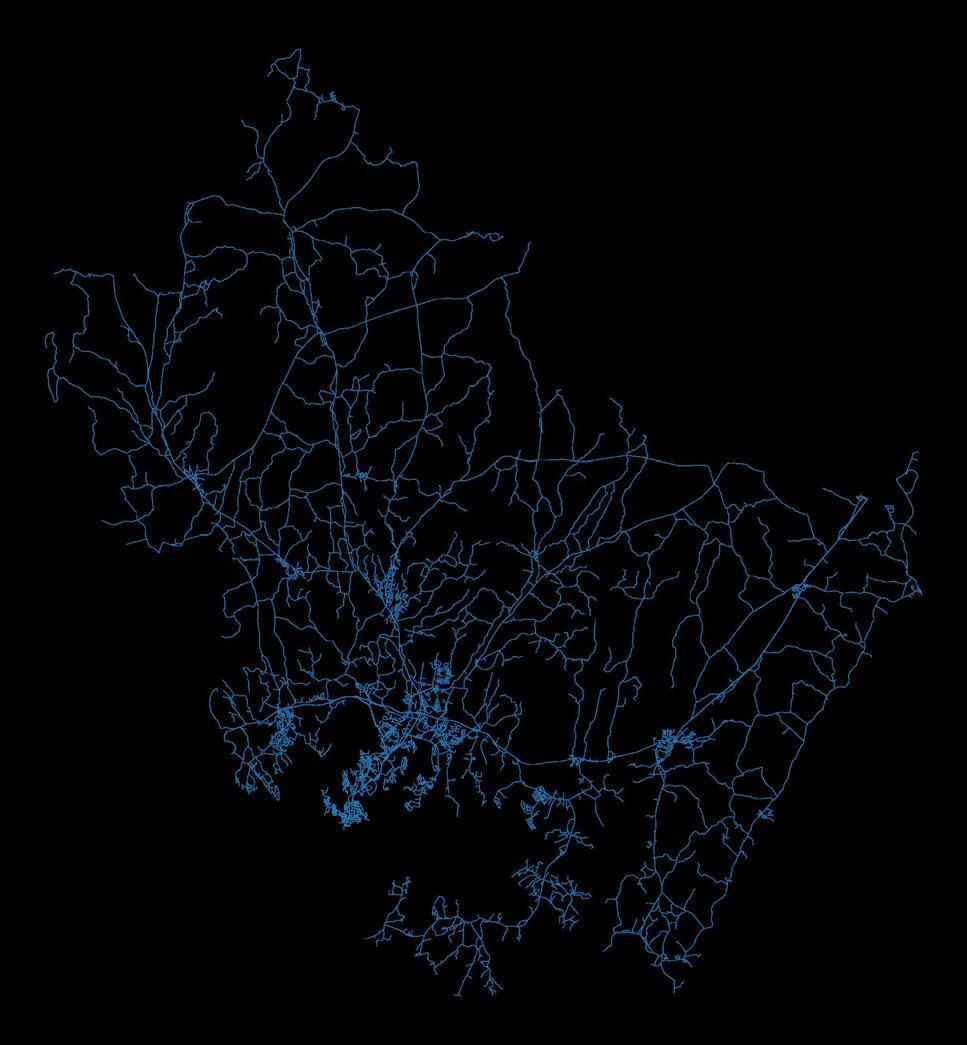
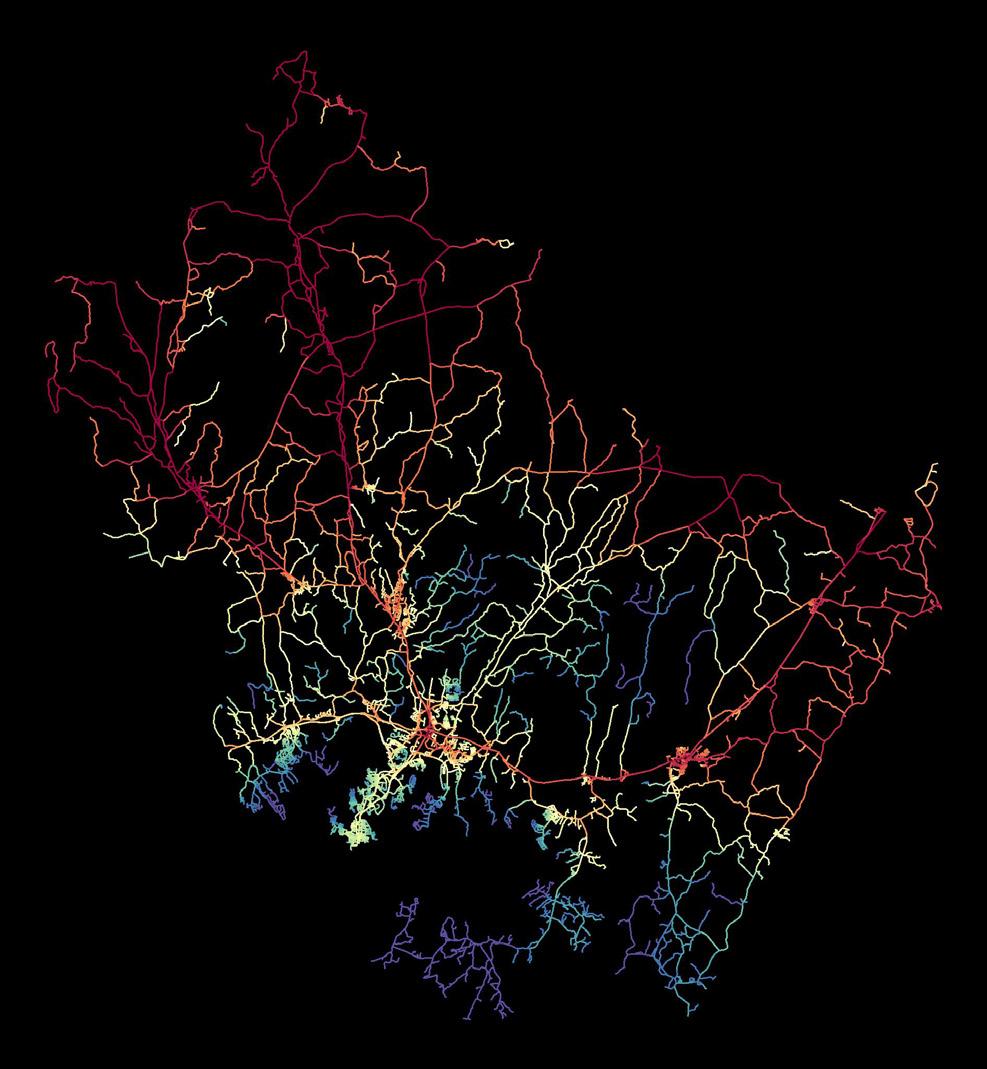
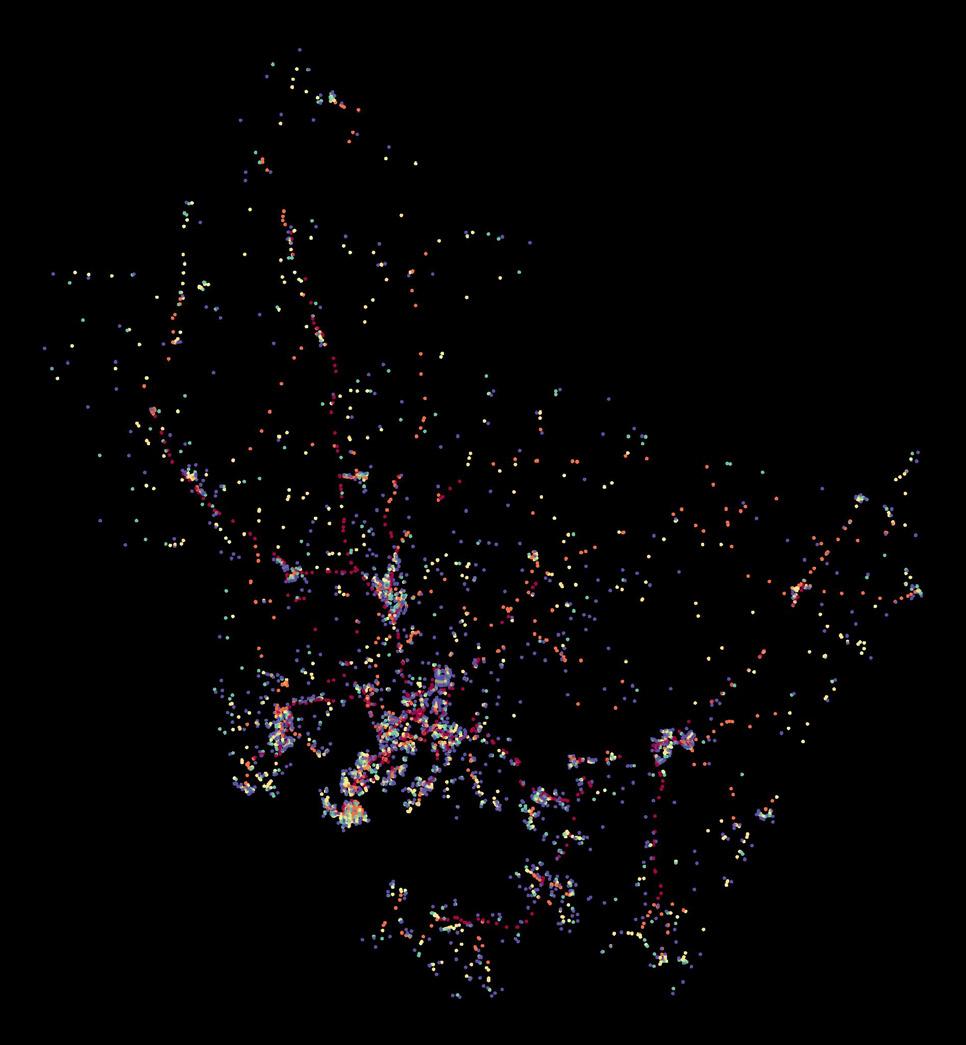
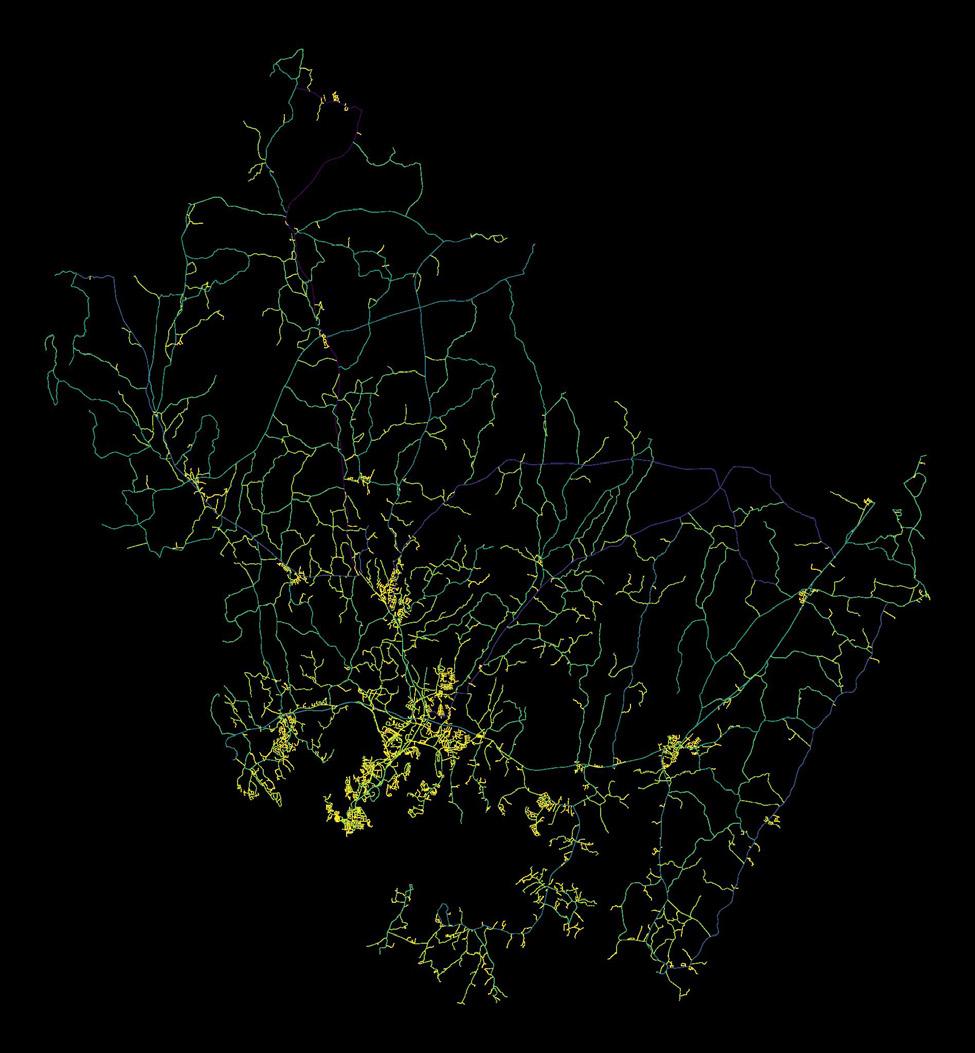
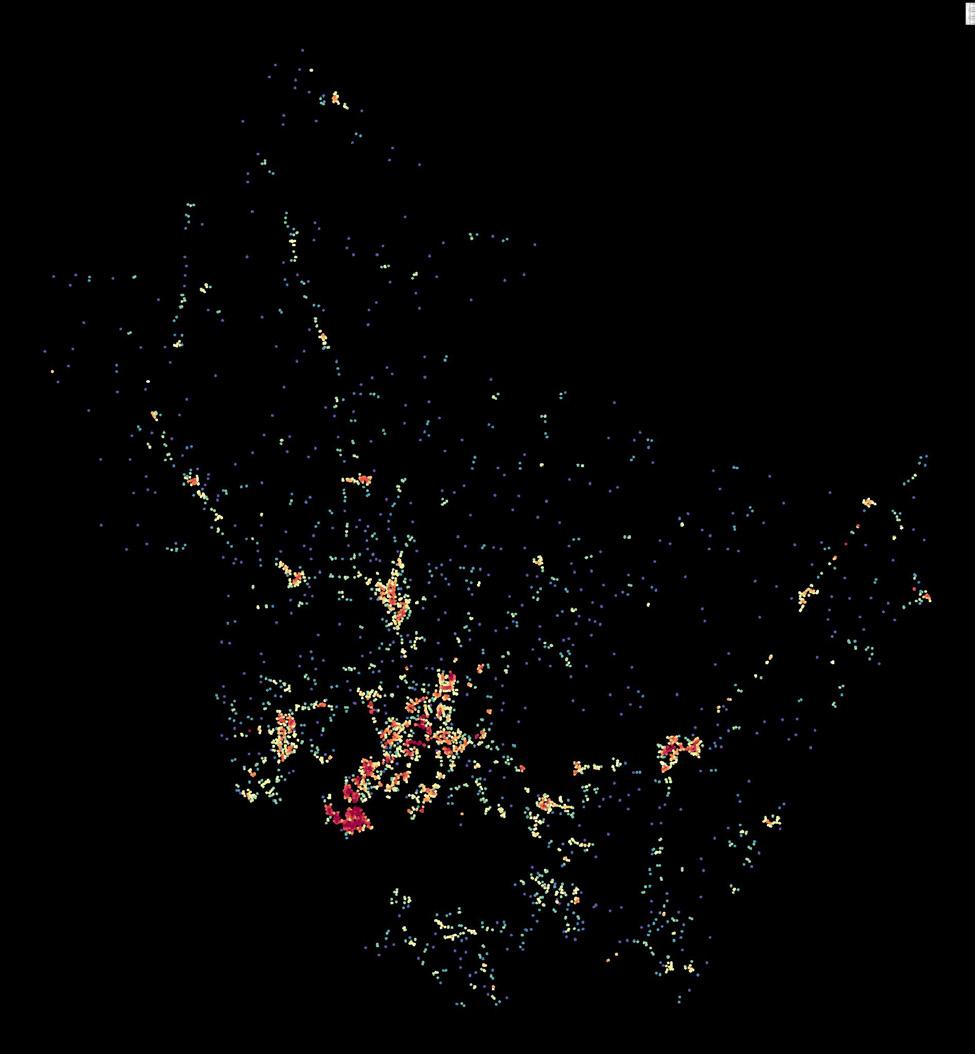
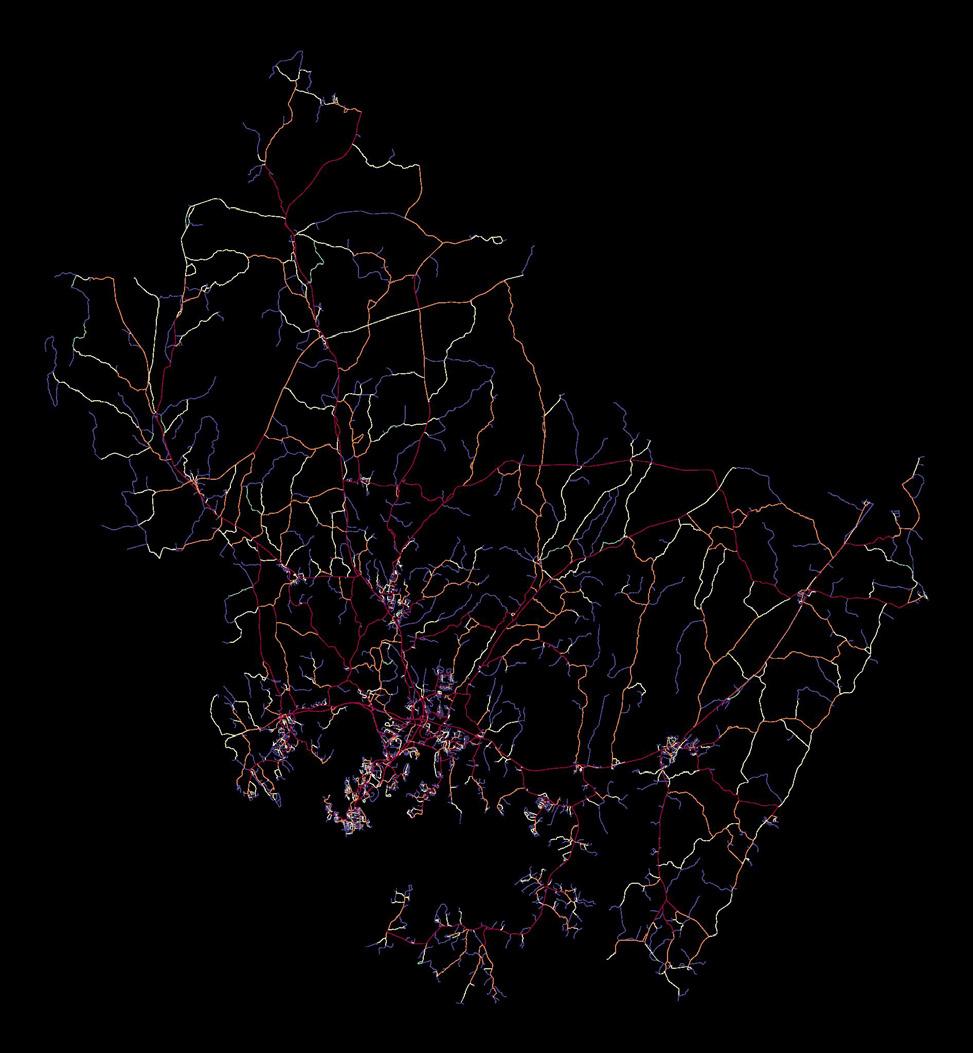
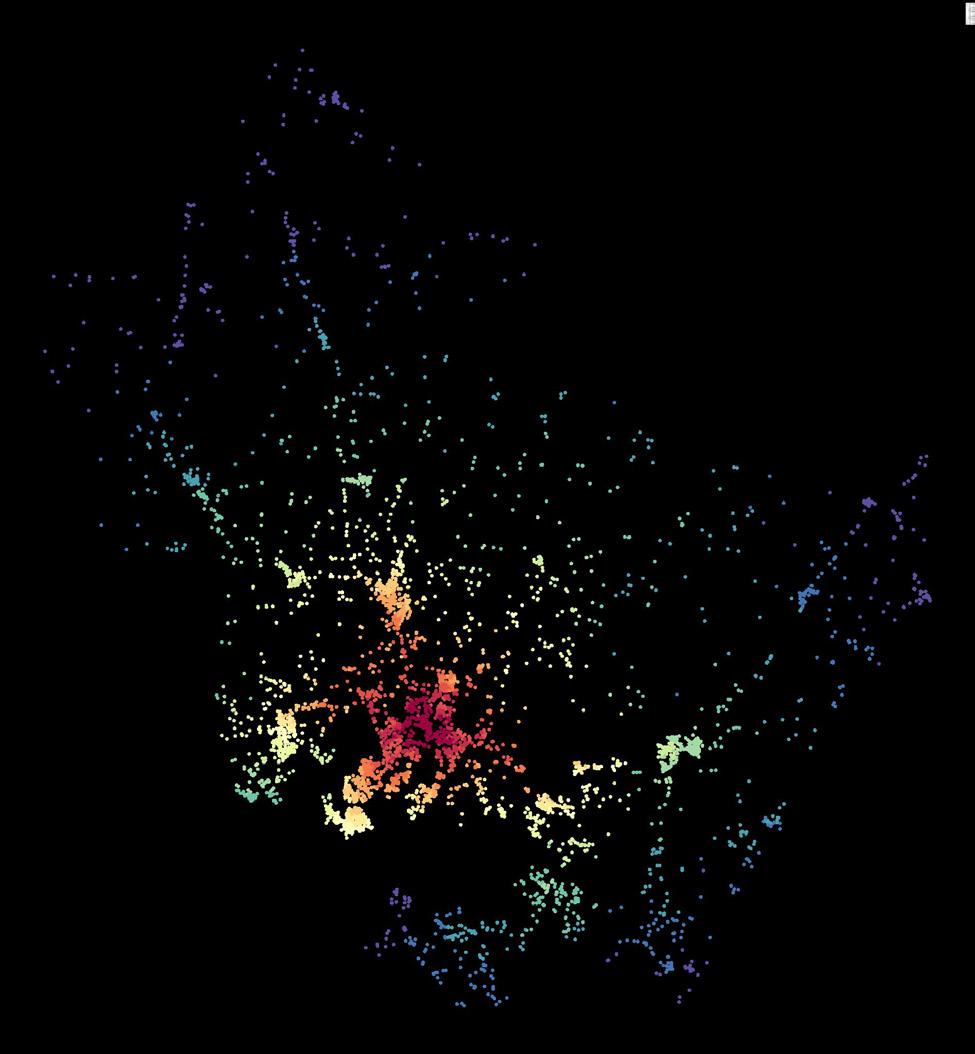
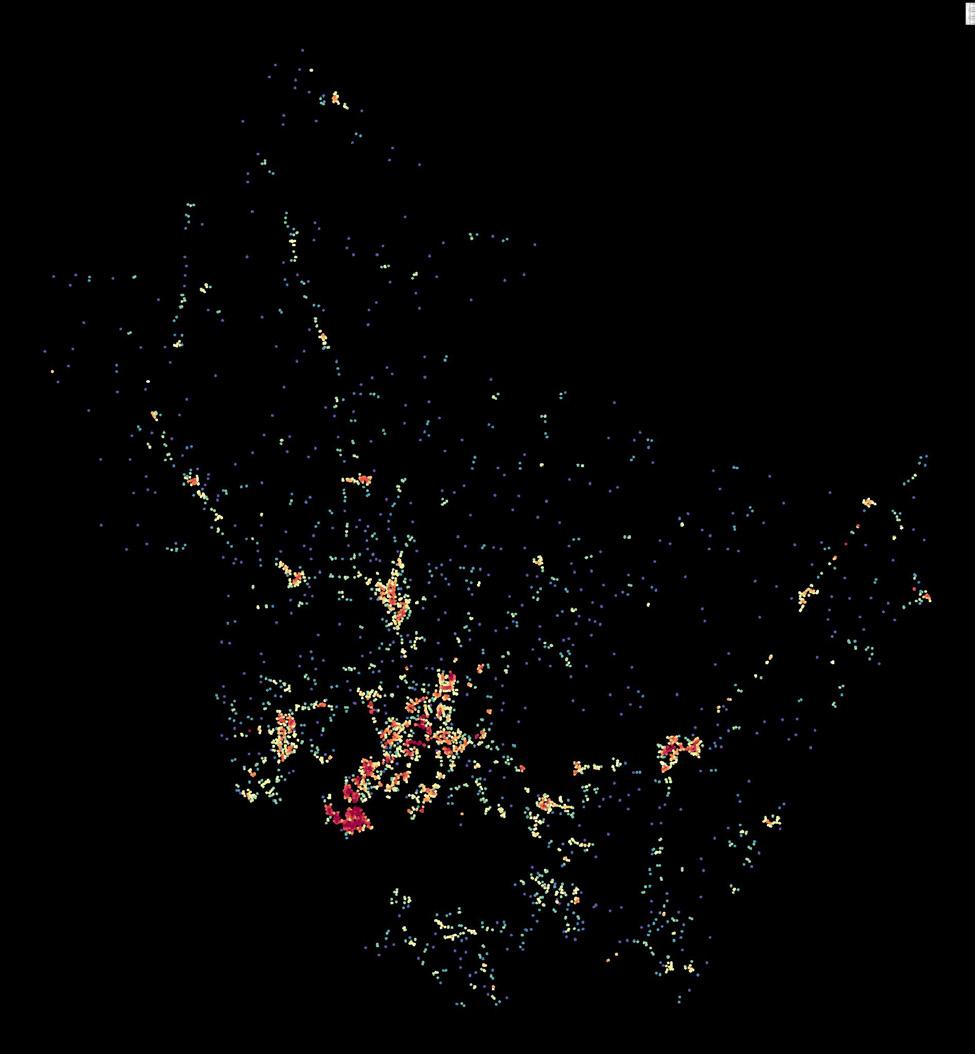
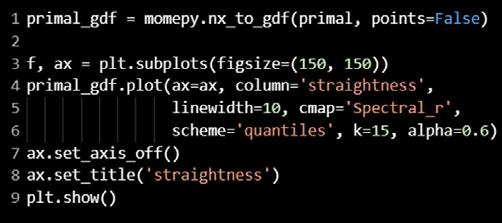
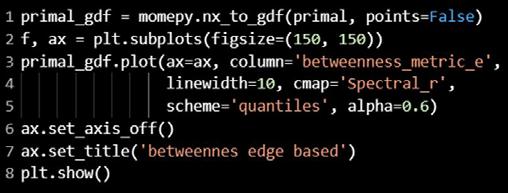
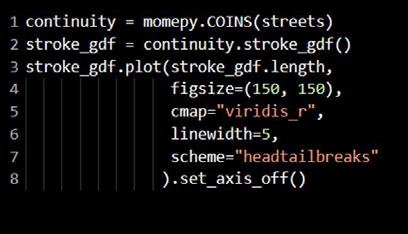

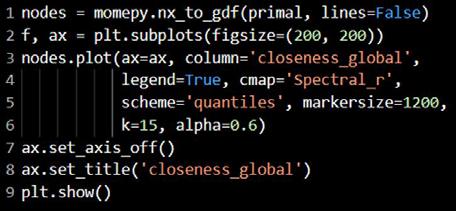
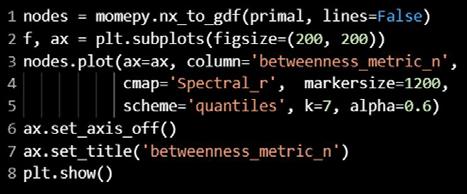
WALKING COURTYARDS
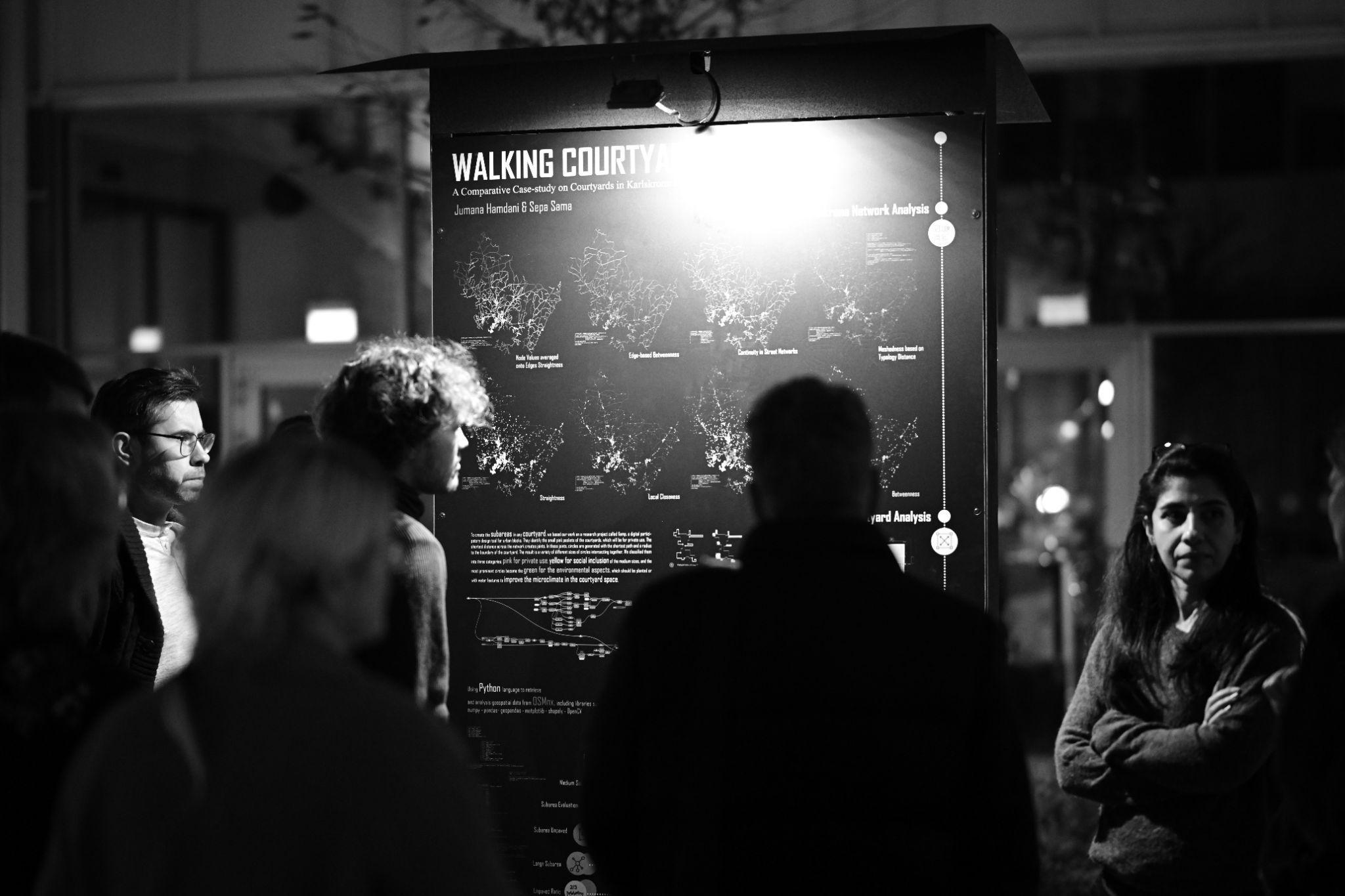
EXHIBITION & WALK
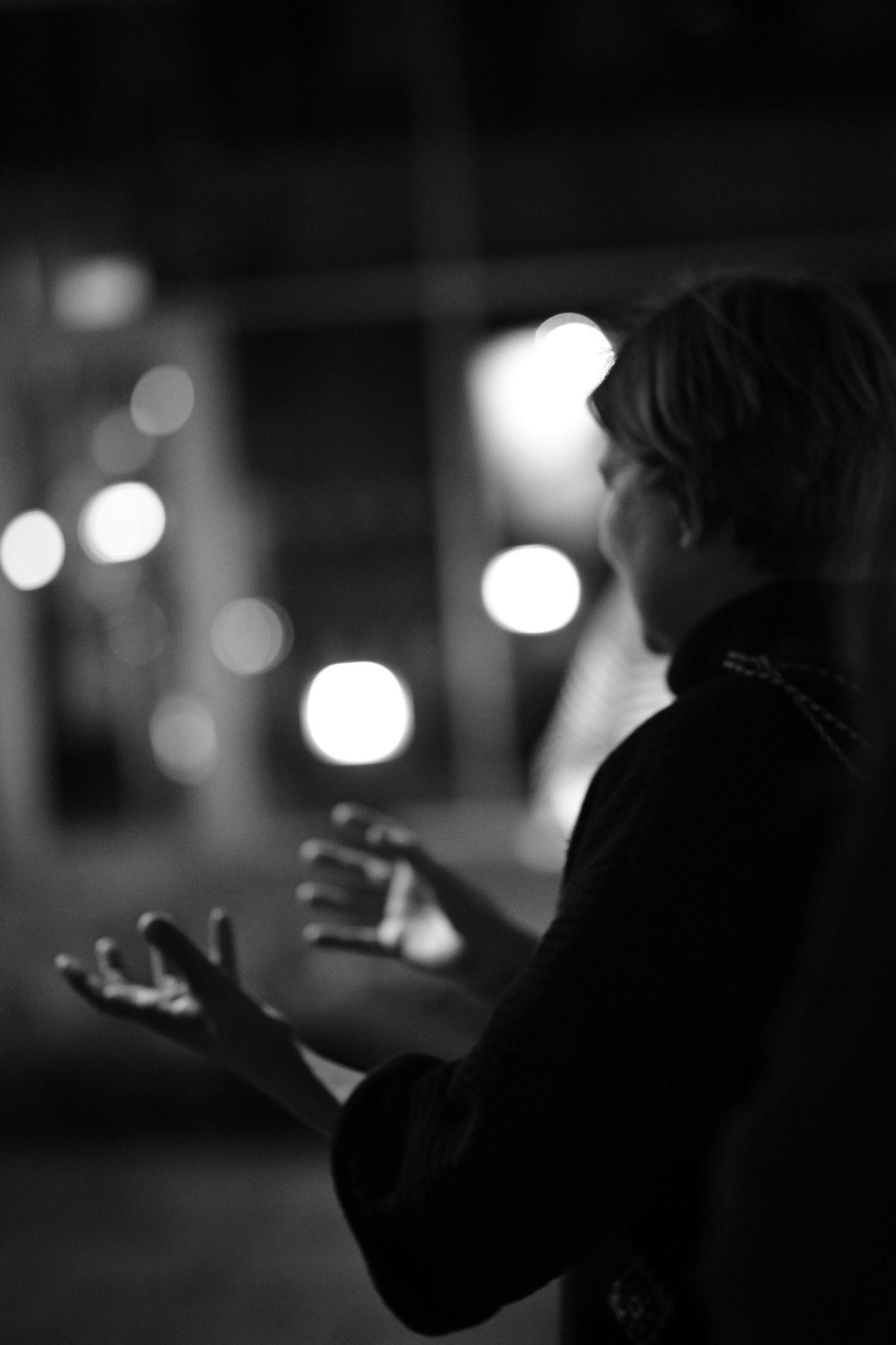
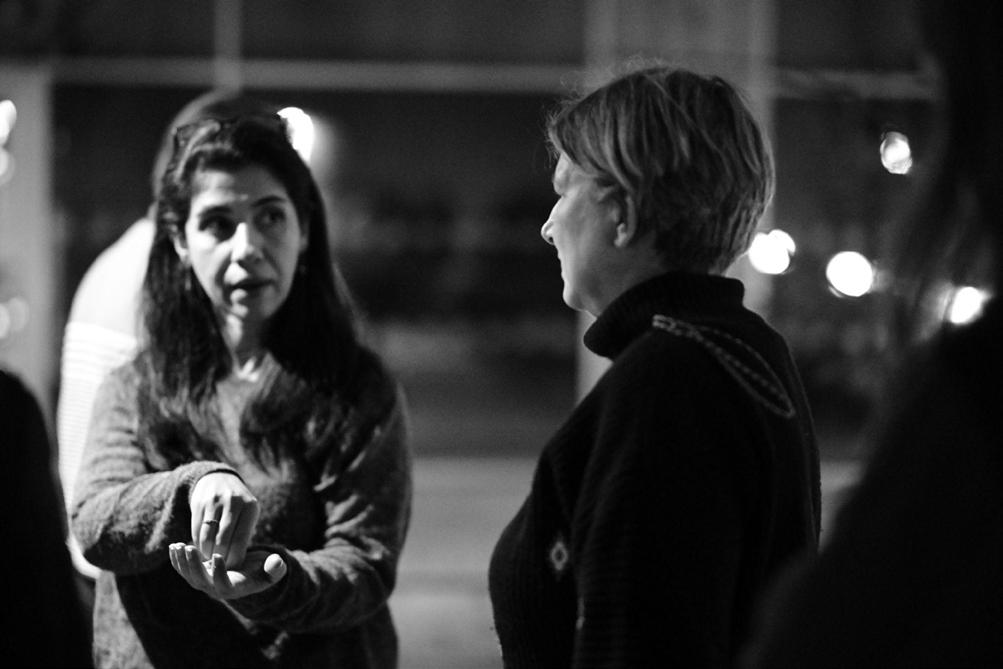
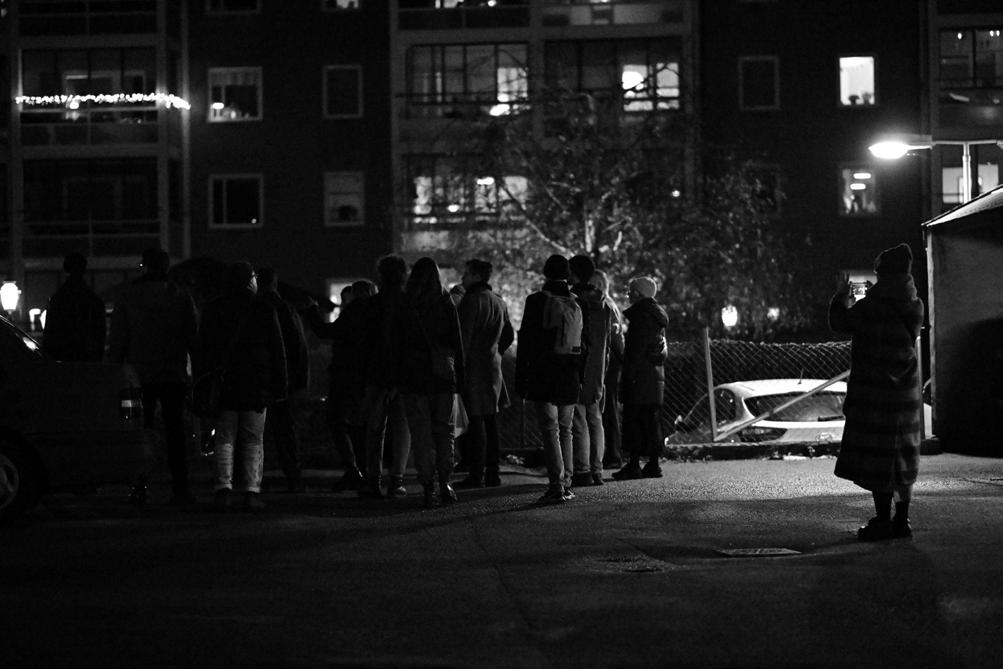
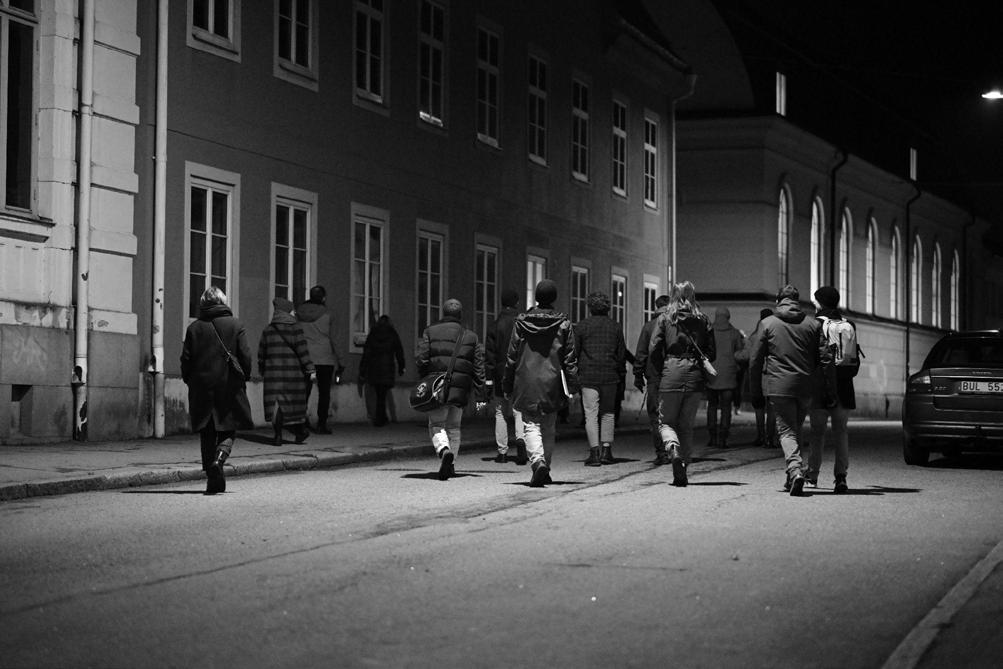
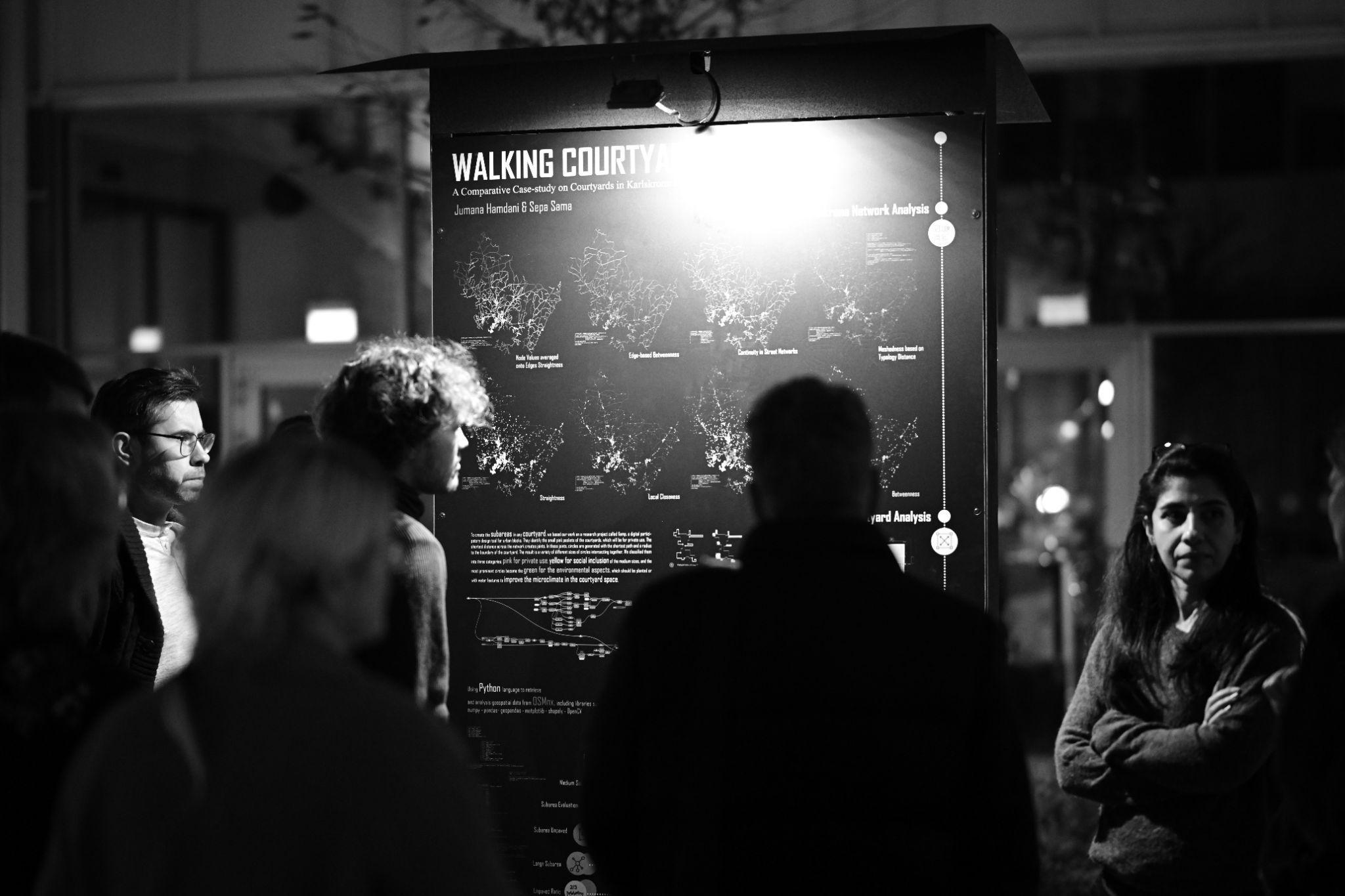
WALK 21 SEOUL CONFERENCE
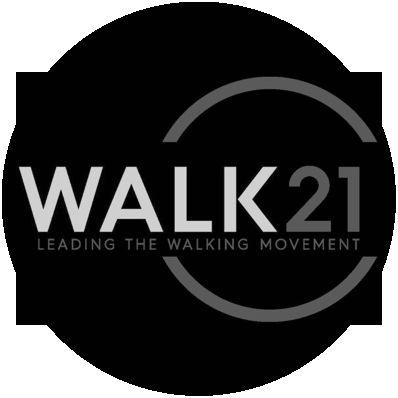
06 Date: 2021
Location: Seoul, South Korea
Collaborators: Jumana Hamdani, Sepa Sama
Project Type: Won the Poster Session at Walk21 Conference
Tools: Rhino, Grasshopper, 3D Max, Photoshop
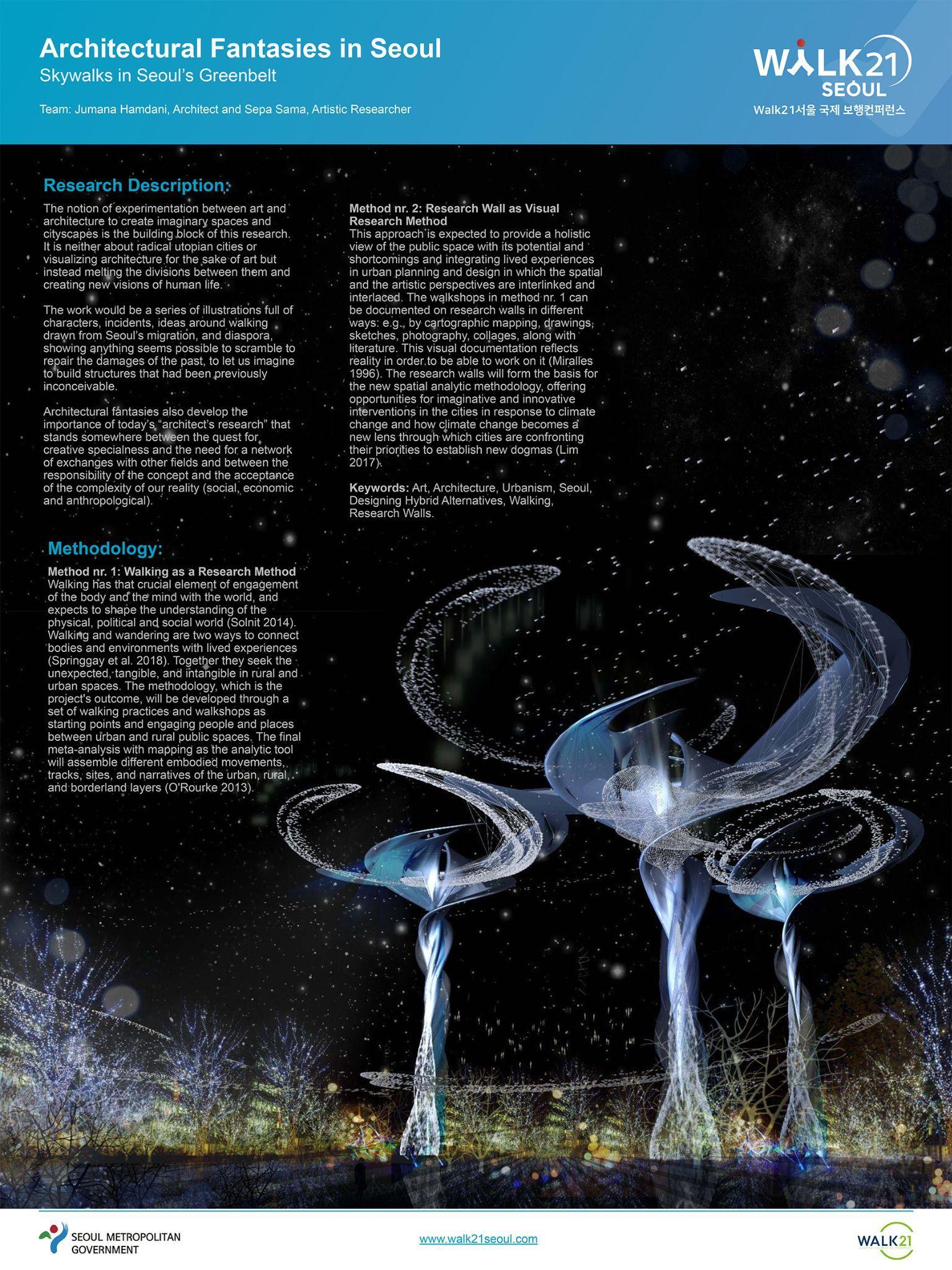
SALT PAVILION
07 Date: 2021
Location: Salar de Uyuni, Bolivia
Collaborators: Abed Badran, Jumana Hamdani
Instructor: Rodrigo Aguirre
Project Type: Academic at IAAC

Tools: Rhino,Grasshopper including plugins: Voronoi, Kangaroo, Wallacei, 3D Max, Photoshop
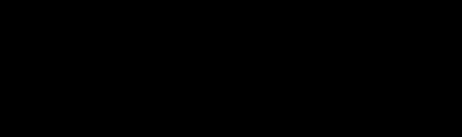
SALT PAVILION
, Bolivia exploring the natural system of the cancellous spongy bone structure. The purpose of the project is to define a new urban structure, using Salt as a local material that can evolves through time.
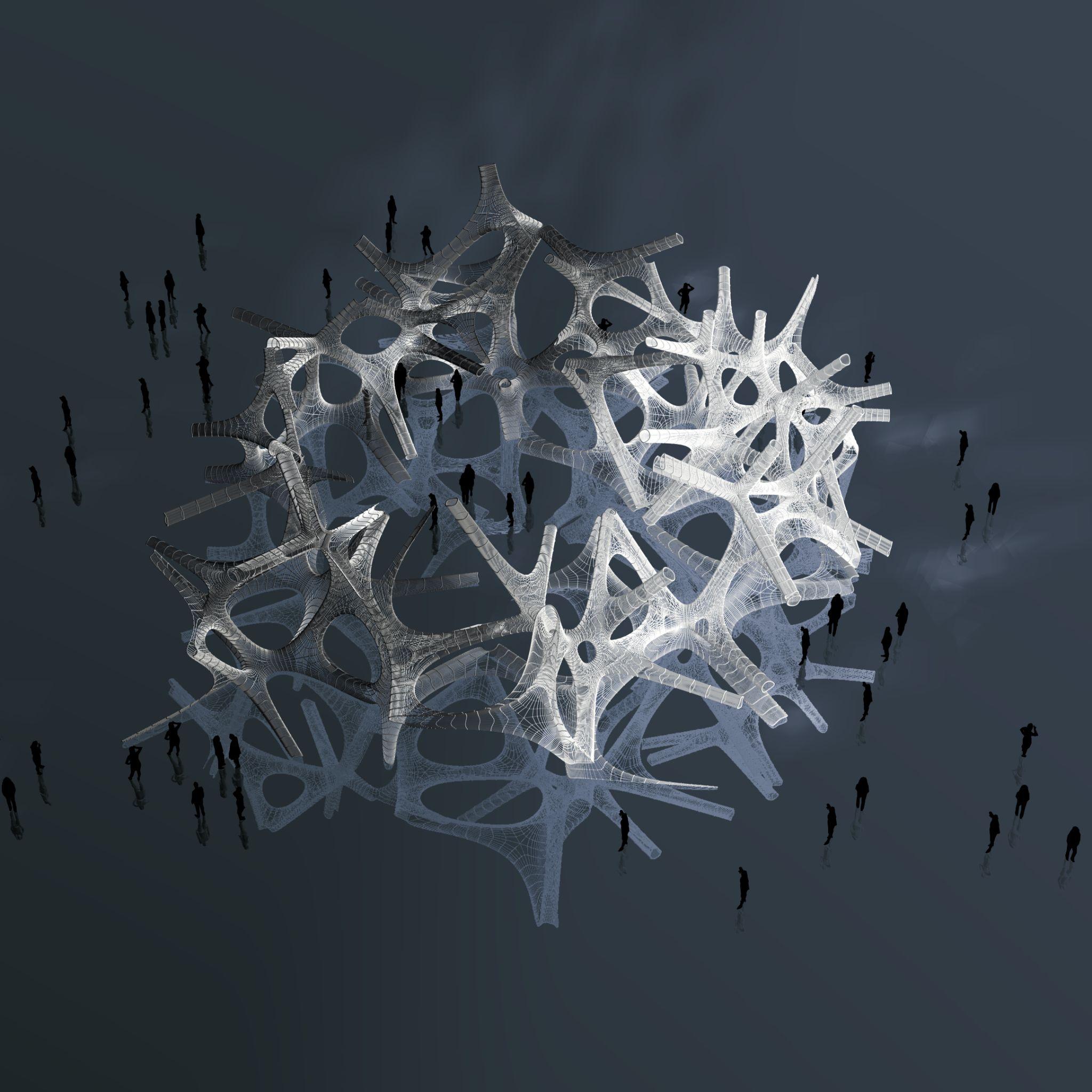

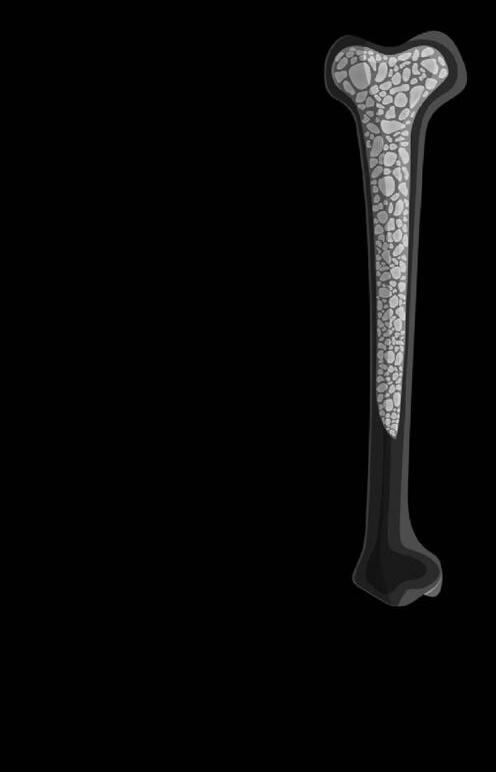
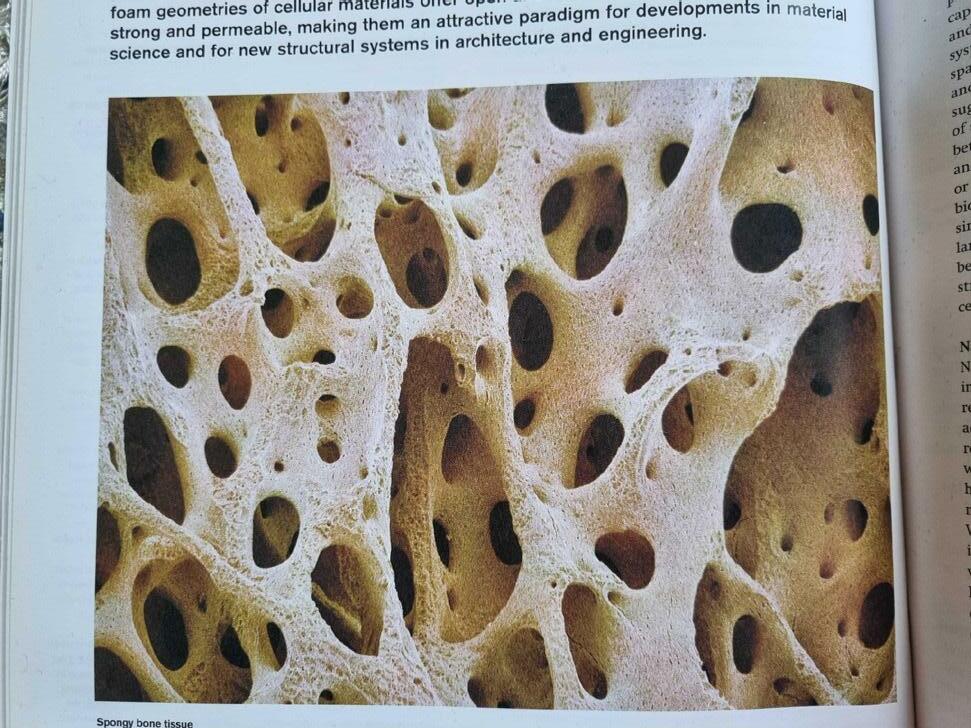
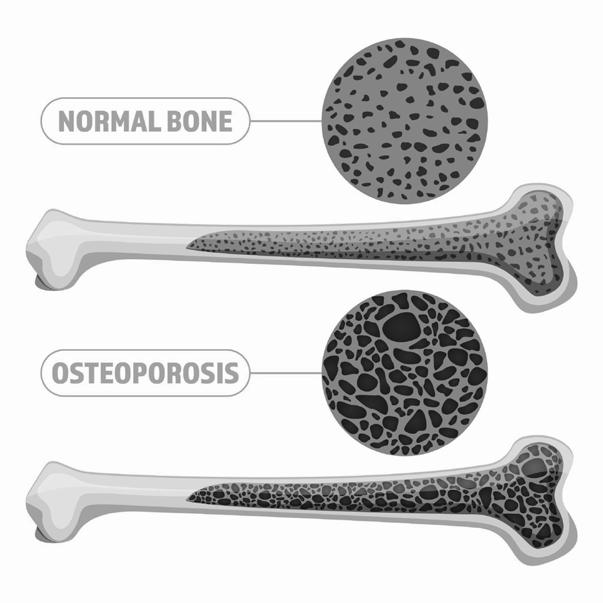
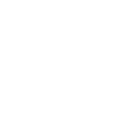
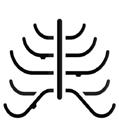
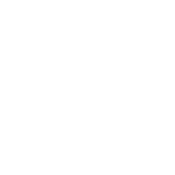

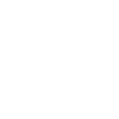




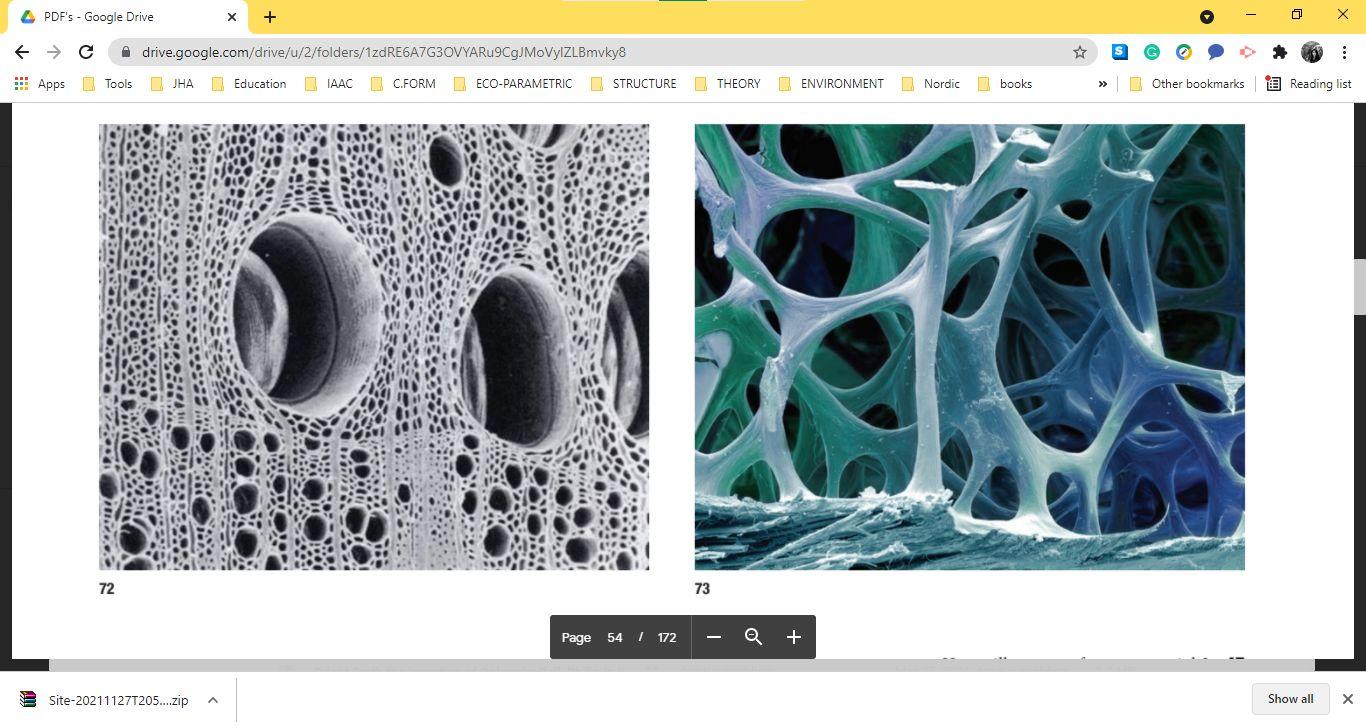
BONE STRUCTURAL BEHAVIOUR
Wolff's Law
The bone is a hierarchical structure of calcium phosphate and collagen molecules in fibrous, laminar, particulate and porous form.
Secondary tensile stress
Muscles Tension load Point of no Stress
COMPUTATIONAL ANALYSIS OF BONE FRACTURE
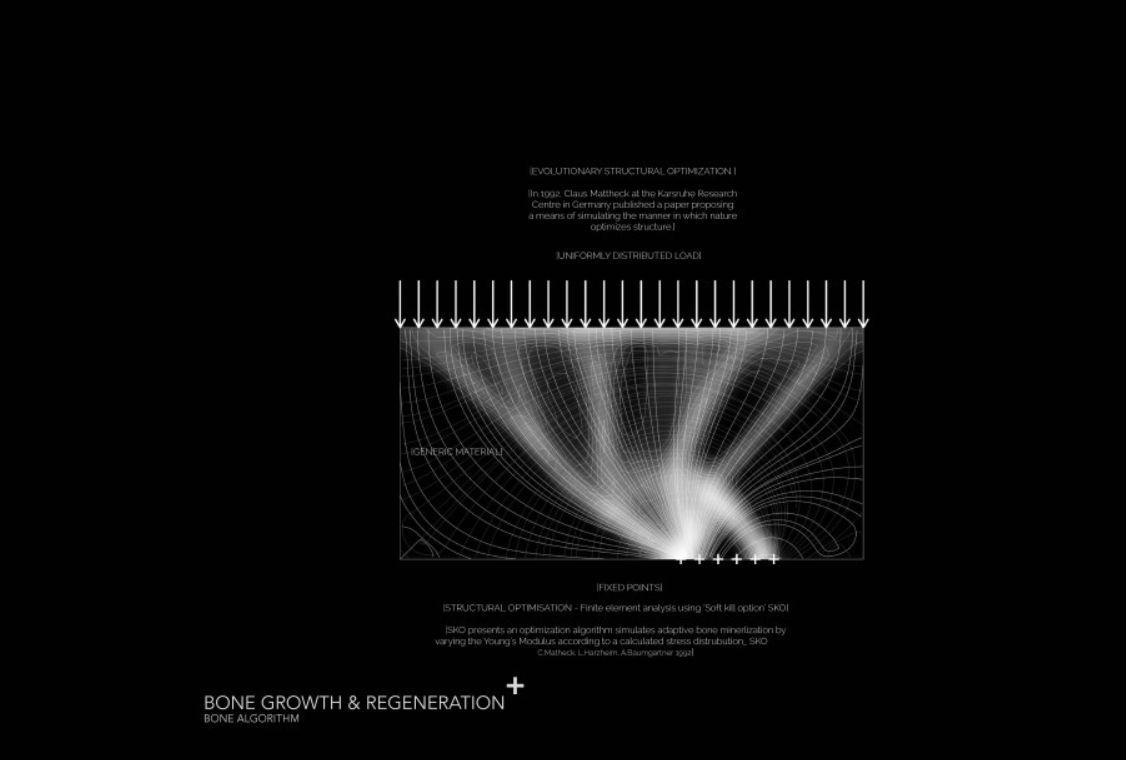
The goal is to understand the structural behaviour of the spongy bone, in order to extract an algorithm that can be applied to influence the formation of the Pavilion
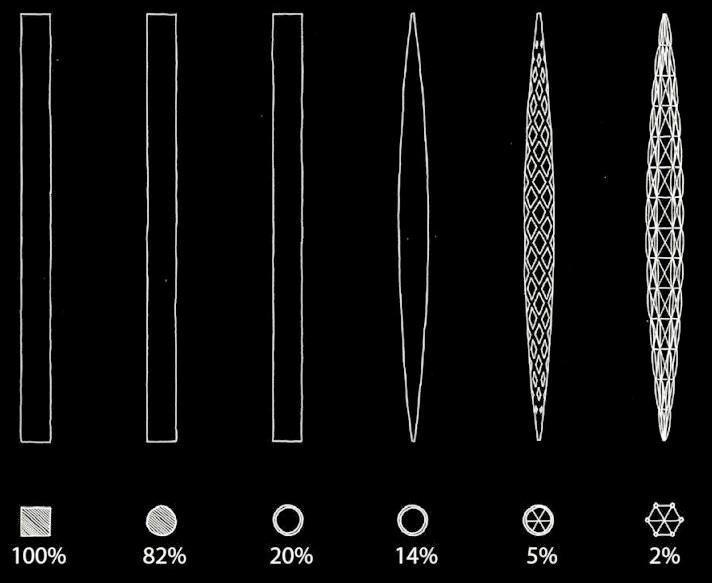
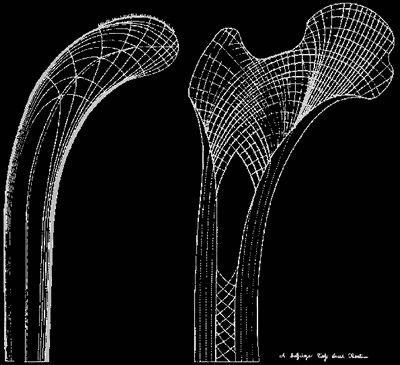
Cell Regulation
Minimal pathing systems for force distribution
Uniformly distributed loads
Fixed Points
SKO (soft kill option) The biological way to find an optimum structure topology, C.Mattheck.
LEVELS OF HIERARCHY
The use of mass and void in the structure Nature organises structure and materials together through hierarchy.
MATERIAL EFFICIENCY
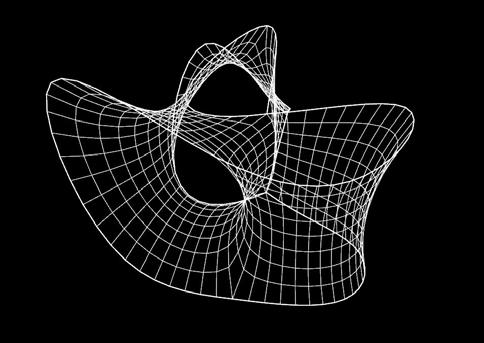
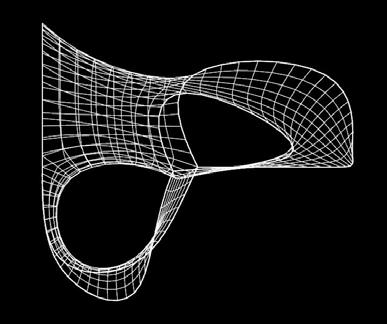
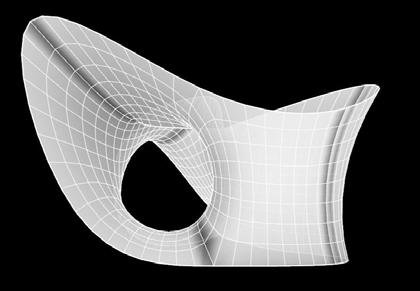
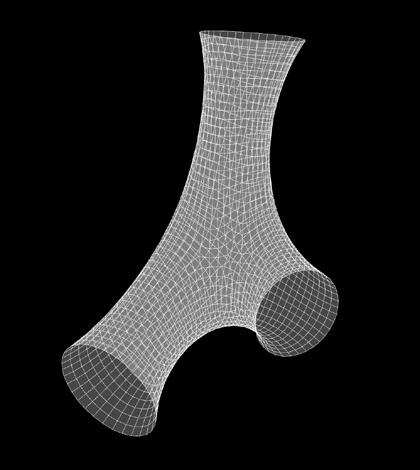
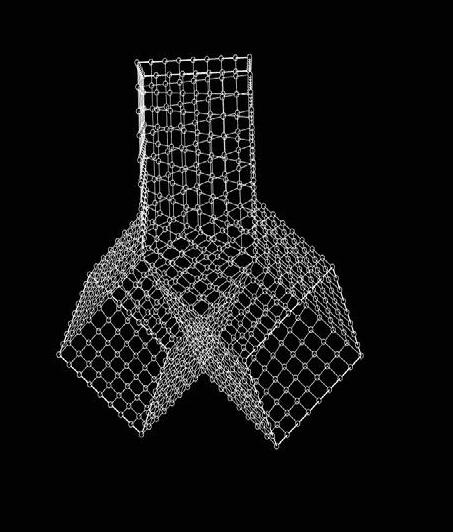
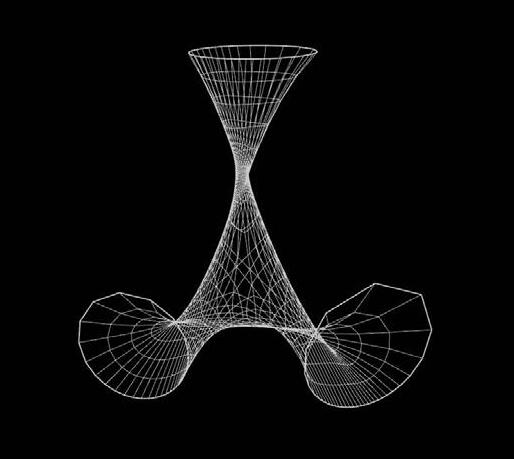

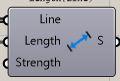
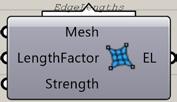
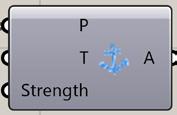
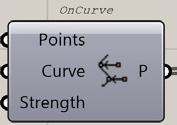

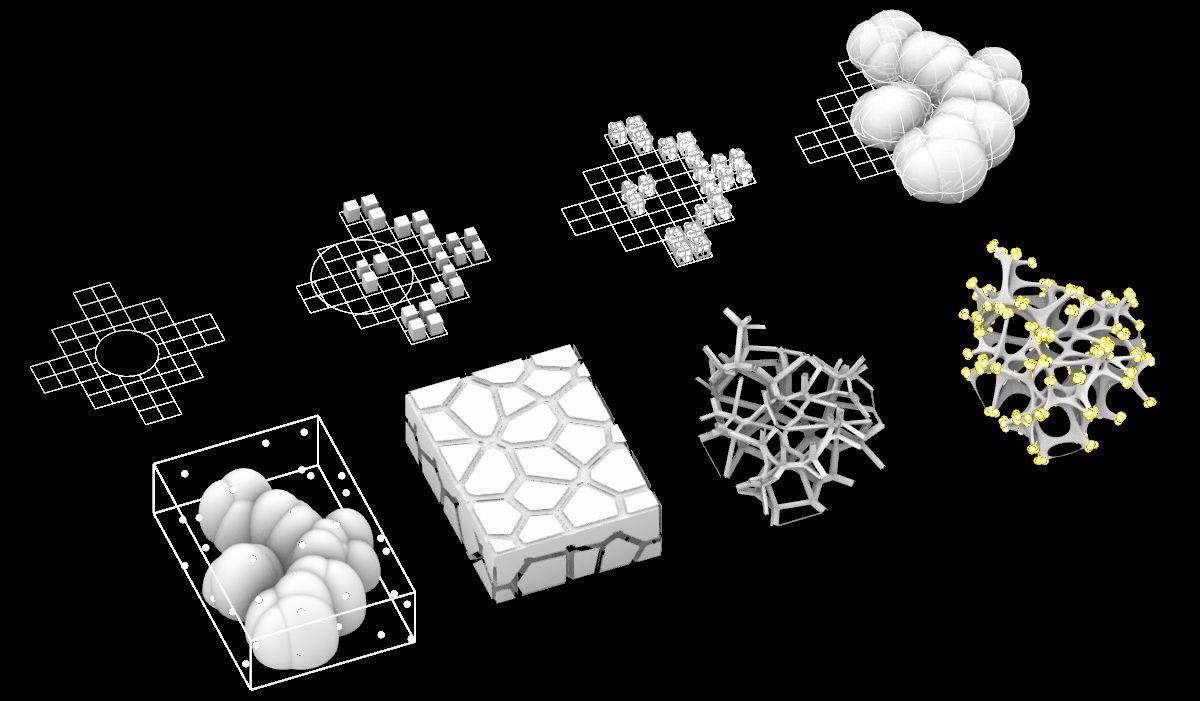
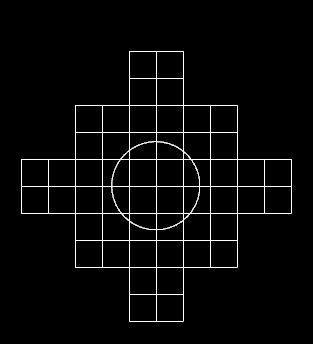
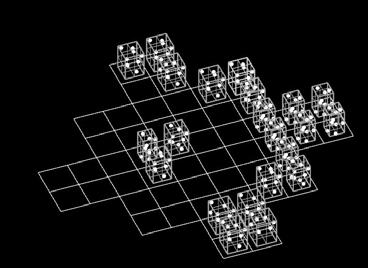
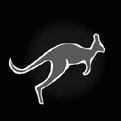
MATERIAL - CRYSTALLIZATION OF SALT
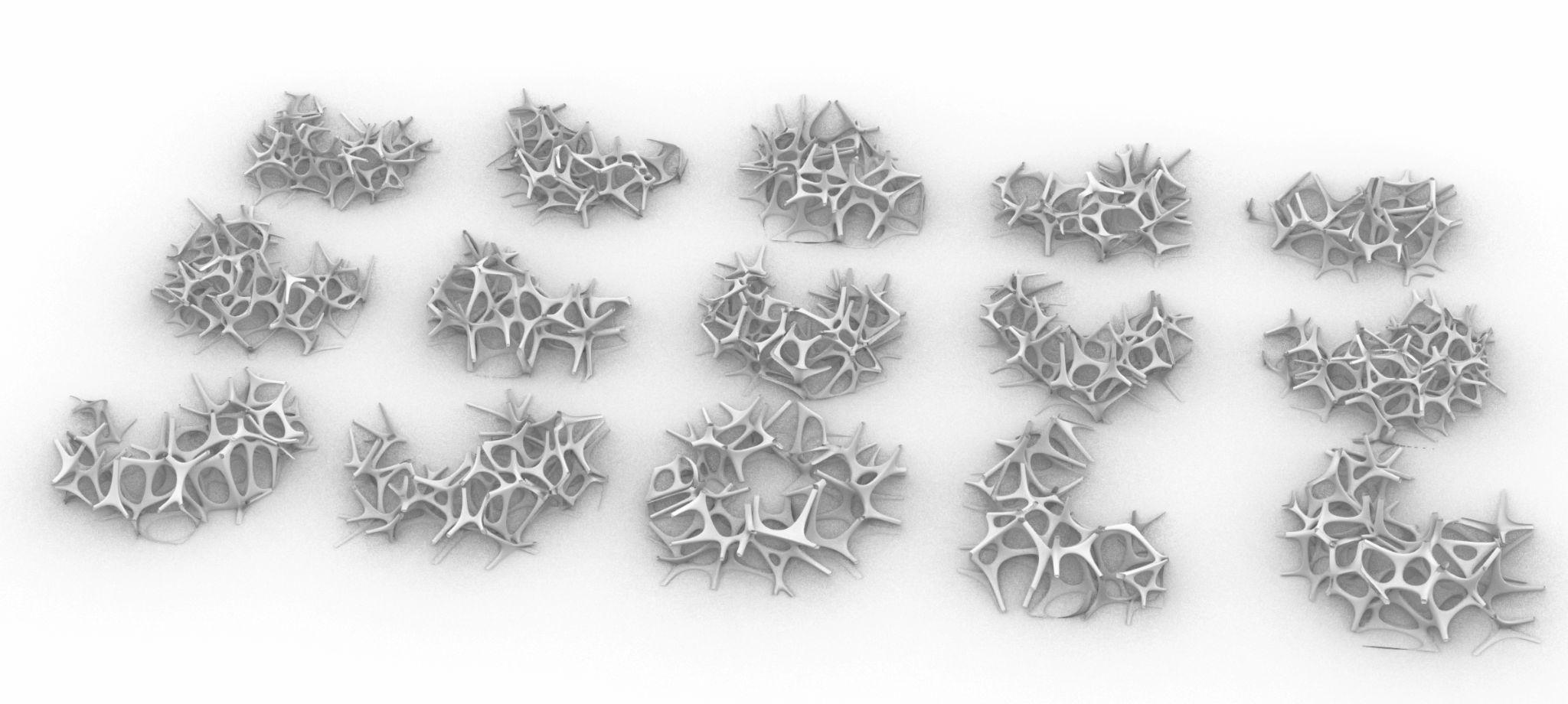
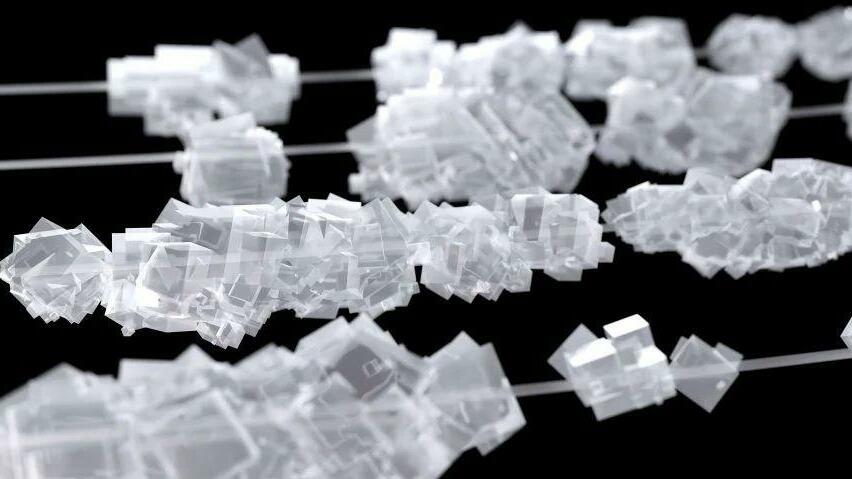
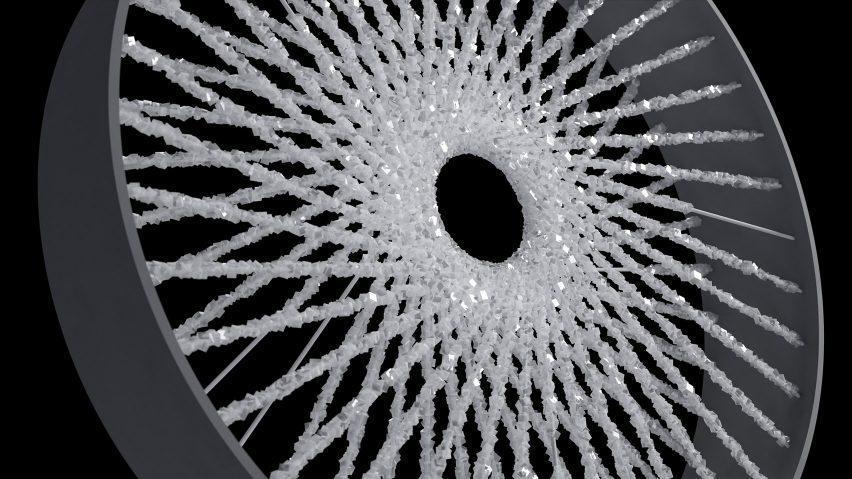
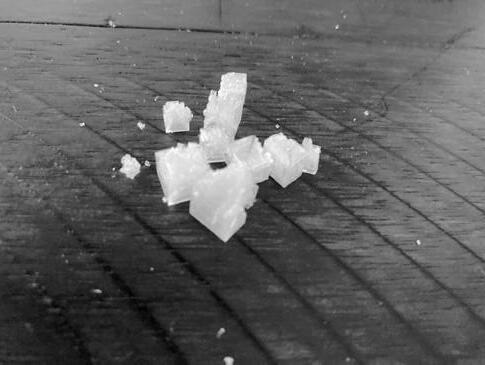
Experiment: testing experiment for crystallization of salt on a rope in a saturated salt solution with/without methanol
EXPERIMENT 01
DAY 1
0 hours. Kite rope is suspended in a vase containing a mix of super saturated NaCl solution and Methanol
EXPERIMENT 02
DAY 1
0 hours. Netted bag is suspended in a vase containing a mix of super saturated NaCl solution and Methanol
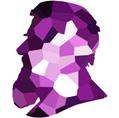
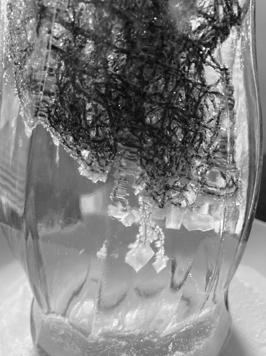
DAY 2 12 hours. Rate of crystallization is greatest in the first 12 hours of the experiment.
40 hours. Salt crystals continue to form at a slower rate.
DAY 2 24 hours. Rate of crystallization is greatest in the first hours of the experiment.
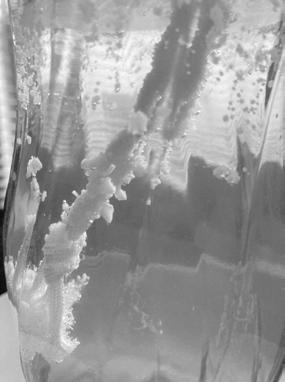
OPTIMISATION
PARAMETERS
Location of Courtyard Number of Interior spaces Size of Courtyard
DAY 3 Resulting crystals
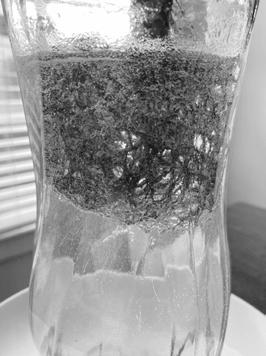
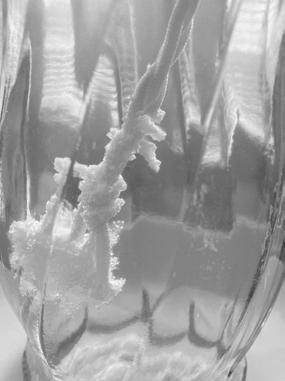
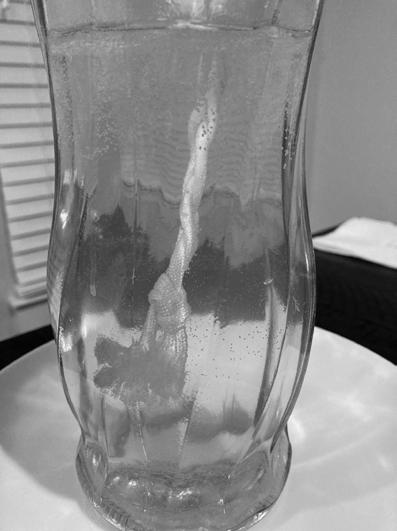
SALT PAVILION
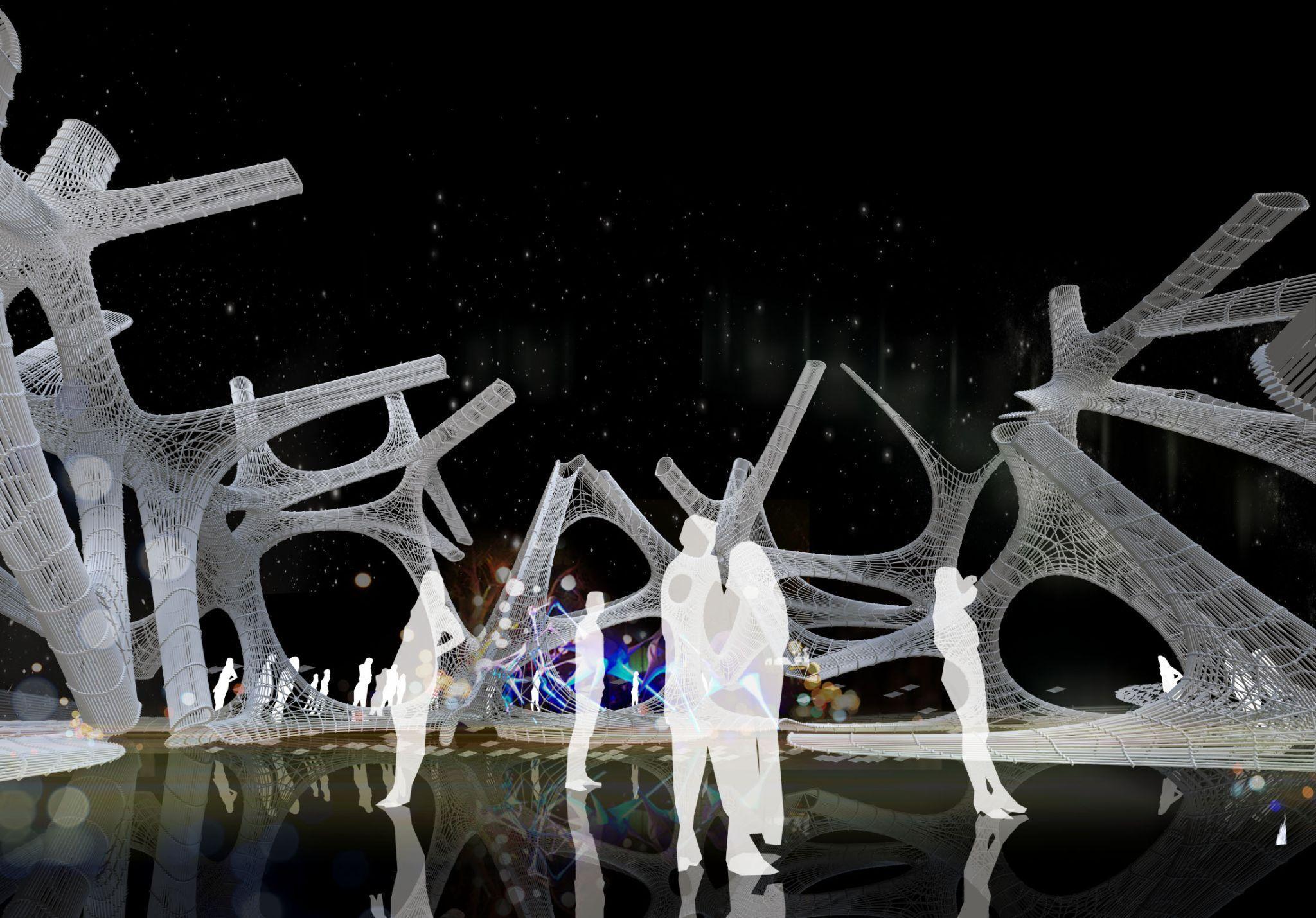
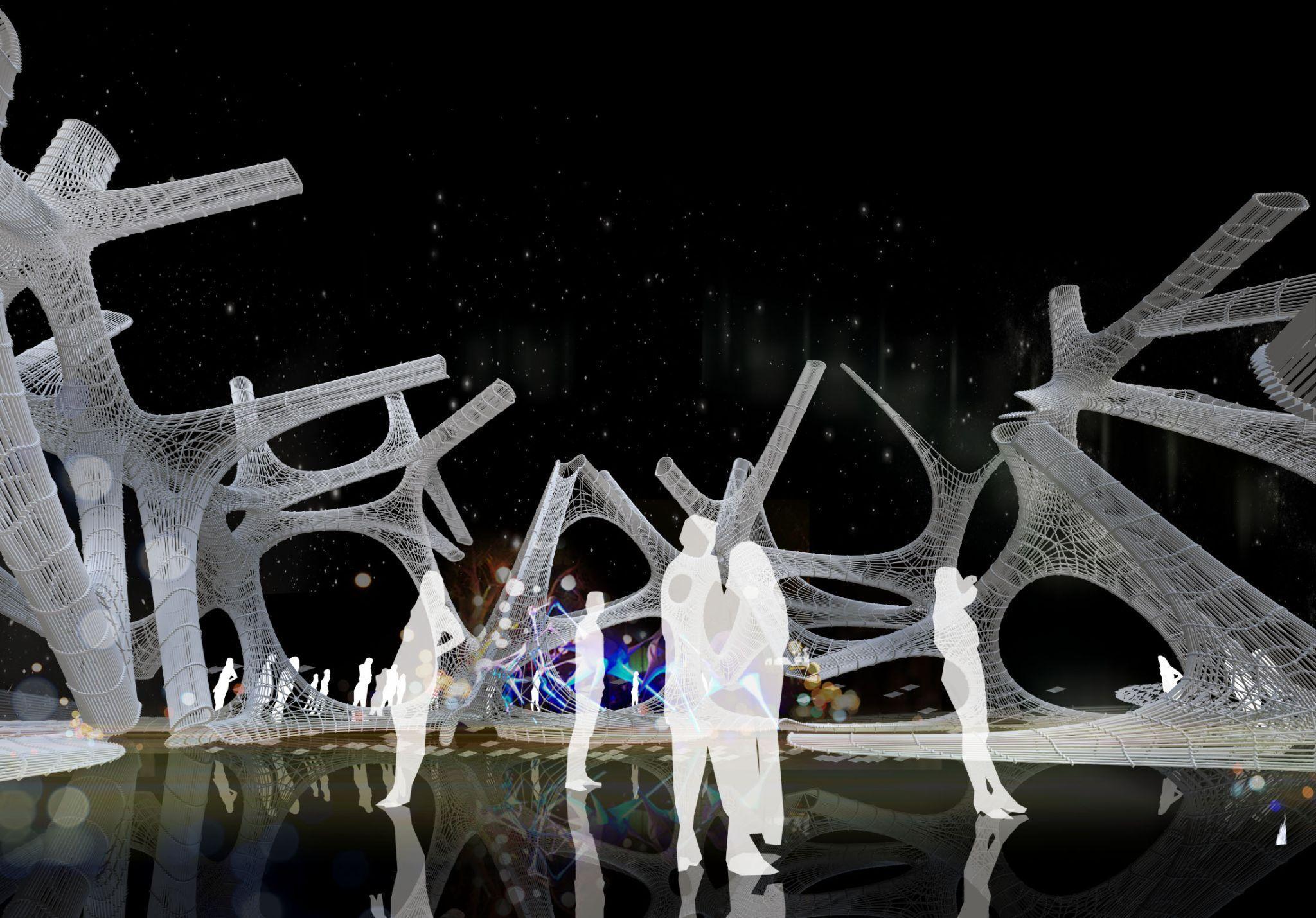
SALT PAVILION
NORTH FACADE
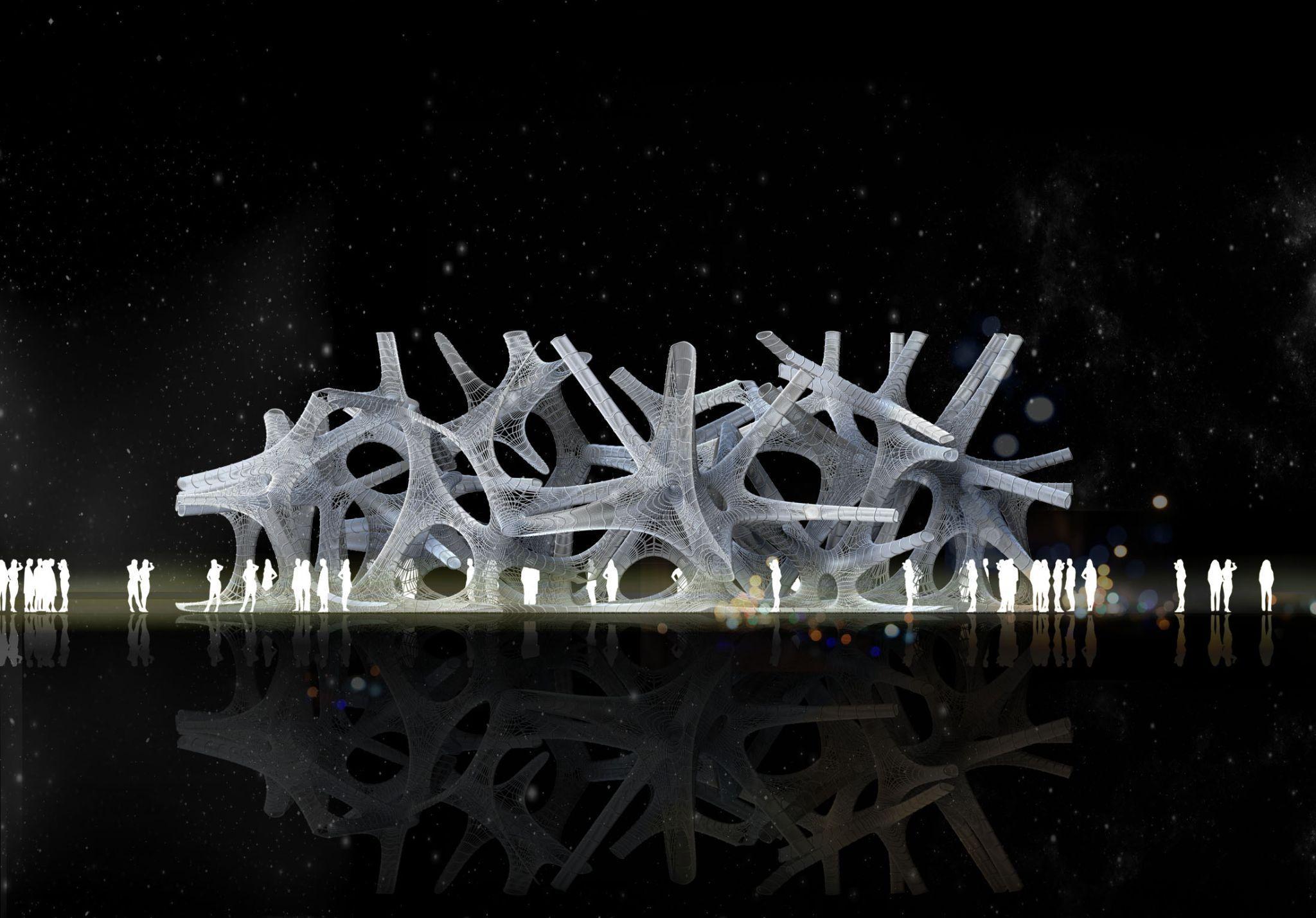
OPTIMISED ITERATION
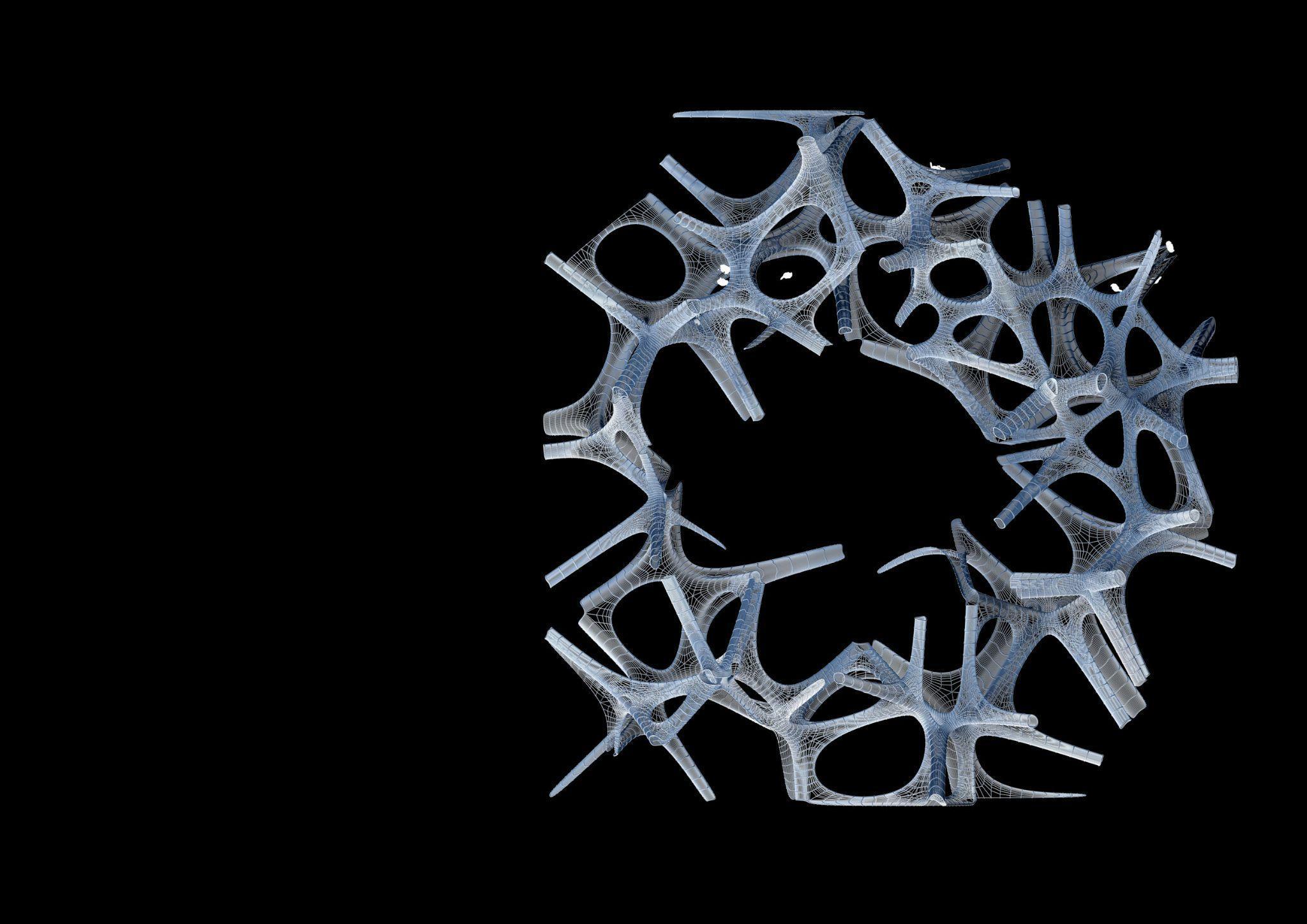
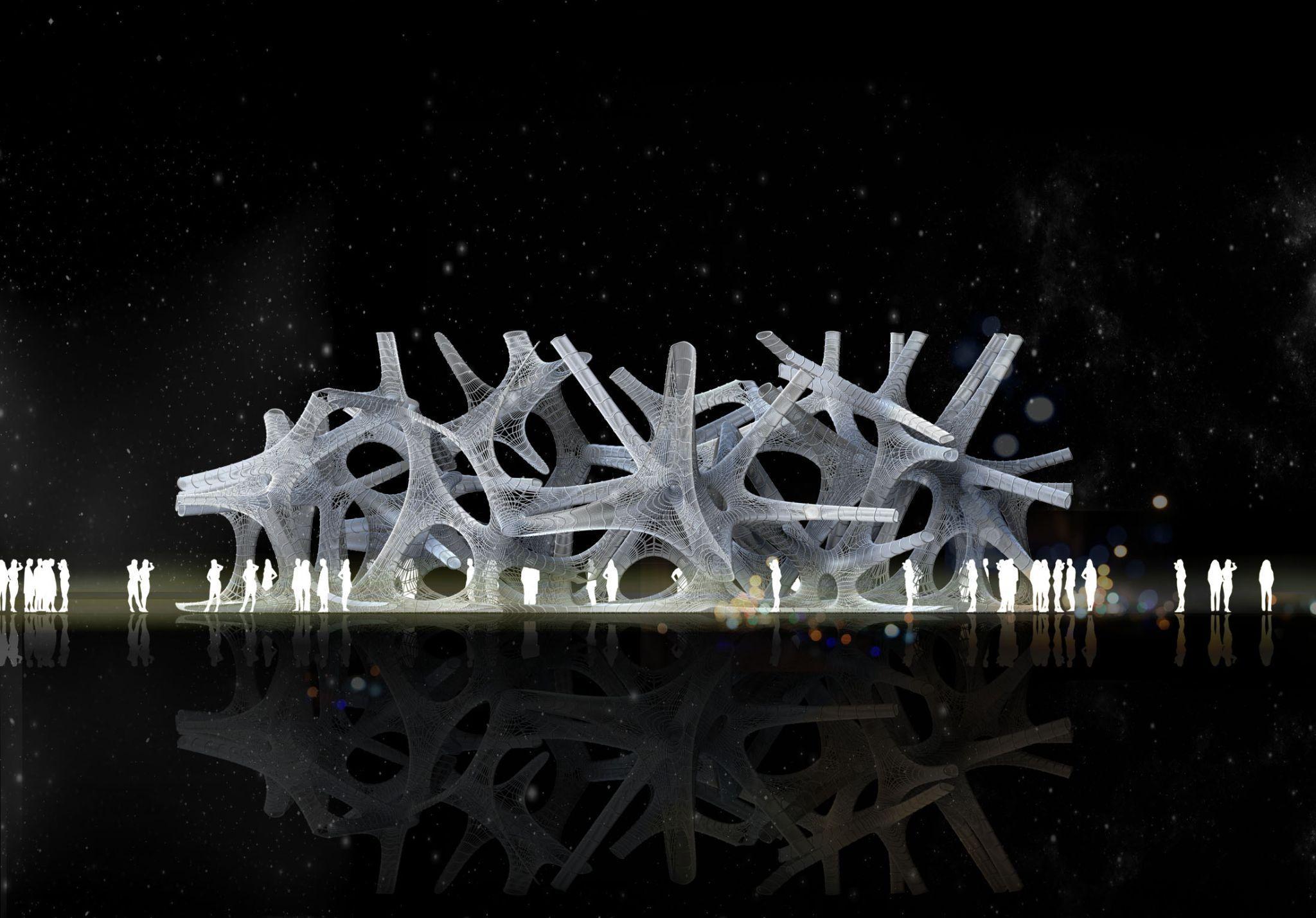
ESPEJO
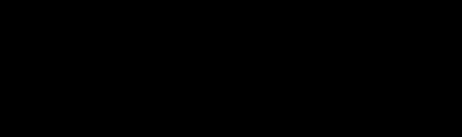
08 Date: 2021
Location: Salar de Uyuni, Bolivia
Collaborators: Abed Badran, Amanda Gioia, Ara Keuroghlian, Jumana Hamdani, Rodrigo Alvarez
Instructor: Arthur Mamou-Mani
Project Type: Academic at IAAC
Tools: Rhino, Grasshopper including plugins: Karamba 3D, Wallacei, Weaverbird, Pufferfish, Crystallon, Droid, Anemone, 3D Max, Photoshop

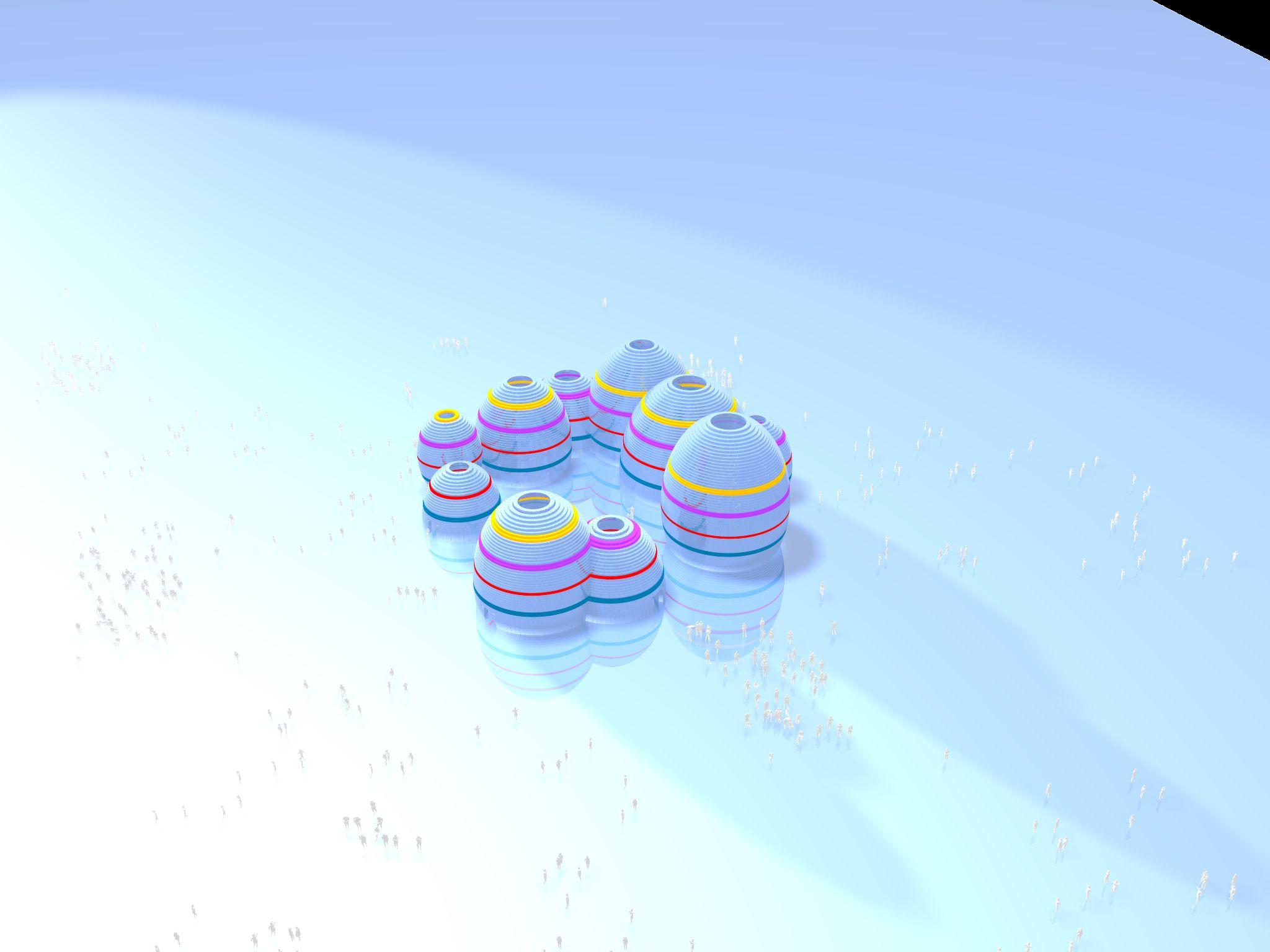
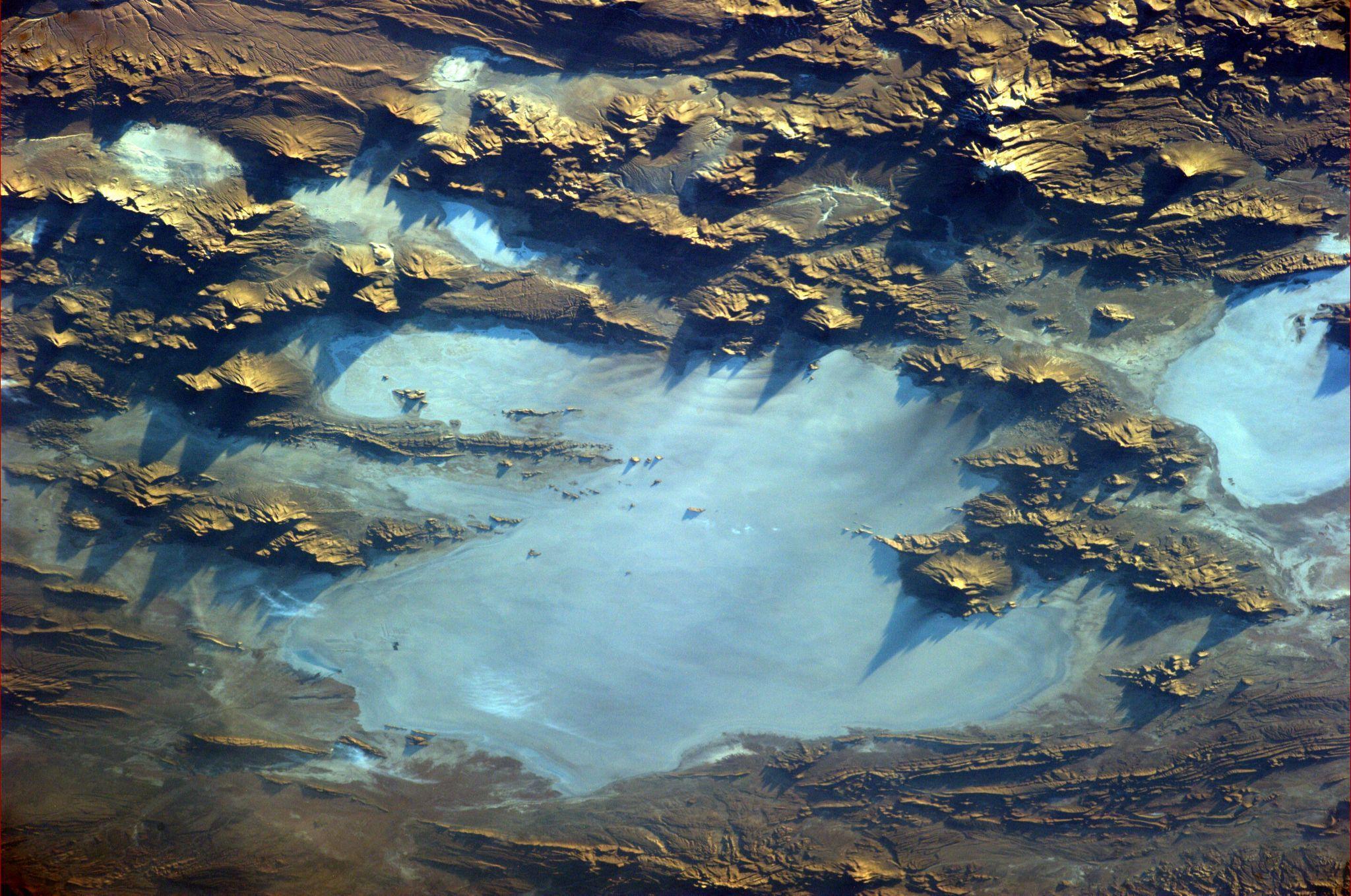


POTENTIAL SITE
The New City Location
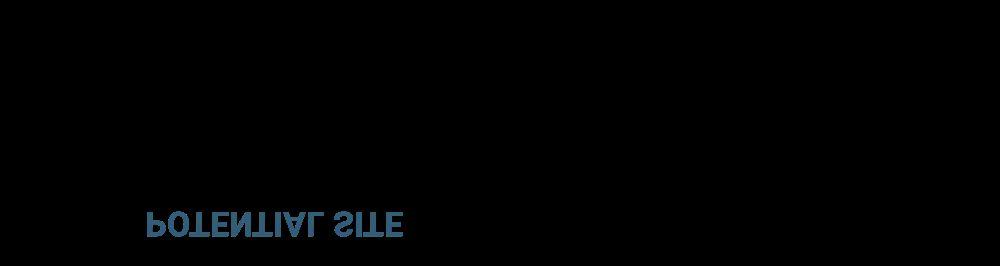
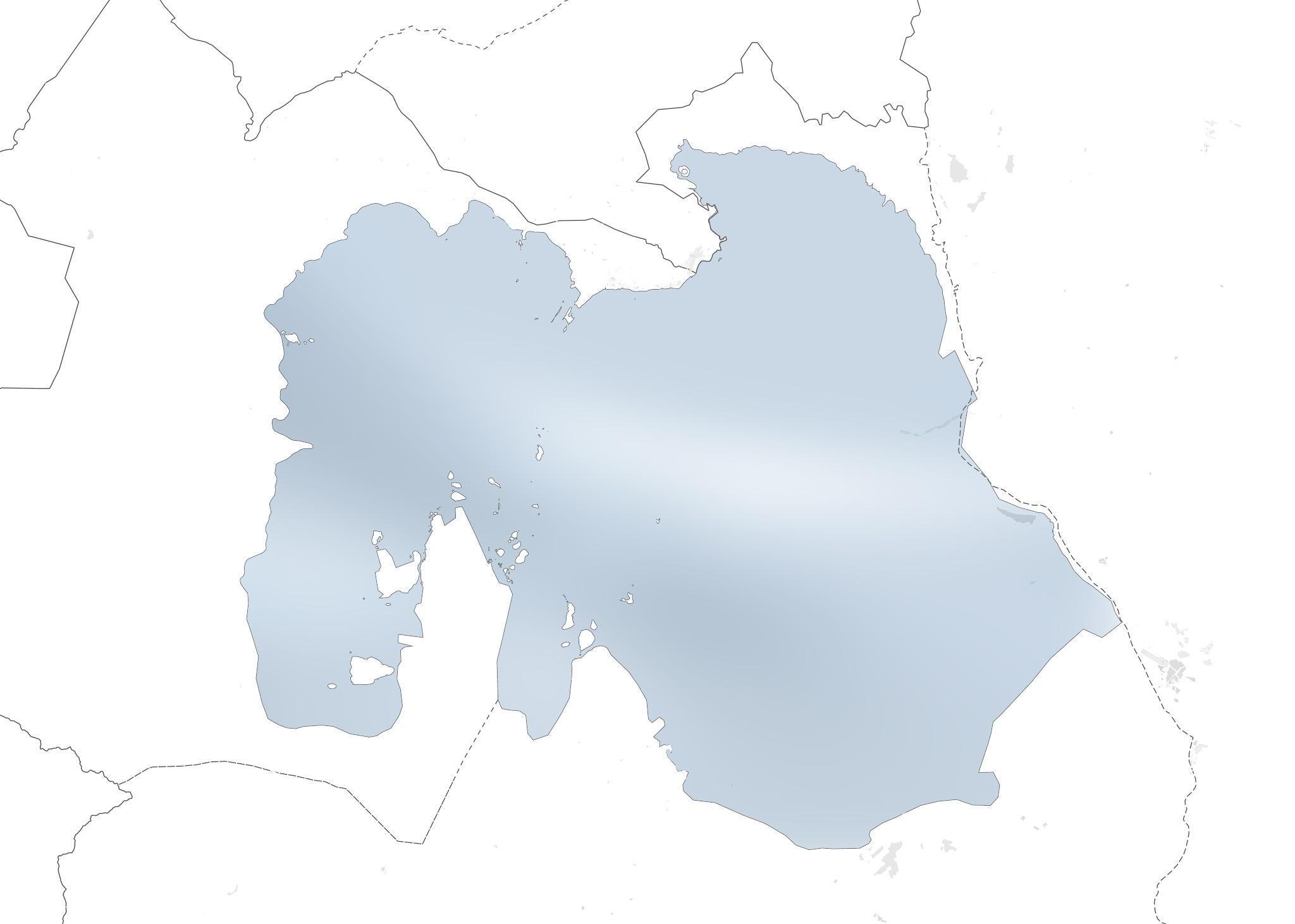
Chacoma Bella Vista
CHIL E
Salar de Coipasa Salar de Empexa 0 50 km N
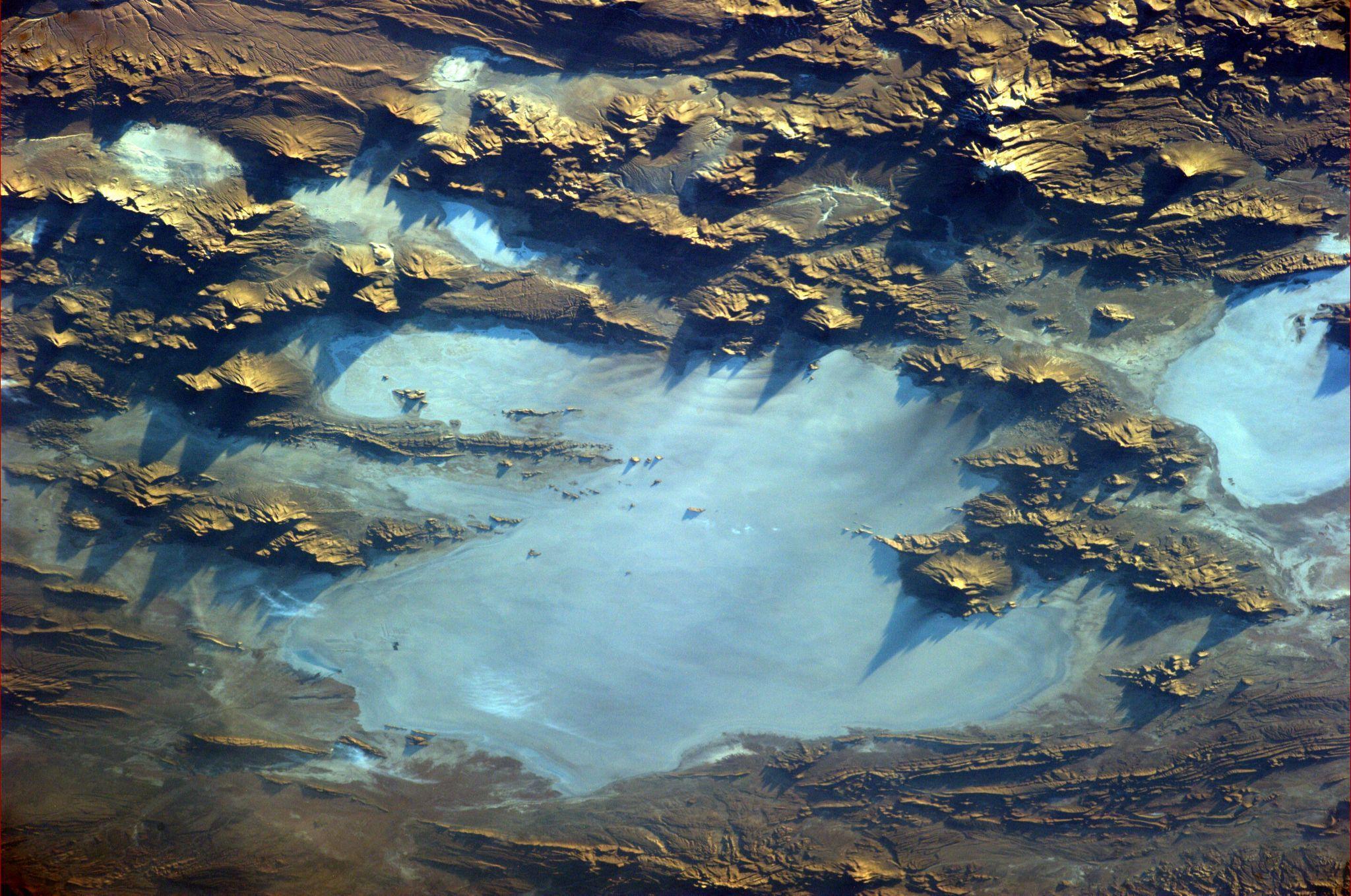
Hizo Tres Cruces Salinas de Garci-Mendoz a
Lavaxa
POTOSI
Tonavi Canquella
Caquena Cha’llacolla
Tahua BOLIVI A
Irpa Isla Pescado r Isla Incahuas i
Biodivers ity
Rio Mulatos Toja
Llica Colchani
Biodivers ity Potential Site
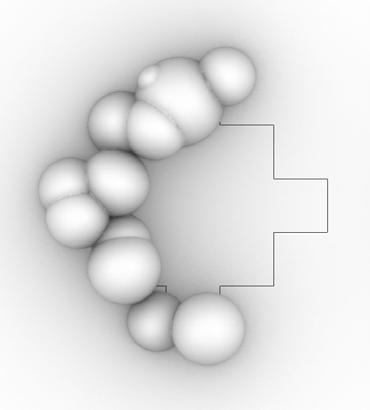
El Desierto Colcha K (Villa Martin)
San Pedro de Quemez
Coquesa
90km 66 km Uyuni Atulcha Chuvi ca
Salar de Uyuni Isla Casara de Huevo
Lithium Reserves
To San Juan (20km), Chiguana (40km), Laguna Colorada (175km)
Culture & Recreatio n
To Potosi (210km)
To Tupiza (225km), Villazon (320km)
To Avaroa (120km), Calma (245km)
The purpose of the ESPEJO project is to define a cultural event held annually in Uyuni, Bolivia that focuses on bringing awareness to the mining in Salar de Uyuni, and a transformative change through art, culture and both local and global community participation.
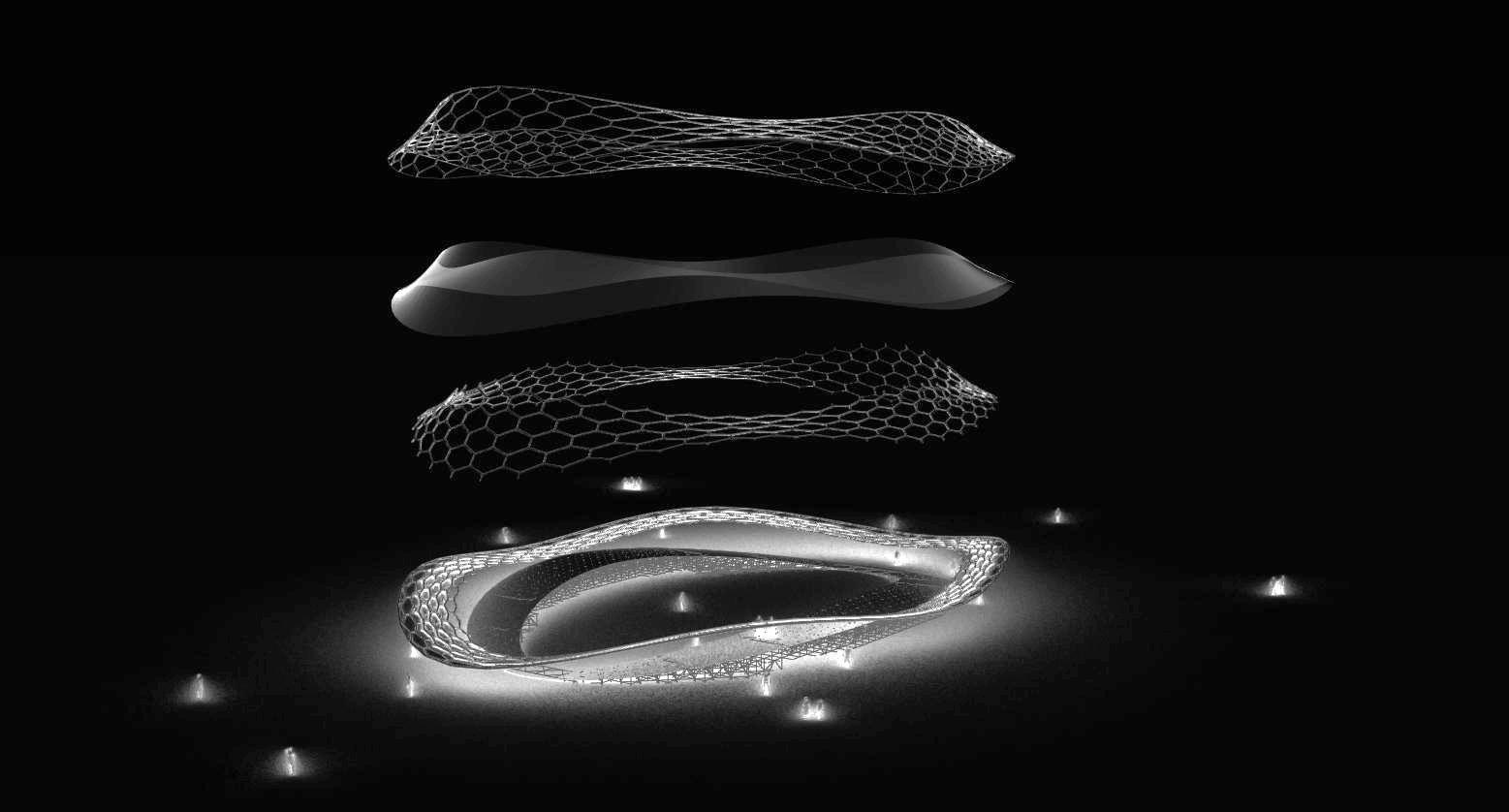
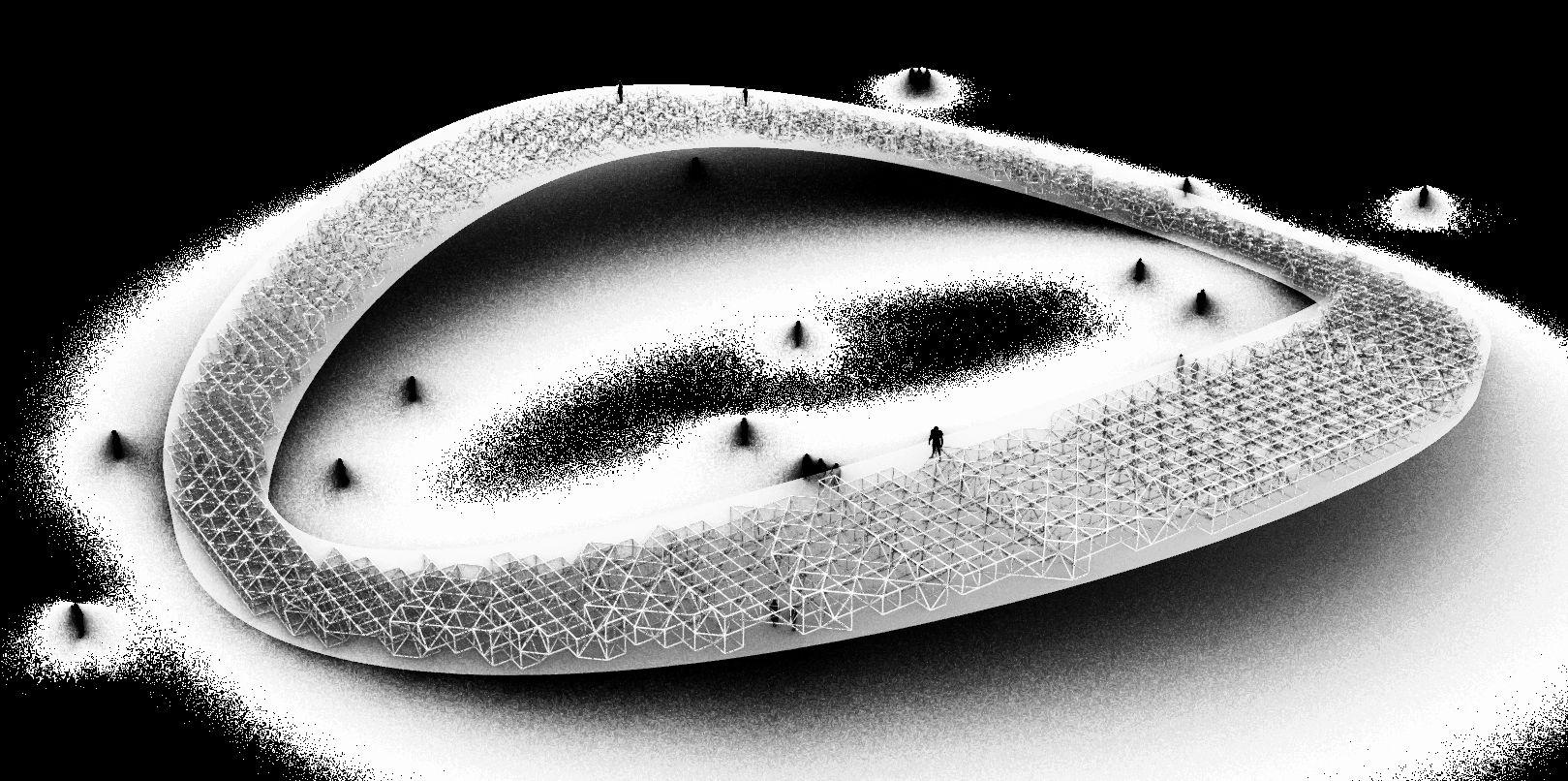
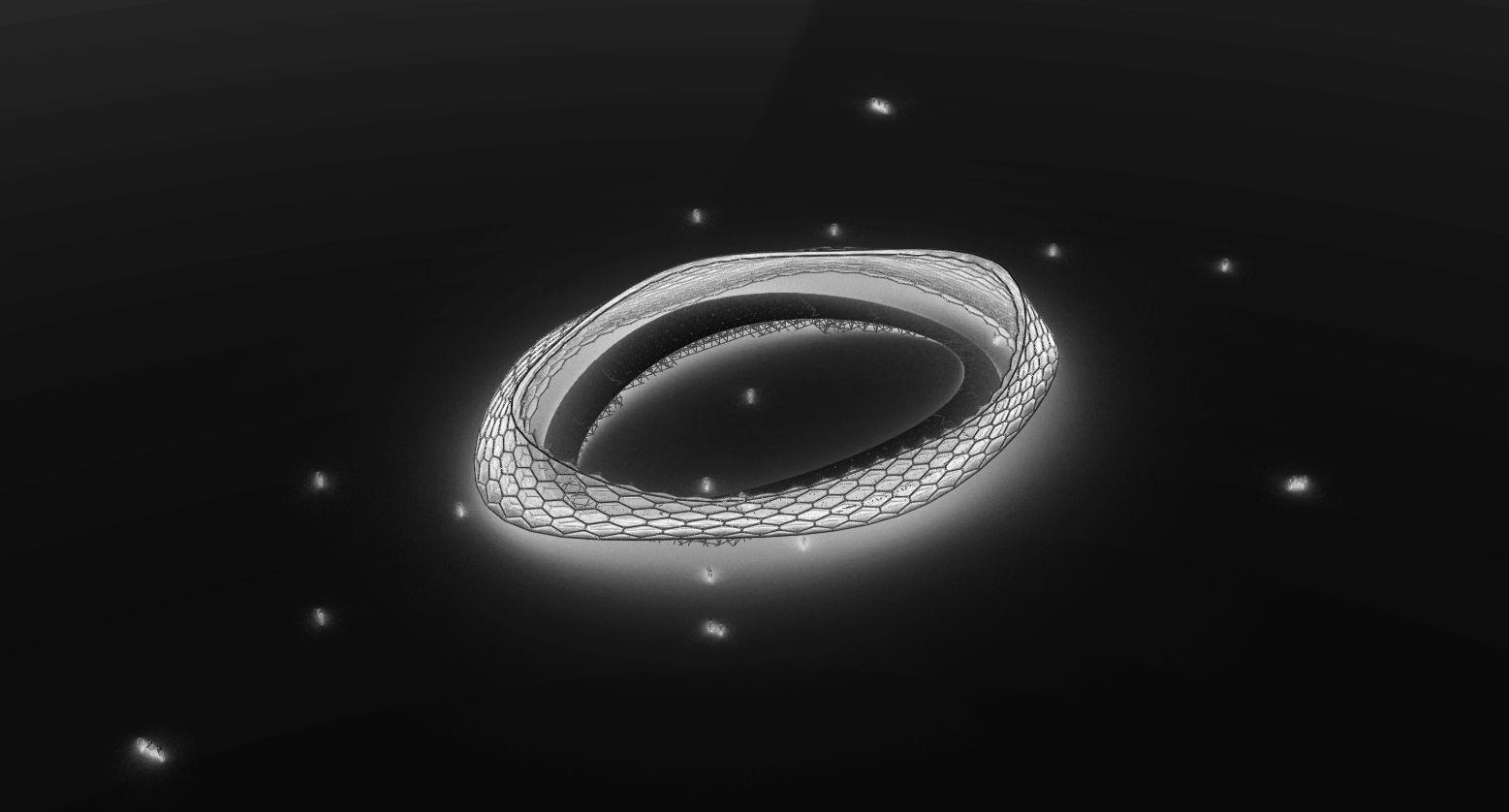
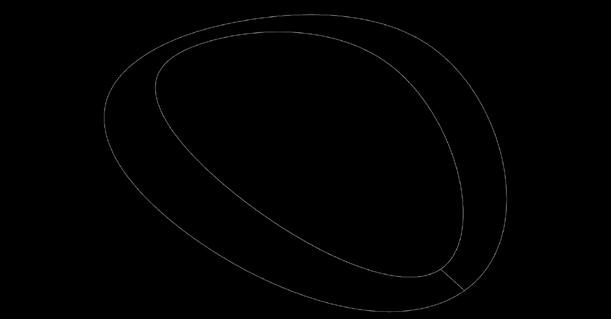
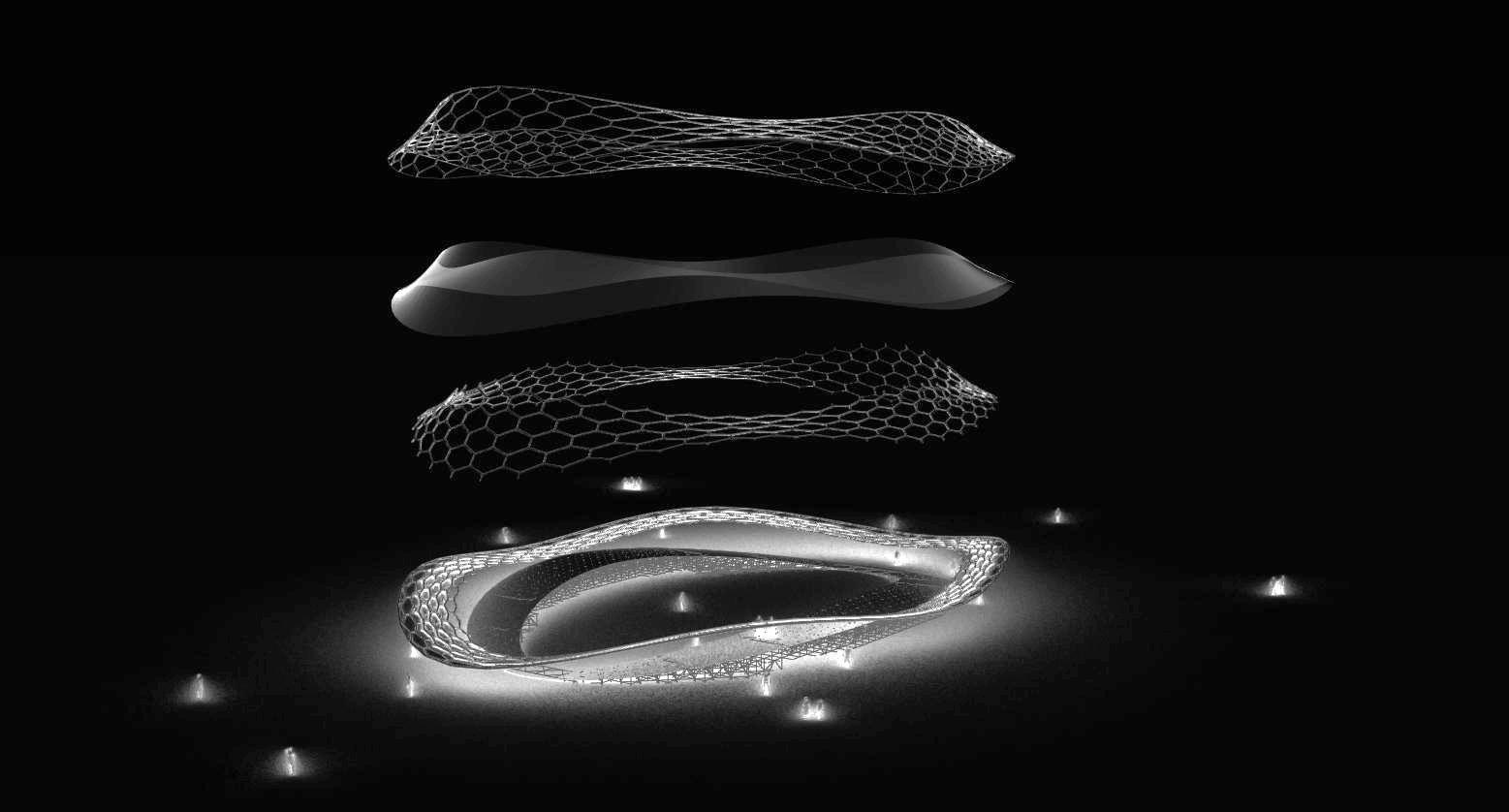
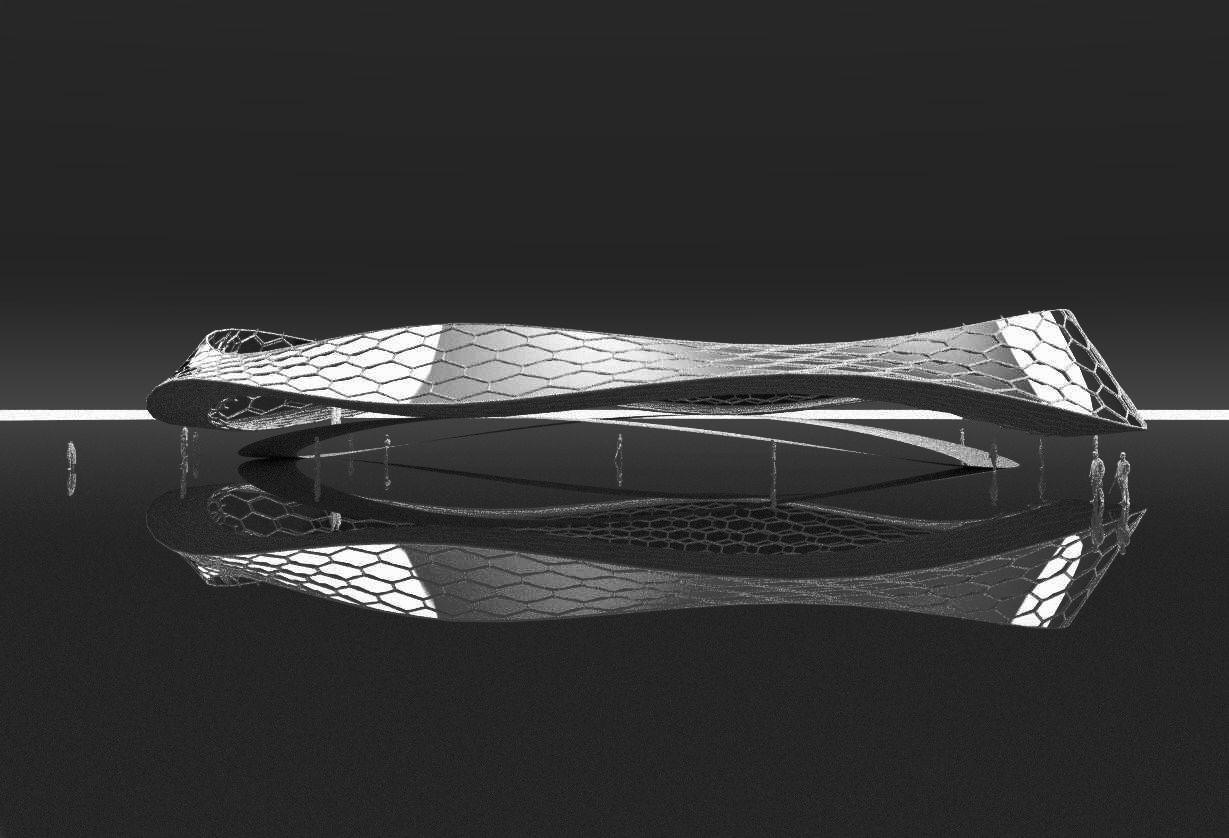
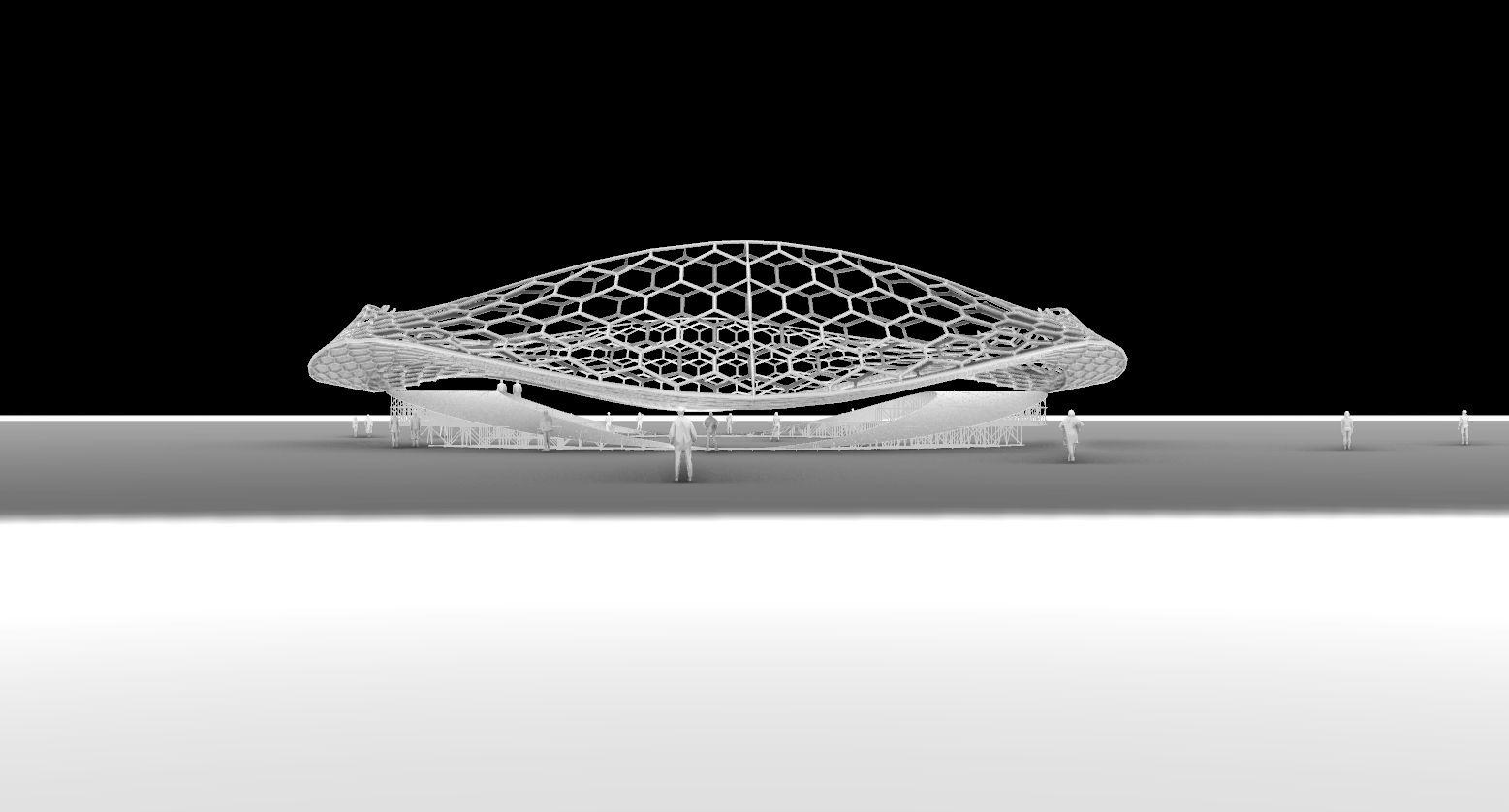
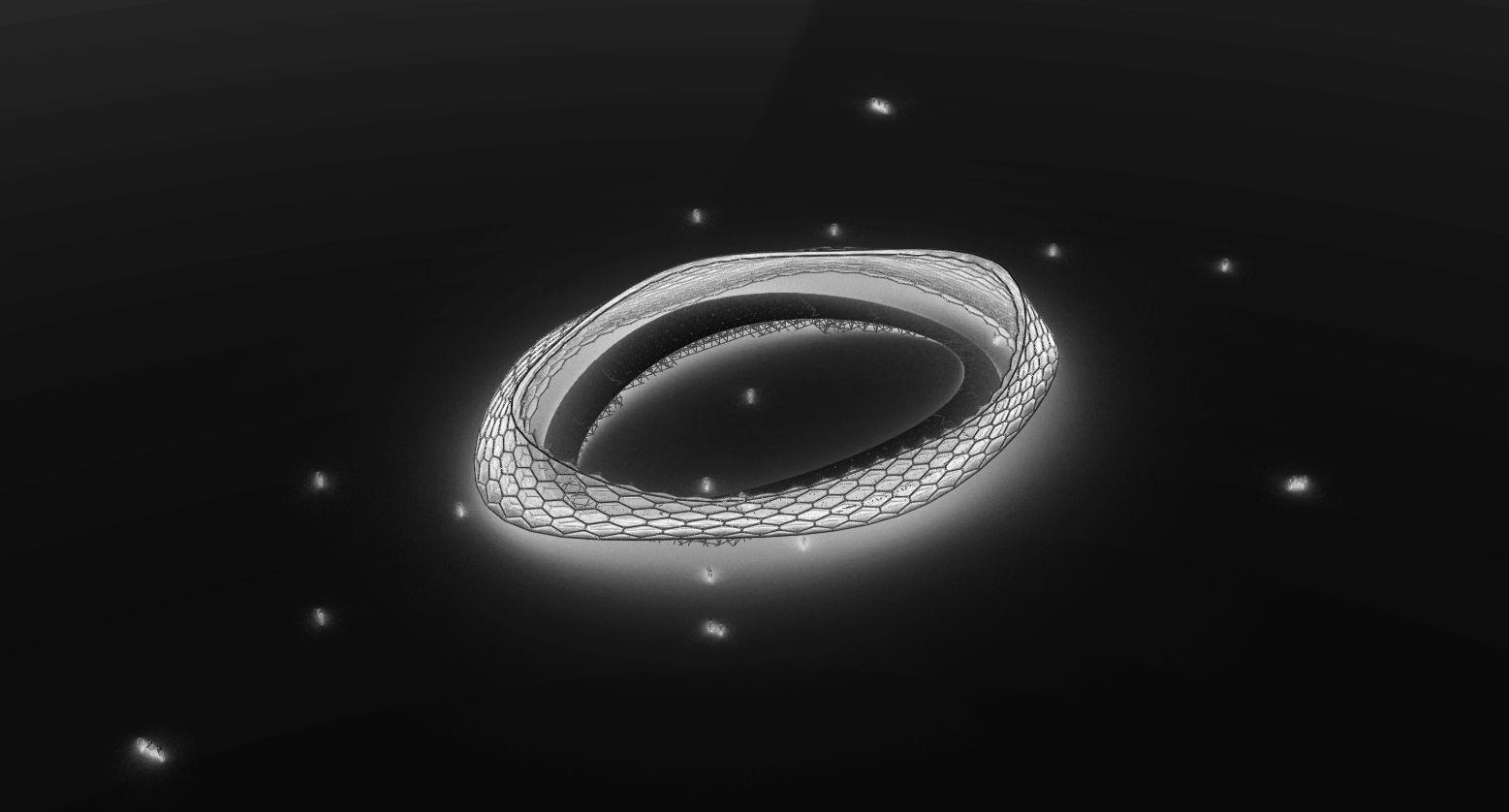


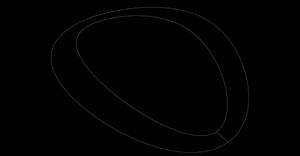
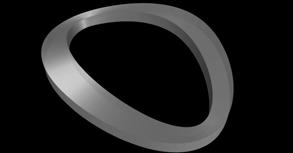




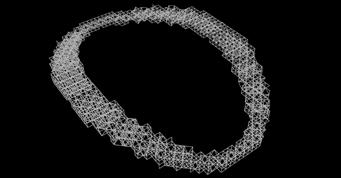


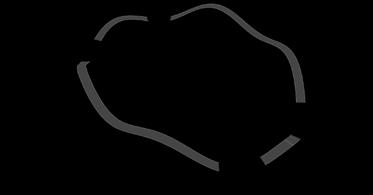



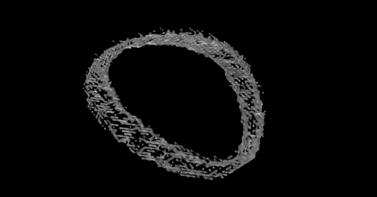
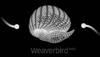




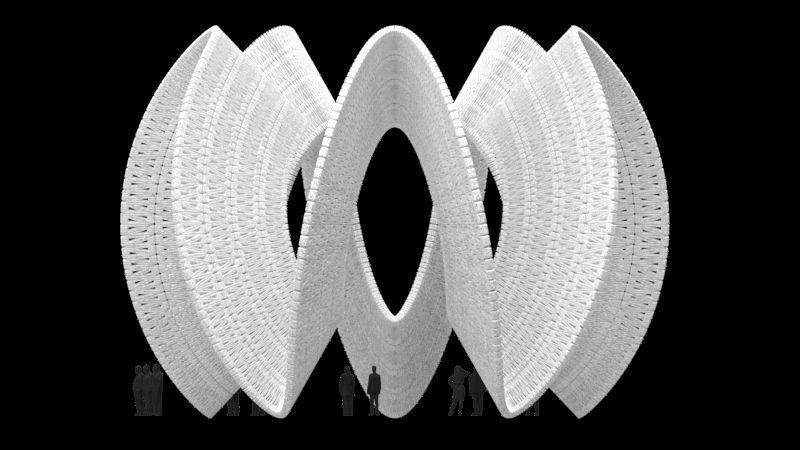
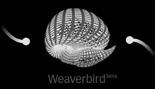
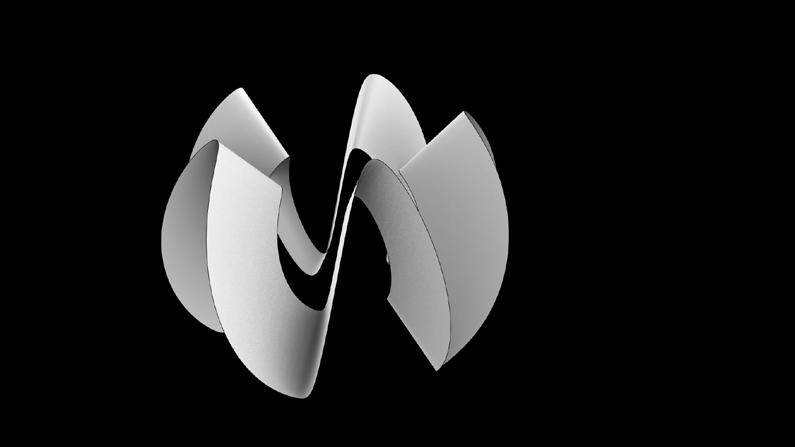
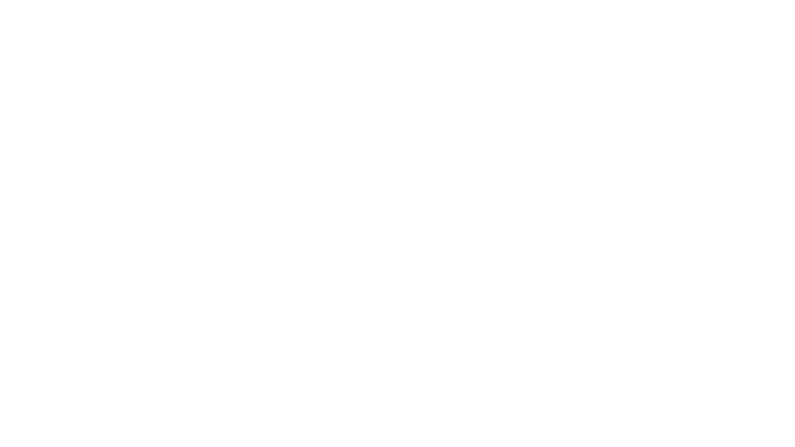
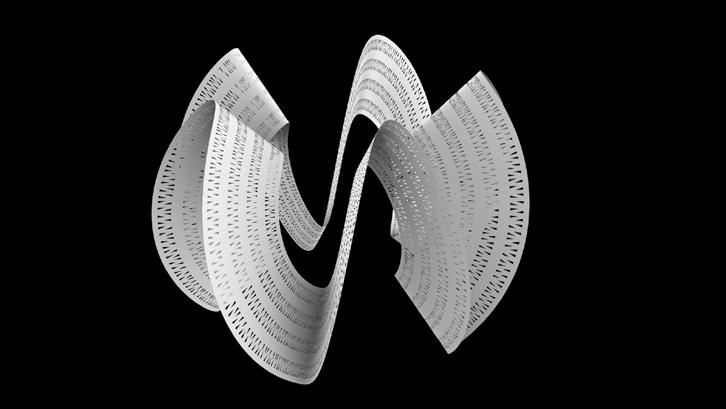
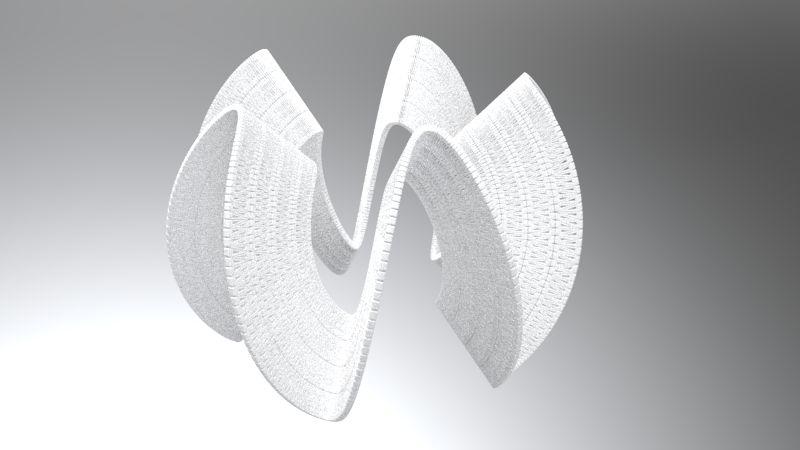
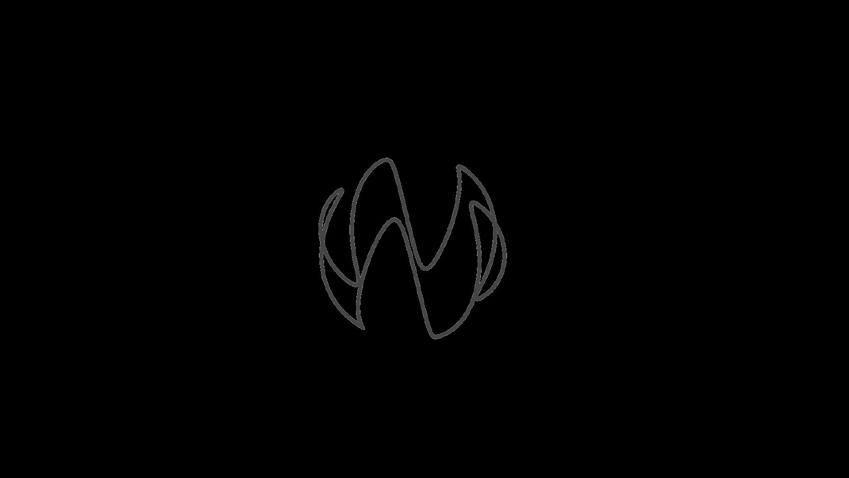

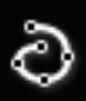








Exploring Brick Patterns Salt Blocks
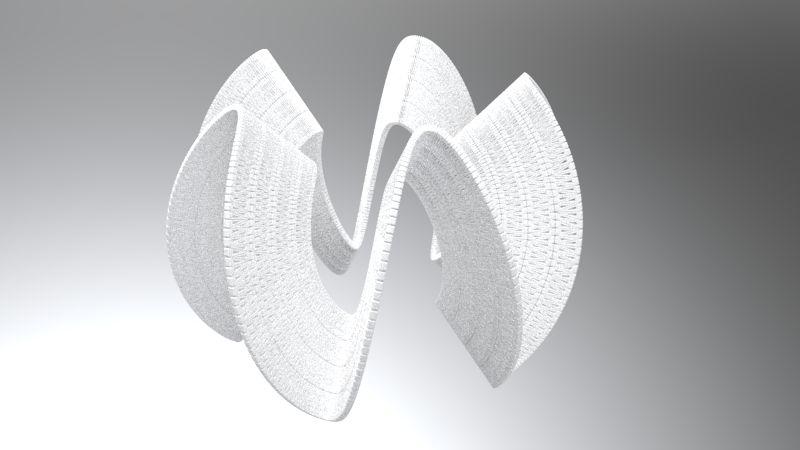
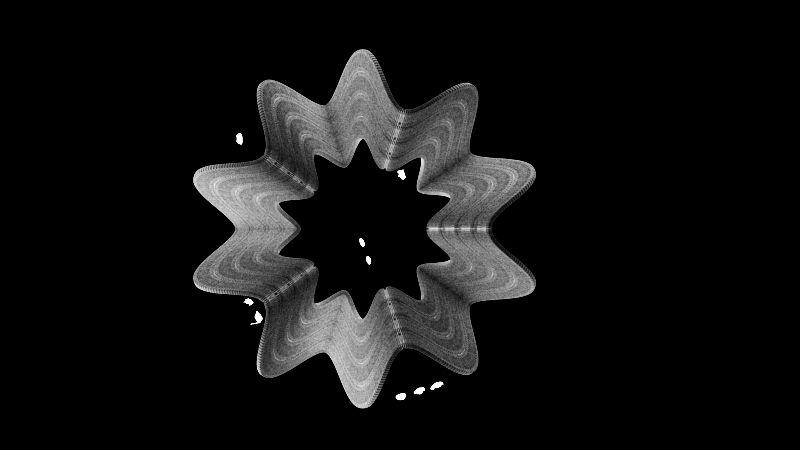
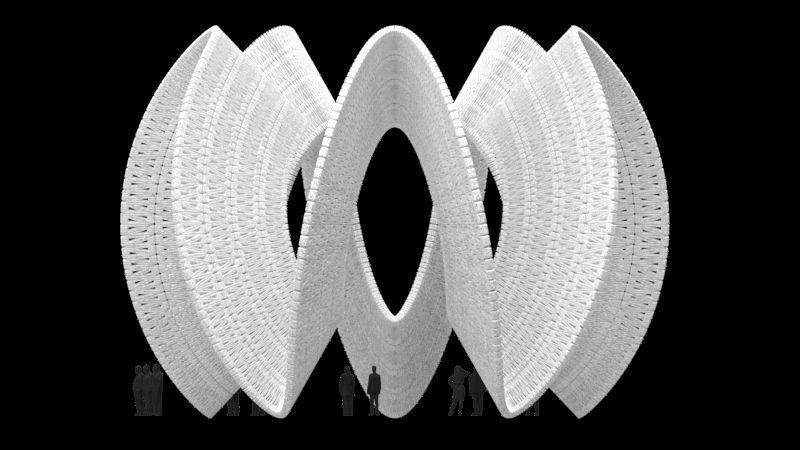
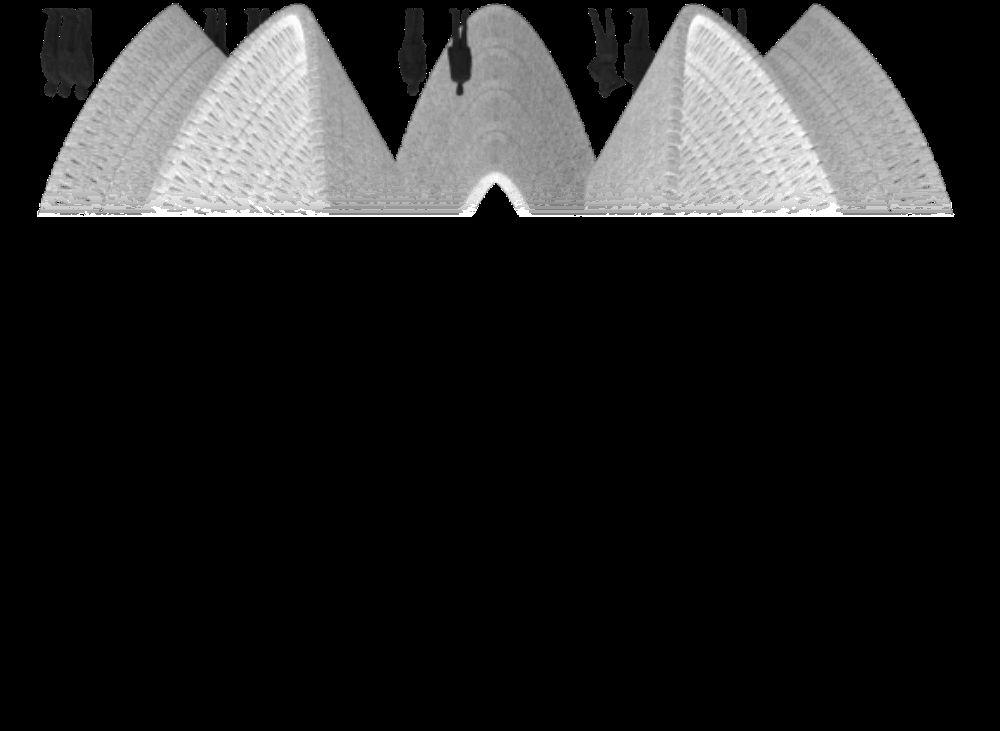
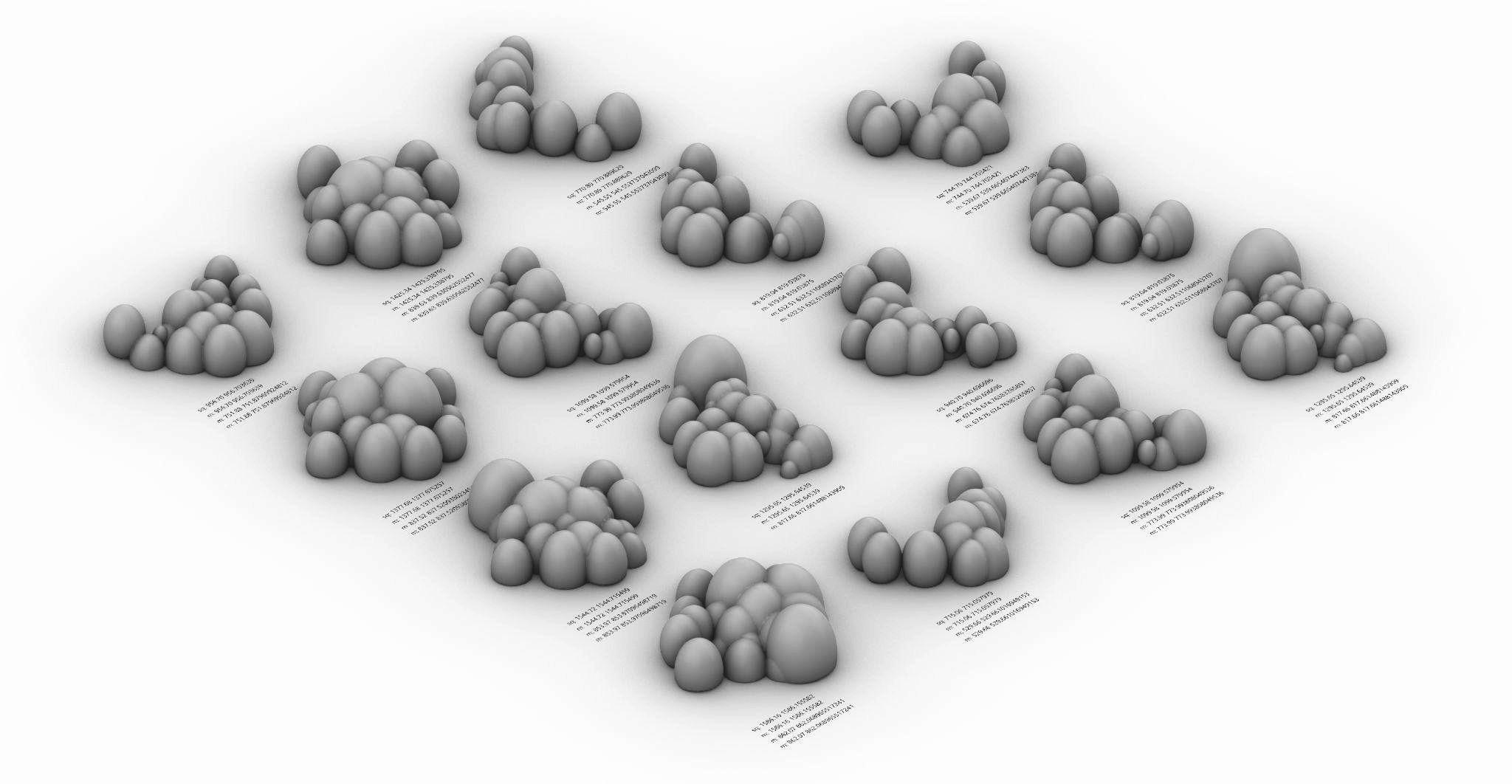
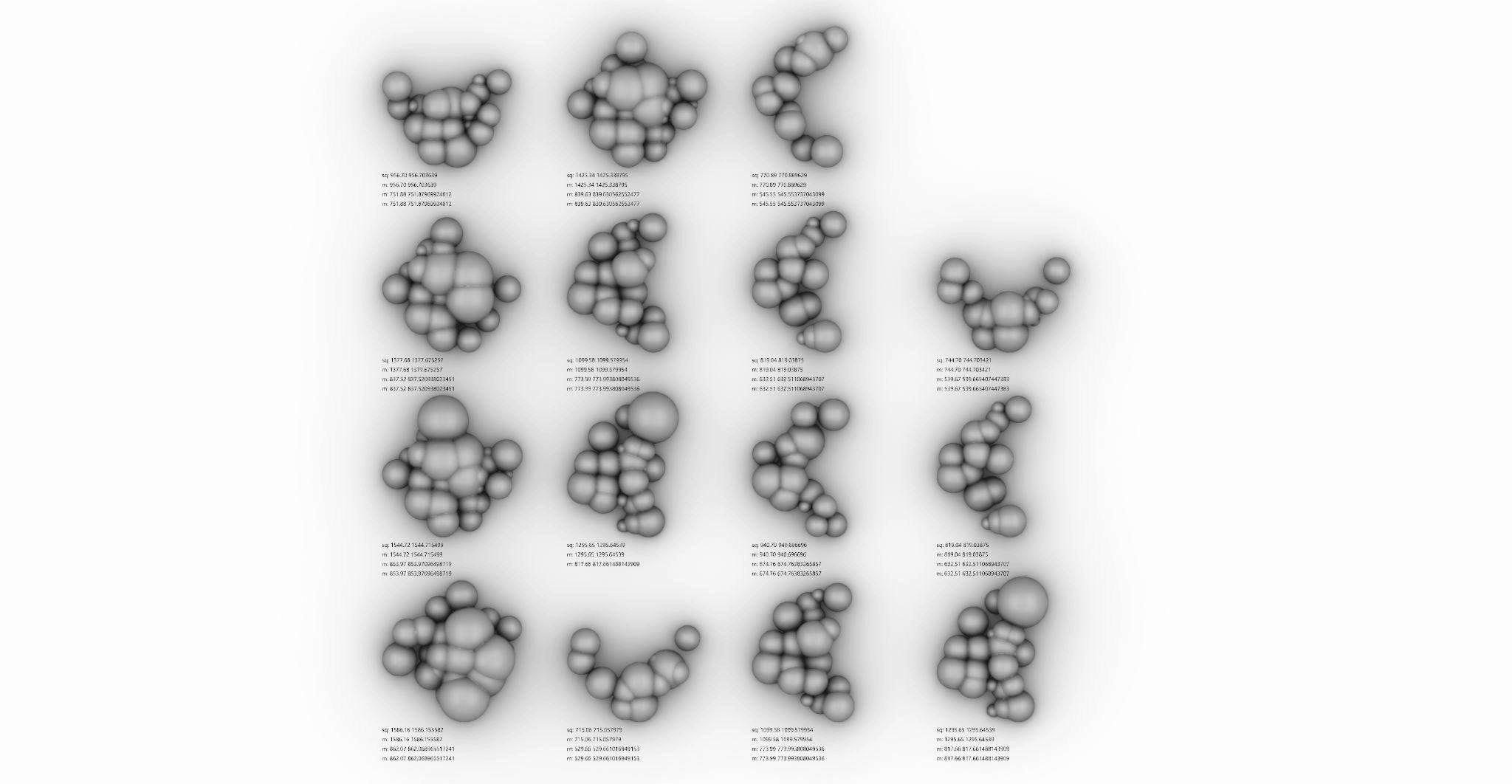
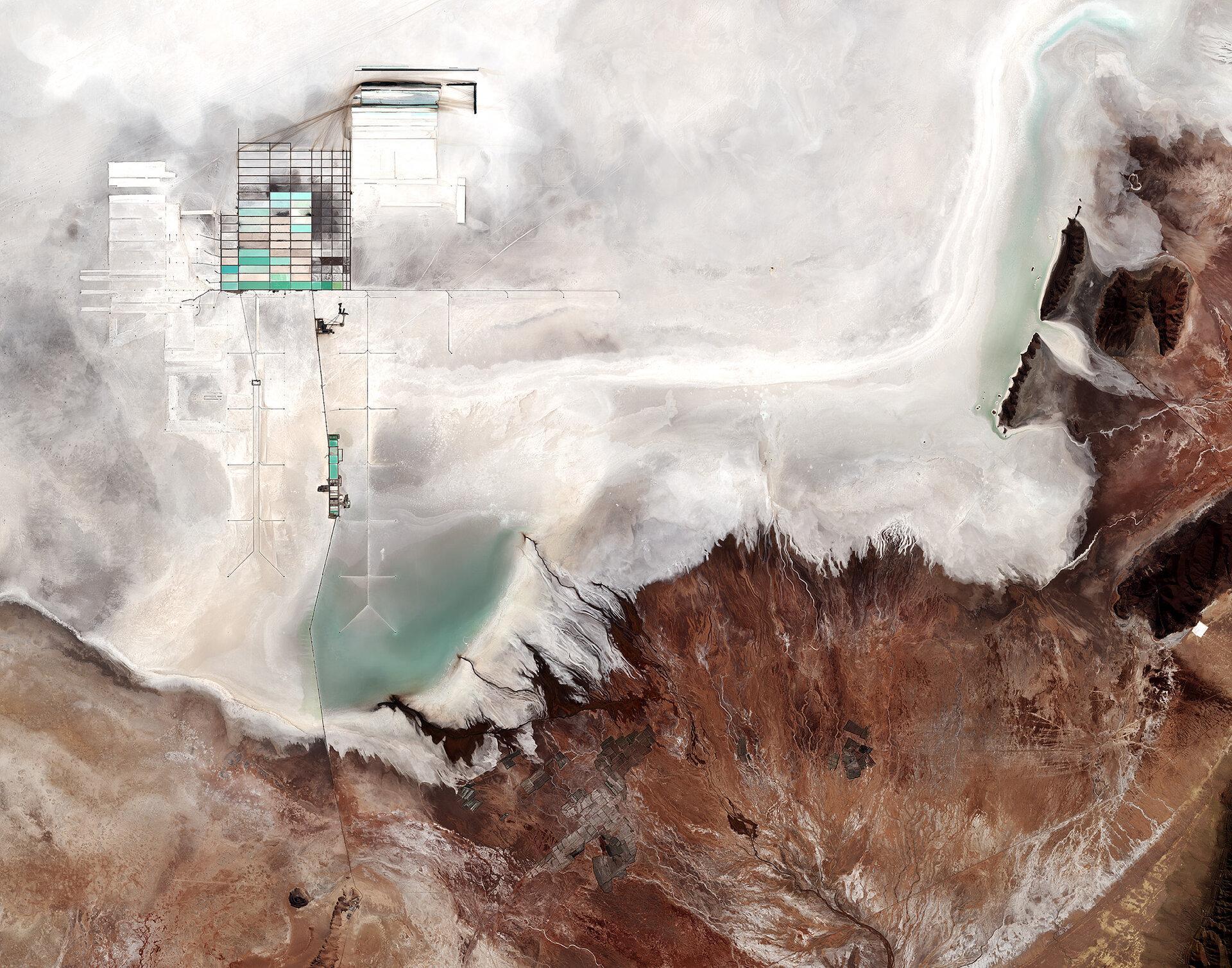
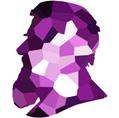
Lithium Reserves
Measuring about 10km2 and situated at an altitude of 3,600 meters. The crust is several meters thick and lies over a salty lake.
Beneath the salt lies the world’s greatest Lithium reserves.
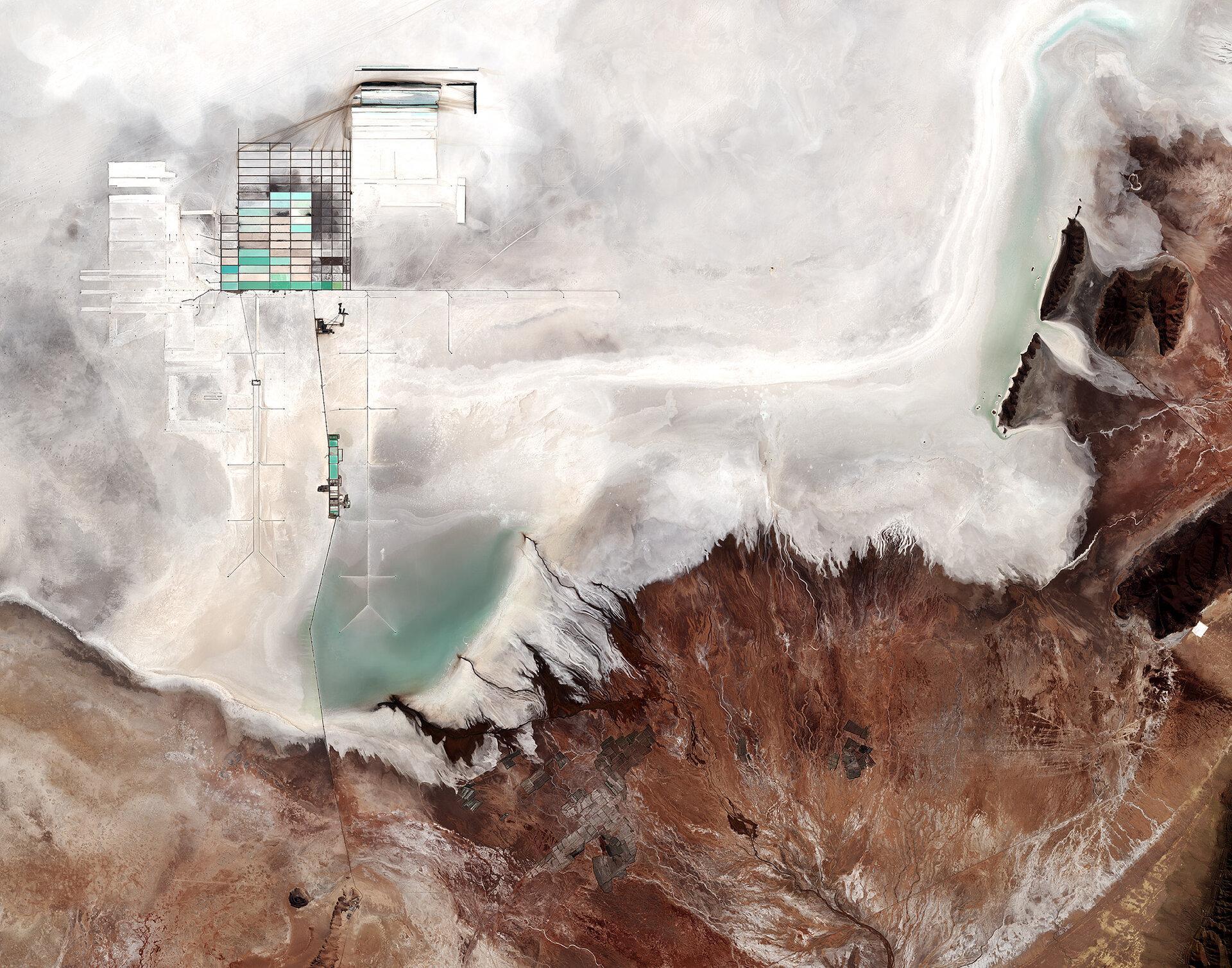
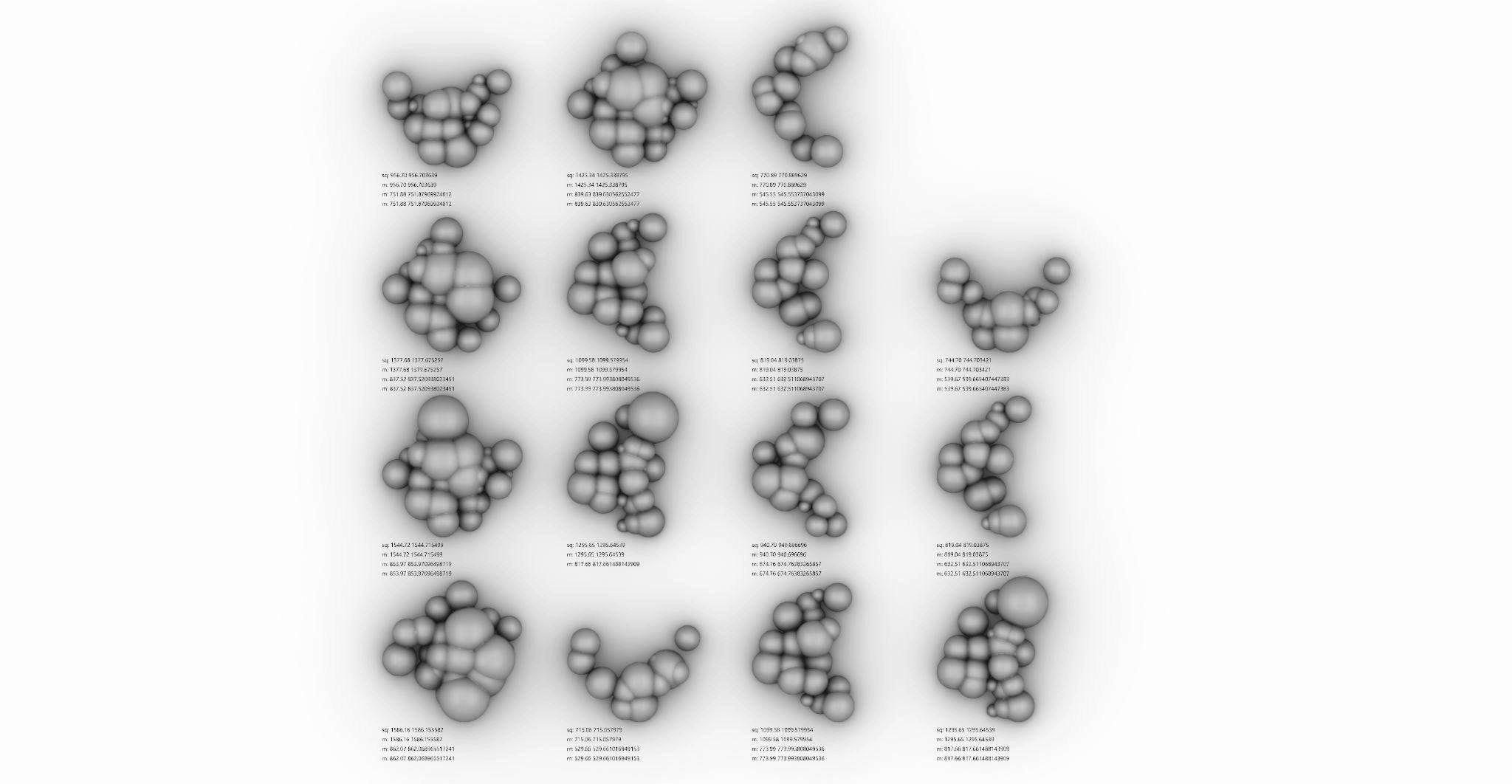
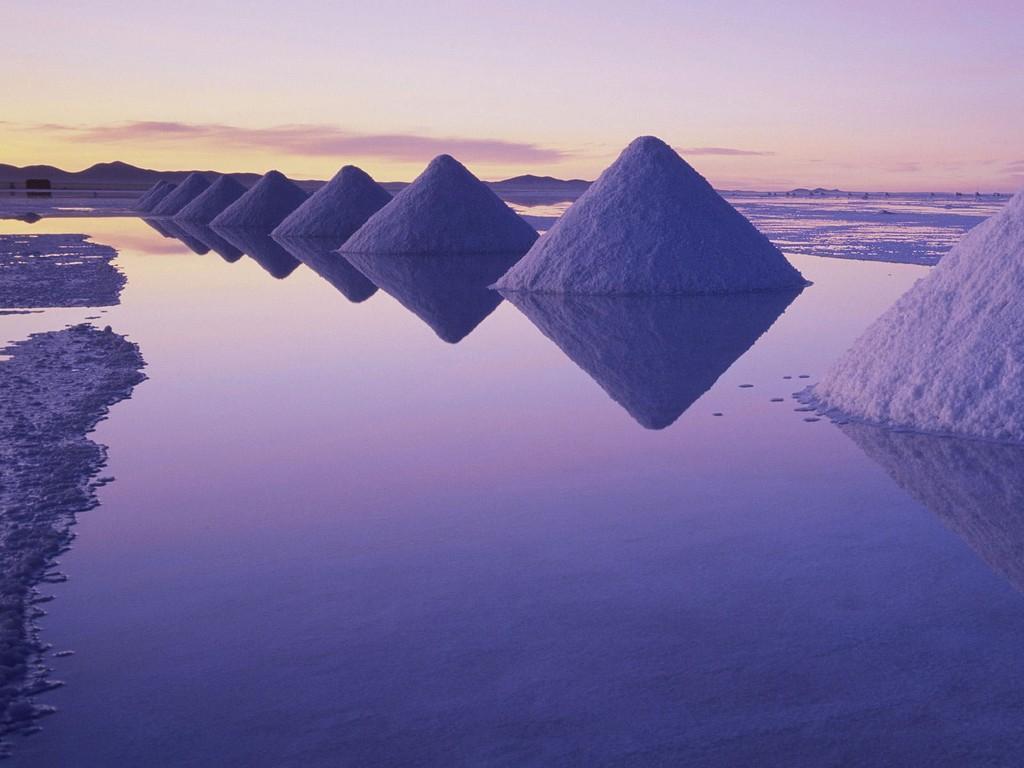
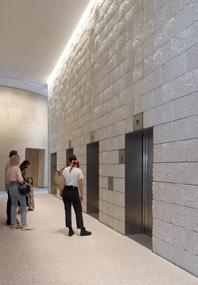
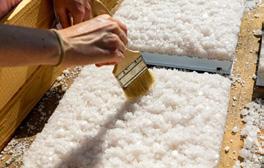
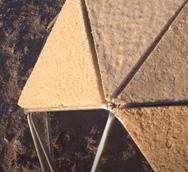
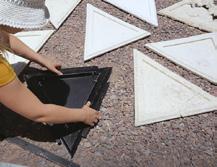
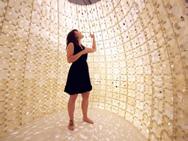
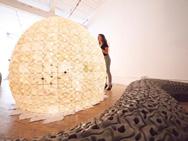
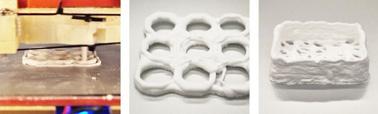
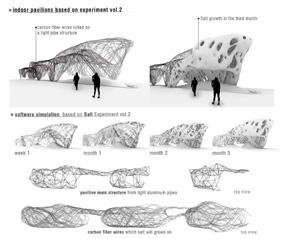
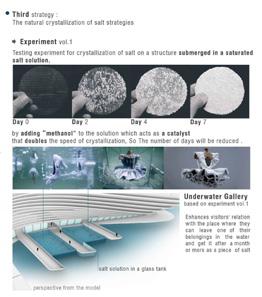
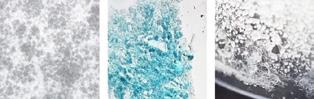
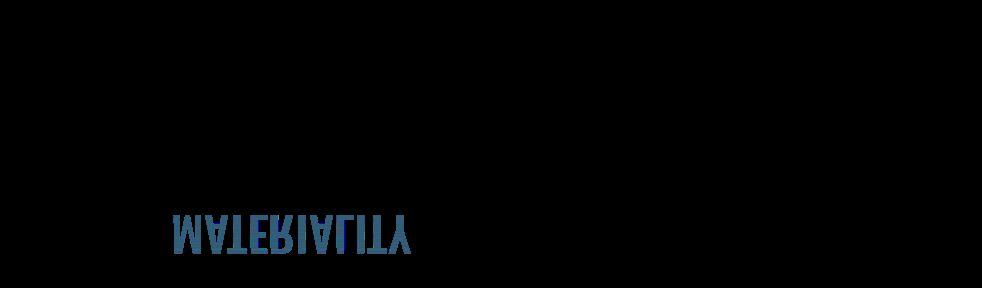
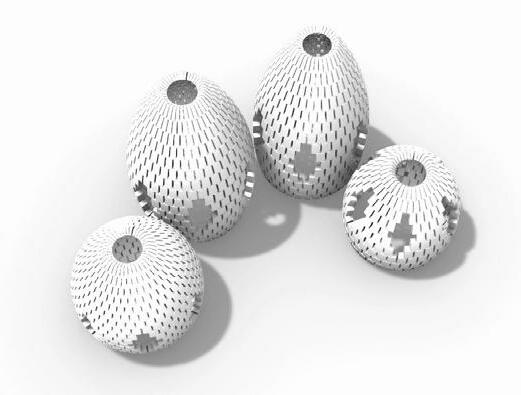
ANALYSIS & RESULTS SHELL

WIND LOAD CASE 2
WIND LOAD CASE 2
WIND LOAD CASE 1
WIND LOAD CASE 2
WIND LOAD CASE 1
WIND LOAD CASE 2
WIND LOAD CASE 1
Wind Load Utilization
If using shells, we could reduce the thickness in the areas that have the lowest utilization or create openings in these locations.
WIND LOAD CASE 2
WIND LOAD CASE 1
Wind Load Displacement
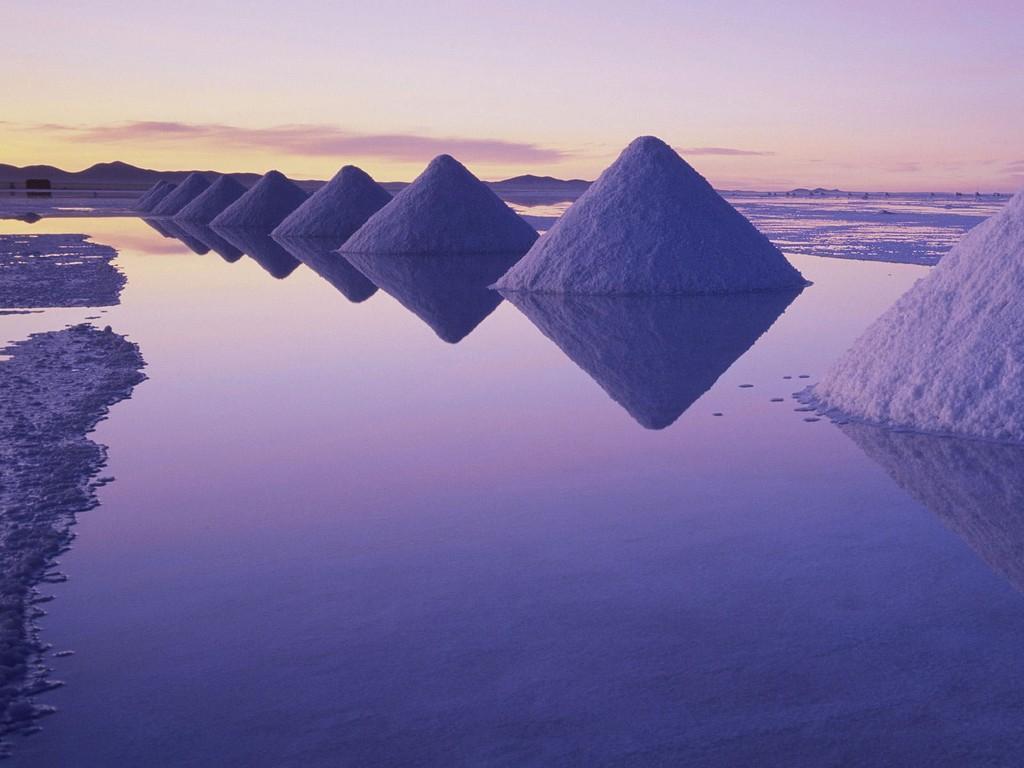
Darker pink represents higher displacement. The lowest displacement is in Yellow. The highest displacement is in green.
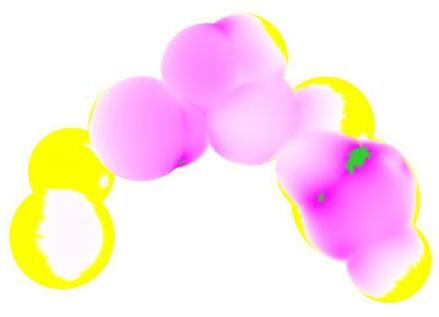
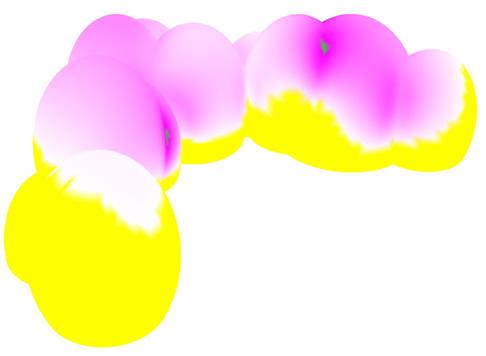
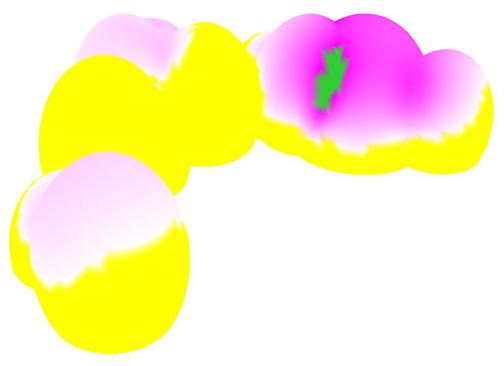
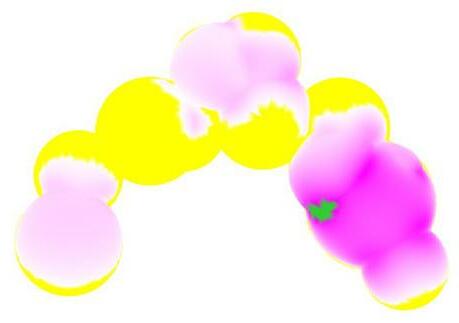
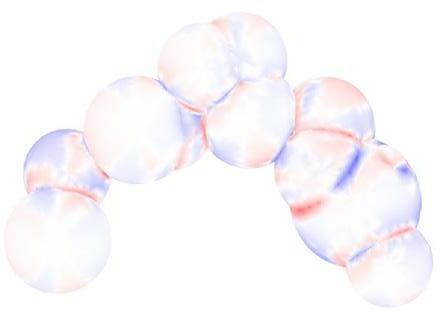
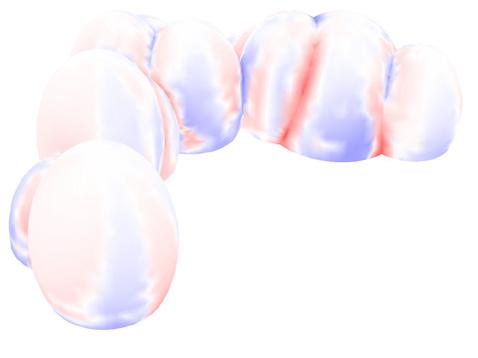
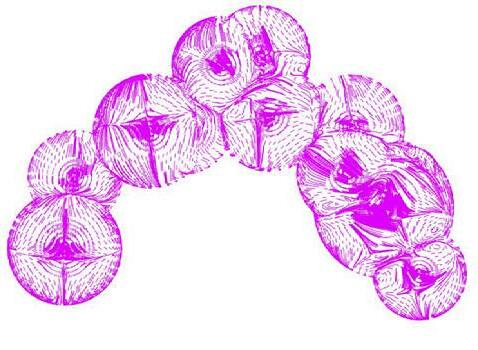
WIND LOAD CASE 2
WIND LOAD CASE 2
WIND LOAD CASE 1
WIND LOAD CASE 2
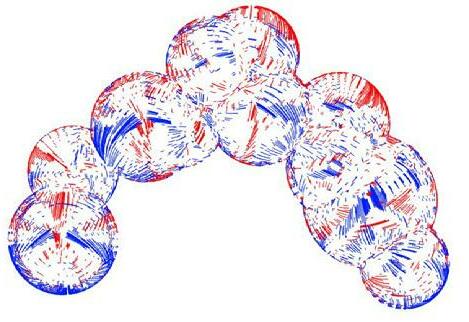
WIND LOAD CASE 1
Principal stress lines
Principal stress lines are important because they distinguishes between Tension and Compression. The red lines are in Tension, the blue lines are in Compression. For the proposed grid design, only the quads that intersect Principle Stress lines are Triangulated.
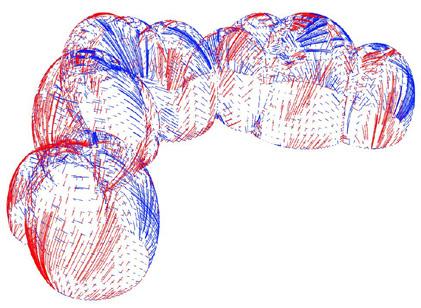
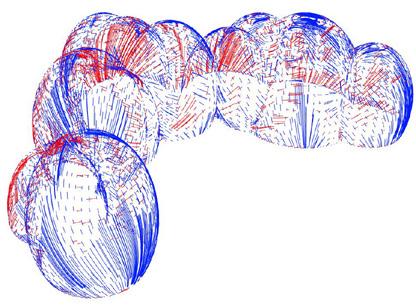
WIND LOAD CASE 1 WIND LOAD CASE 1
Forceflow lines
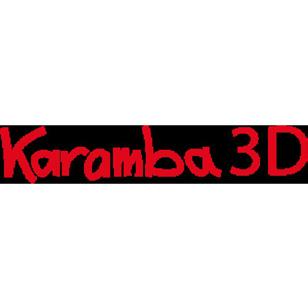
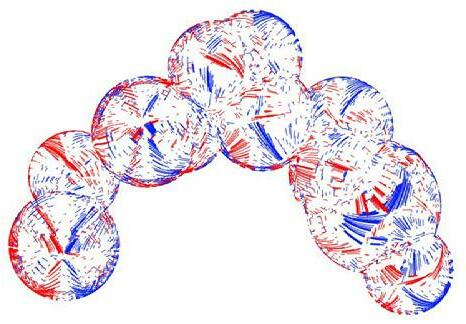
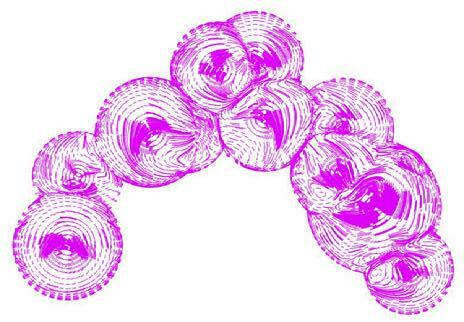
As expected, the forceflow lines would simply scale up in the Z-direction.
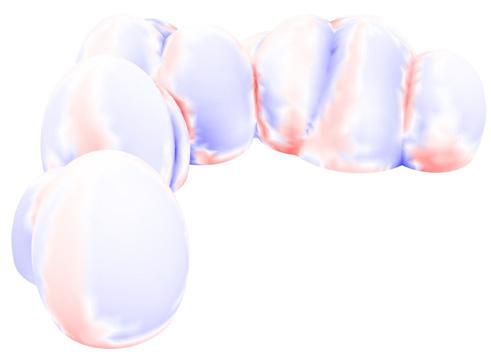
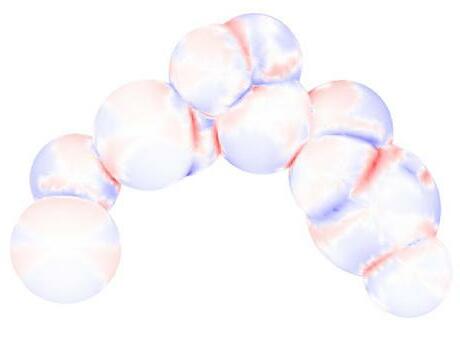
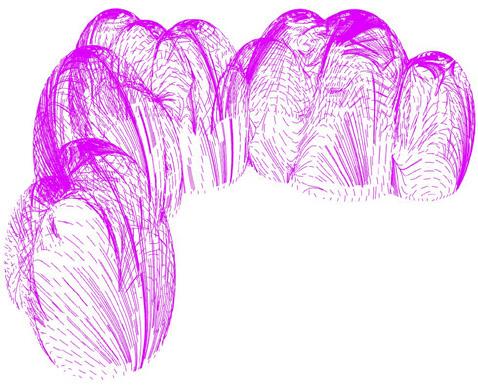
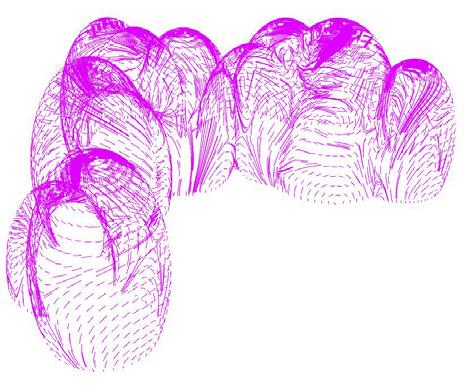
ESPEJO
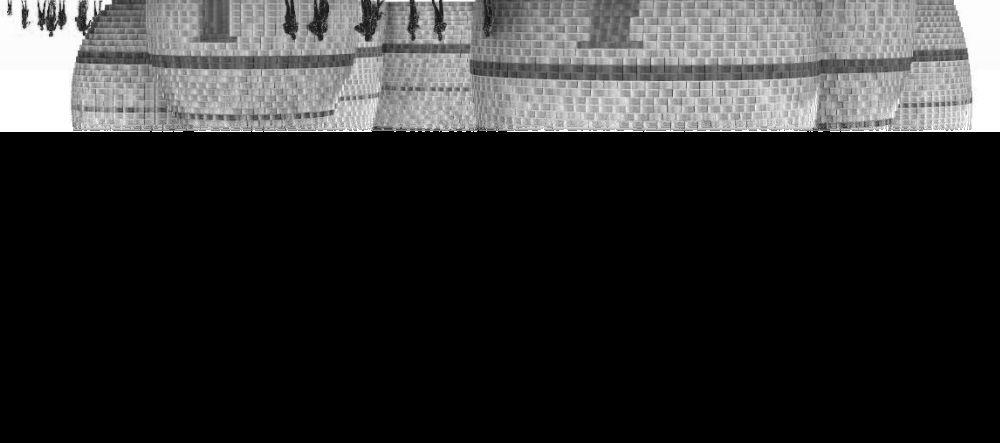
Salt Domes
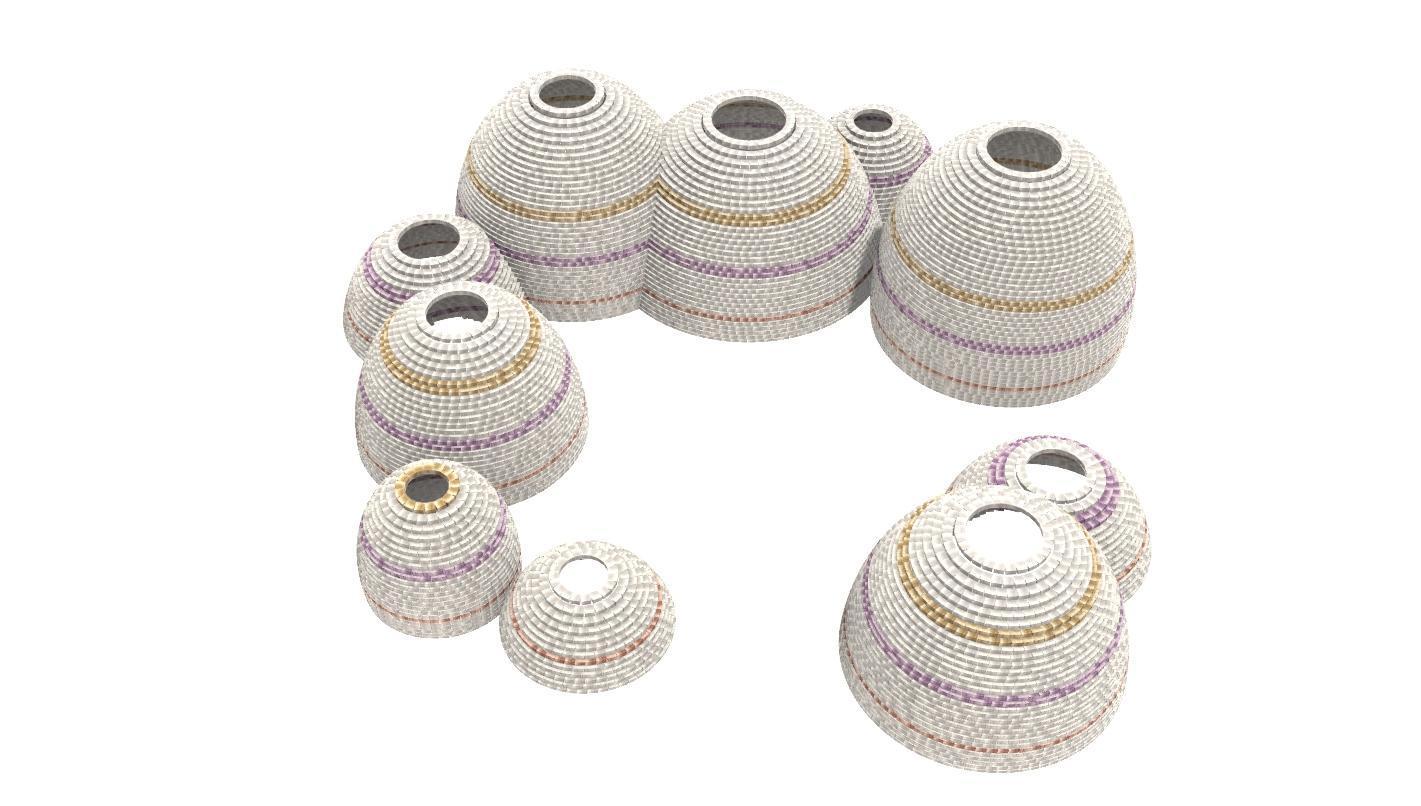
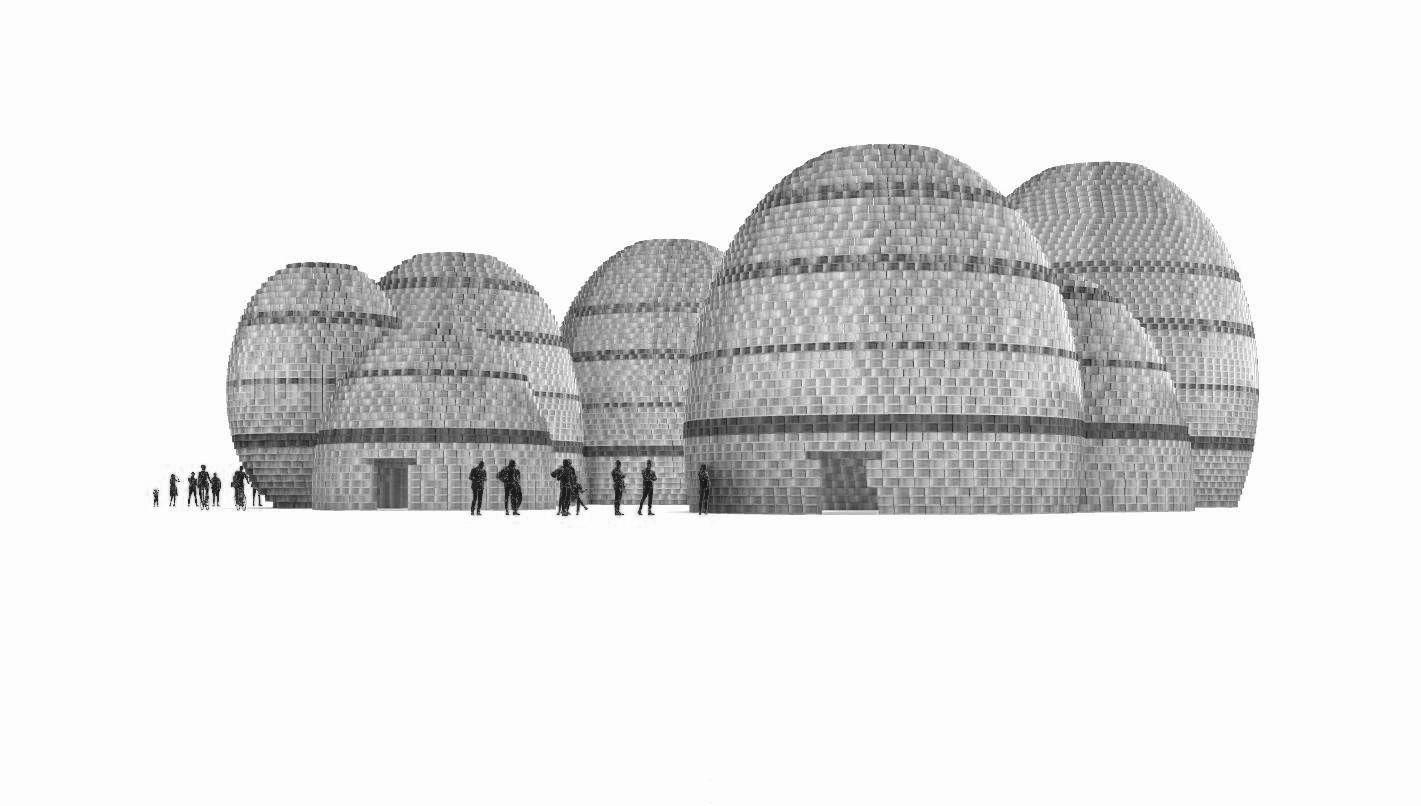
Bazars areas
The functions
The size and all the iteration can be applied for too many uses , like sell areas , art spaces , relax areas, between others.
Exposition areas
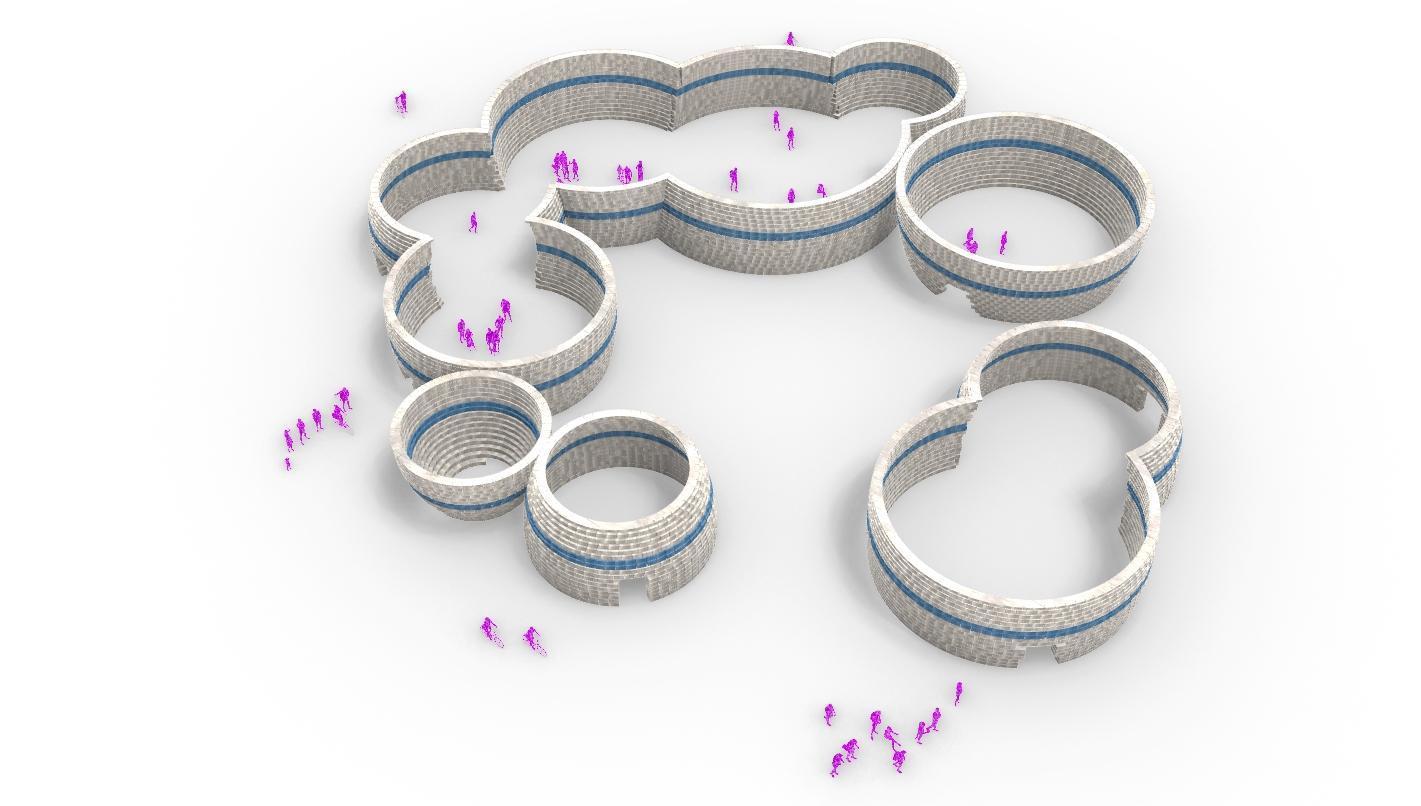
Salt Tower Cluster
LAYER OF TOWER
External structure
Woven structure where the natives will add the colors of the fabrics and wool colored.
Base Structure
The structure will be developed with wood and totora (plant near the Lake Titicaca)
Salt Bricks
The principal structure of the proposal , based in brics.
Base Structure
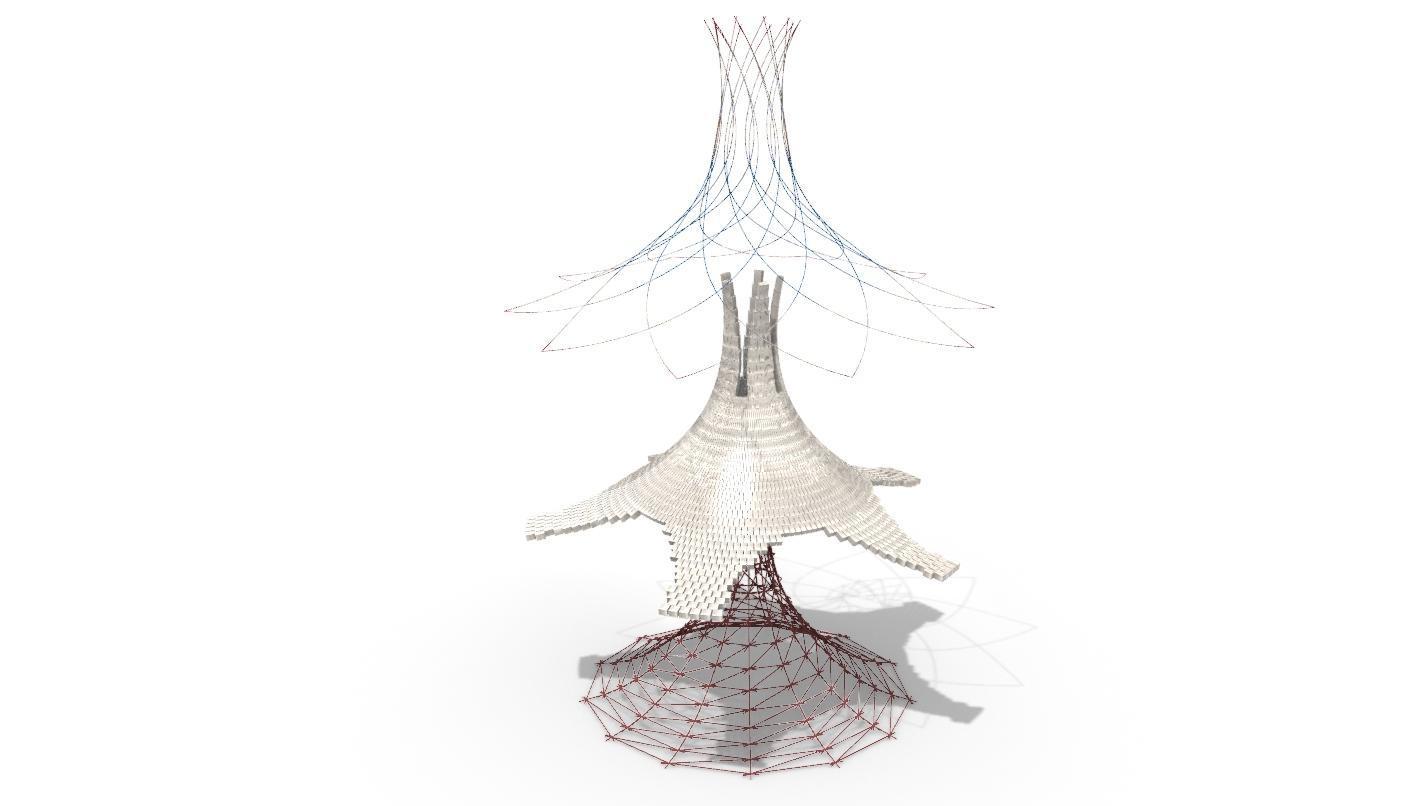
The structure will be developed with wood and totora (plant near the Lake Titicaca)
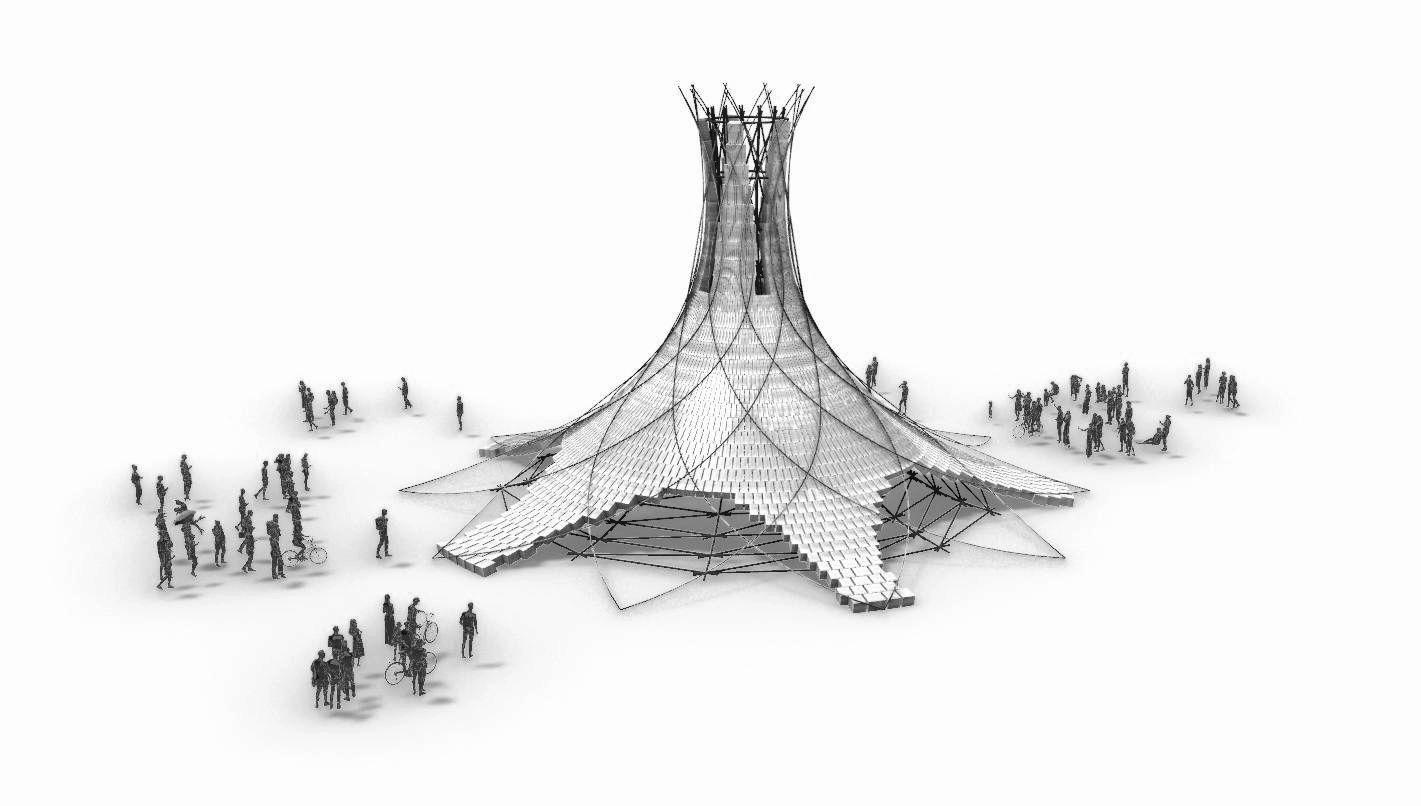
Colored Bricks
Pattern Variation , painted as the fabrics of the communities near the salar
Mini Domes
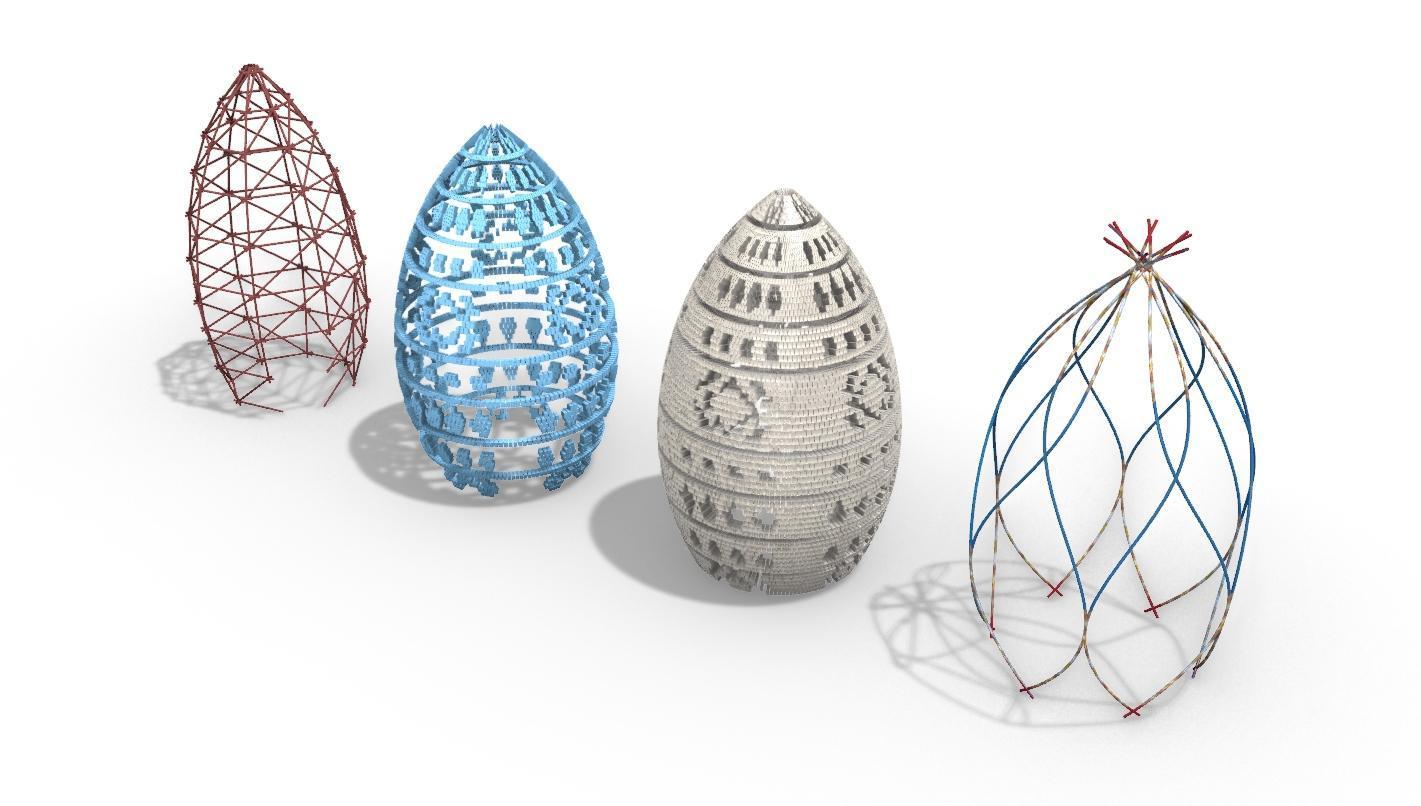
Salt Bricks
The principal structure of the proposal , based in brics.
External structure
Woven structure where the natives will add the colors of the fabrics and wool colored.
The place where the visitors can interact with the structure , inside and outside , The external structure are cover with the traditional fabricsWINGS OF CHANGE COMPETITION
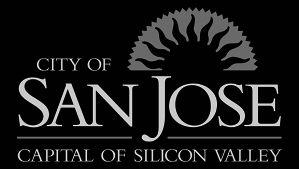
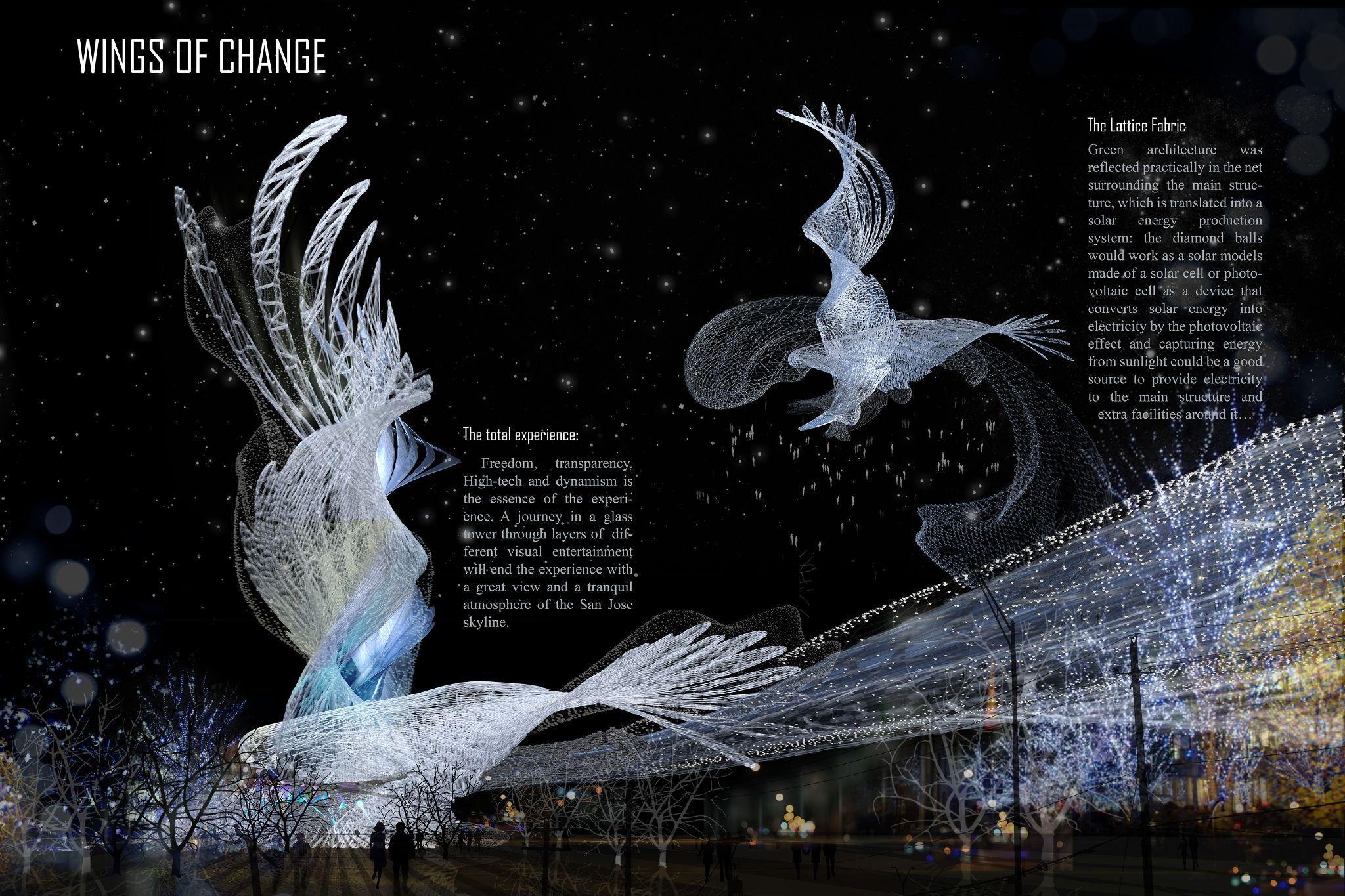
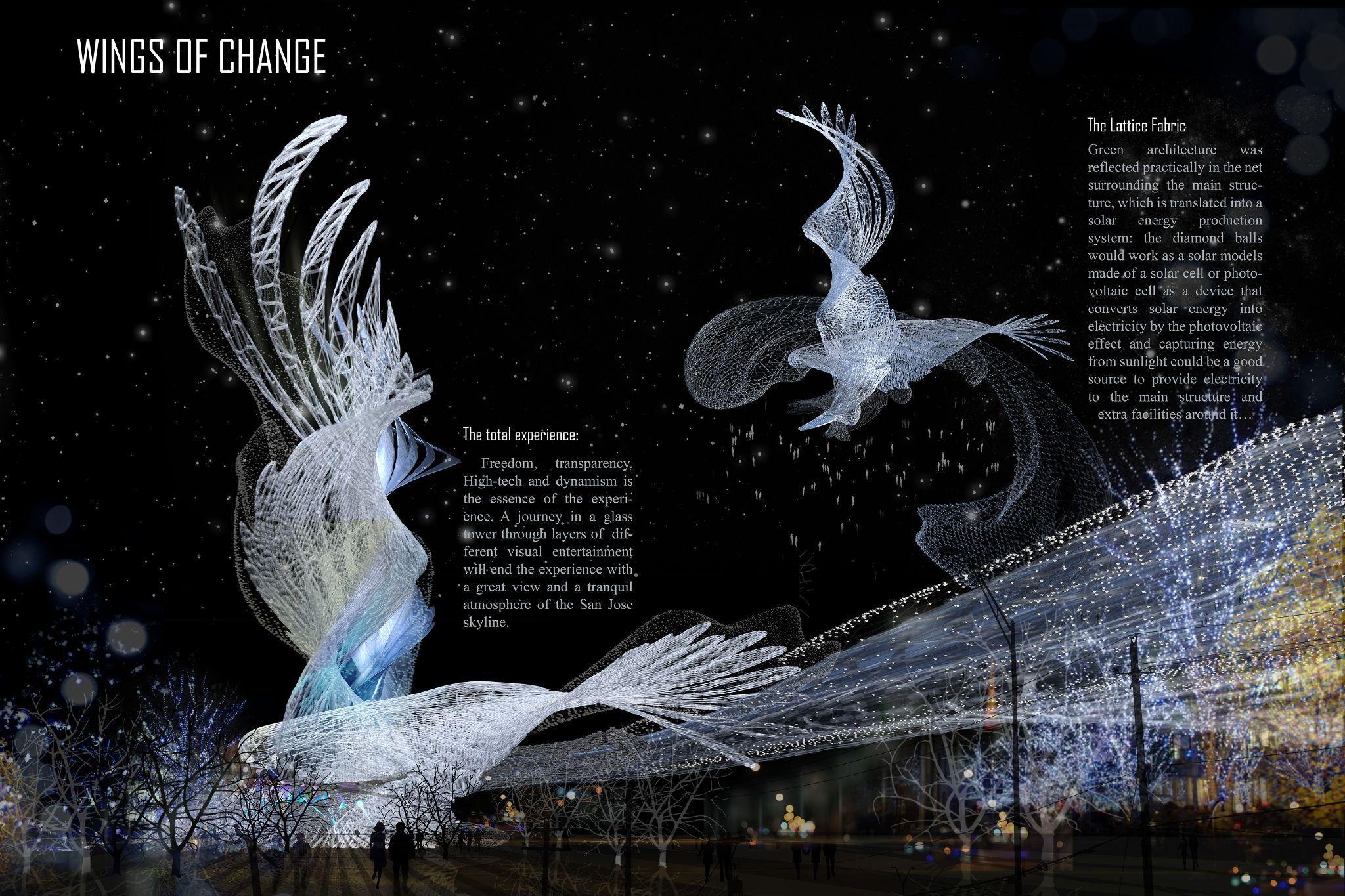
FUTURE CITY
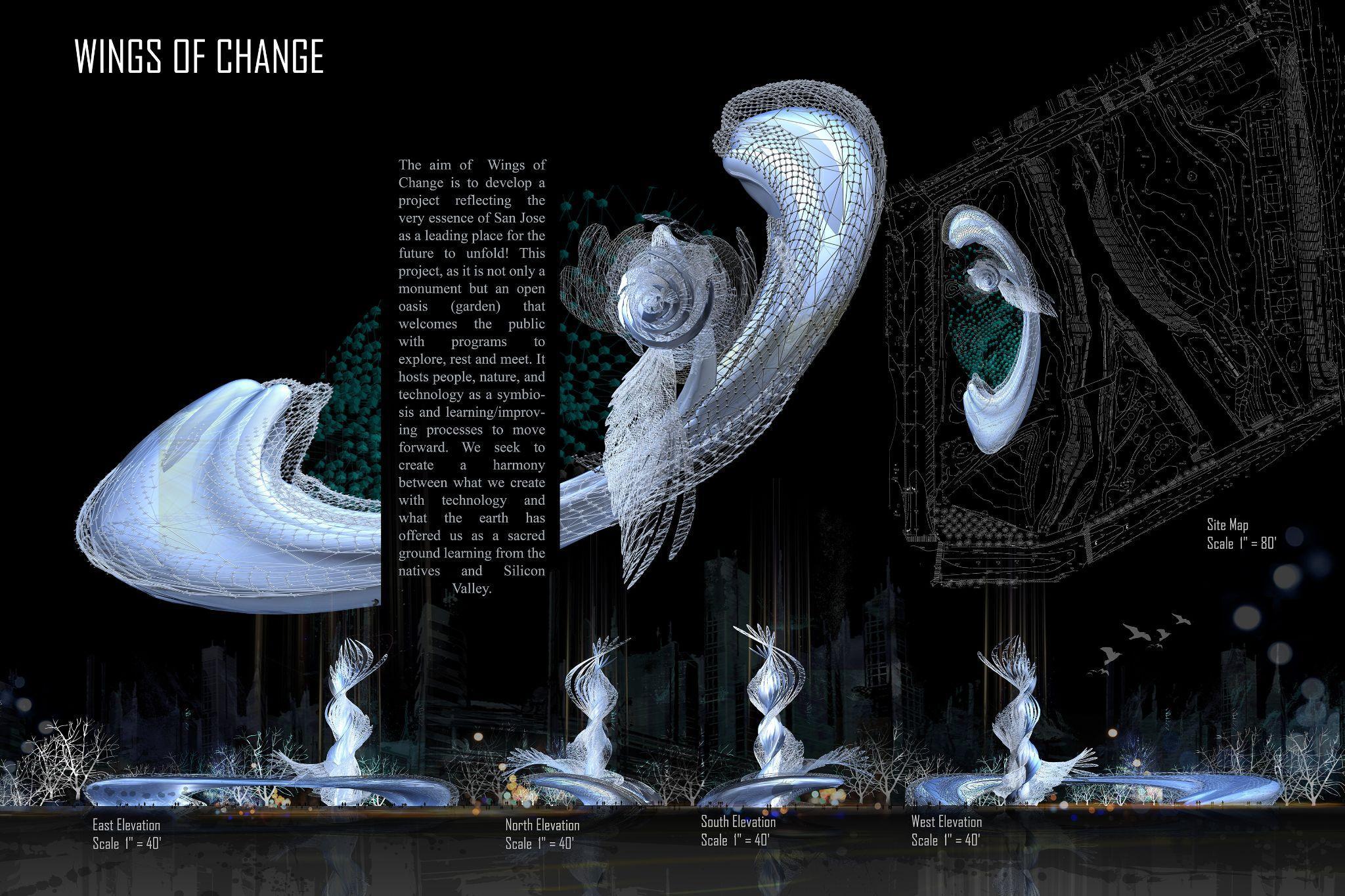
Date:2000
10Location: Baghdad, Iraq
Collaborators: Jumana Hamdani
Project Type: Academic at Baghdad University
Tools: Hand drawings: Ink, Zipatone
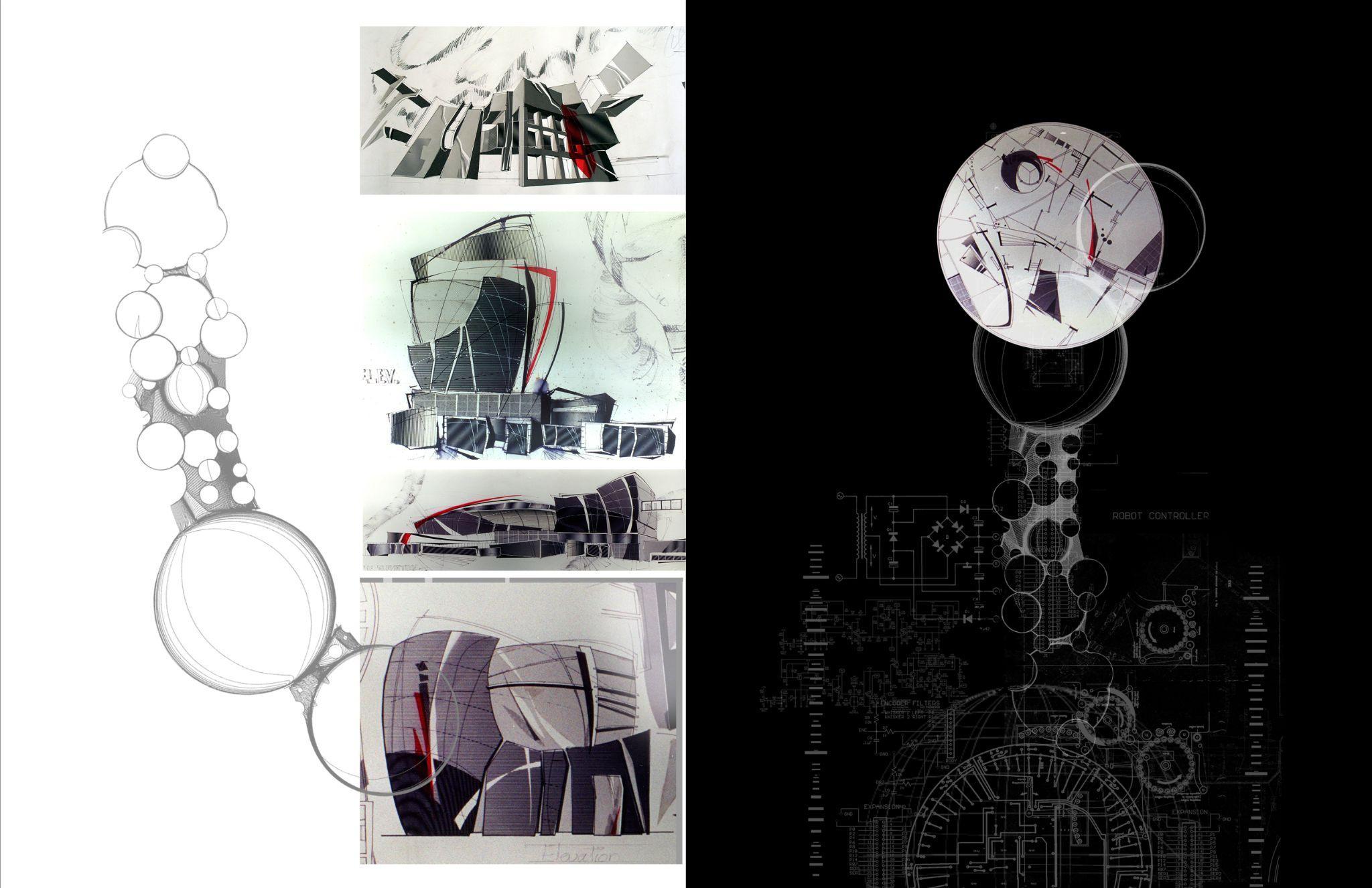
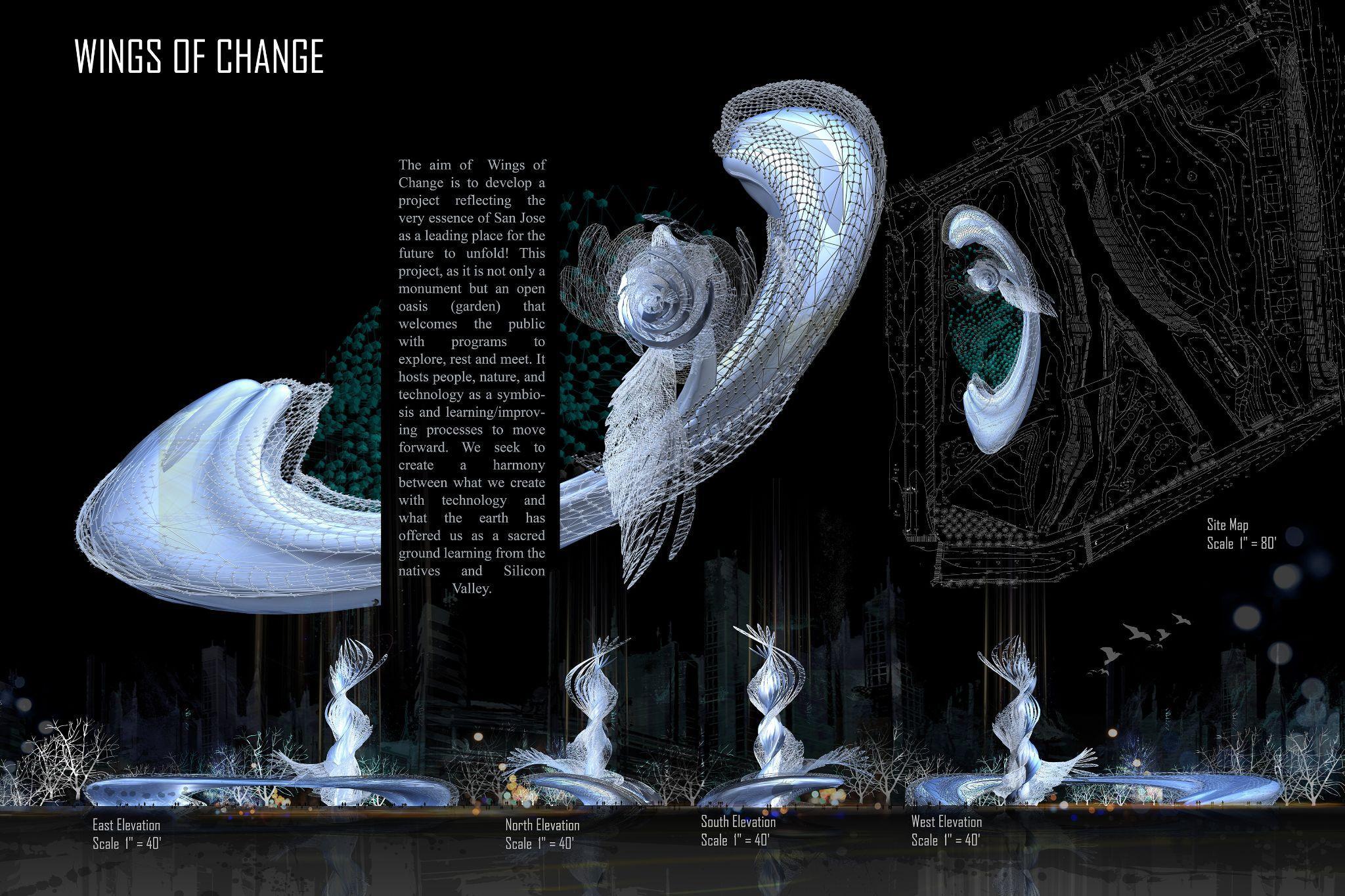
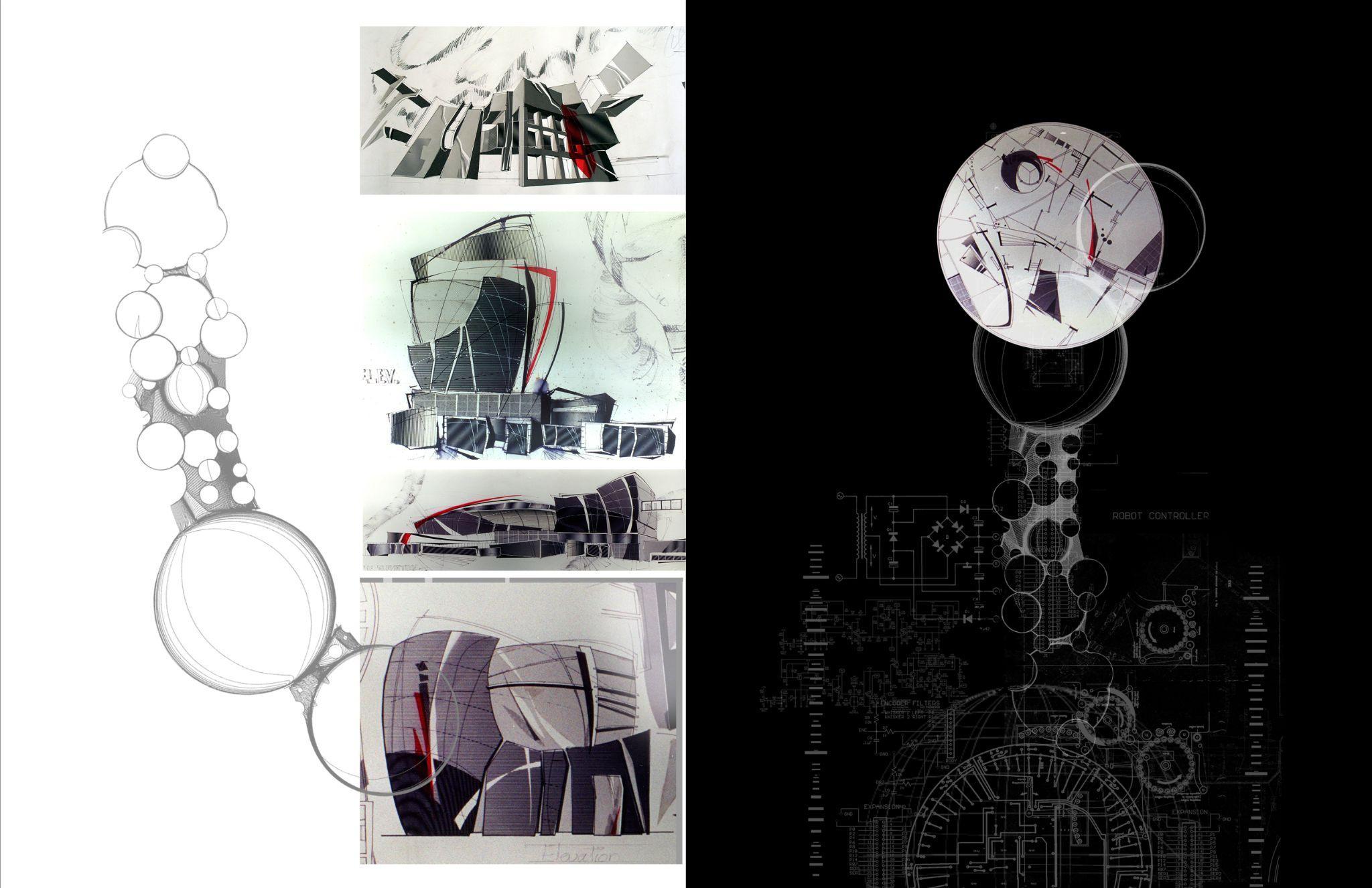
MÖRRUM CONFERENCE CENTER
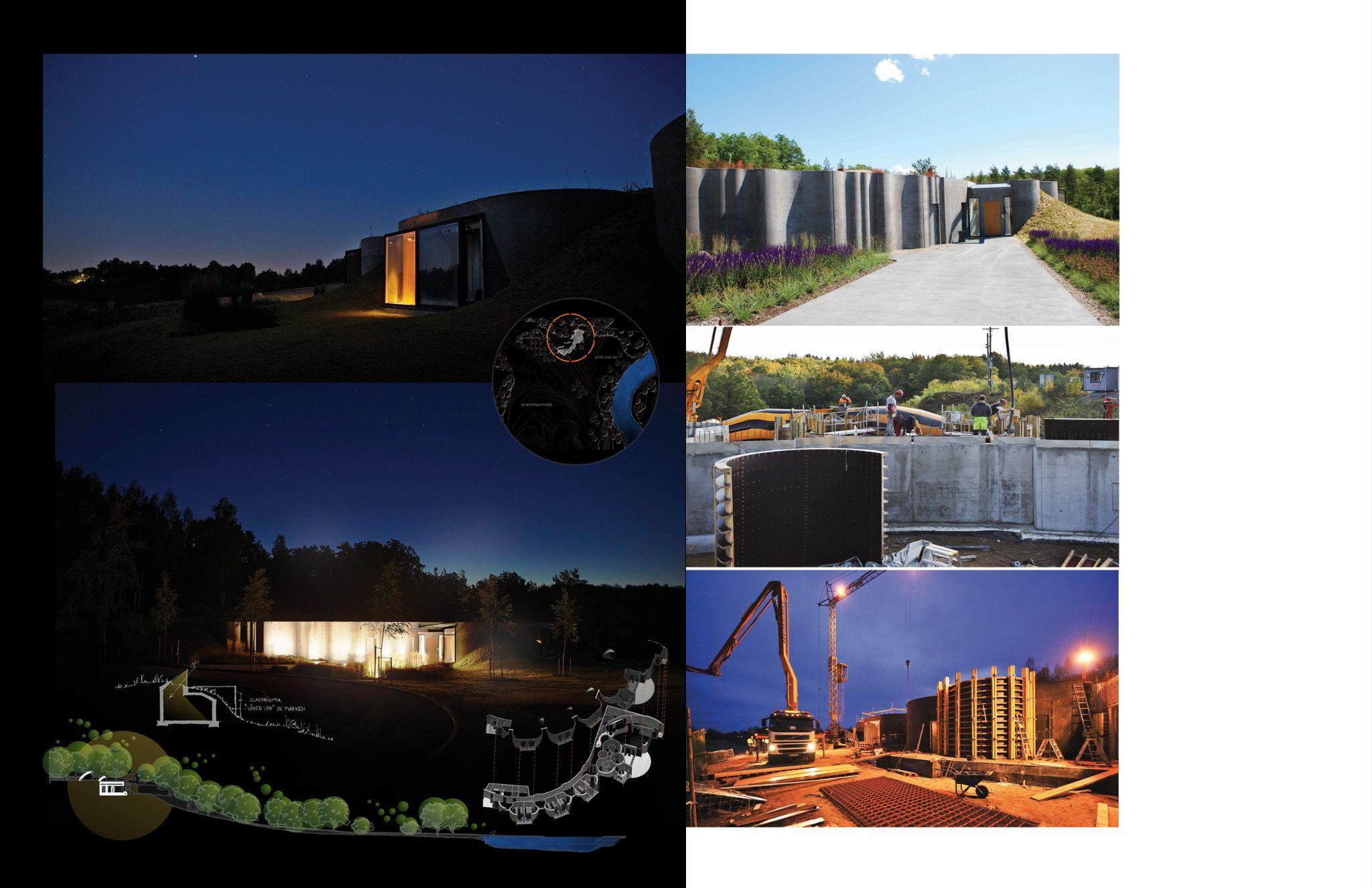
Project description:
Components: conference room, five double rooms, sauna, bathrooms, kitchen bar, wine storage, guest room and outdoor terrace
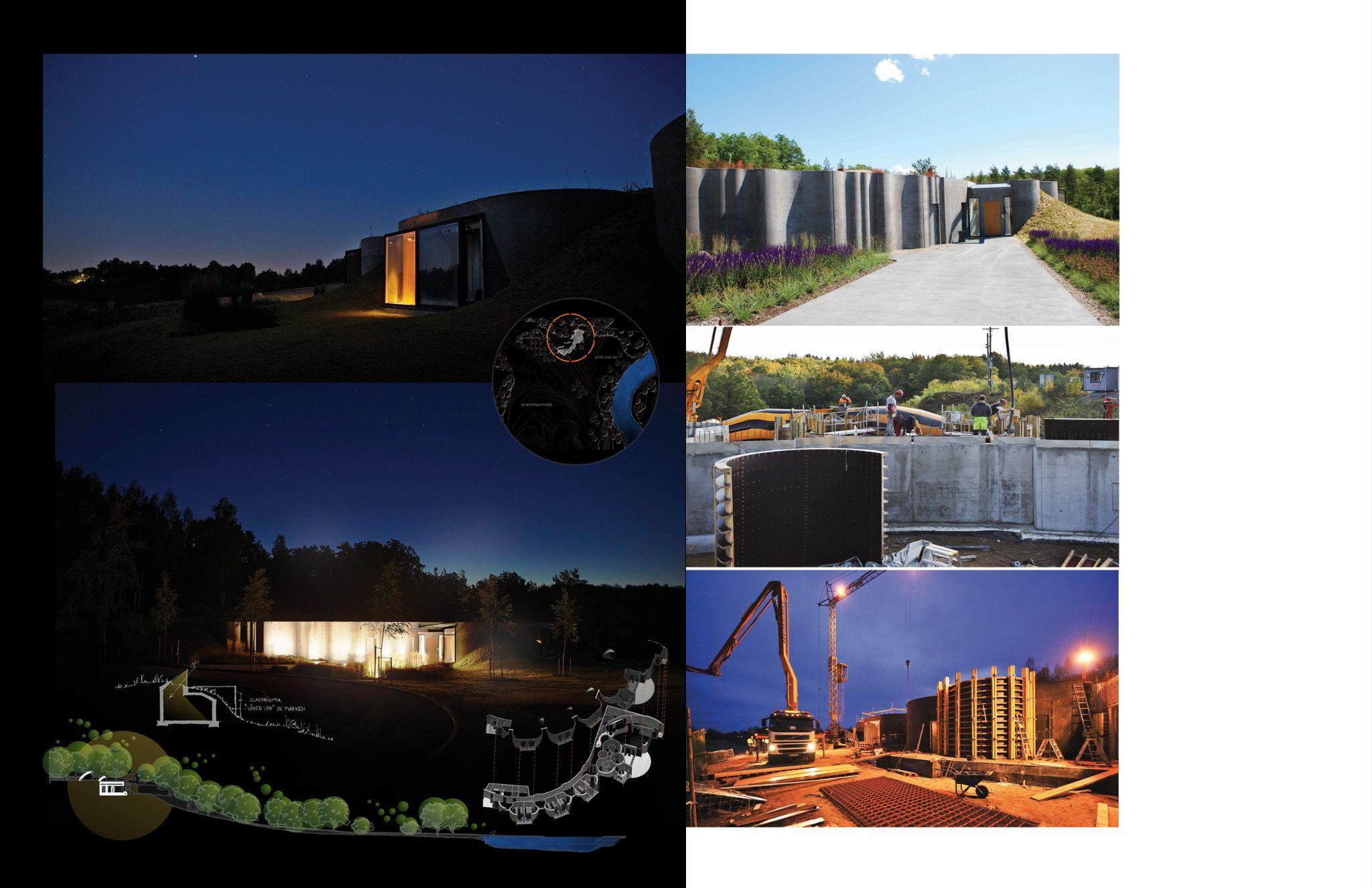
ARAB OPEN UNIVERSITY
Date: 2006-2007
Location: Amman, Jordan
Collaborators: at CCG & Jafar Tukan
Project Type: Built, Educational
Tools: AutoCad, Revit, 3D Max, Photoshop
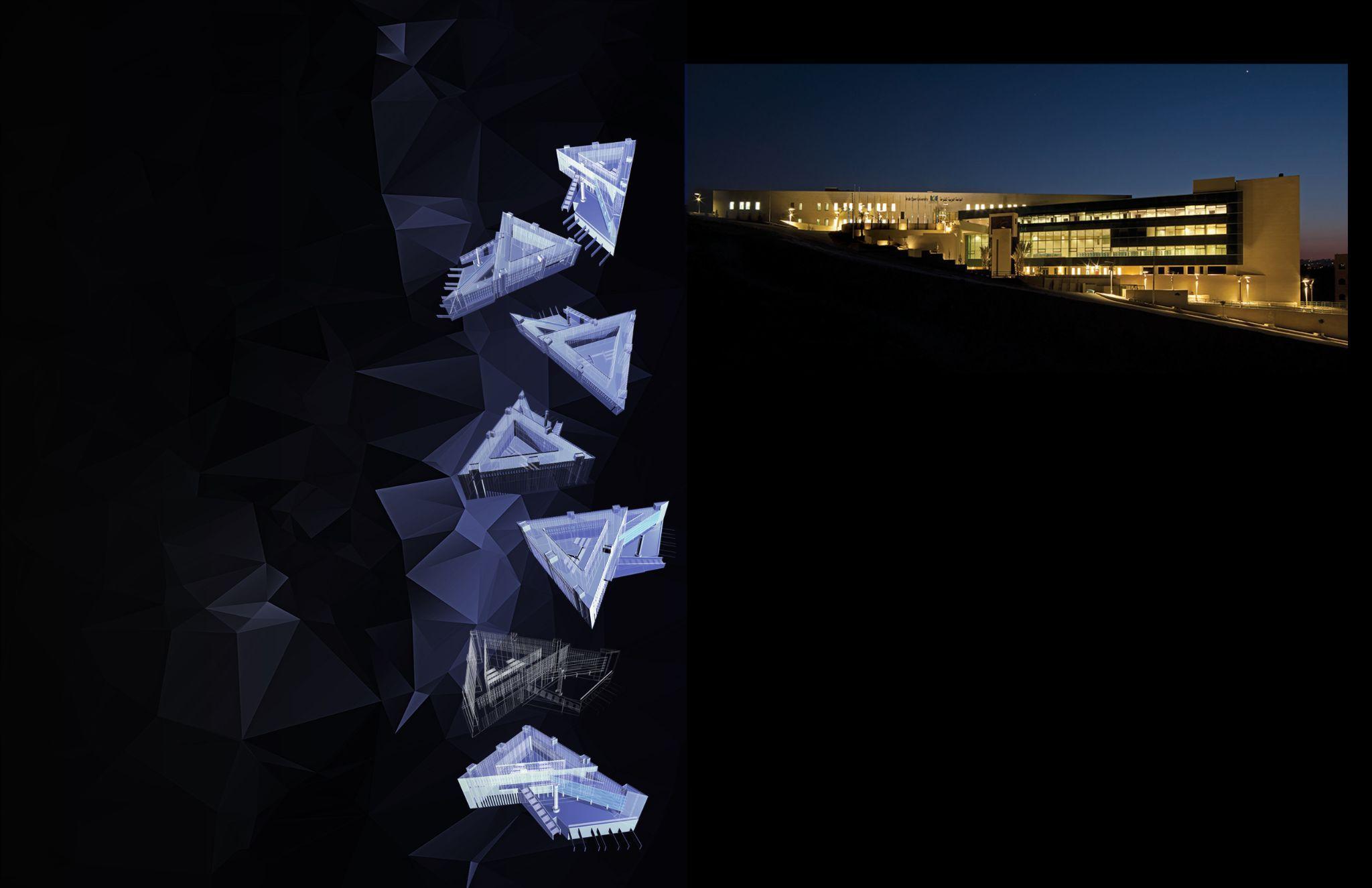
Client: Arab Open University
Project Description: The project consist of a permanent educational branch facility for the Arab Open University that includes the following main departments: - Senior branch management - Administrative affairs - Academic affairs - Academic programs and instructional spaces - Education and information technology - Branch support
Total built-up area of 11,000 m2
Landscape Design Elements
Ceremonial court: This court which is located at the east side forming the formal festival entrance of the project is designed as occasional gathering space for special events, paved with geometric Arabic decorative patterns, provided with elevated stage, and some planters.
Amphitheatre Court: Semi-formal space, planted with vertical elements (palm trees) to break down the scale of the surrounding building and to provide pleasant atmosphere for the users, this court is to be paved formally with tree pits covered by decorative metal and suitable furniture to enrich the space as functionally usable area.
Courts Connecting Stairs: The shaded stairs connecting the main outdoor spaces are designed not only as connecting stairs but as, gathering seating steps, with colorful plants to soften the atmosphere of this space.
North-west Court: This court is the main activity, gathering, circulation and recreation area of the whole project, this court is treated as informal, relaxing area, paved with durable multi texture multi color patterns, provided with outdoor cafeteria service zone, table tennis and floor chessboard, clock tower and some sculptural elements and exhibition panels. This court is proposed to be an active, interesting, yet functional oriented meeting and gathering space.
North-West Garden: This area is the beginning point of the main pedestrian stairs and ramp leading to the project through the main court. Since this is the only natural - relatively small- part of the site free of buildings; it is simply designed and naturally planted.
ITC Services: The IT services for the university were designed in order to provide full internet accessibility for video conferencing, streaming and online teaching system. Giga speed infra-structure was maintained and provided for all IP based systems e.g. Telephone, data, internet and CCTV.
The scope of work comprised main server room design, core/edge switches designs, passive components design, vertical and horizontal backbone designs. Moreover, CC provided all tendering and supervision services for all IT installations.
Services Provided Architecture Design, Engineering Design, Landscape Design, Irrigation Design, Construction documents, Supervision & ITC Services
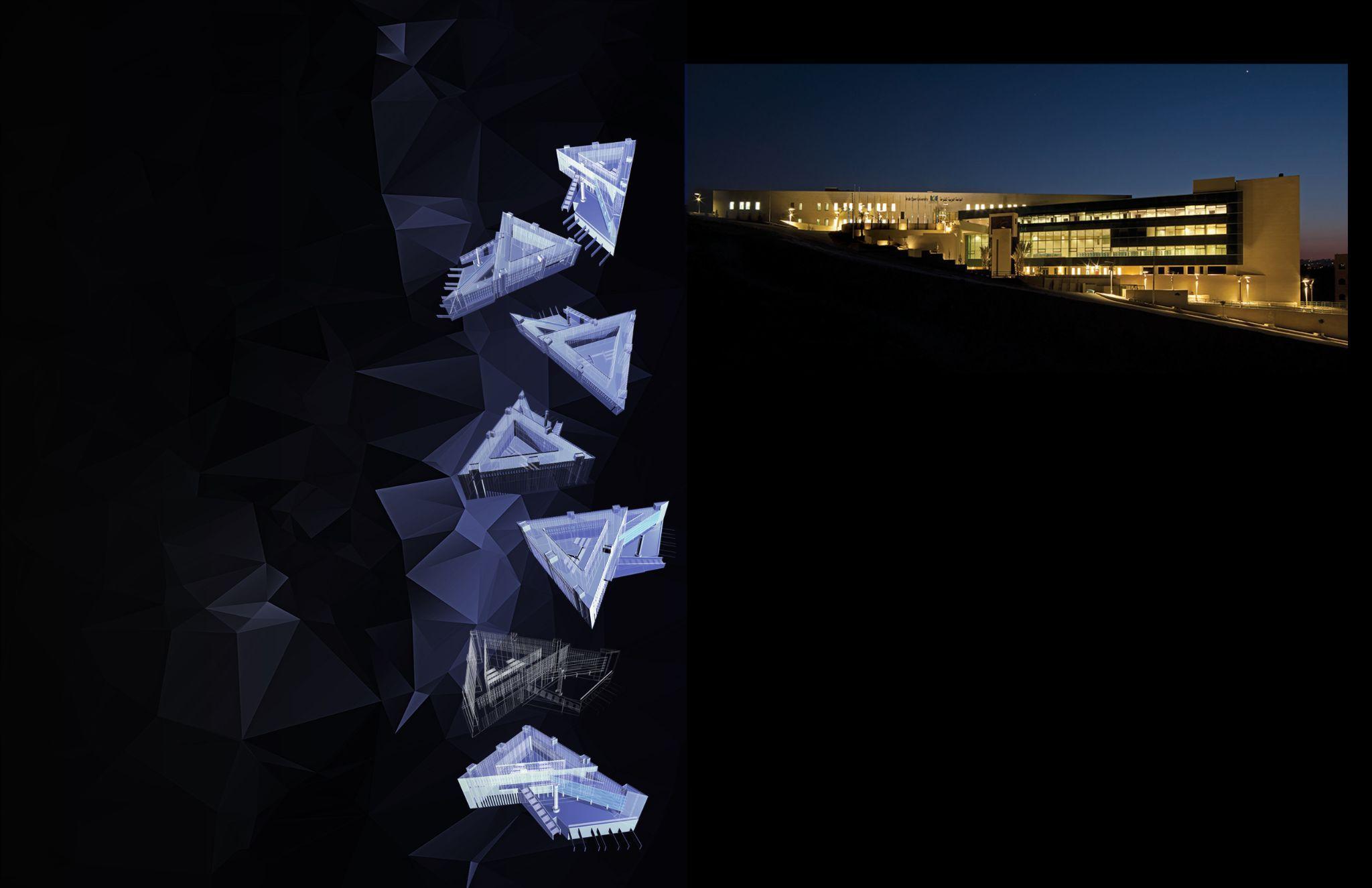
DUBAI EMBLEM STRUCTURE
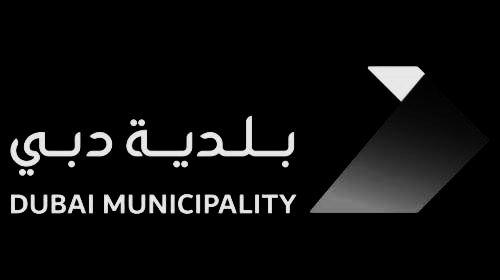
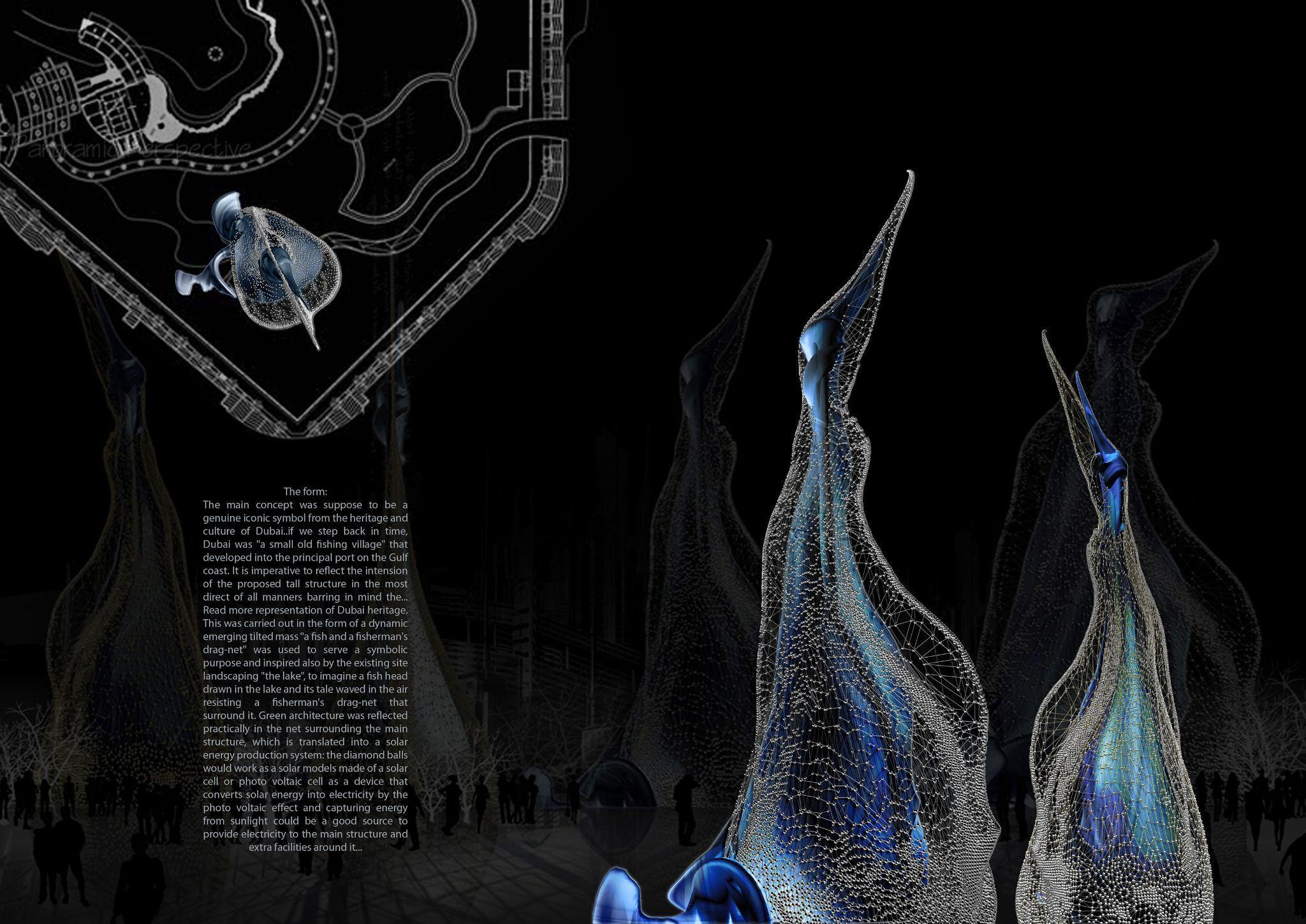
DUBAI EMBLEM STRUCTURE
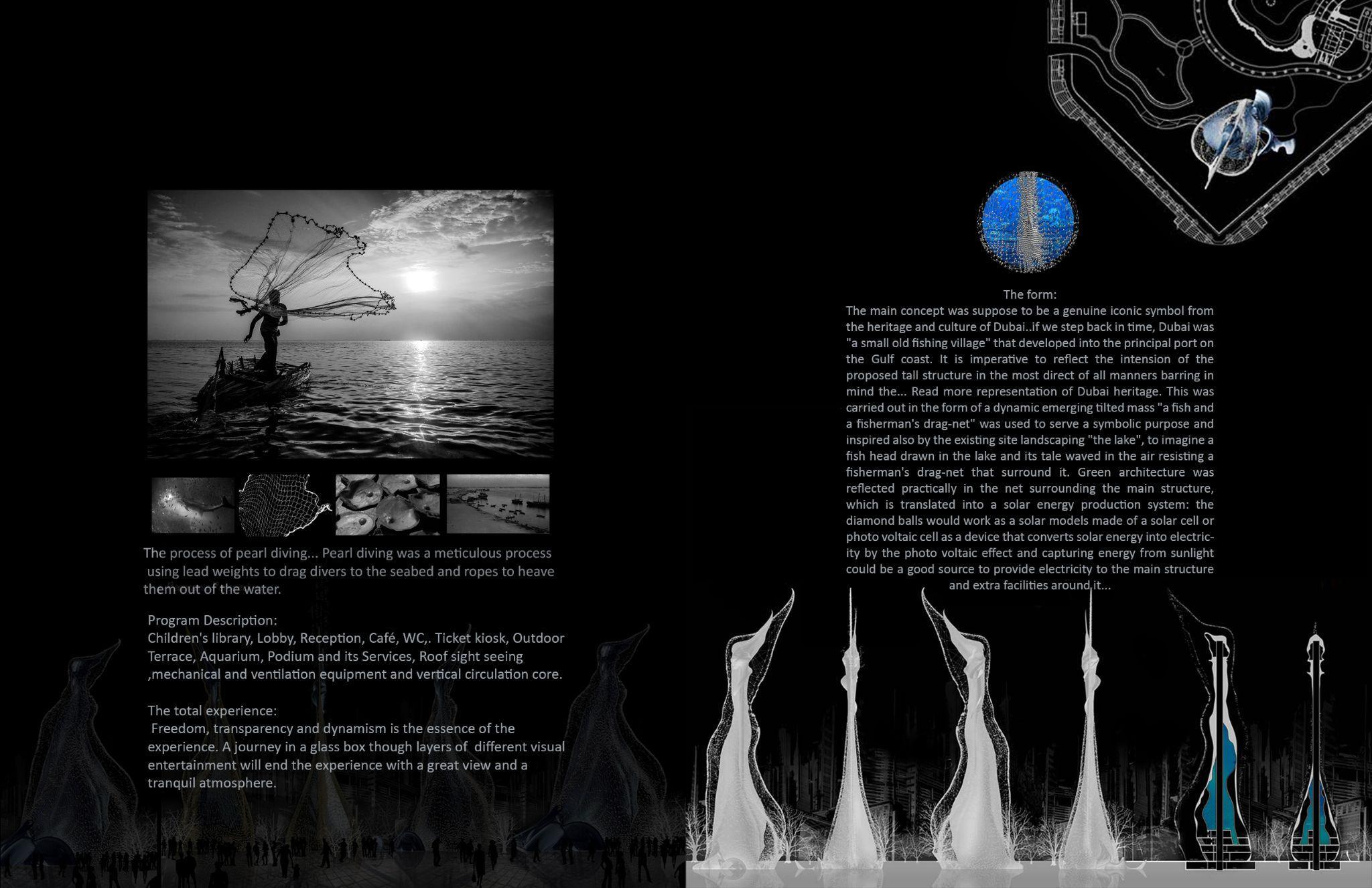
Elevations
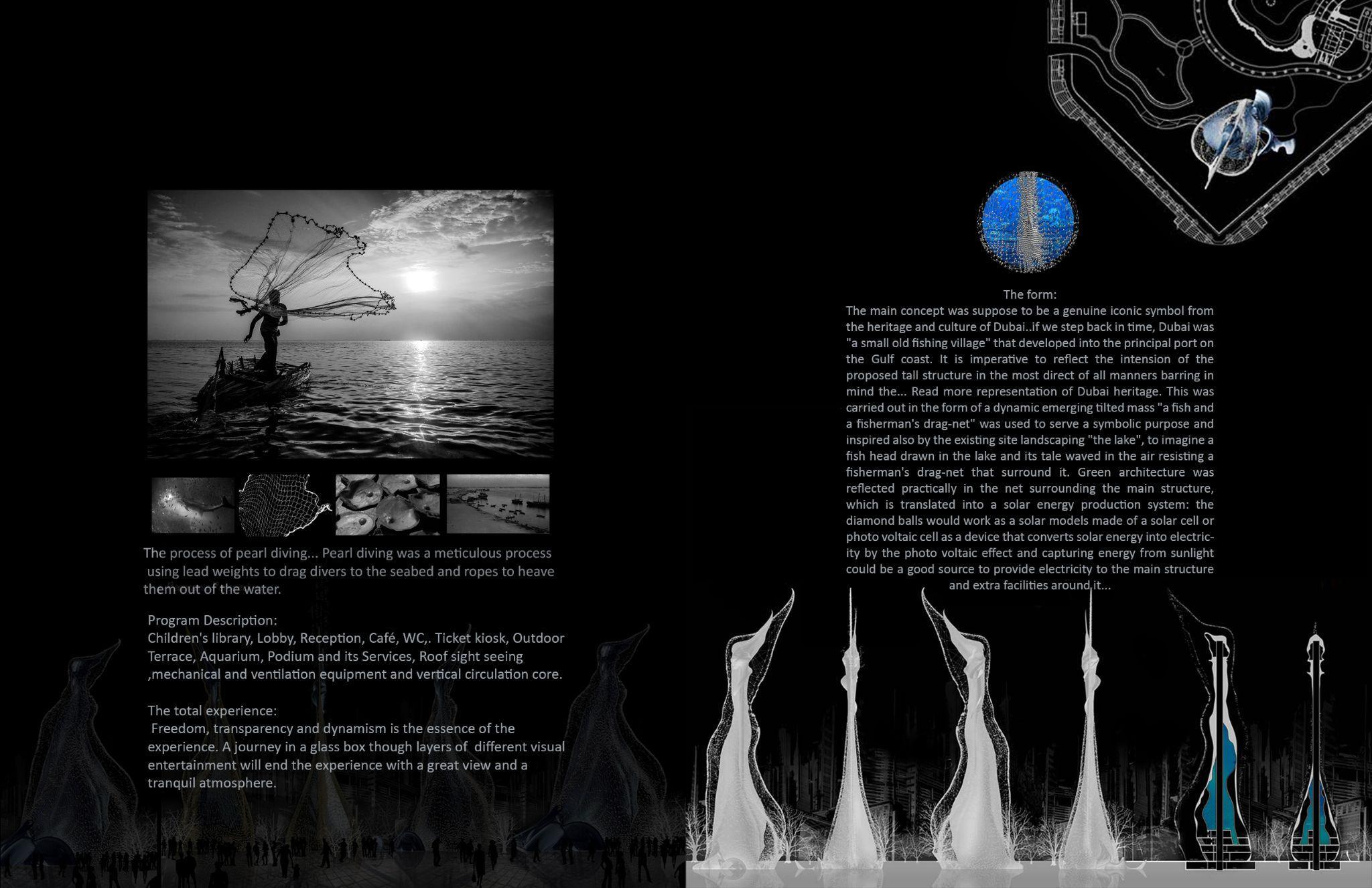
DUBAI EMBLEM STRUCTURE
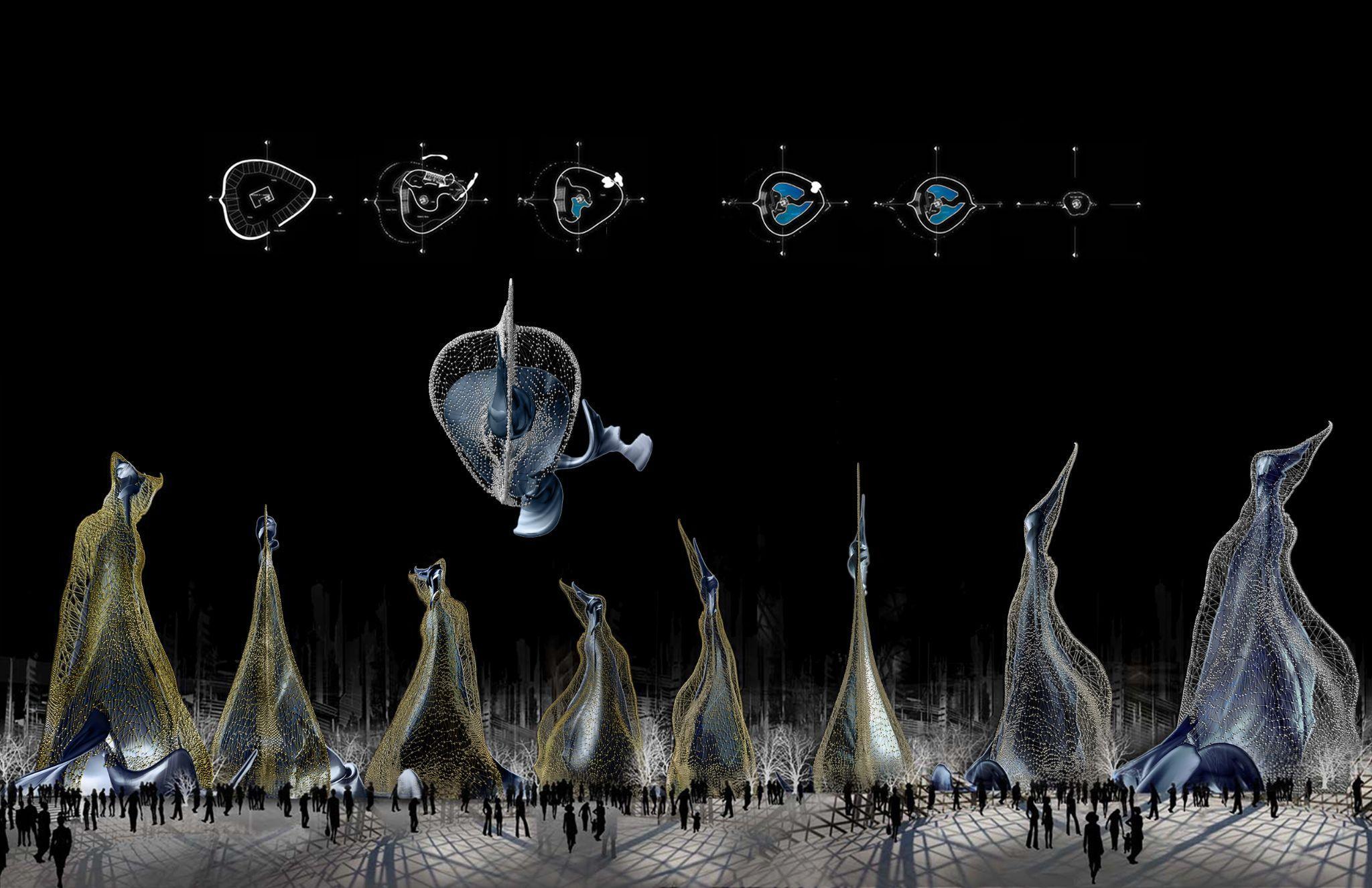
Panoramic View
Top view
Plans
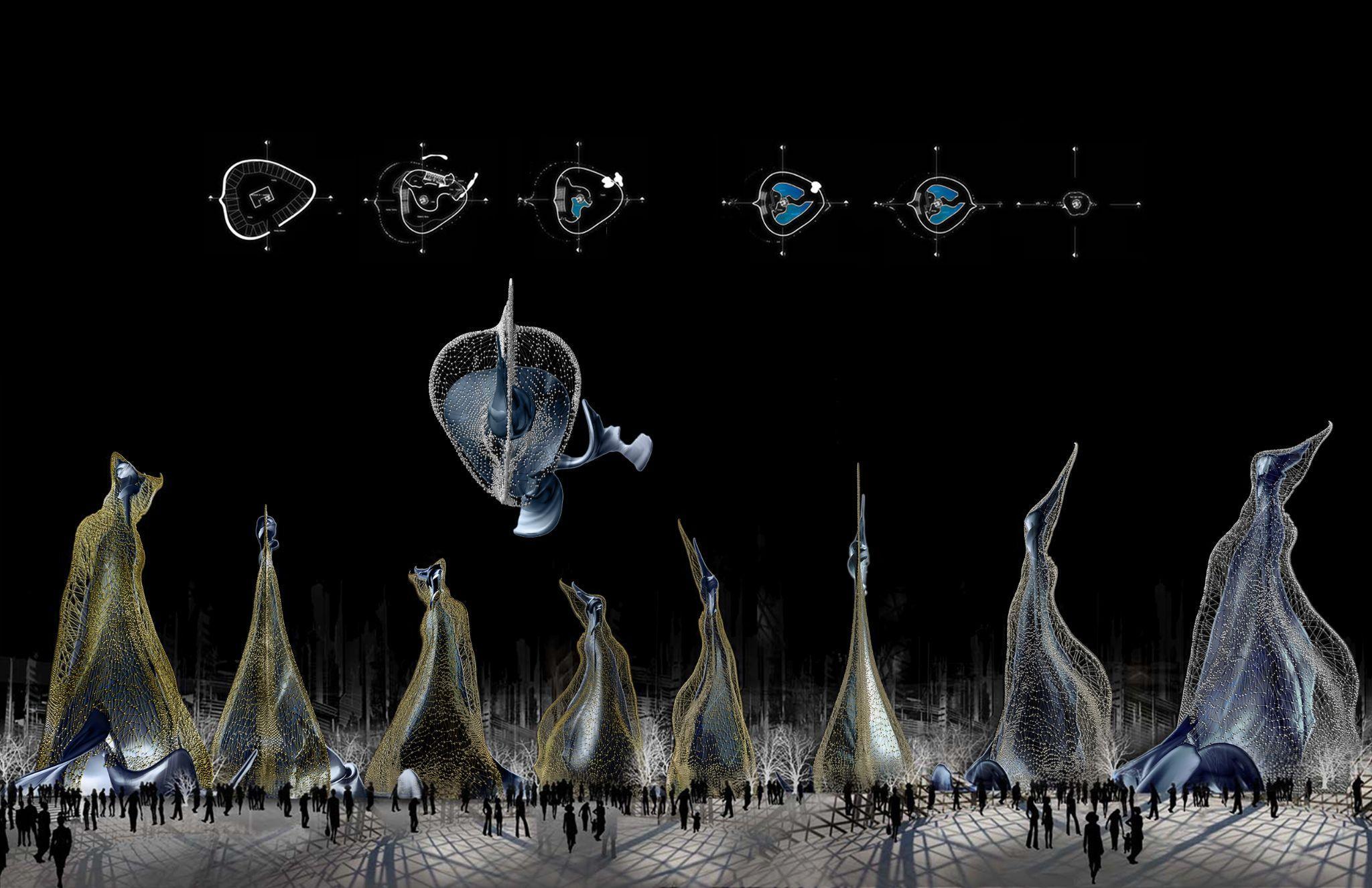
LONDON CLIMATE ANALYSIS
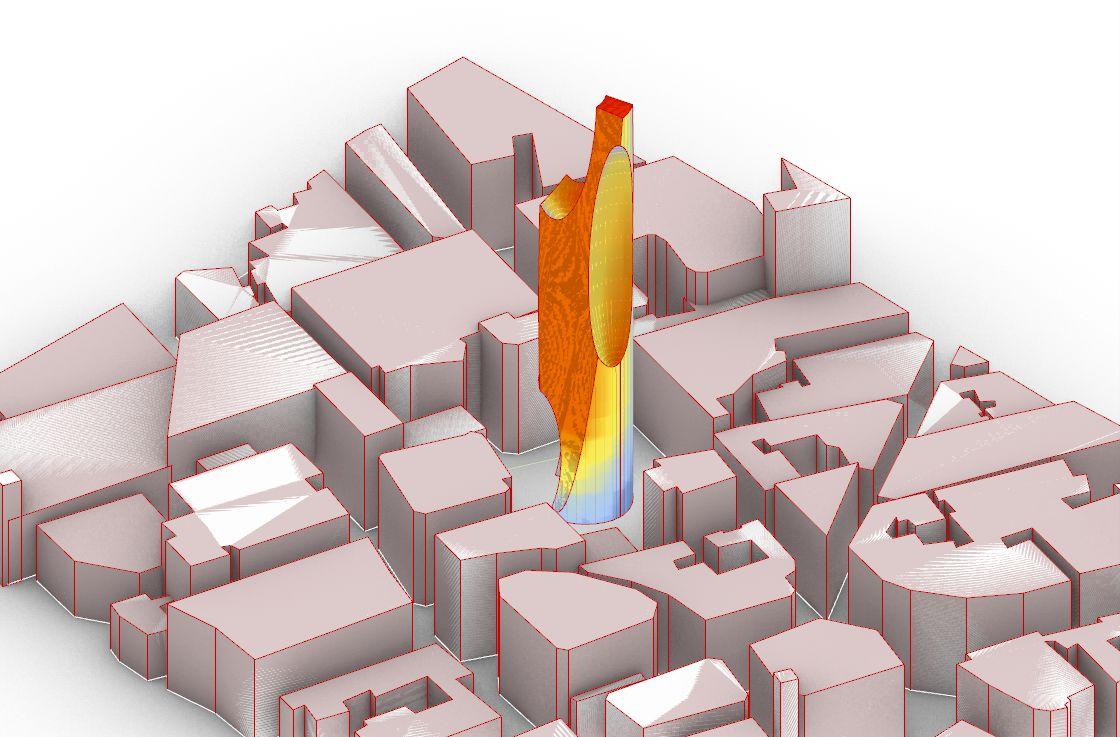
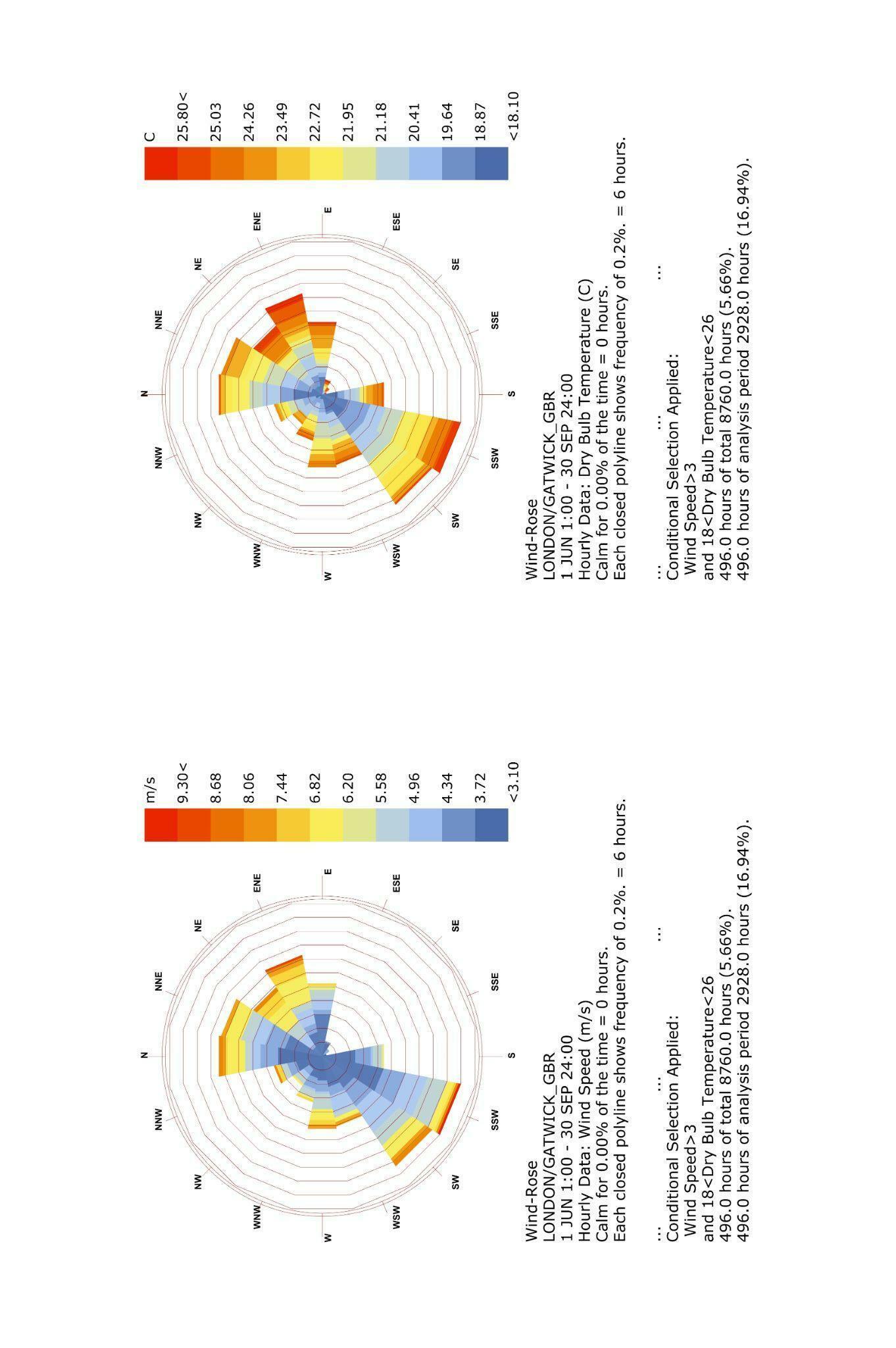
14
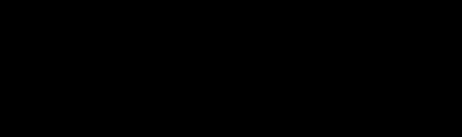
Date: 2021
Location: London, UK
Collaborators: Abed Badran Jumana Hamdani
Instructor: Angelos Chronis
Project Type: Academic at IAAC
Tools: Rhino, Grasshopper including plugins: Ladybug, Honeybee, Eddy 3D, InFraRed

THE SITE// LONDON
The context & Street view
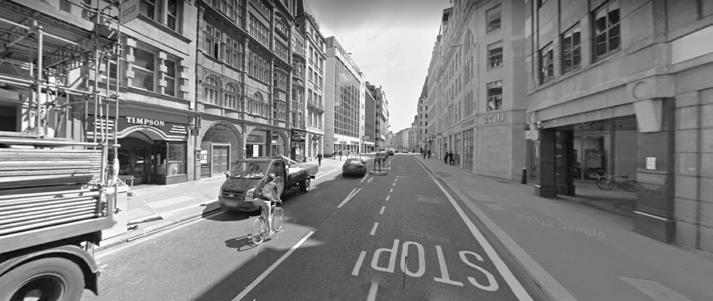
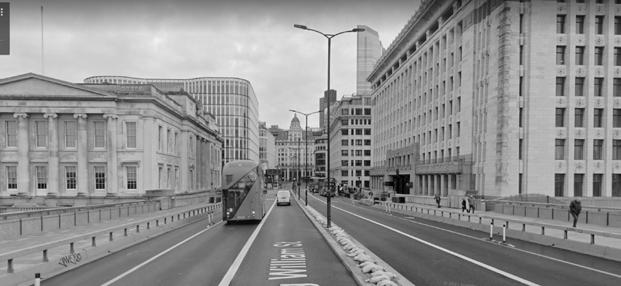
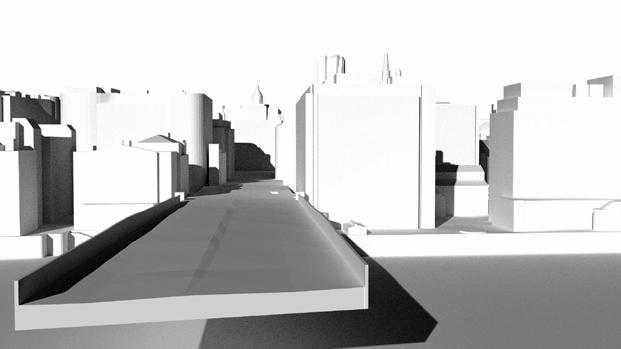
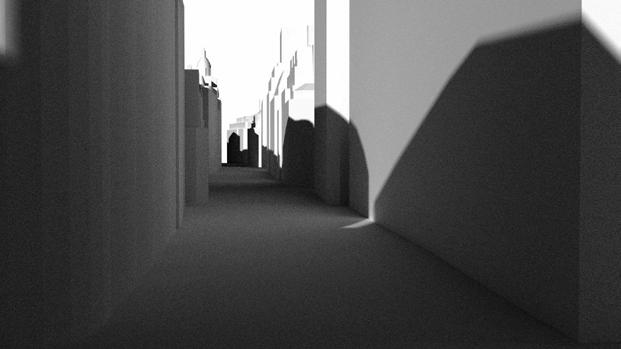
Square Mile-LONDON
500 X 500 meters
Our site is located in the traditional financial centre (Square Mile) of London.
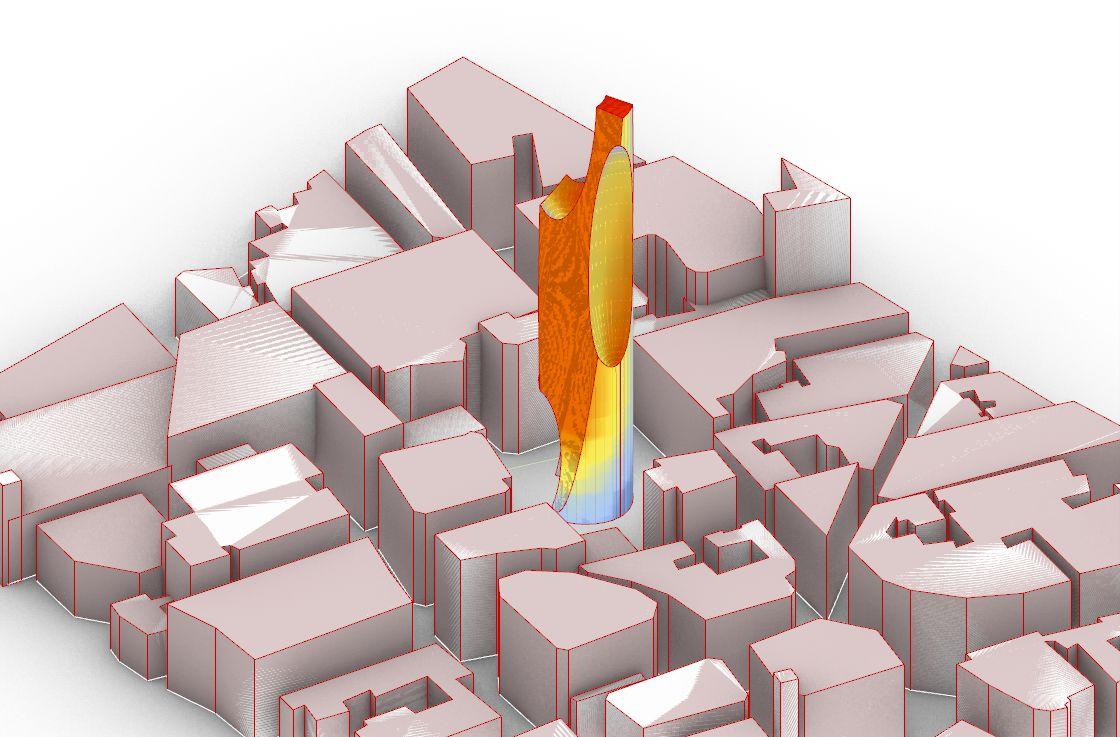
The area is very common by its high density of commuting; workers and visitors at day time.
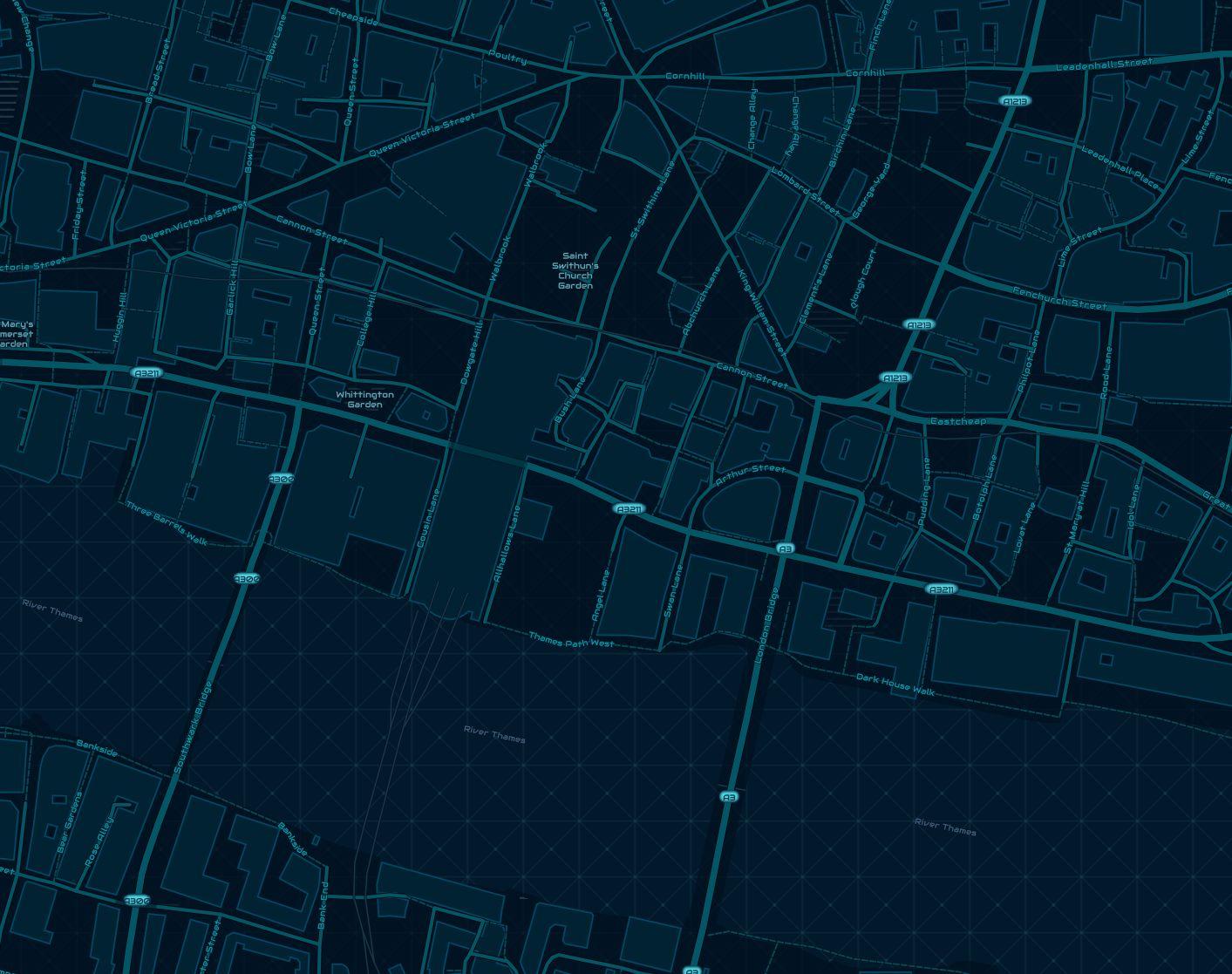
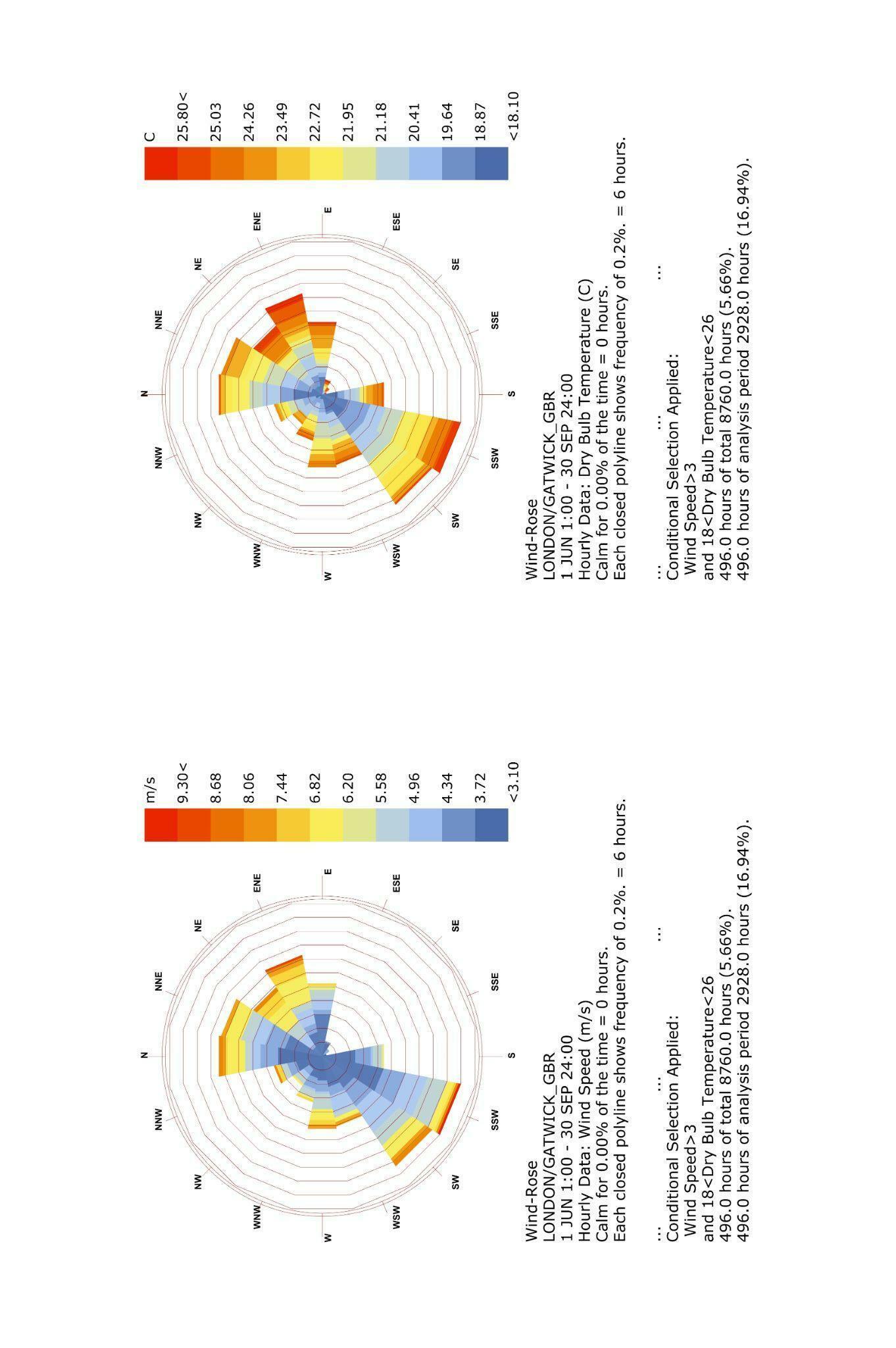
LONDON CLIMATE ANALYSIS
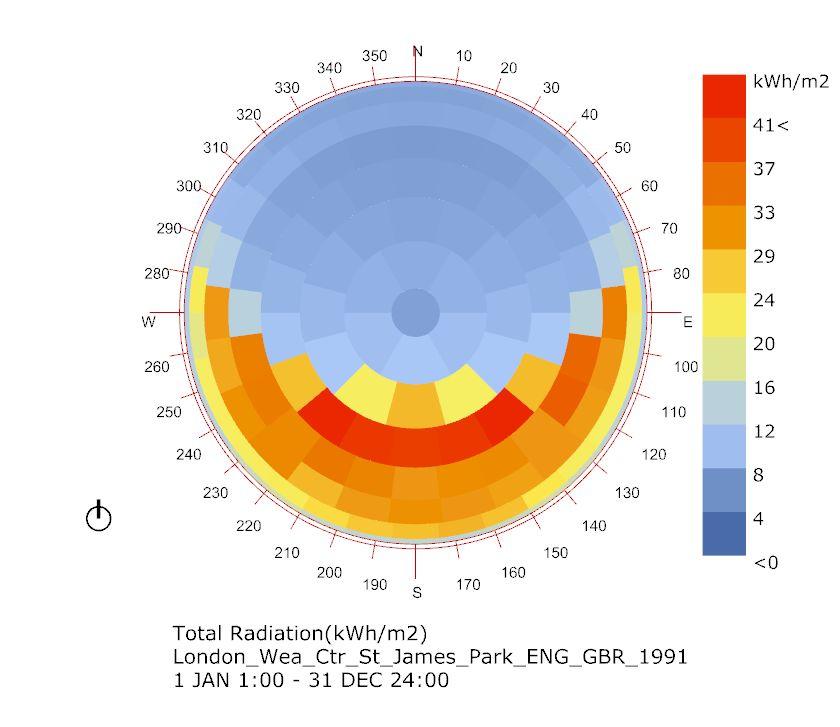
DAYLIGHT SUN PATH VISUALIZATION
The sun path helps to understand potential heating patterns
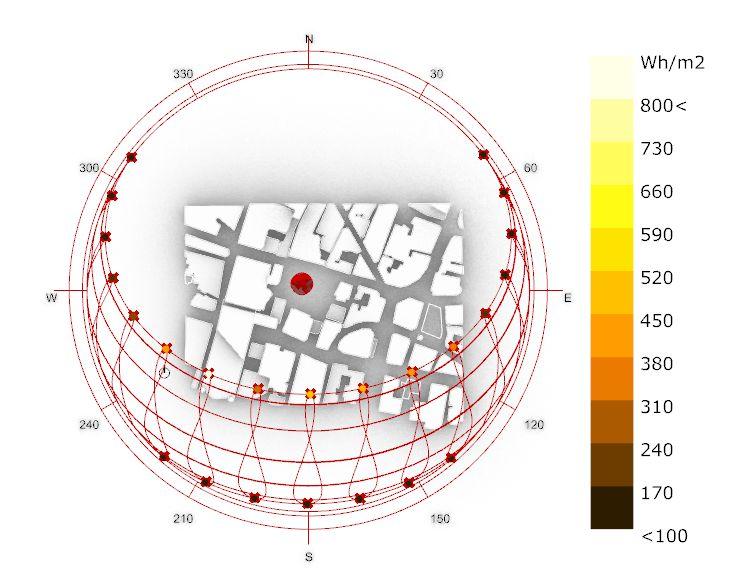
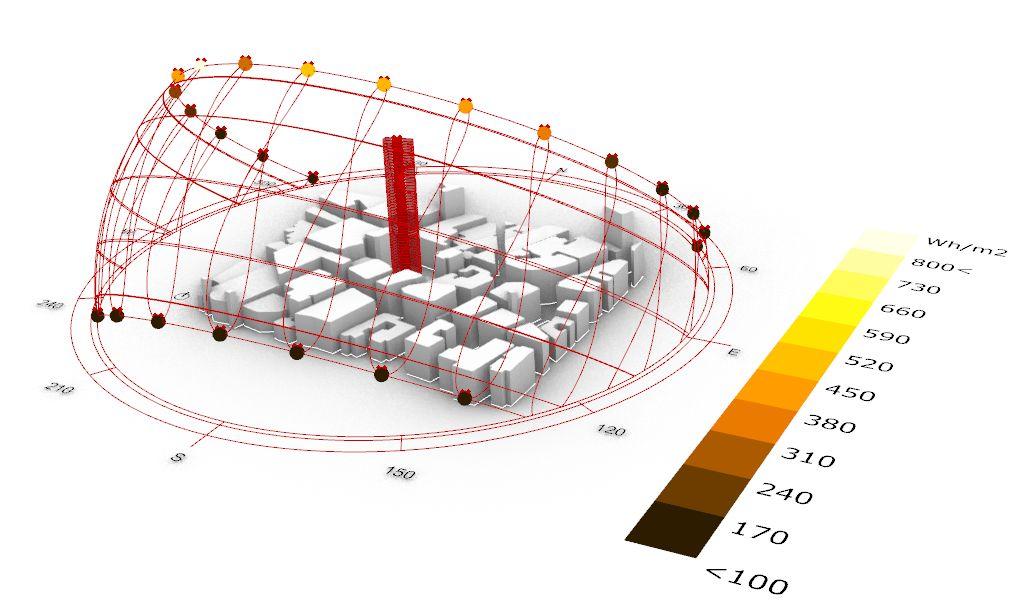
DAYLIGHT
Sun Radiation Study in different Periods
Solar Radiation Annual Period
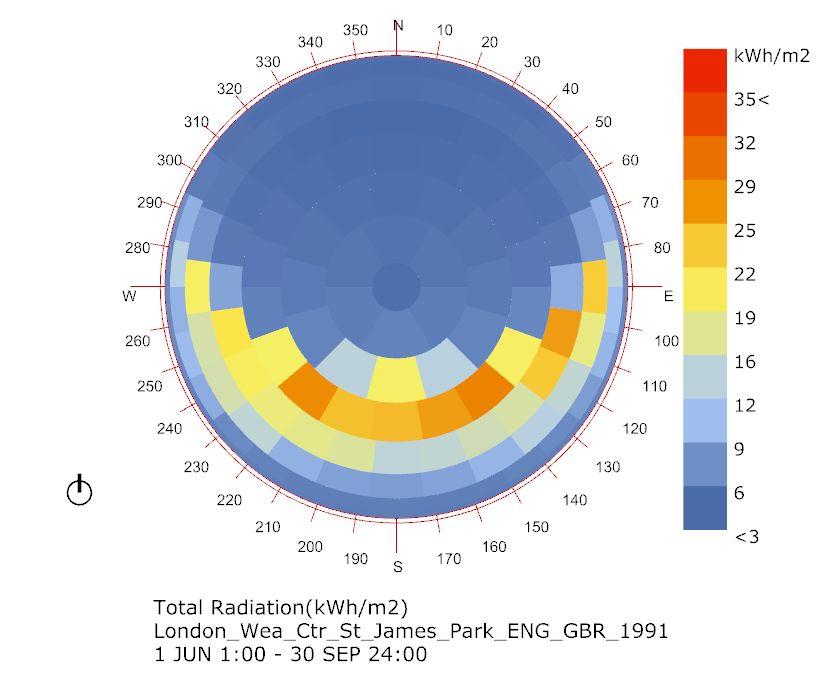
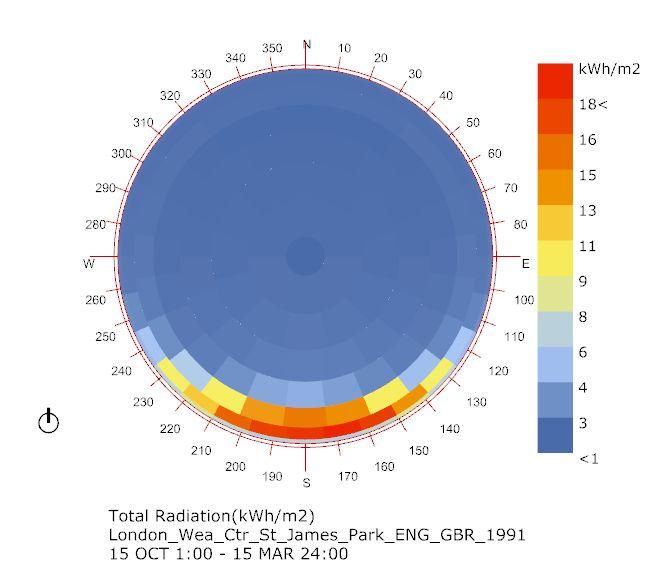
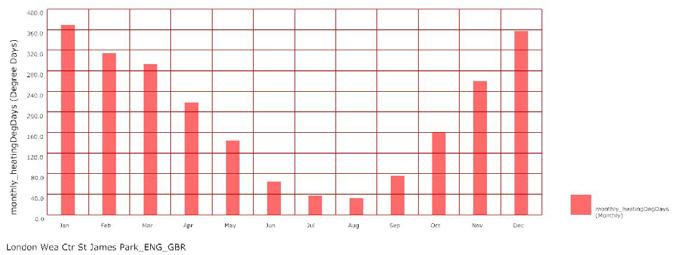
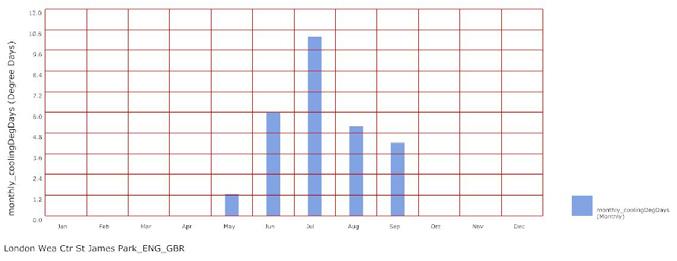
Solar Radiation Cooling Period
Solar Radiation Heating Period
AIR TEMPERATURE



Basic climatic charting/ Hourly
Basic climatic charting/ Hourly
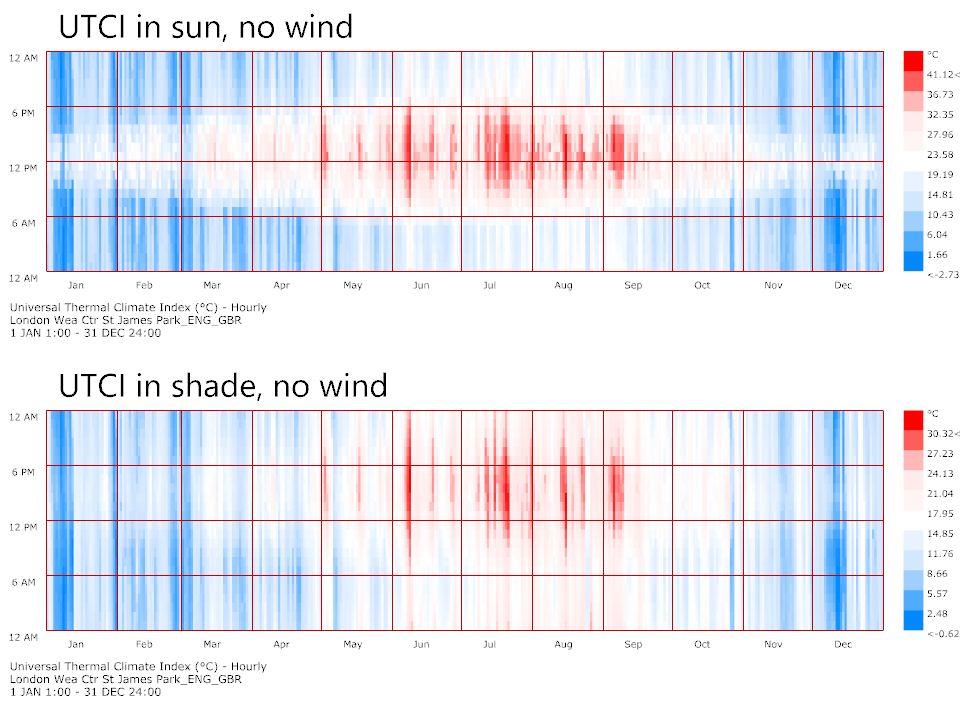
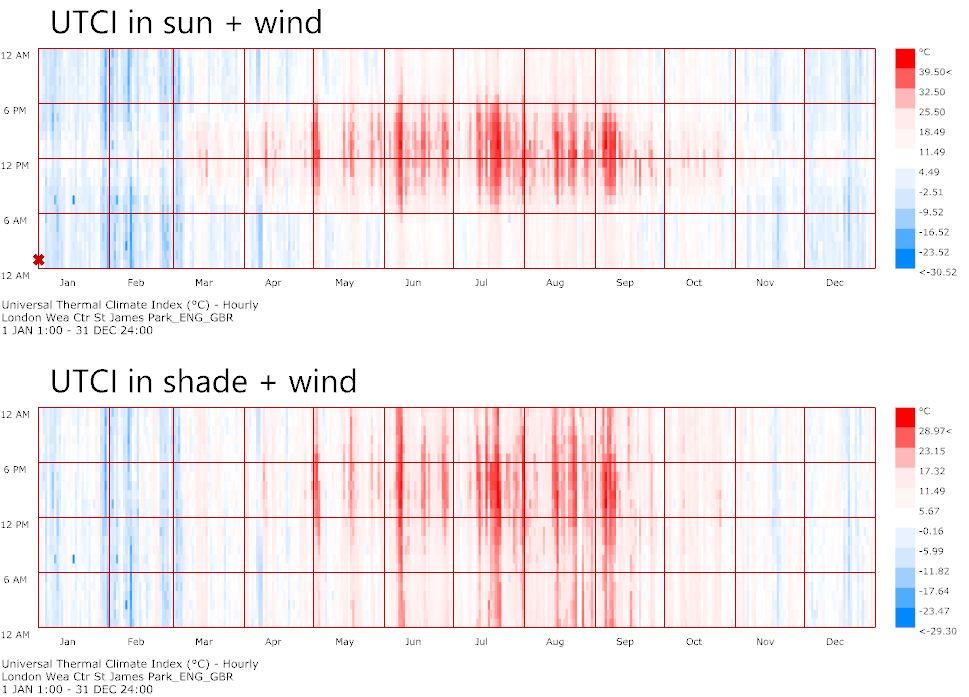
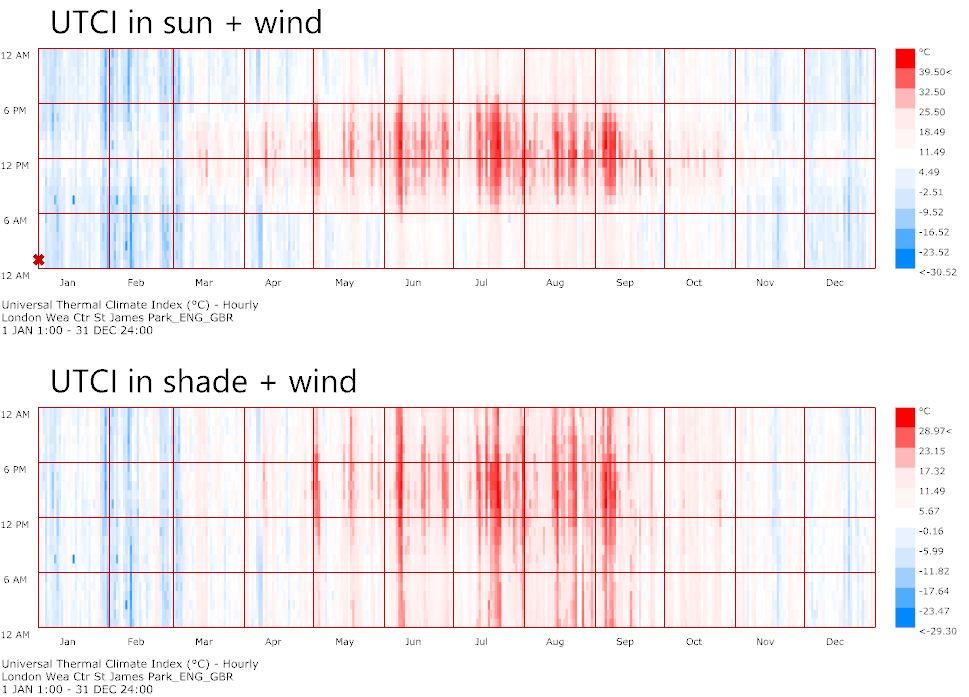
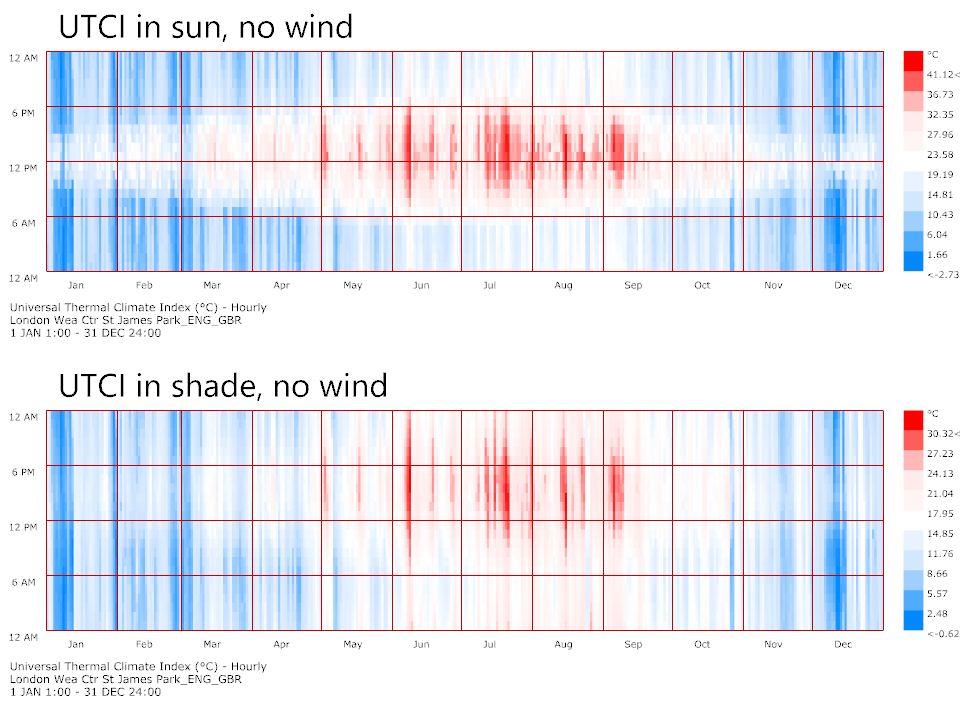


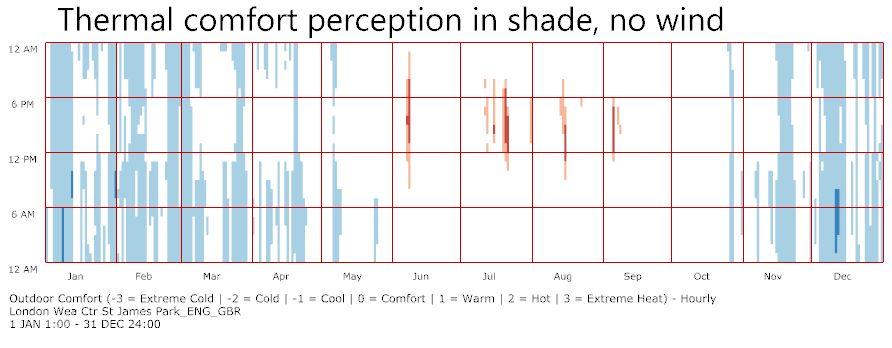
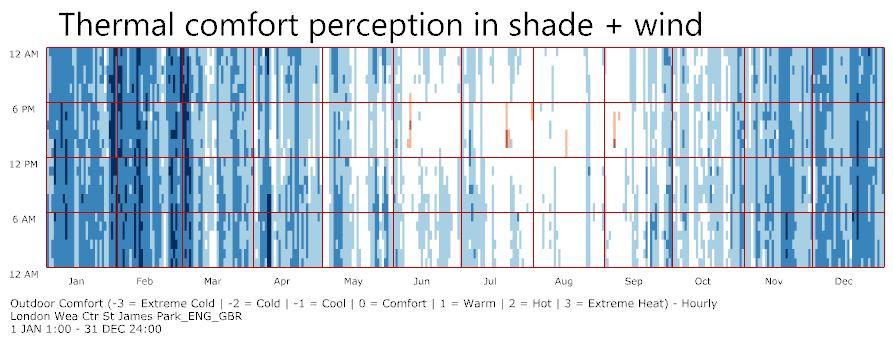

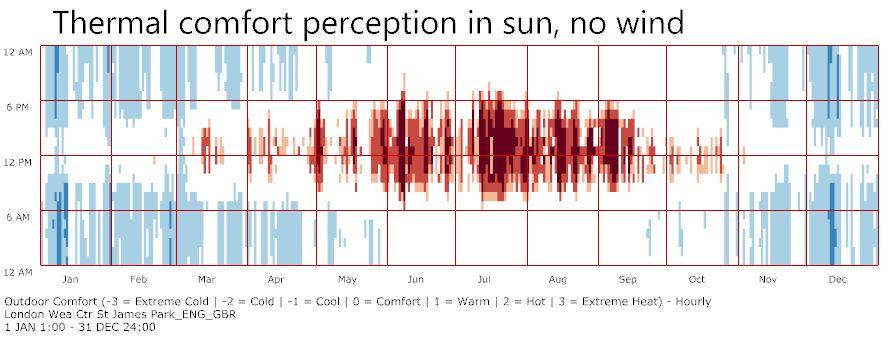
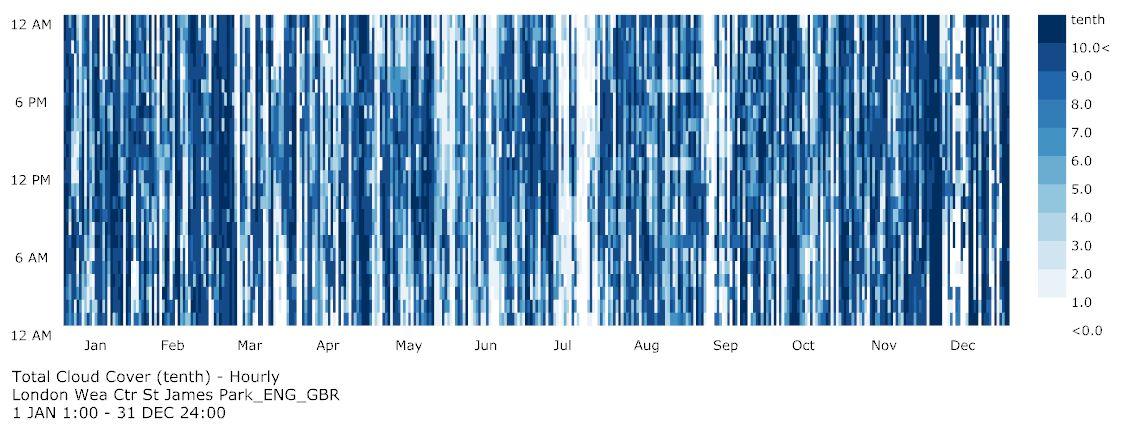
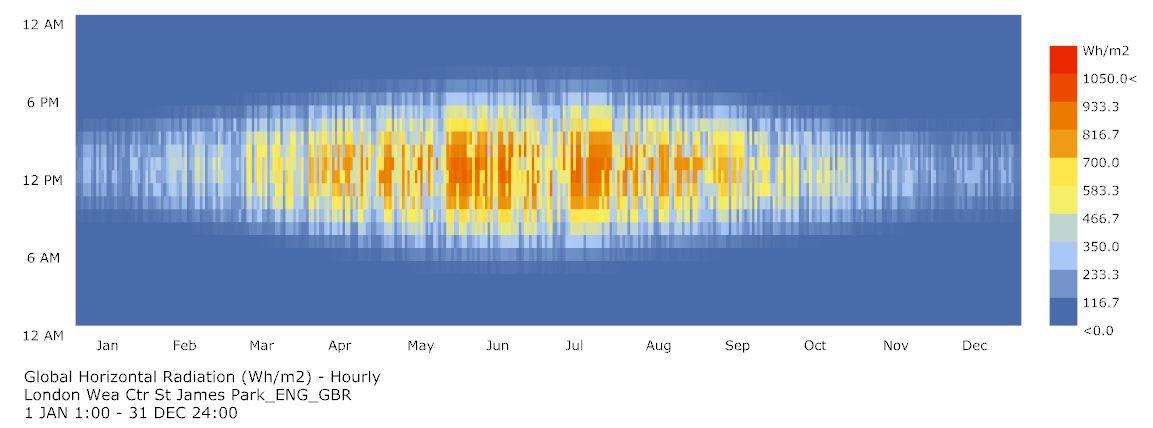
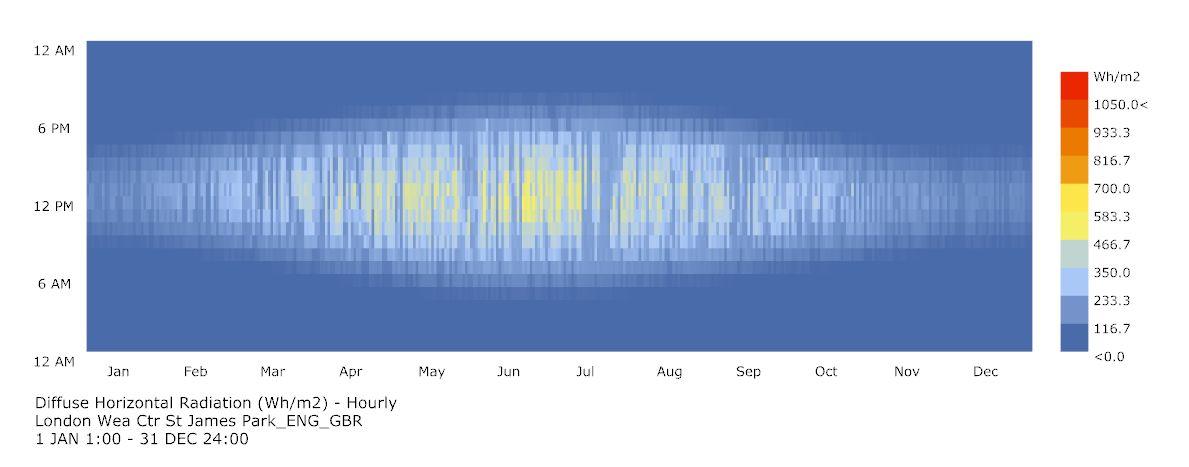
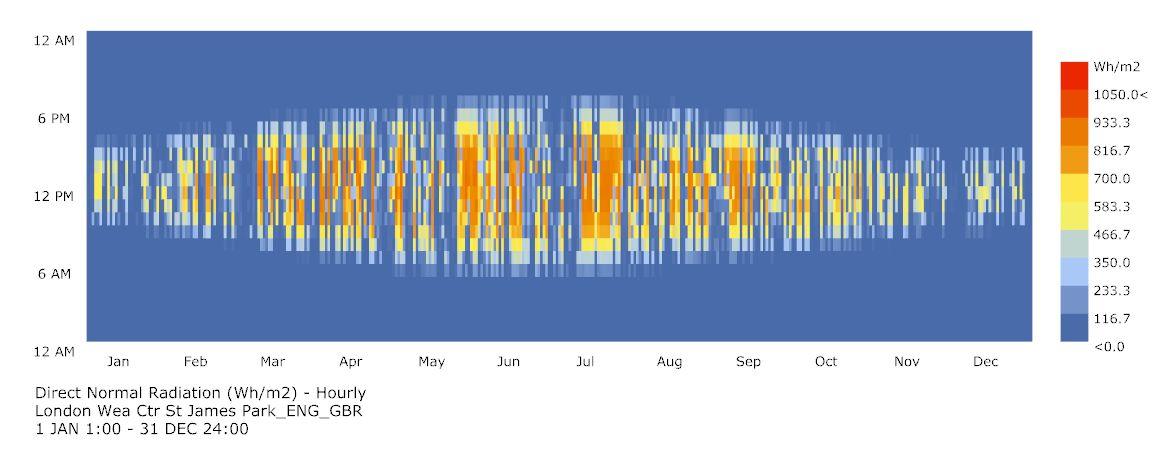
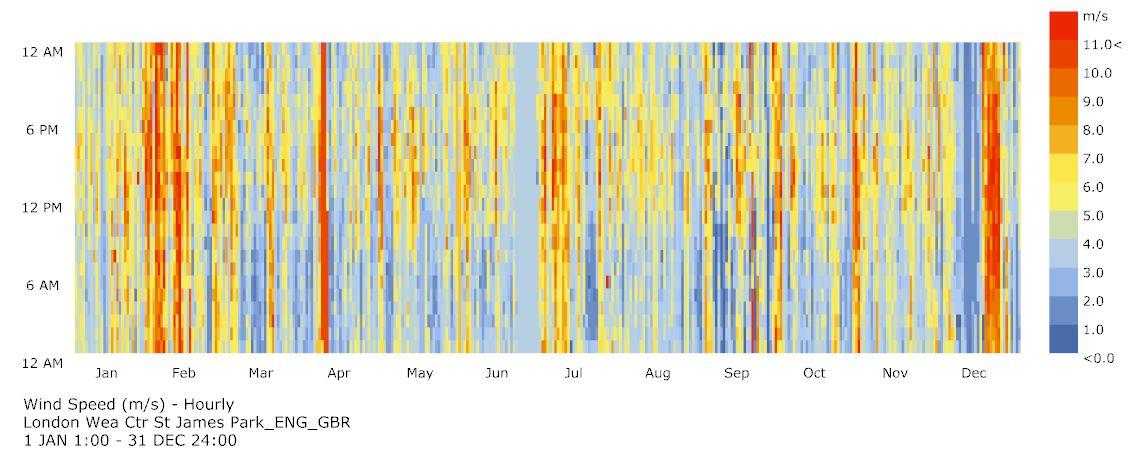
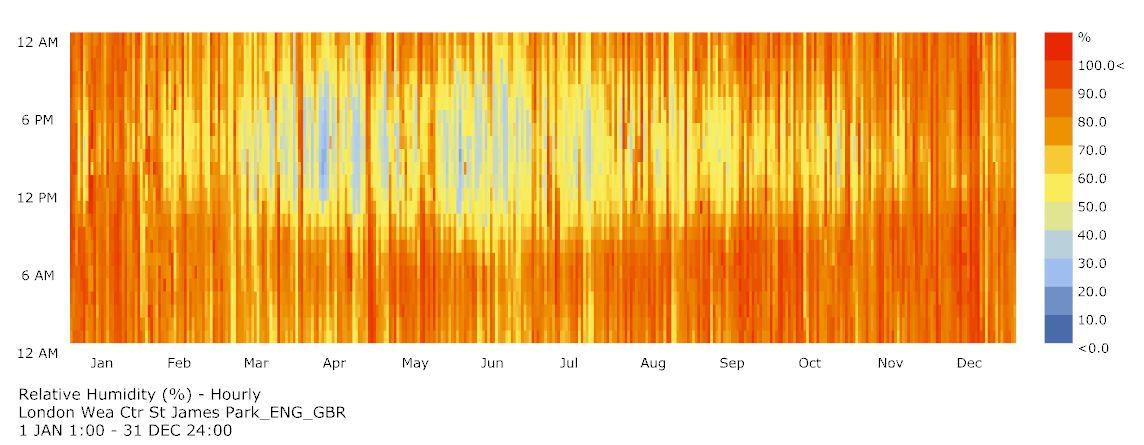
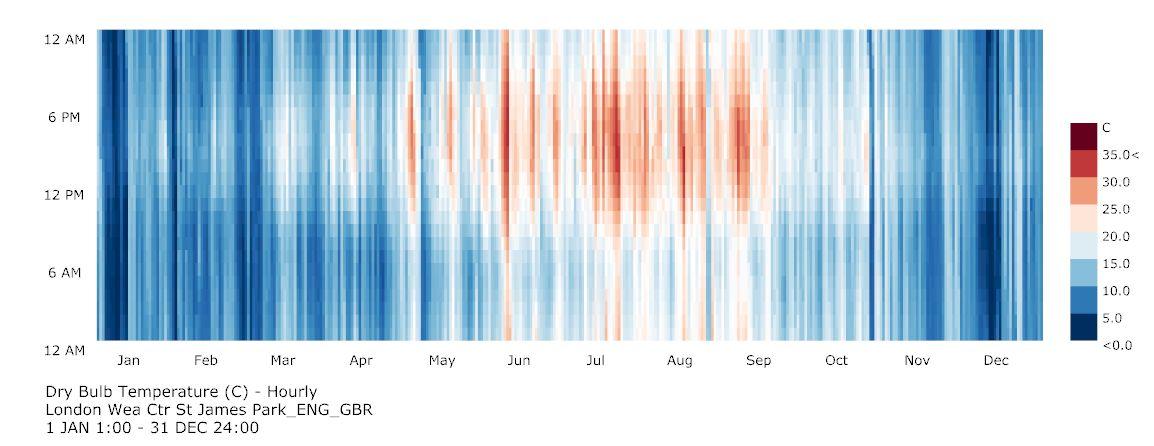
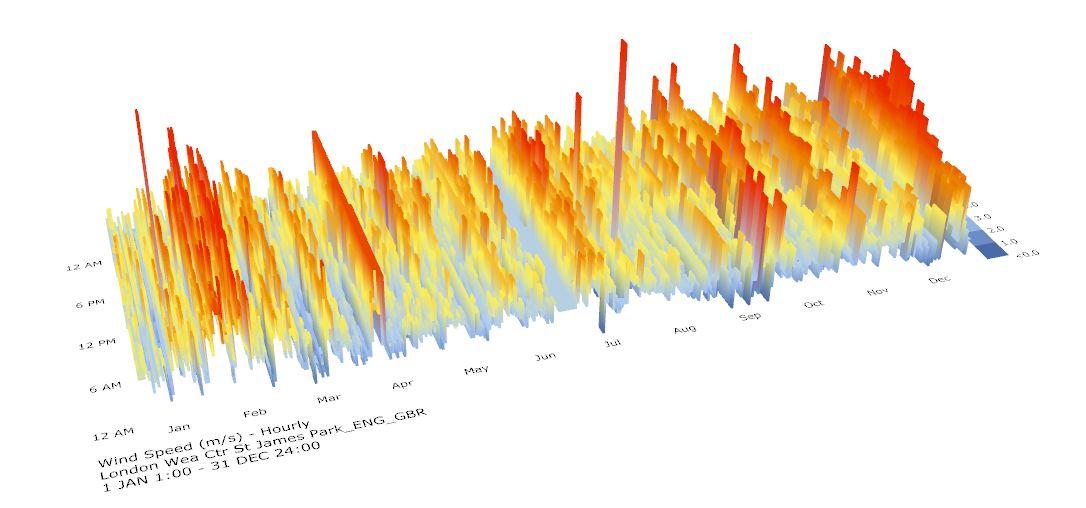
LONDON CLIMATE ANALYSIS
DAYLIGHT SUNLIGHT HOURS SOLSTICES
The maximum solar gains happen at the South West, while the North stays shaded most of the year.
Sunlight-hour analysis for specific day 6th July 15:00

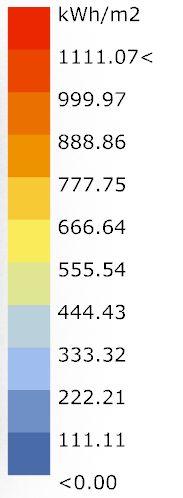
DAYLIGHT SOLAR IRRADIATION SOLSTICES
Sunlight-hour analysis for the winter solstice
Sunlight-hour analysis for the summer solstice
In the building geometry it shows that the top part of the tower receives higher solar irradiation compare to the bottom floors
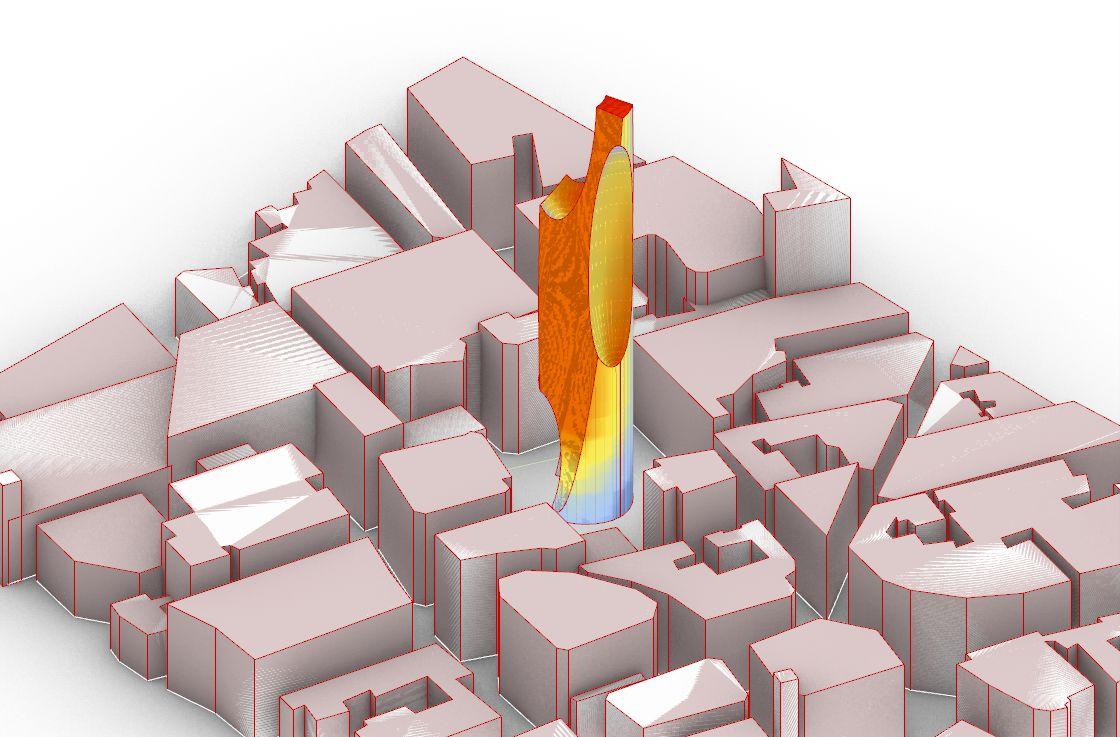
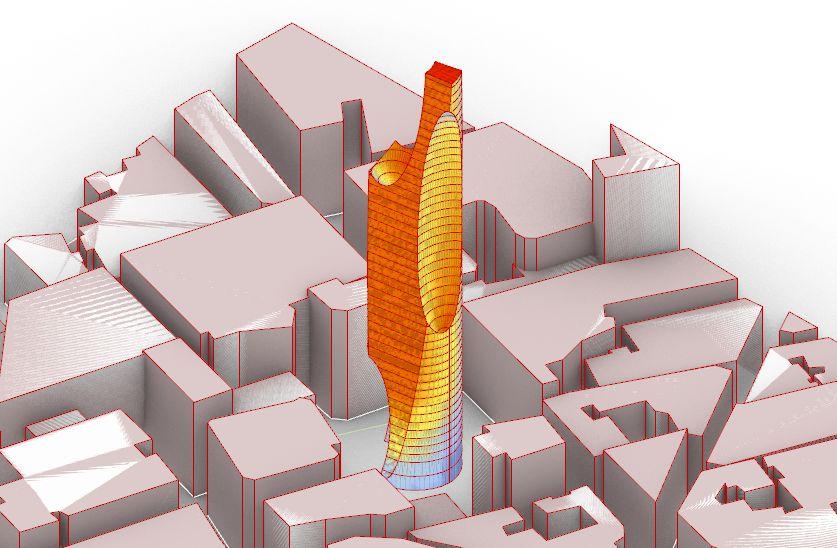
Irradiation analysis for Massing and site
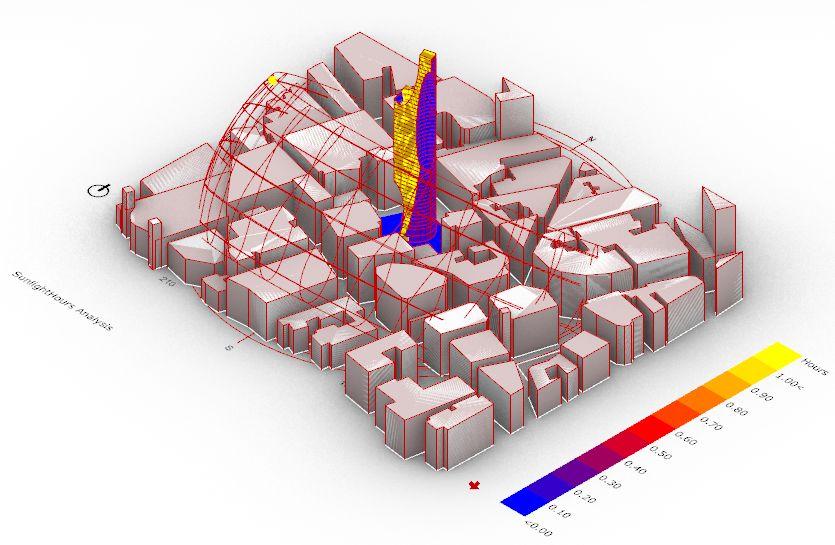
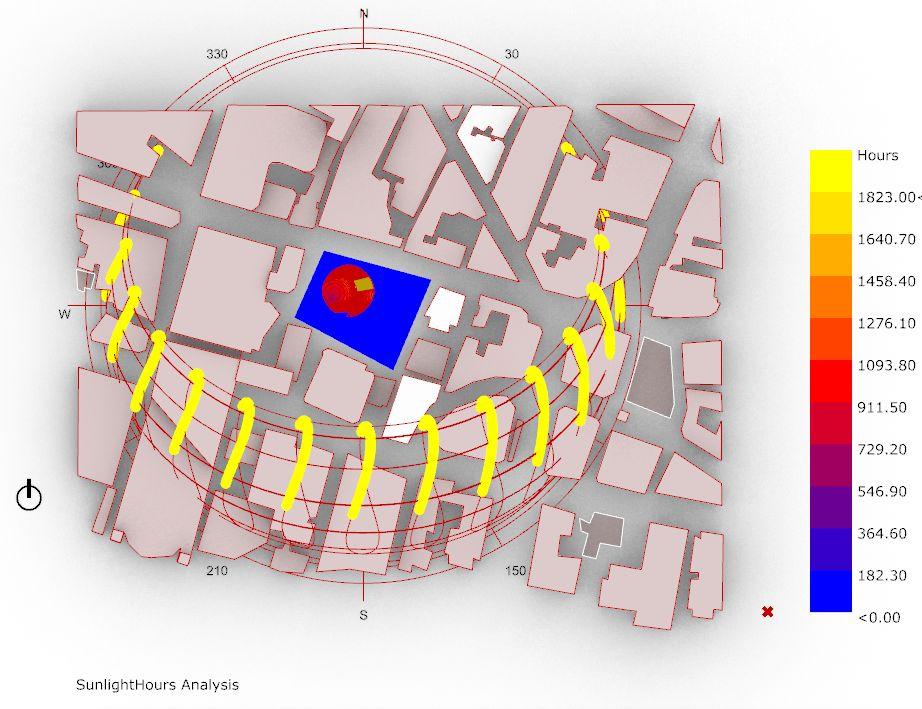
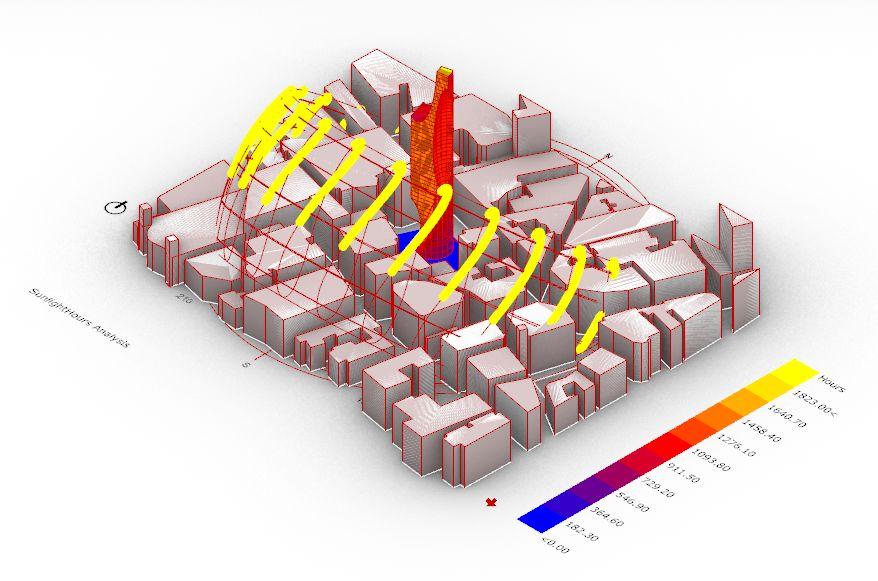
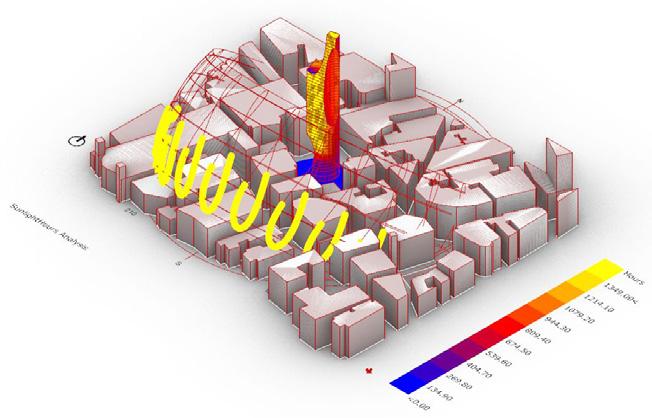
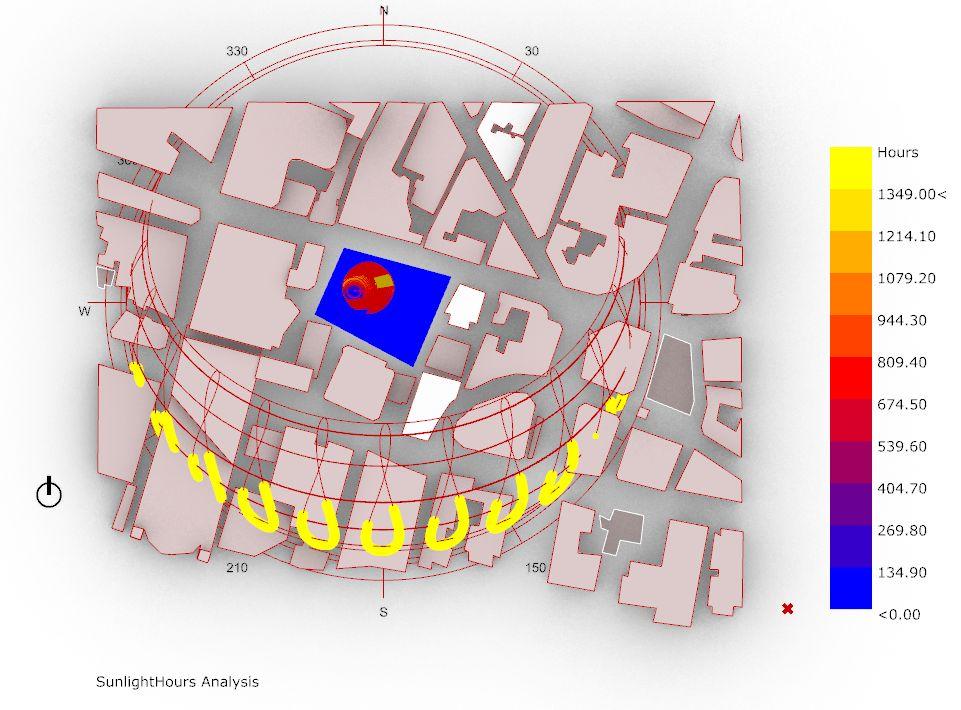
Irradiation analysis for Massing
Irradiation analysis for windows simulation
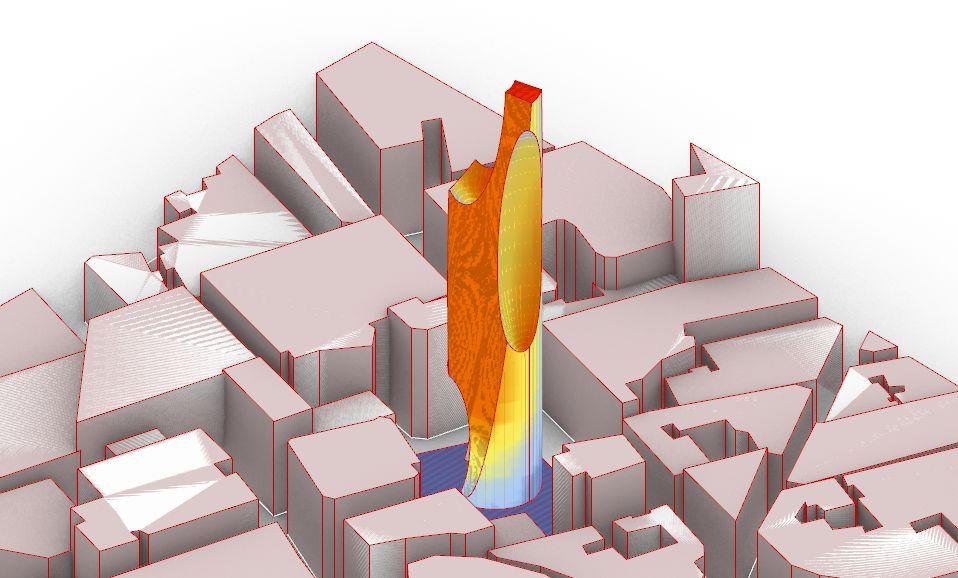
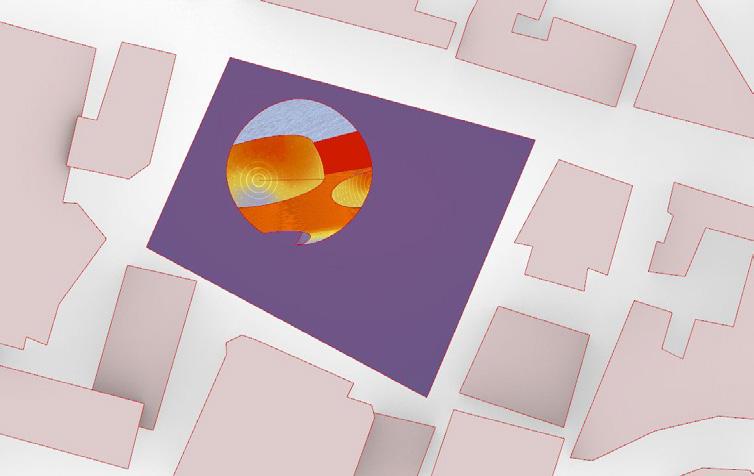
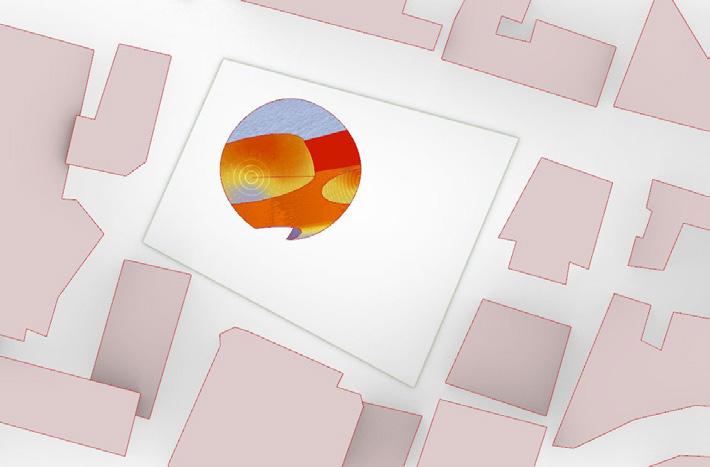
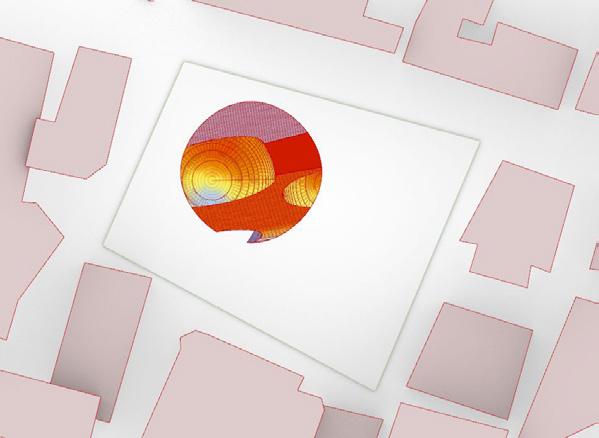
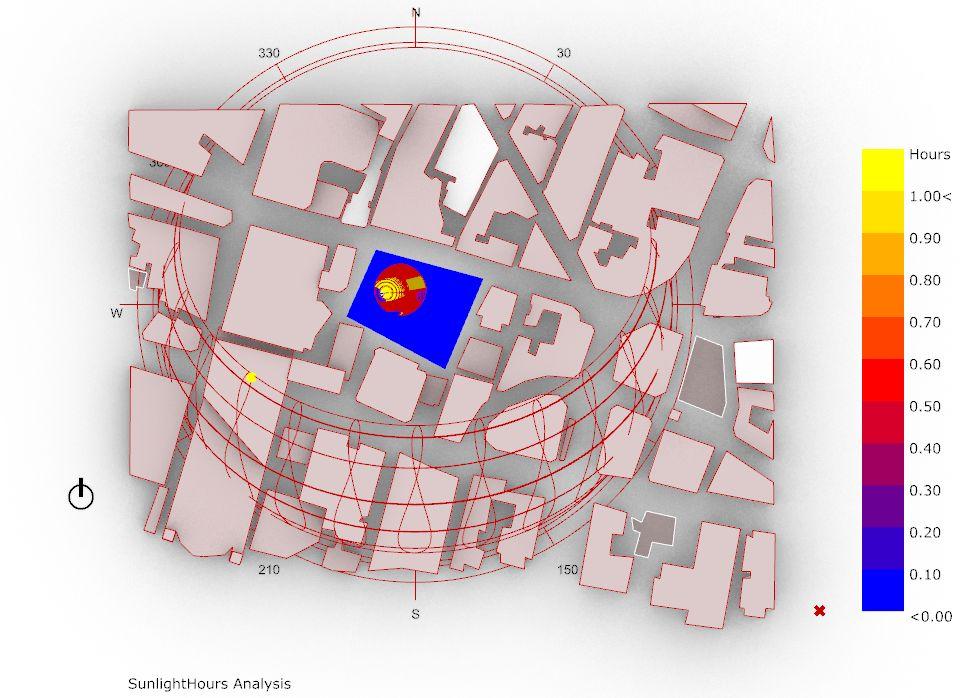
DAYLIGHT INSOLATION
Insolation analysis for Massing and site
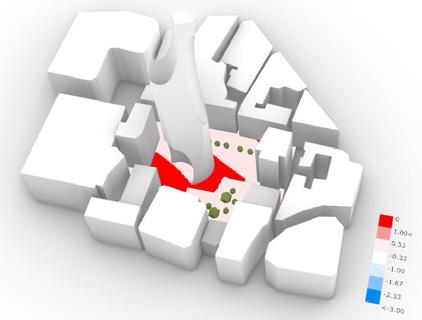
Low angle radiation especially in winter could cause potential glare issues.
THERMAL COMFORT - UTCI PERCEPTION
15th of Aug.
9:00 am 15:00 am 12:00 a m 18:00 am
THERMAL COMFORT - UTCI PERCEPTION
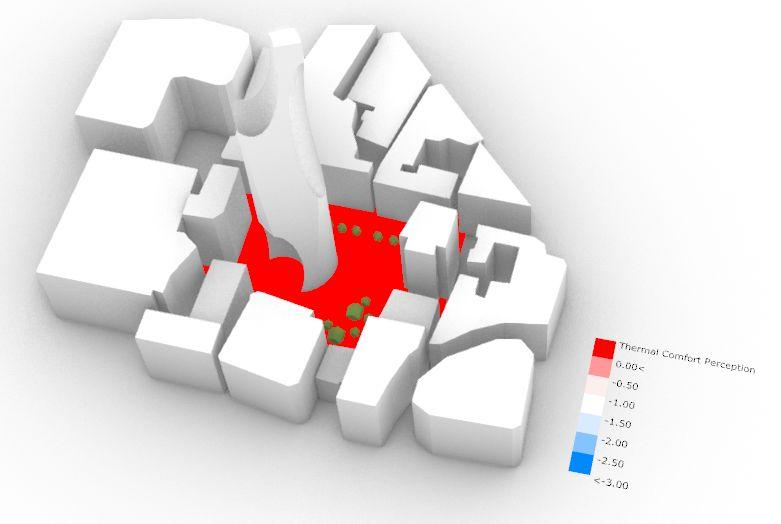
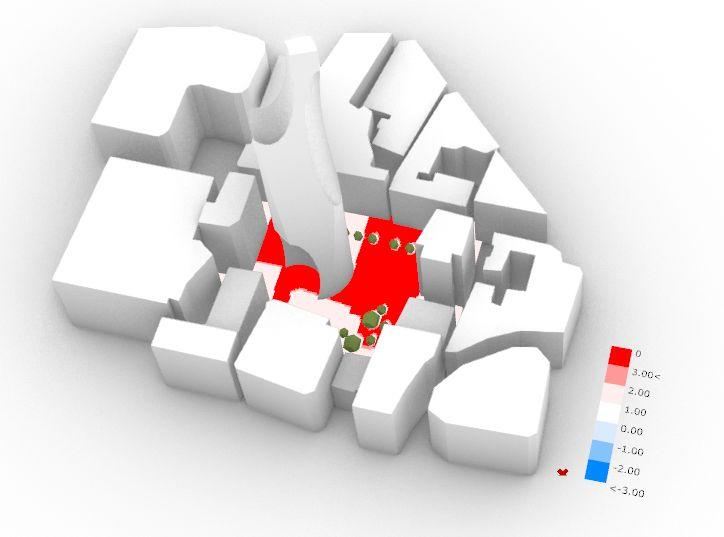
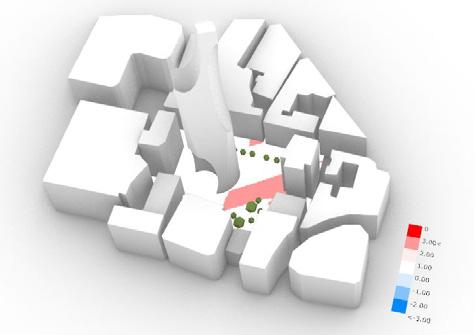
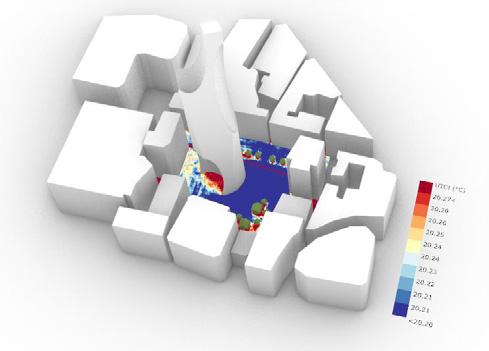
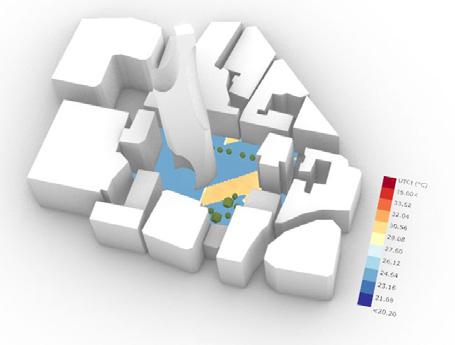
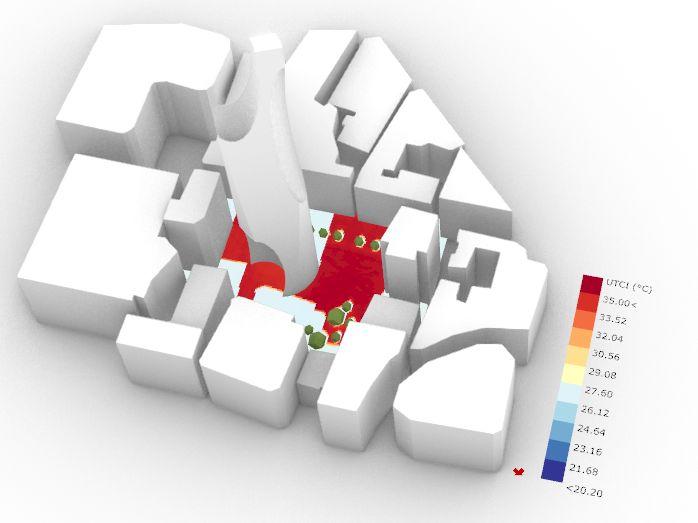
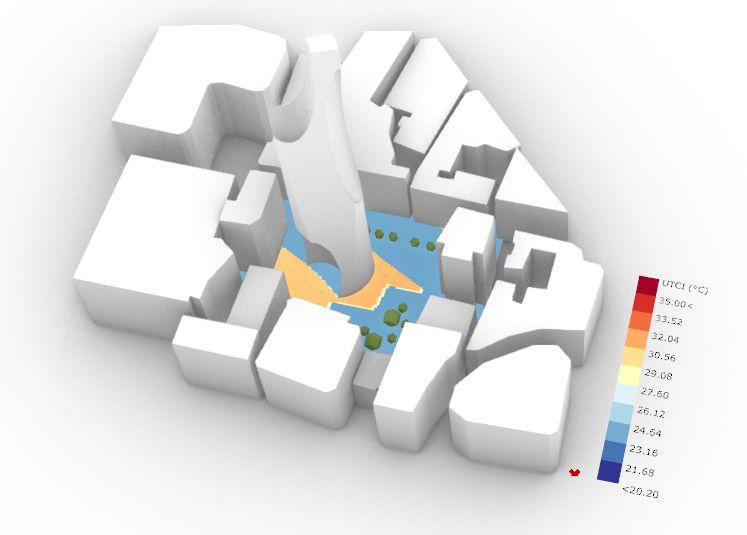
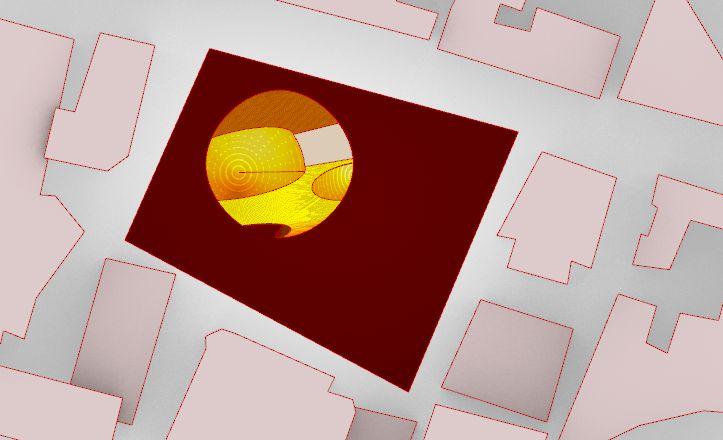
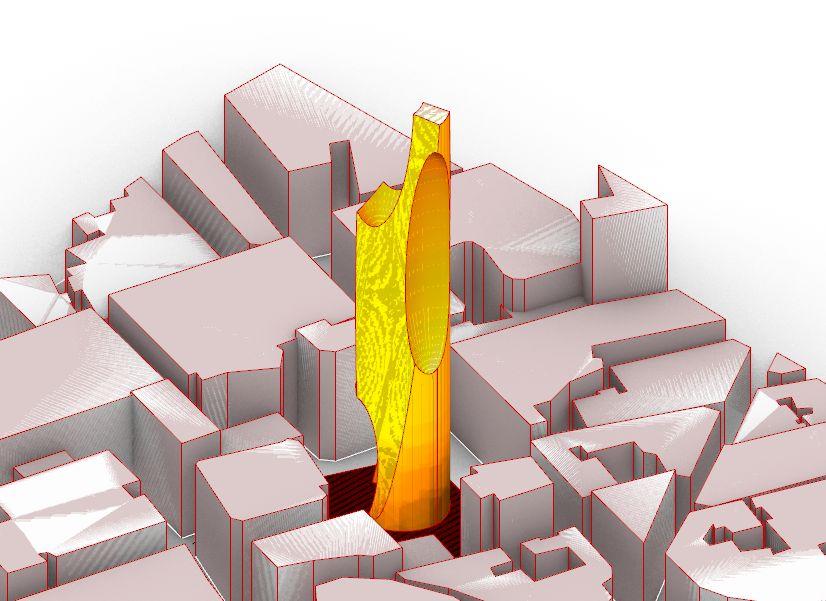
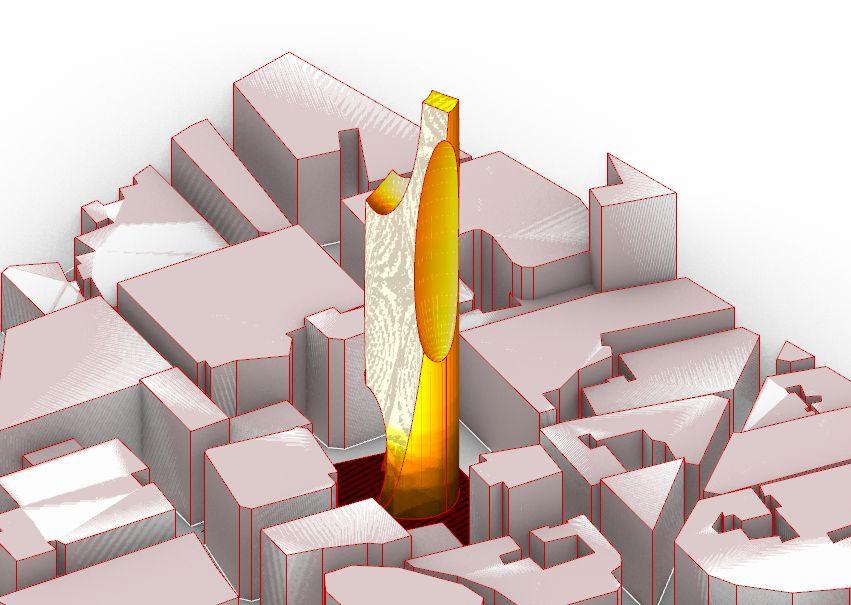
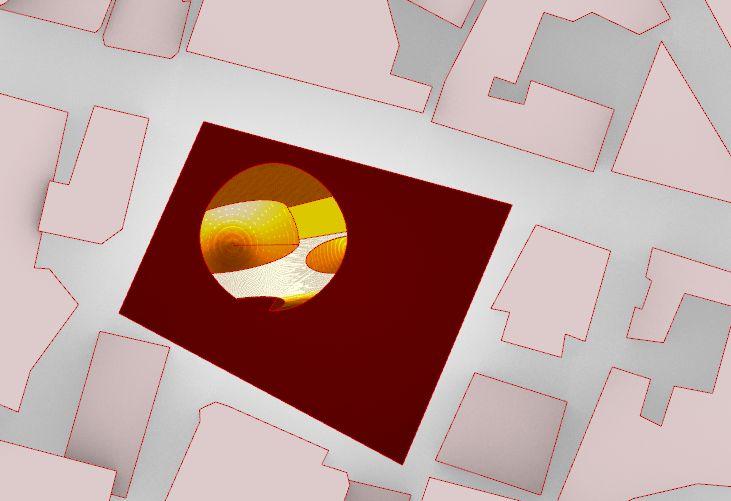
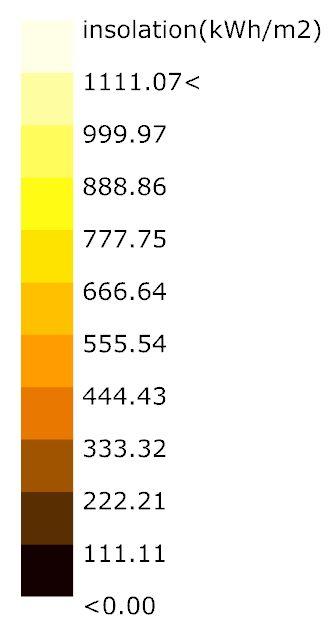
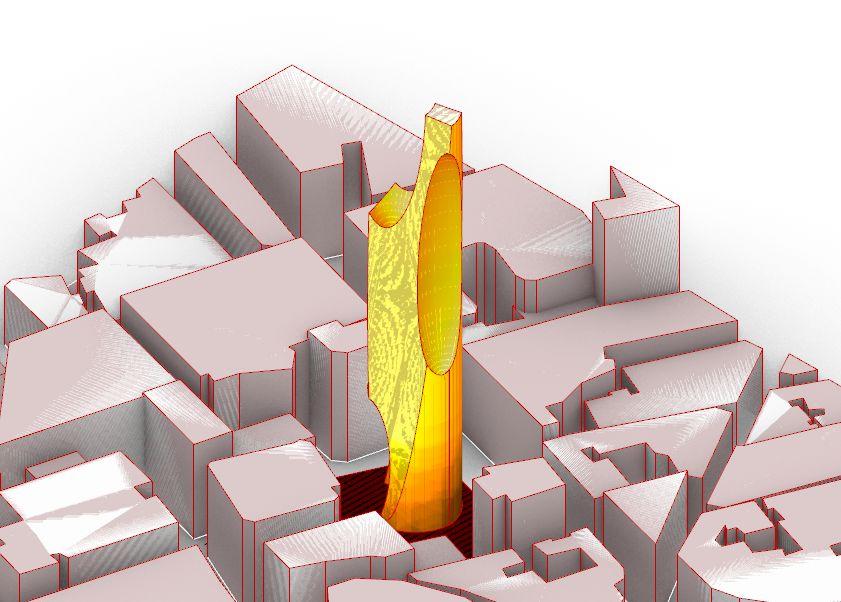
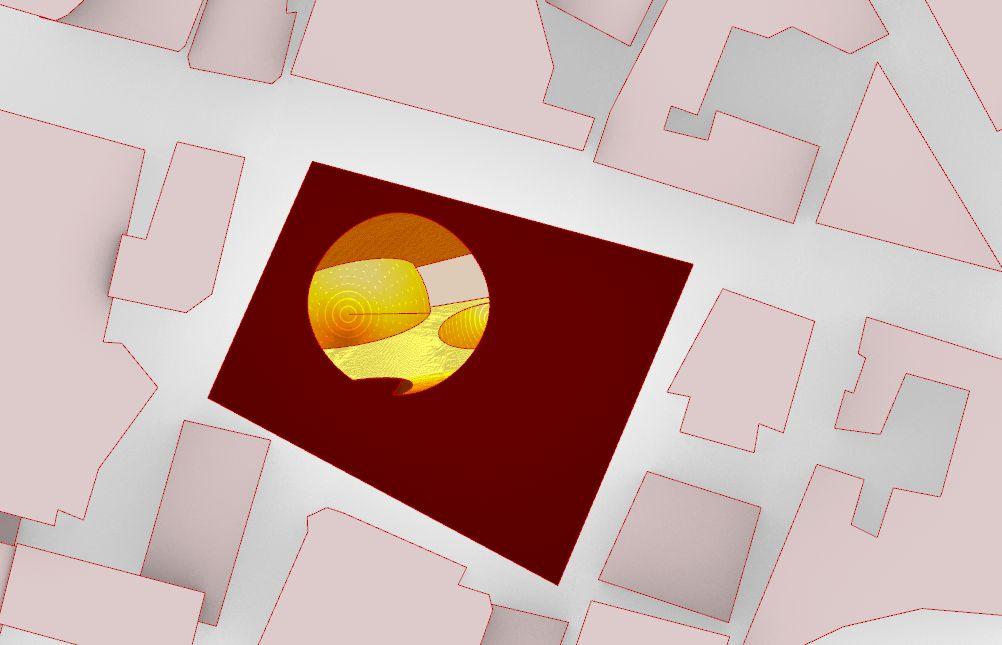
15th of Aug.
9:00 am 12:00 am 15:00 am 18:00 am
LONDON CLIMATE ANALYSIS
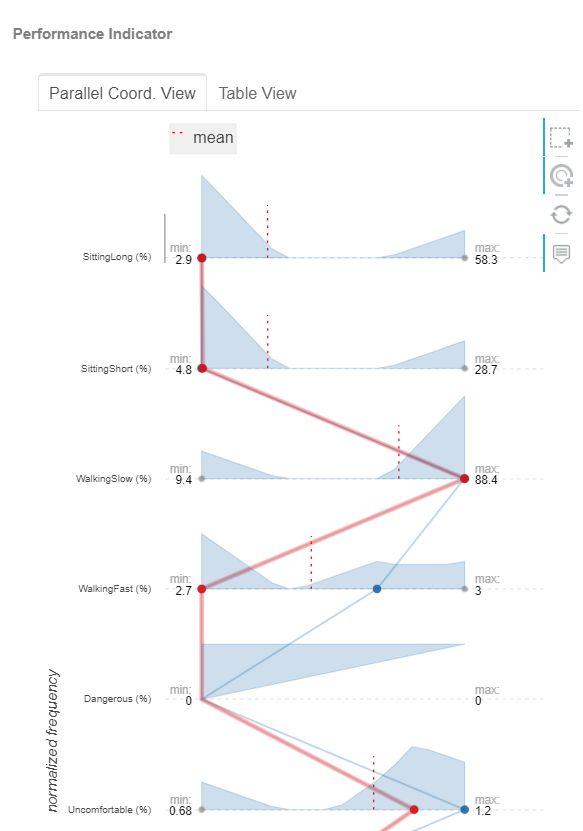
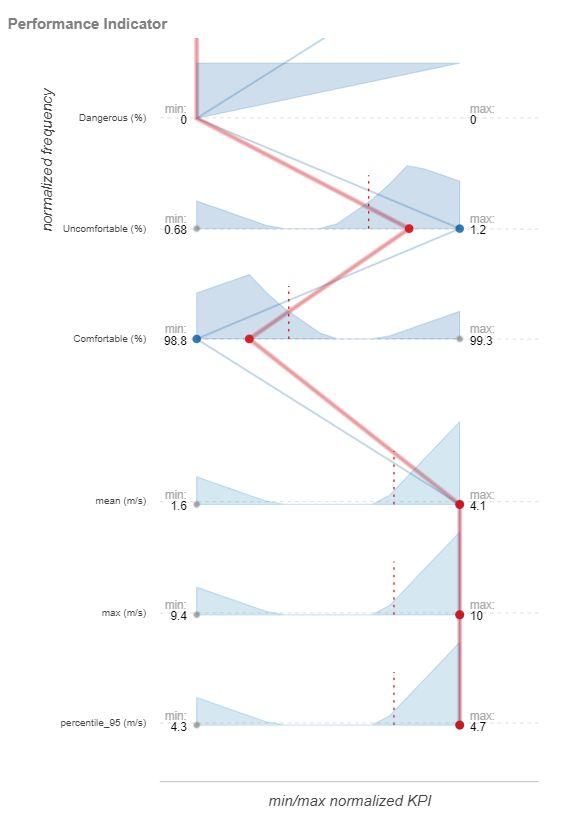
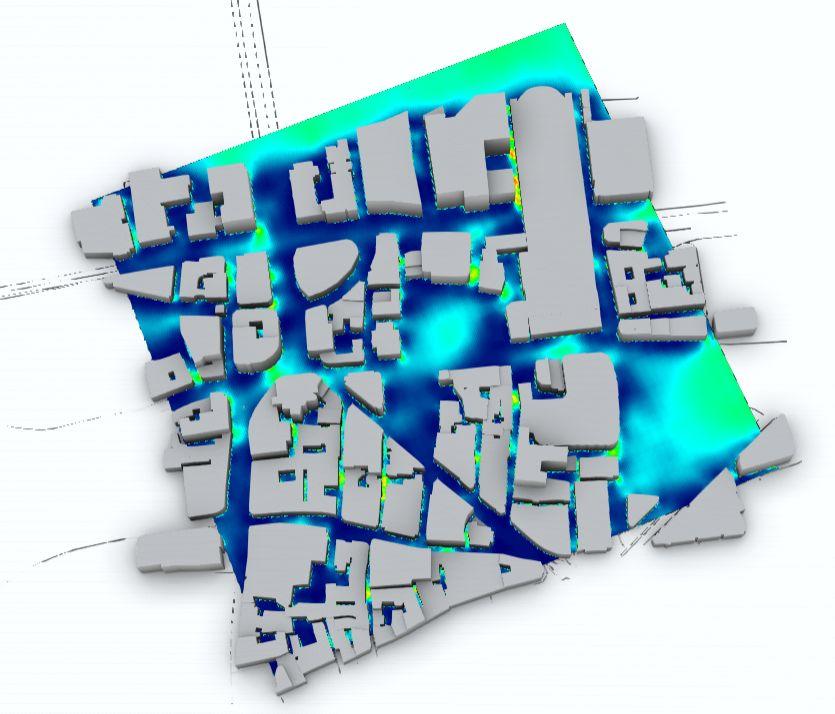
Wind Speed
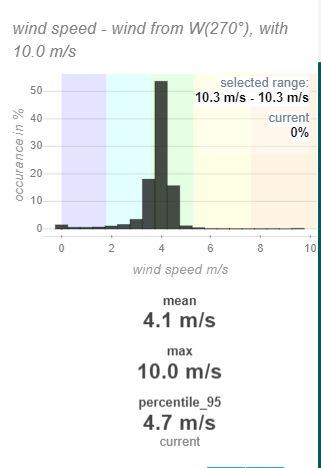
InFraRed Project Name: Tower Root: Origin Site
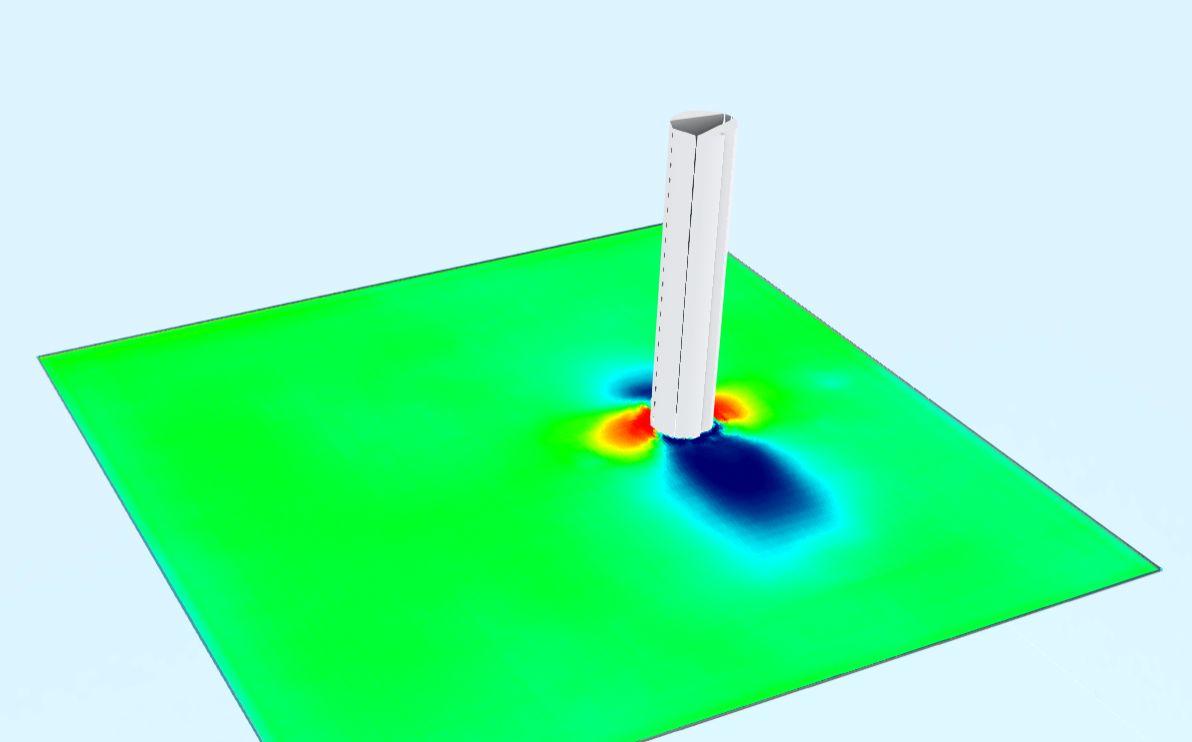
Wind Speed Comparison
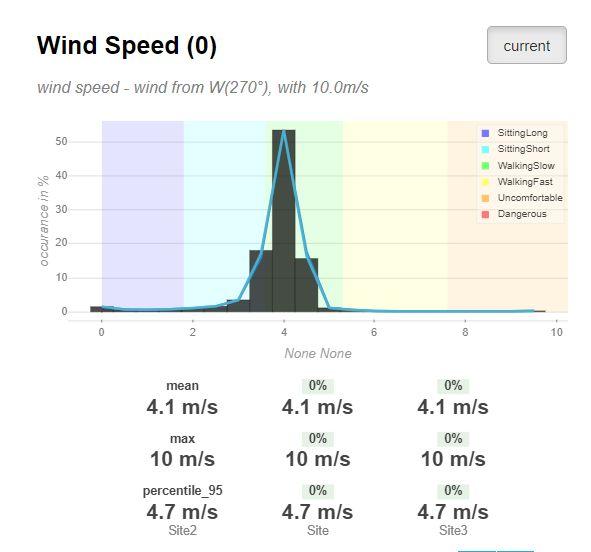
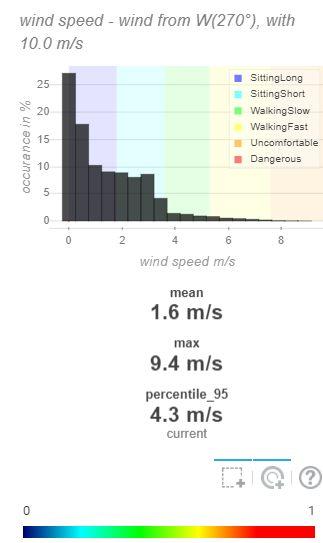
Wind Comfort
InFraRed
Project Name: Tower Root: Origin
Wind Comfort Comparison
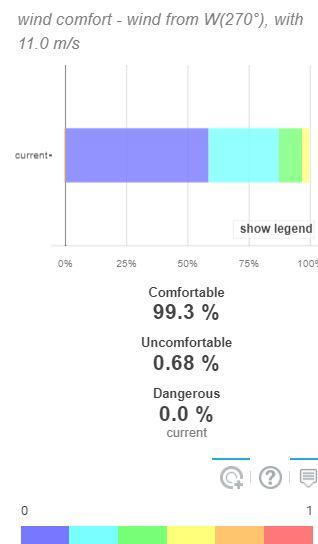
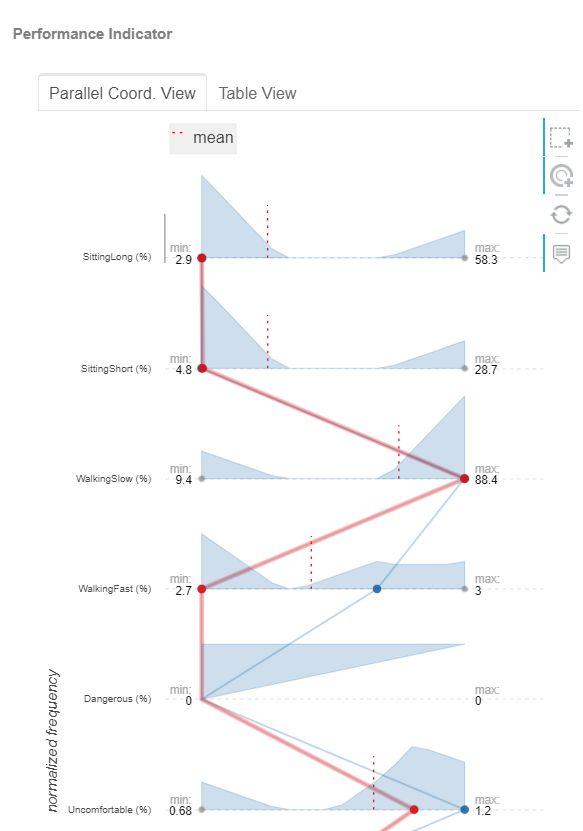
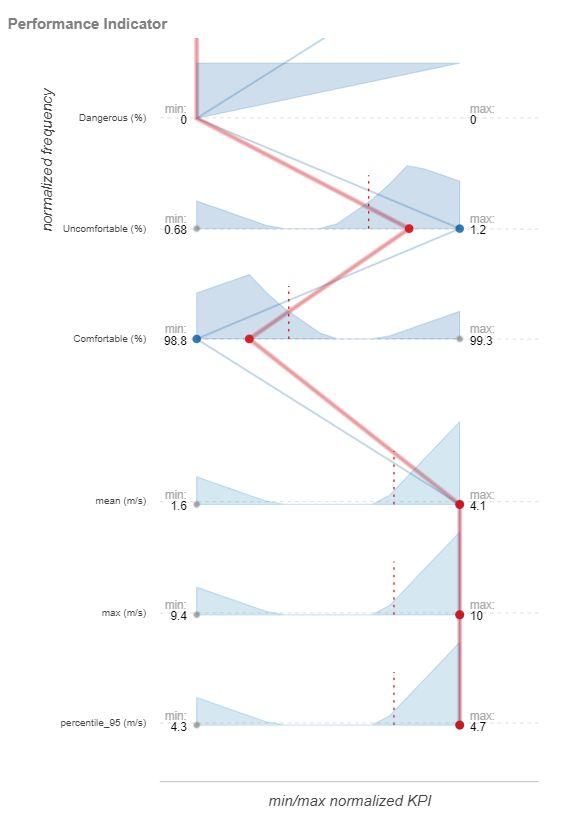
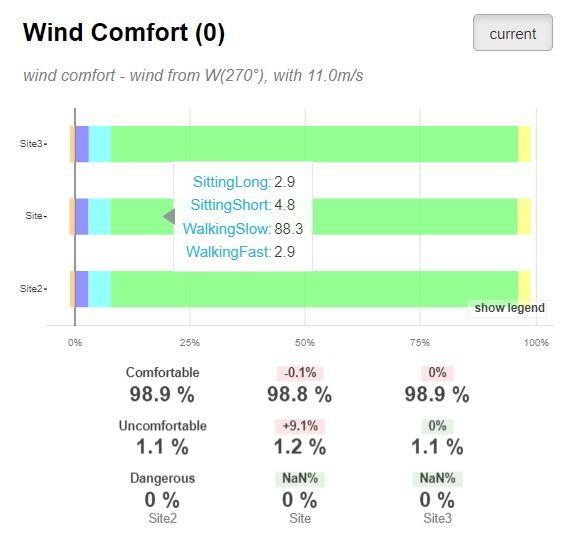
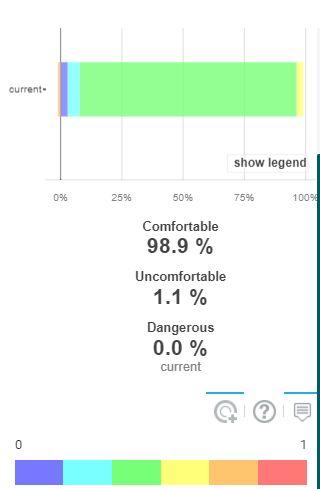
The predominant wind is usually comes from the west.
We tried to rotate the main concave entrance of the tower in three different direction to examine the outdoor wind comfort.
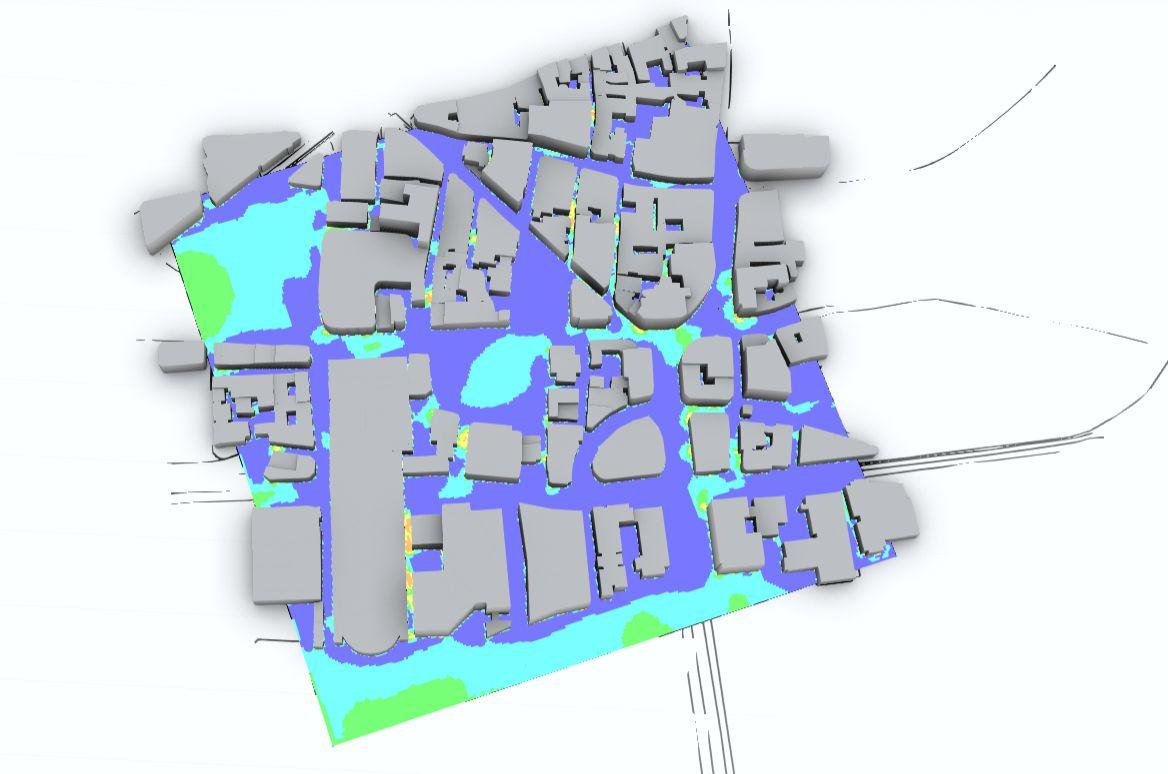
but the result was almost the same cause the tower takes a circular shape.
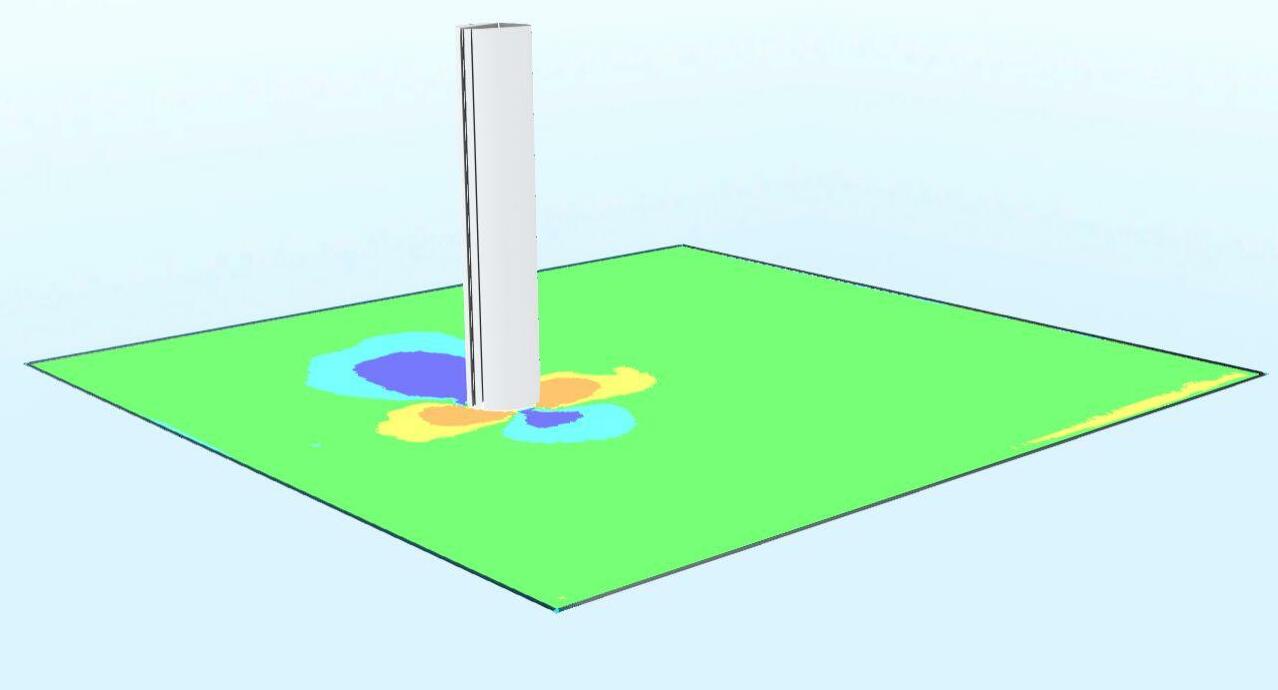
WHAT IS MY PLAZA FOR?
15
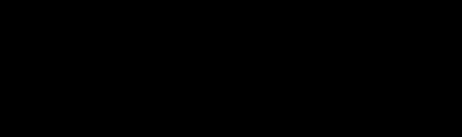
Date: 2022
Location: Madrid, Spain
Collaborators: Jumana Hamdani Lucía Leva, Pablo Antuña Molina
Instructor: Gabriella Rossi
Project Type: Academic at IAAC
Tools: Rhino,Grasshopper, Python, Google Colab, Open Street Maps, Self Organizing MapsKohonen Maps, Hops, Shallow Learning, ANN Learning, PCA, EDA, Classification Machine Learning model

WHAT IS MY PLAZA FOR?
What is my plaza for? aims to predict the possibility that a given event will occur in a urban plaza according to different factors associated with the features of the public space.
Based on traditional urbanism indicators, a critical analysis is carried out through a machine learning process to evaluate the resilience of the spaces designed for the community.
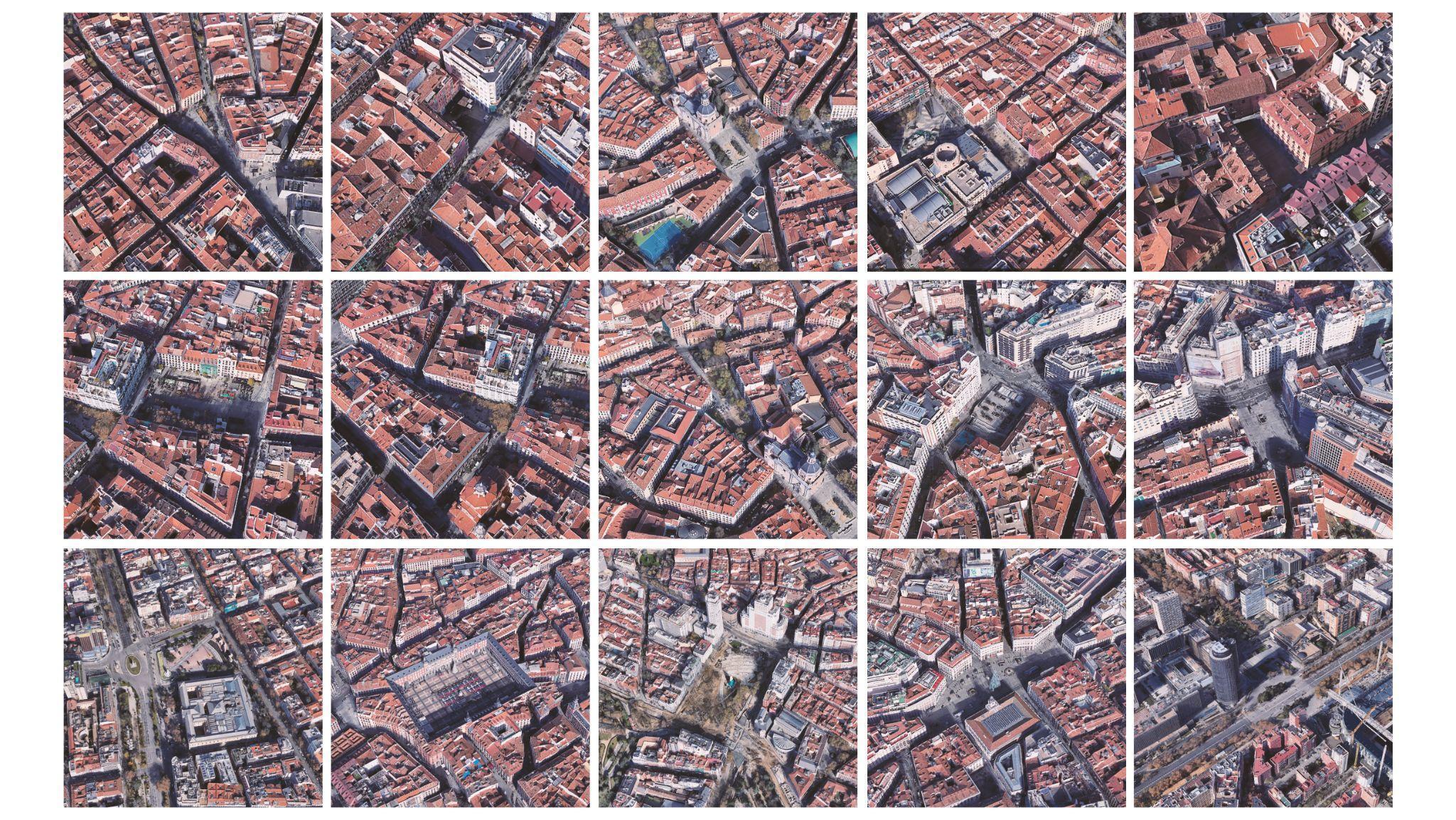
WHAT IS MY PLAZA FOR?
GEOMETRY GENERATION
# BIG PLAZAS big1 = (40.4248508, -3.6890901553611517)
big1l = 'b1_plazacolon' big2 = (40.4231703, -3.7125722)
big2l = 'b2_plazaespana' big3 = (40.415392, -3.7073743182788528)
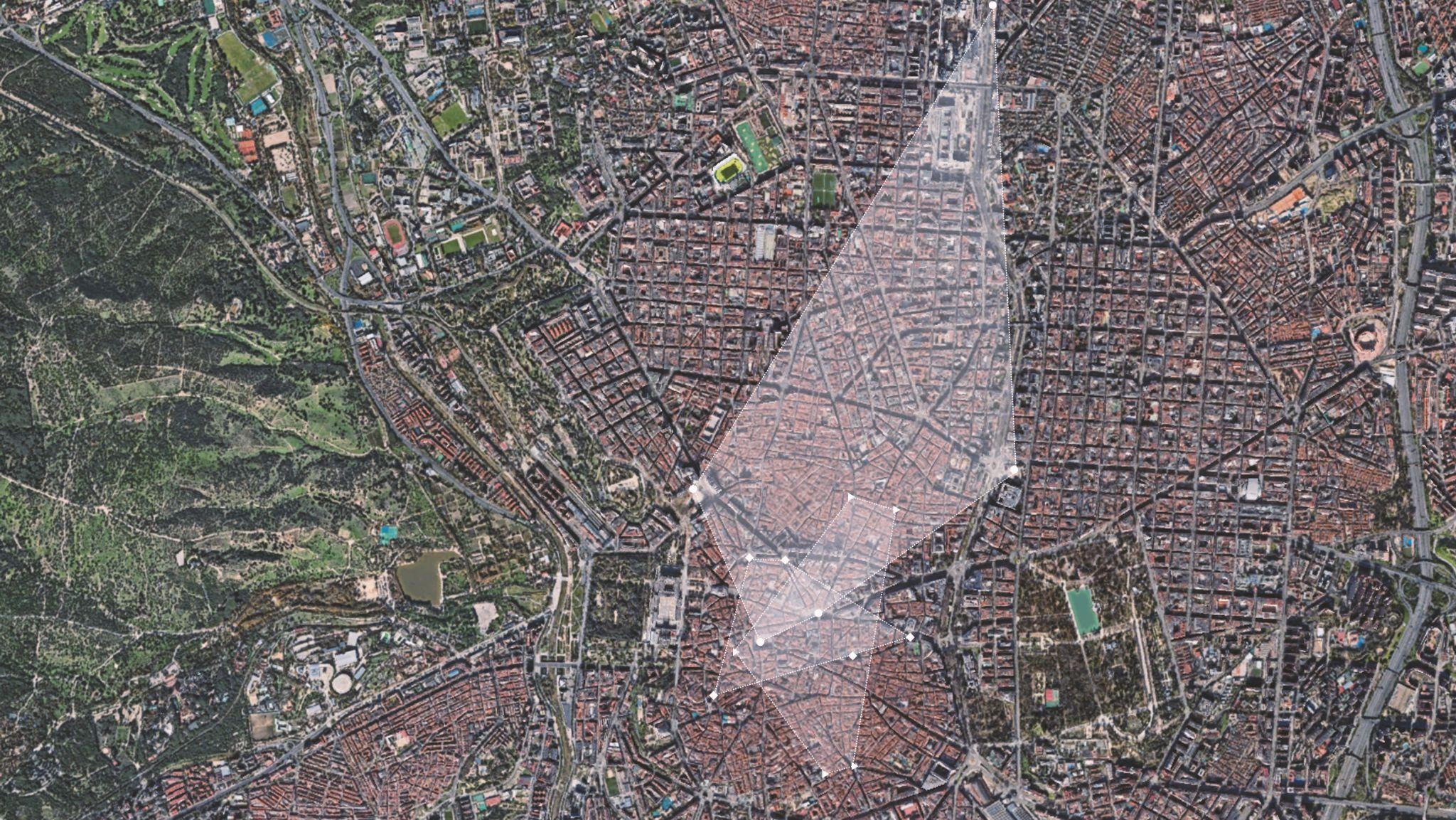
big3l = 'b3_plazamayor' big4 = (40.4168624, -3.7043032918742496)
big4l = 'b4_puertadelsol' big5 = (40.4528601, -3.6918548) # Plaza Joan Miró big5l = 'b5_plazajoanmiro'
# MEDIUM PLAZAS med1 = (40.41480405,-3.700791305837731) # Plaza de Santa Ana med1l = 'm1_plazasantaana' med2 =(40.414318300000005,-3.7018736655575606) # Plaza del Ángel med2l = 'm2_plazaangel' med3 = (40.4129726,-3.7115924640117006) # Plaza de la Paja med3l = 'm3_plazapaja' med4 = (40.420003699999995,-3.7086054878361954) # Plaza de Santo Domingo med4l = 'm4_plazadomingo' med5 = (40.41985725,-3.705811999578338) # Plaza de Callao med5l = 'm5_plazacallao' med6 = (40.415769444444, -3.6966277777778) # Plaza de las Cortes med6l = 'm6_plazacortes'
# SMALL PLAZAS sma1 = (40.40876035,-3.7011310653563037) # Plaza de Lavapiés sma1l = 's1_plazaLavapiés' sma2 = (40.423085400000005,-3.7009577744619797) # Plaza de Raffaella Carrà sma2l = 's2_plazacarra' sma3 = (40.41146565,-3.710911327563633) # Plaza de la Puerta de Moros sma3l = 's3_plazamoros' sma4 = (40.40806785,-3.702809551597745) # Plaza de la Corrala de Sombrerete sma4l = 's4_plazacorrala' sma5 = (40.4148187,-3.7094801592775593) # Plaza del Conde de Miranda sma5l = 's5_plazamiranda' sma6 = (40.422691666667, -3.6975972222222) # Plaza de Chueca sma6l = 's6_plazachueca'
The analysis of the initial 15 study case is reduced to plazas located the city of Madrid. This decision simplifies the process by assuming that all the items share same cultural and environmental conditions To try to include as many urban situations as possible in this research, squares of different sizes (small, medium and large) have been selected. Therefore, the initial hypothesis considers the probability of any of our target events occurring in a place is much more dependent of its design factors than of its size.
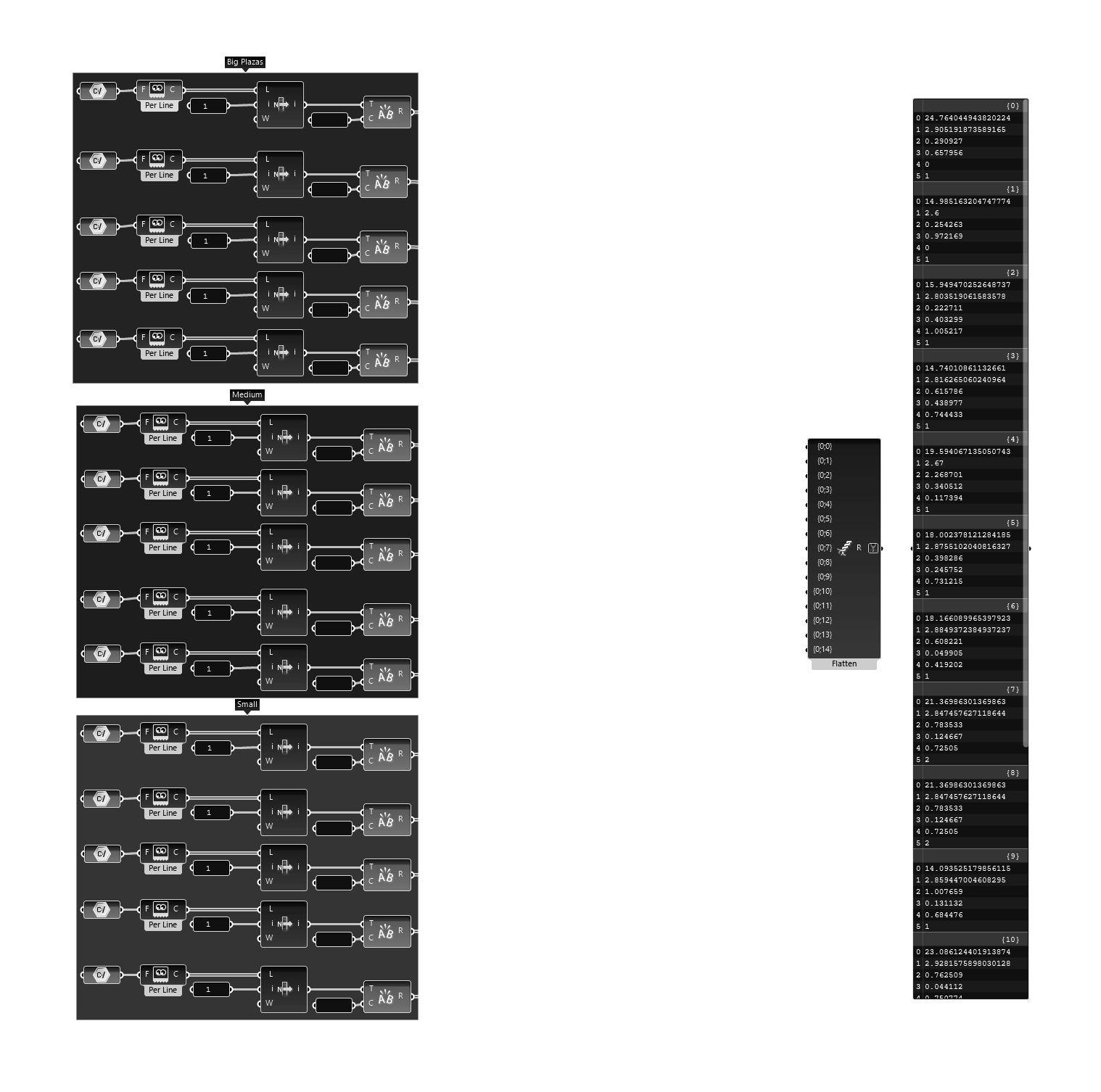
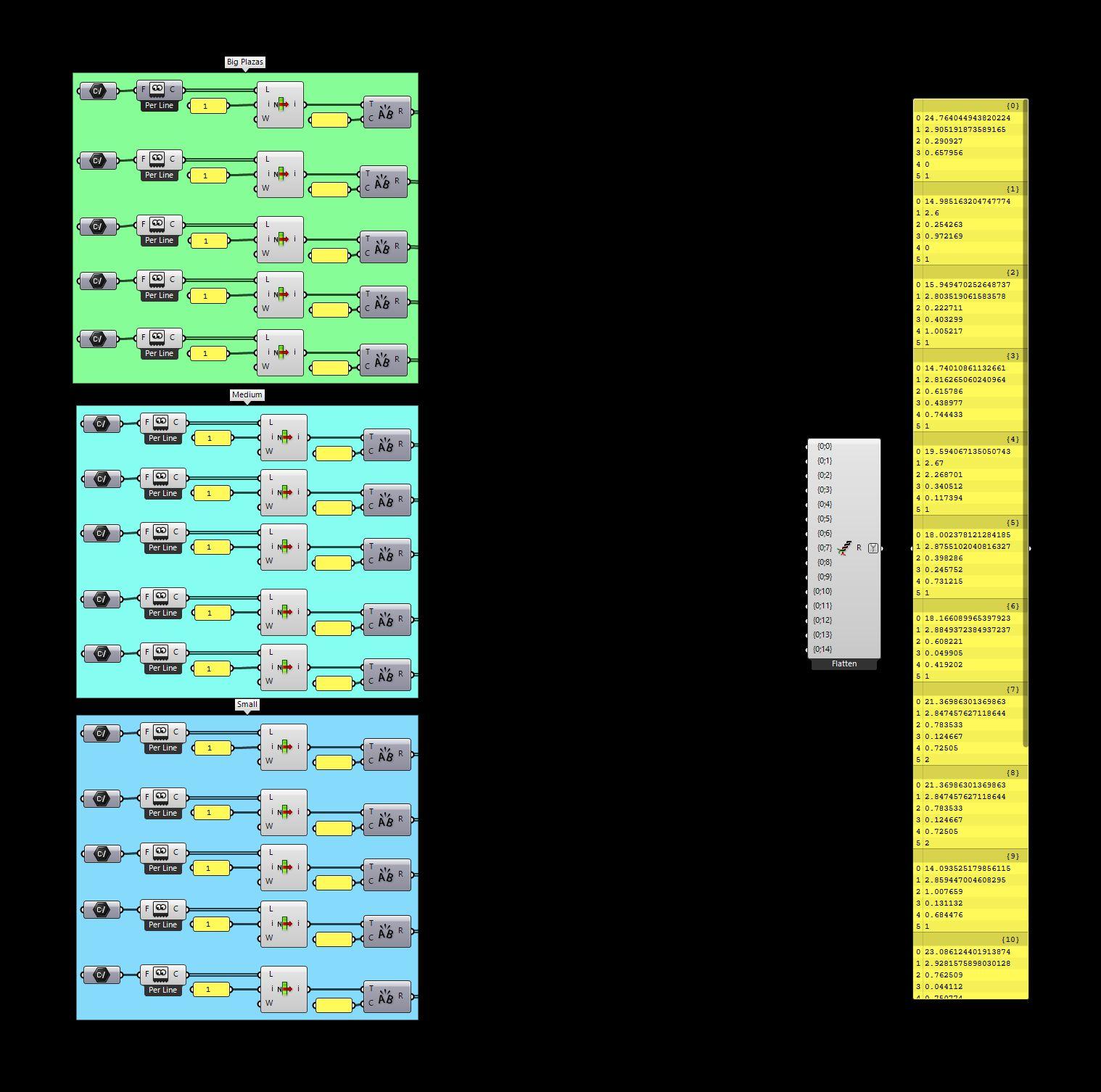
DATASET CREATION
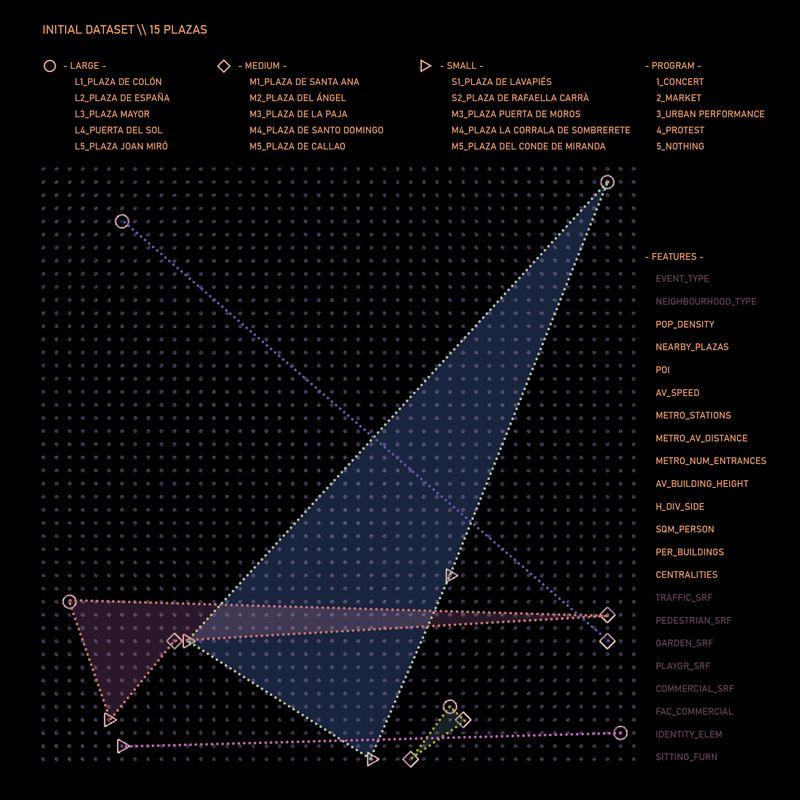
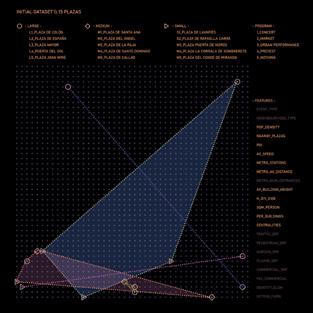
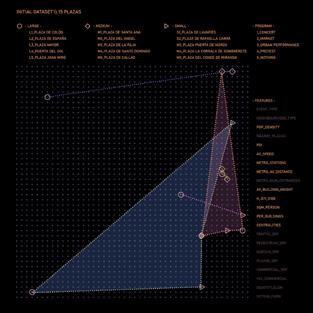
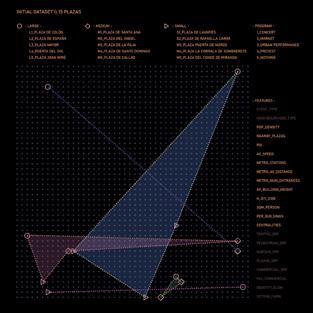
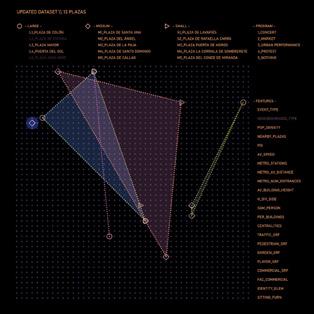
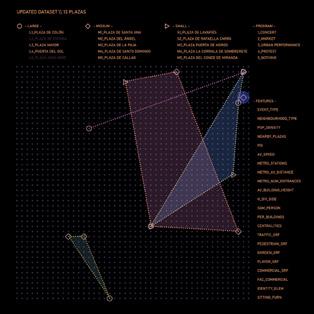
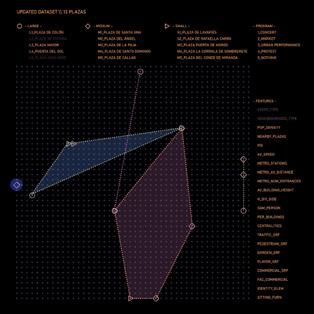
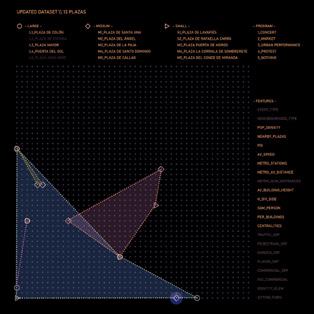
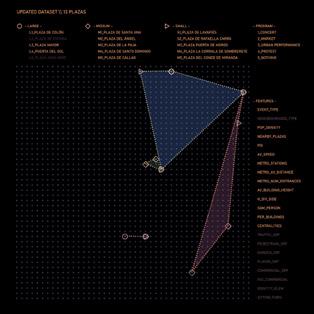
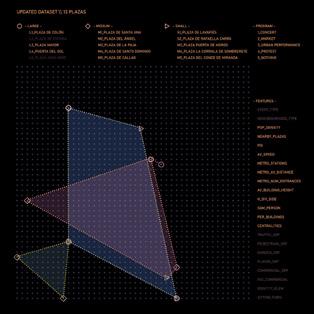
An initial dataset large and balanced enough in samples and urban situations is needed to train the classification model. The process is achieved by generating a Kohonen Map that interpolates the values of the initial squares and produces around 2000 unreal items
After testing different combinations to determine which size of board better allows the seeds to occupy the boundaries of the map (30x70, 40x50 and 45x45), the square ratio of 45x45 seems to produce the most distributed results.
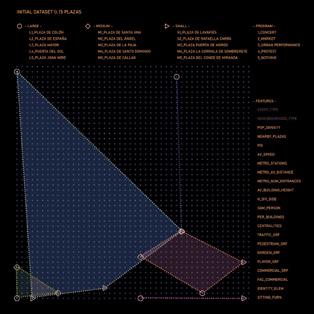
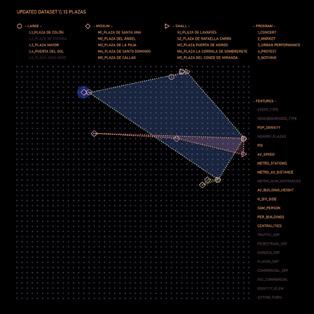
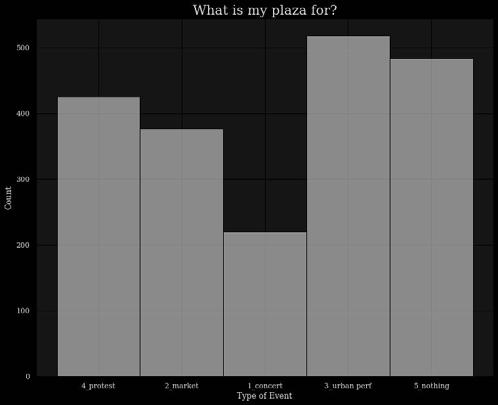
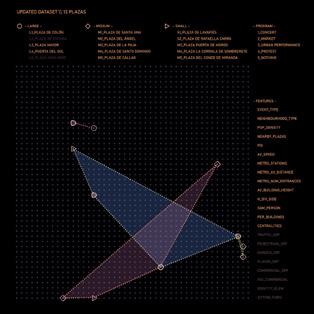
Finally, two of the initial plazas (one small-sized and one medium-sized), whose urban features placed them extremely close to others of different use and, therefore, reduced the number of samples relating to both programs, are replaced for two other items with some geometrical factors that were overlooked in the first analysis.
The two large squares previously excluded, as they positioned themselves on the boundaries and expand the board, are reintroduced as case study.
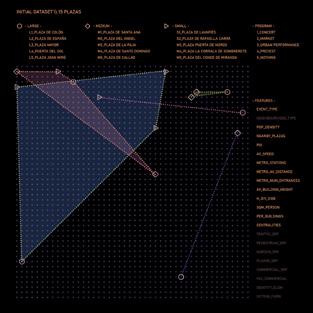
Finally, some of the metrics initially considered are modified or refined with the intention of avoid highly correlated values (directly or indirectly) in the dataset.
These types of variations and backward steps in the creation of our dataset highlight, once again, the complexity of including the appropriate metrics to analyze public spaces without oversimplifying the parameters that define them.
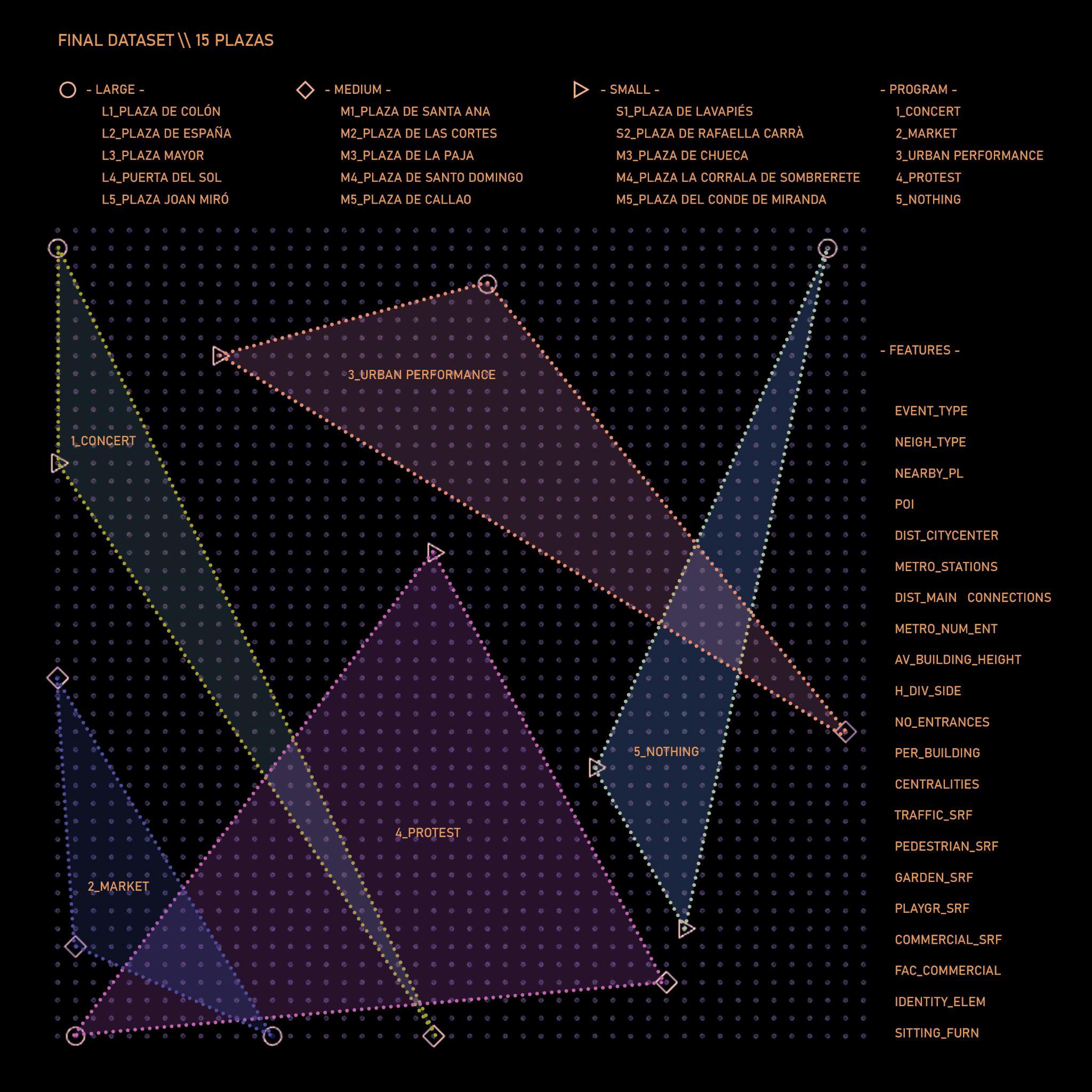
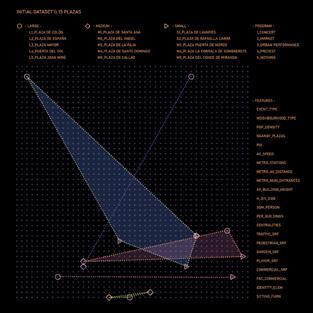
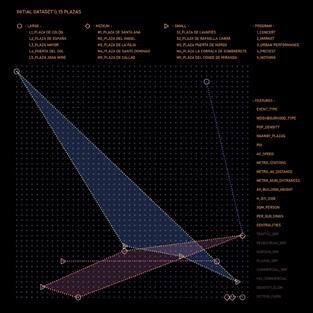
WHAT IS MY PLAZA FOR?
MACHINE LEARNING | EDA
MACHINE LEARNING | CORRELATION HEATMAP
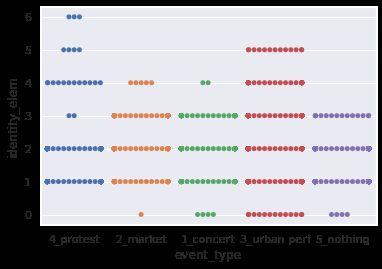
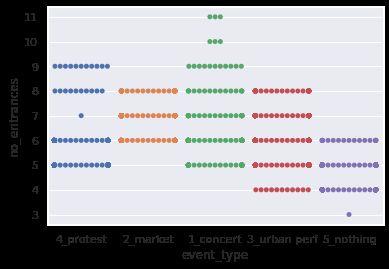
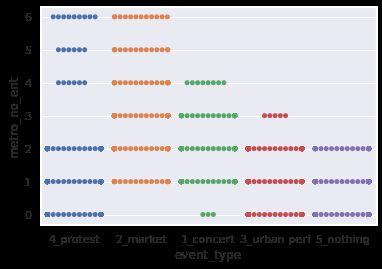
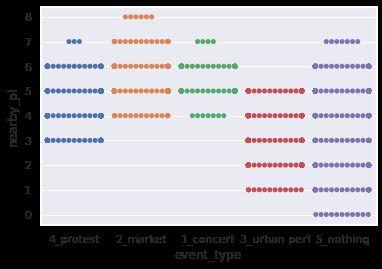
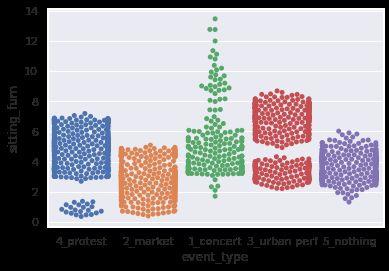
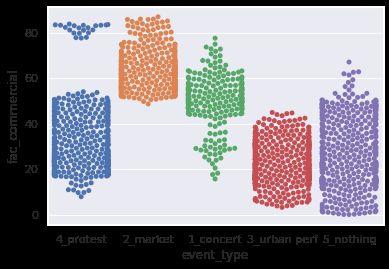
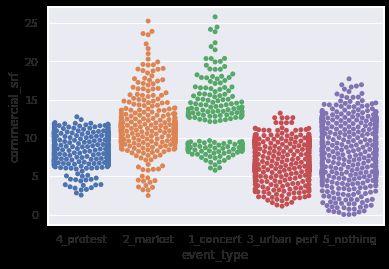
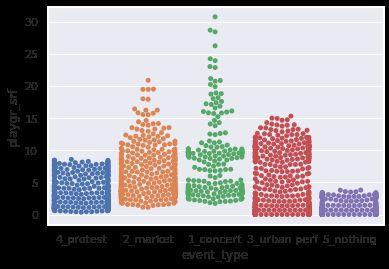
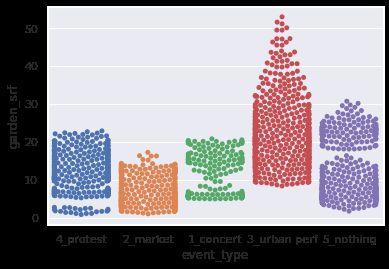
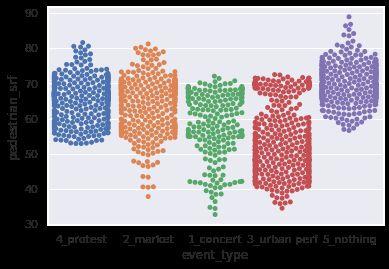
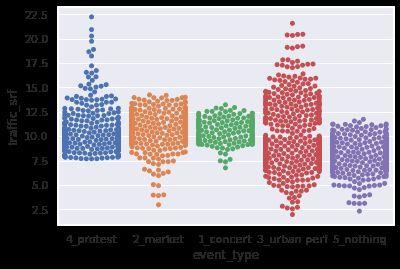
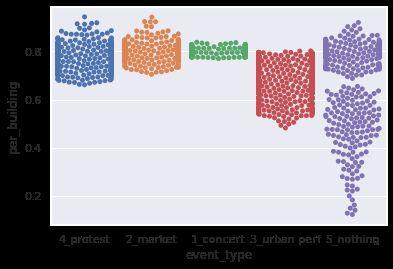
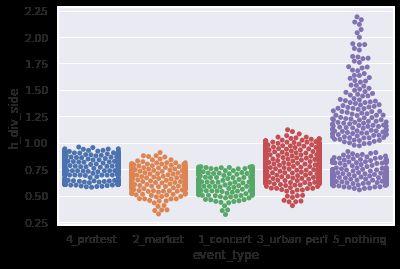
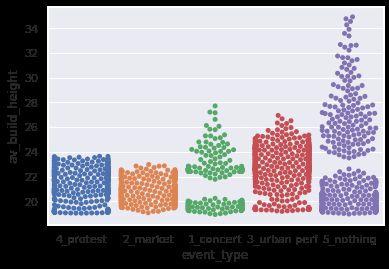
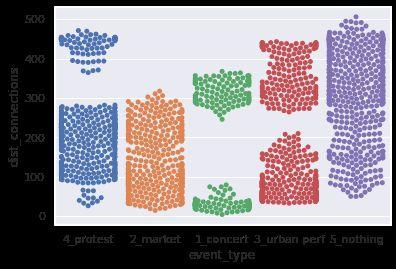
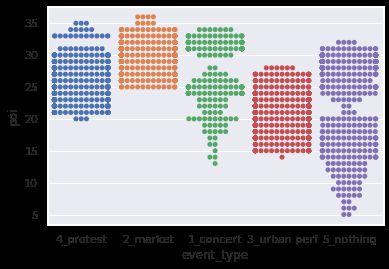
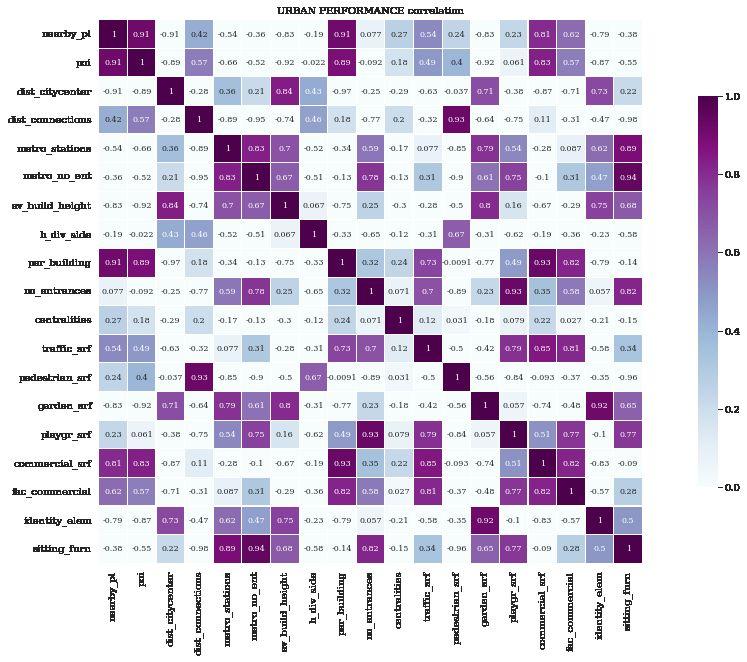
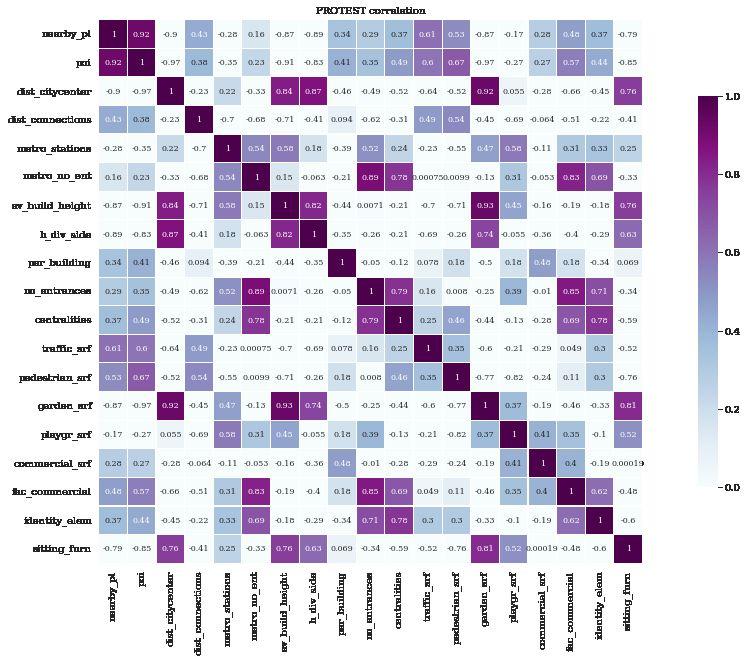
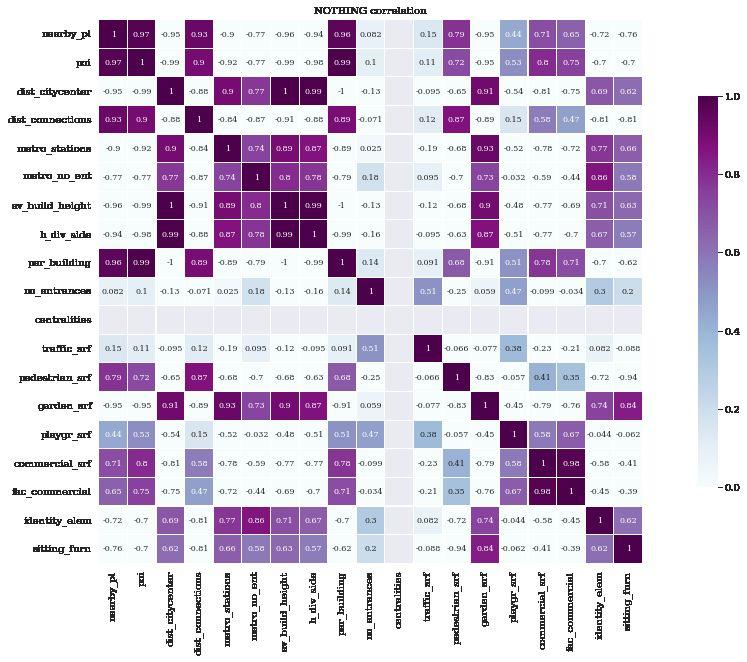
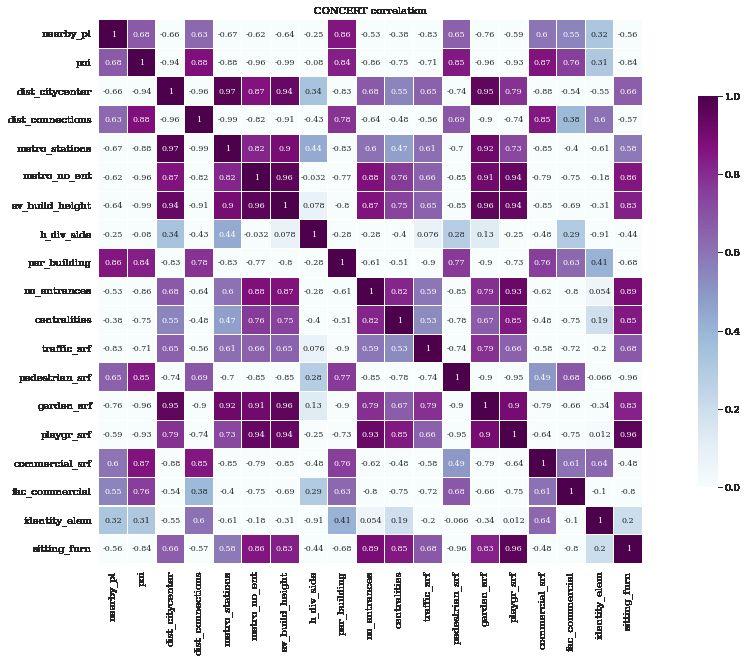
MACHINE LEARNING | CORRELATION HEATMAP
CORRELATION HEATMAP - PEARSON COEFFICIENT
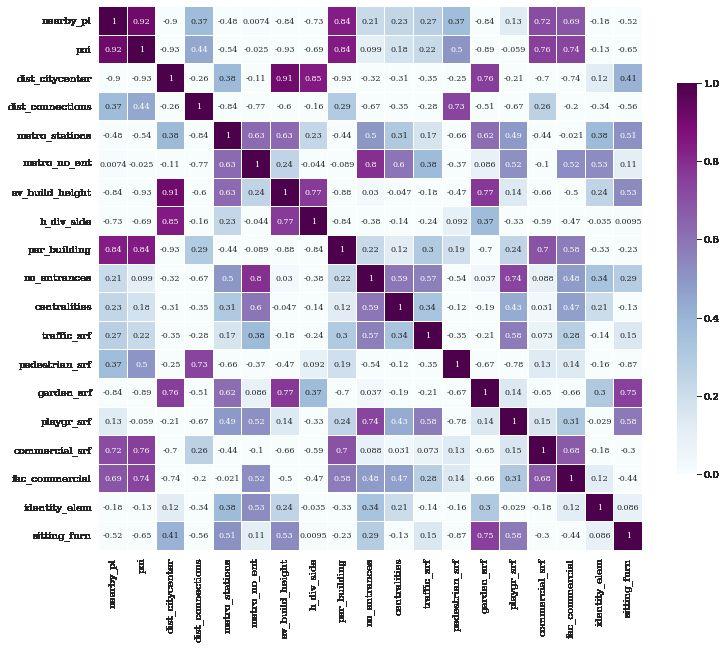
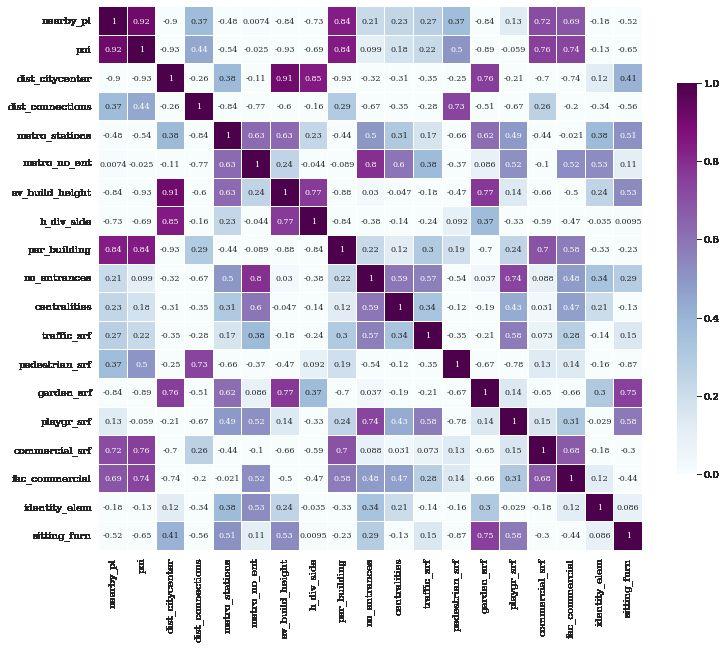
By plotting a correlation matrix we can study the covariance between the features considered for our plazas and, in case of observing a high (direct or indirect) proportional relationship, to analyze if that covariance implies causality or if it is due to the effect of a third variable, such as the type of event. In this case, a high proportionality is observed between the number of nearby squares and the number of POIs
When performing an exclusive heatmap for each type of event, these two columns present covariance for each of the cases, so we can consider reducing one of these parameters to simplify our study model. Some relationship between other pairs of features is observed in the individual analysis per type of event, but they are not considered relevant for the model since this covariance is not observed in the general matrix.
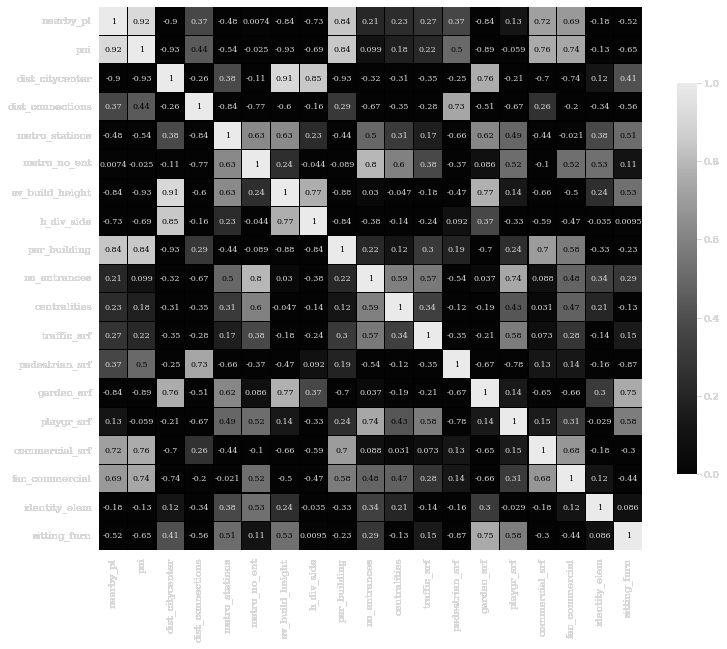
WHAT IS MY PLAZA FOR?
BIPLOT PRINCIPAL COMPONENT
By bi-plotting the results of our first two PCA analysis on a two-dimensional graph it can be determined (as in the subsequent heatmap) that, although some events are more related to certain parameters than others, most of our indicators have a similar relevance in the prediction of our target if we consider all possible events.
In consequence, the first attempts of our classification model will consider all the initial metrics from the dataset.
ANALYSIS
PRINCIPAL COMPONENT HEATMAP
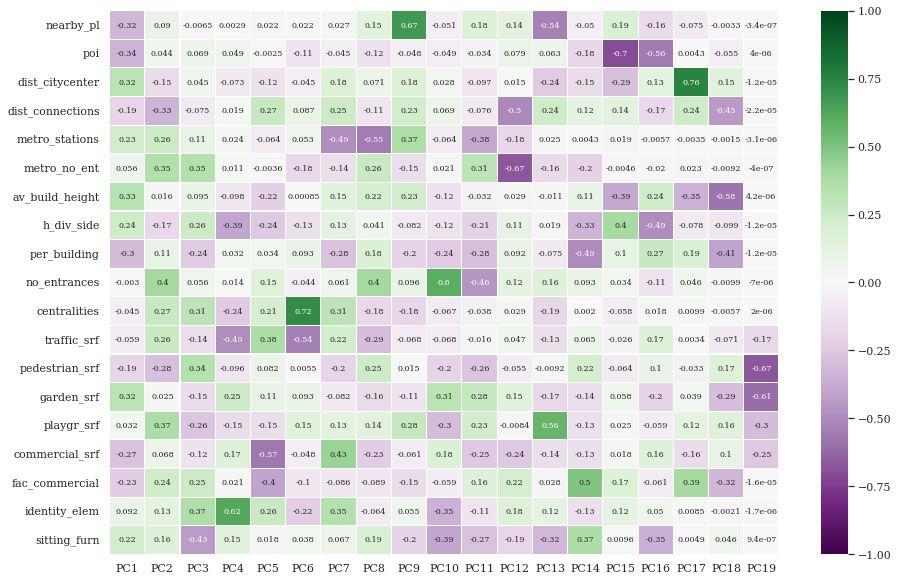
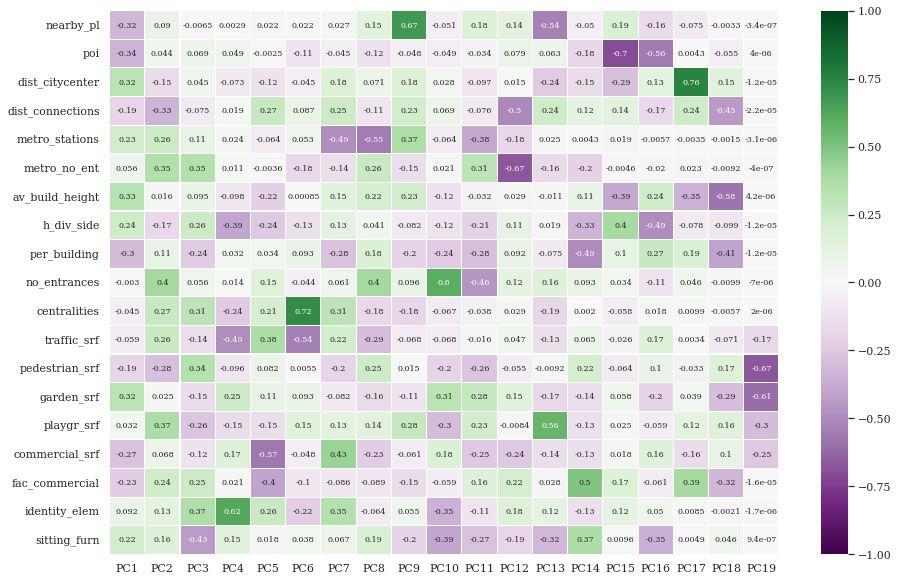
In the relationship matrix between the features and the PCA analysis, we can observe that for the first three iterations none of our parameters stands out as highly determinant for the prediction of our target, which reveals that the definition of our initial metrics includes sufficient variability to the model.
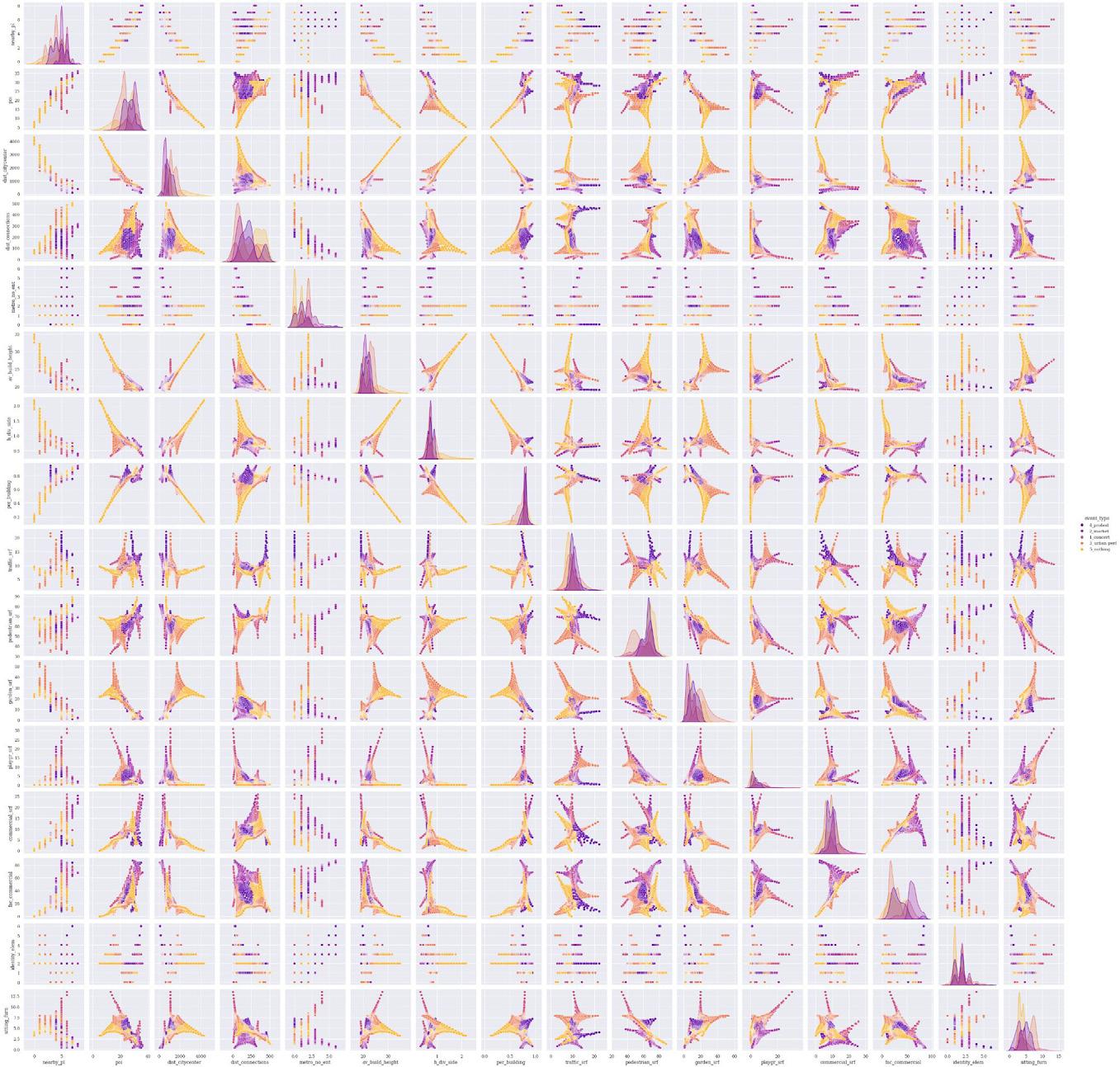
MACHINE LEARNING | PCA ANALYSIS
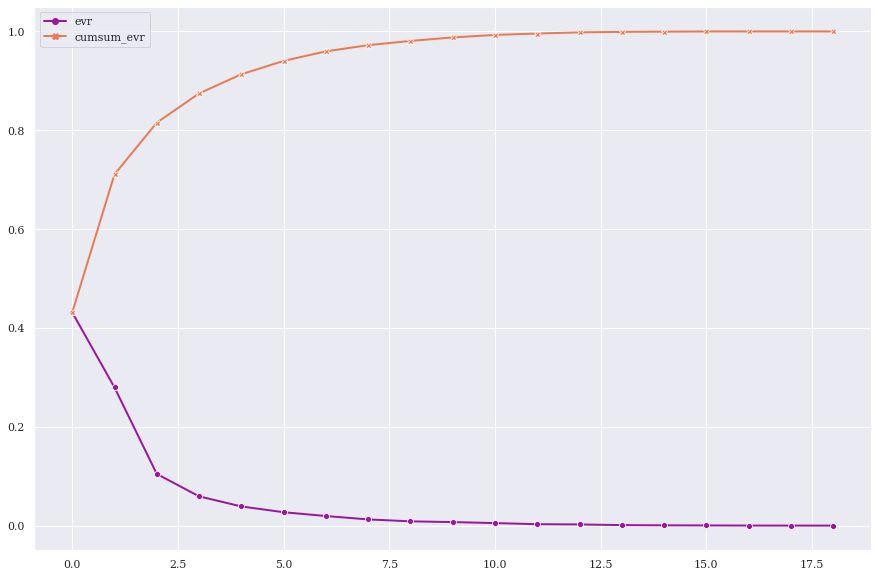
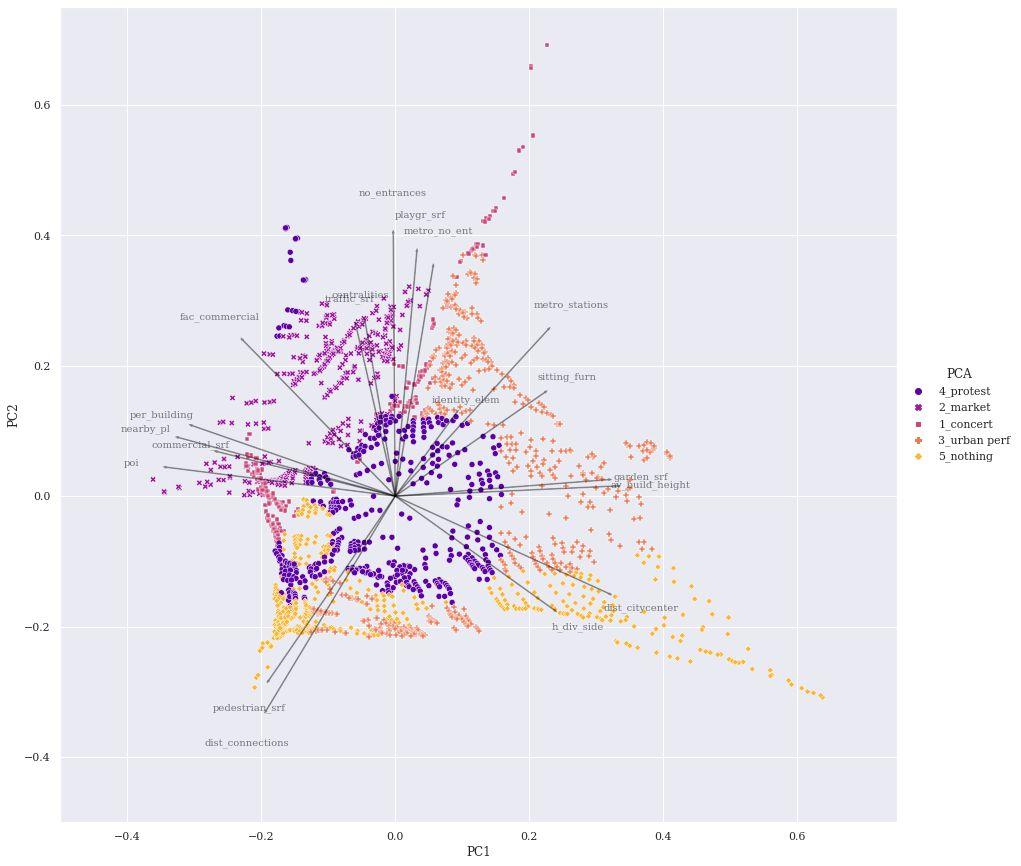
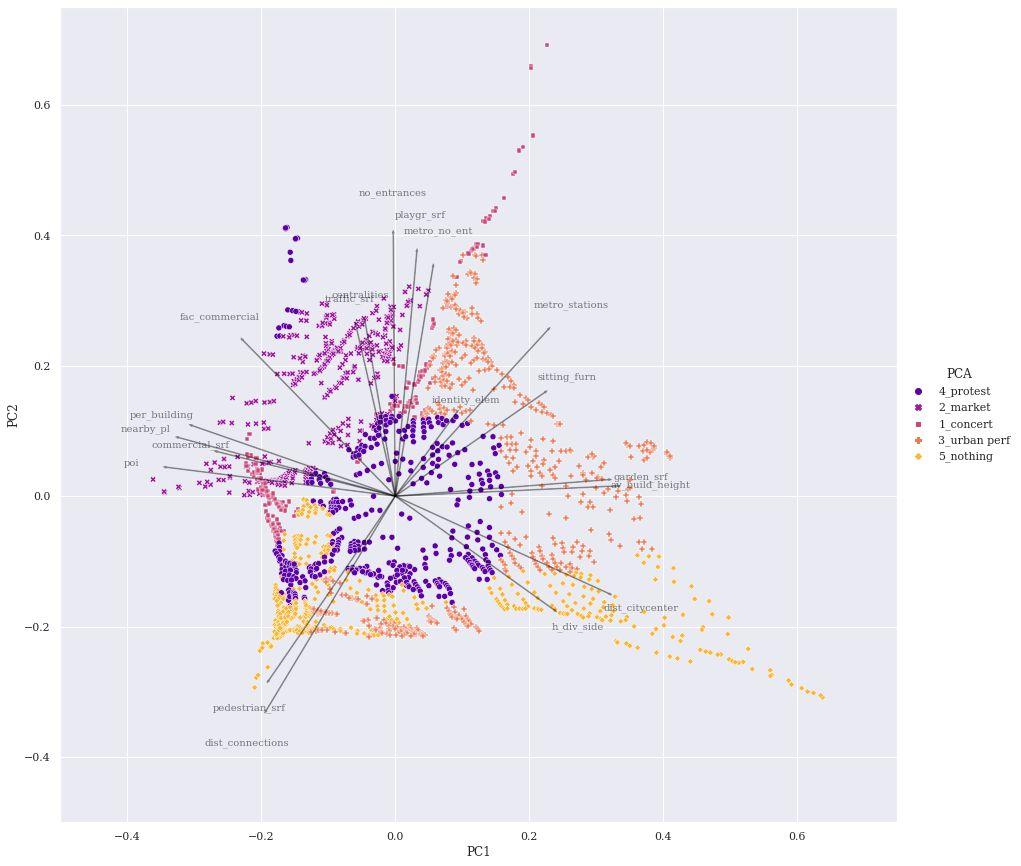
PC1 poi
PC2 no_entrances
PC3 sitting_furn
PC4 identity_elem
PC5 commercial_srf
PC6 centralities
PC7 metro_stations
PC8 metro_stations
PC9 nearby_pl
PC10 no_entrances
PC11 no_entrances
PC12 metro_no_ent
PC13 playgr_srf
PC14 fac_commercial
PC15 poi
PC16 poi
PC17 dist_citycenter
PC18 av_build_height
PC19 pedestrian_srf
72% 81% 87% 90% 94% 43%
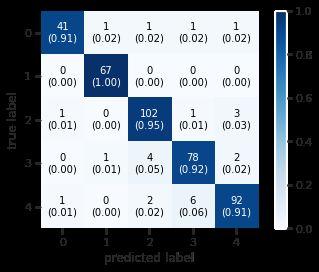
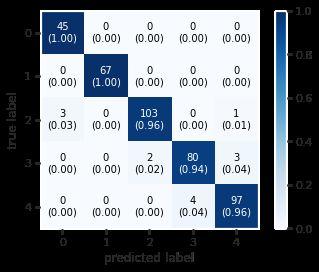
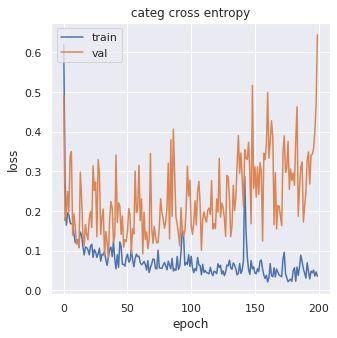
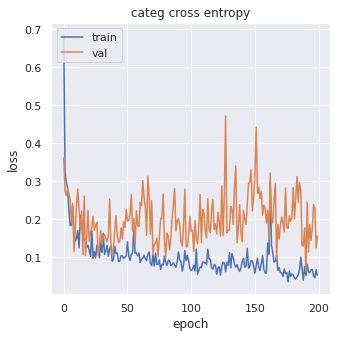
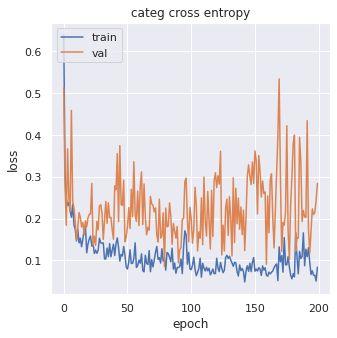
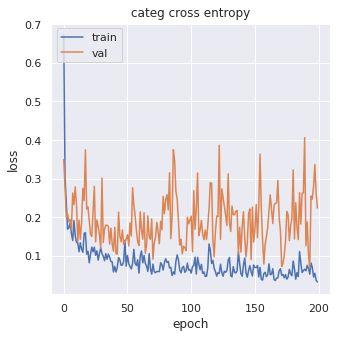
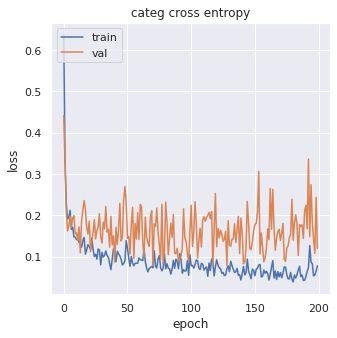
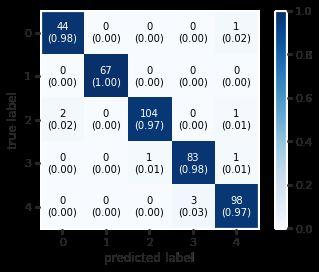
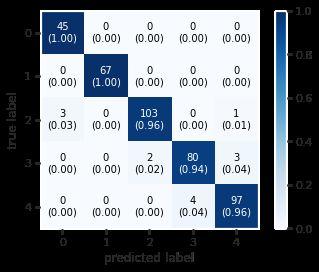
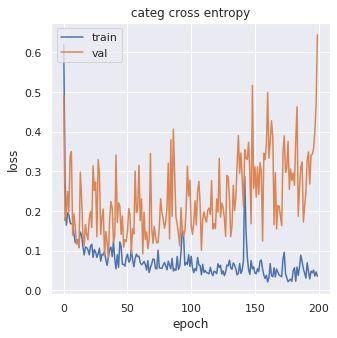
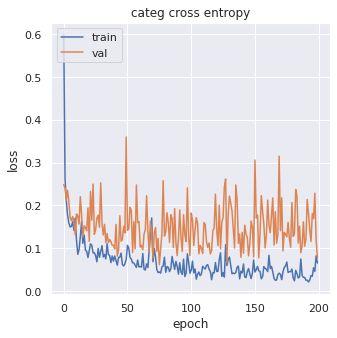
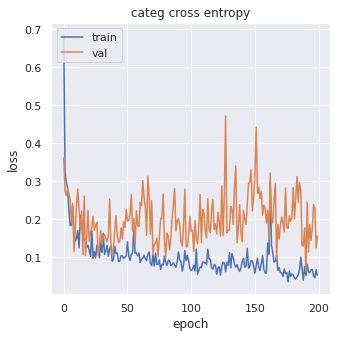
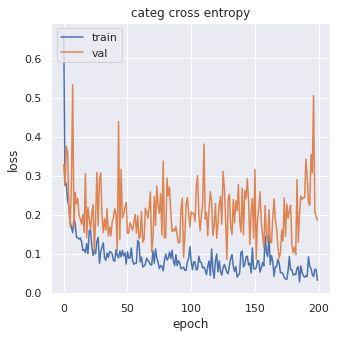
RESULTS EVALUATION | LOCAL ML PREDICTION/HOPS & GH Geometry Verification
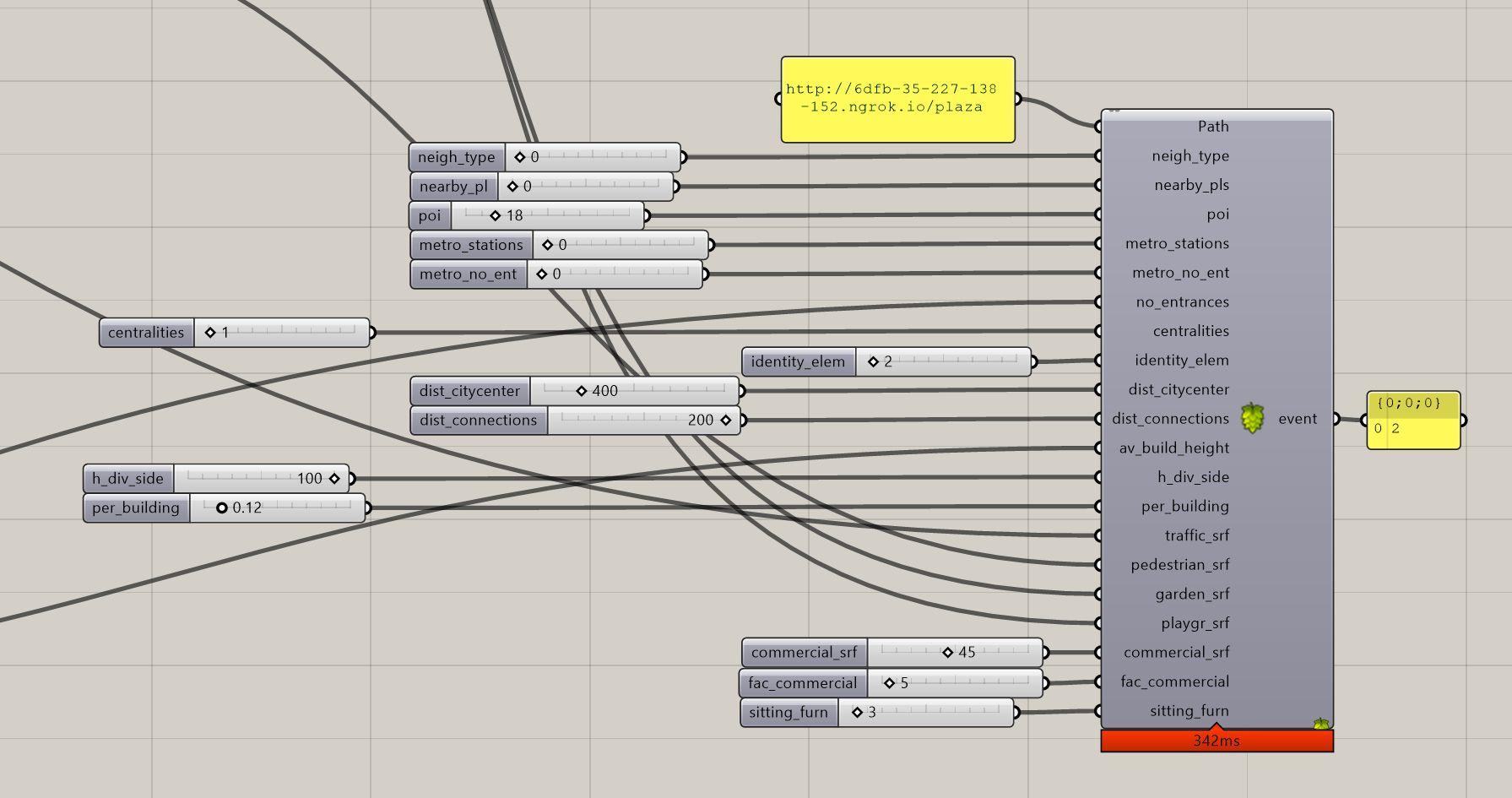
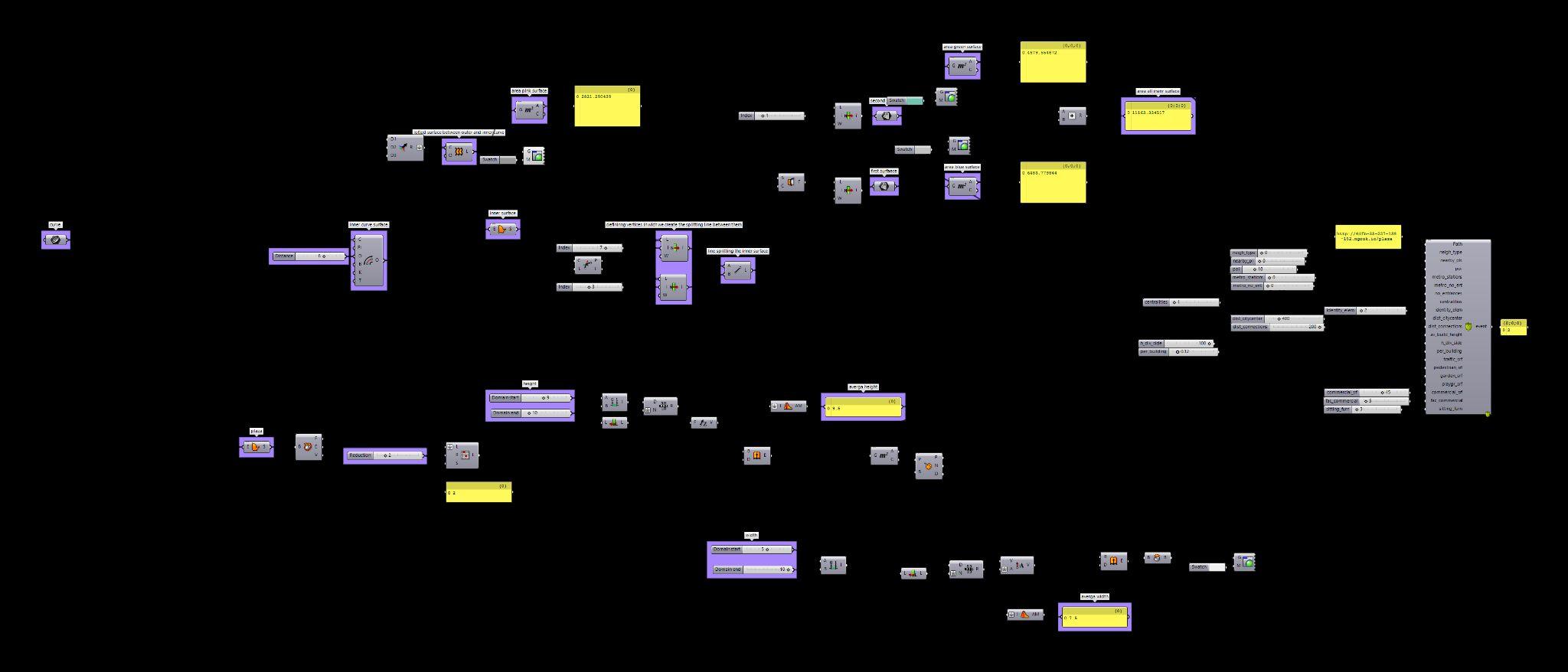
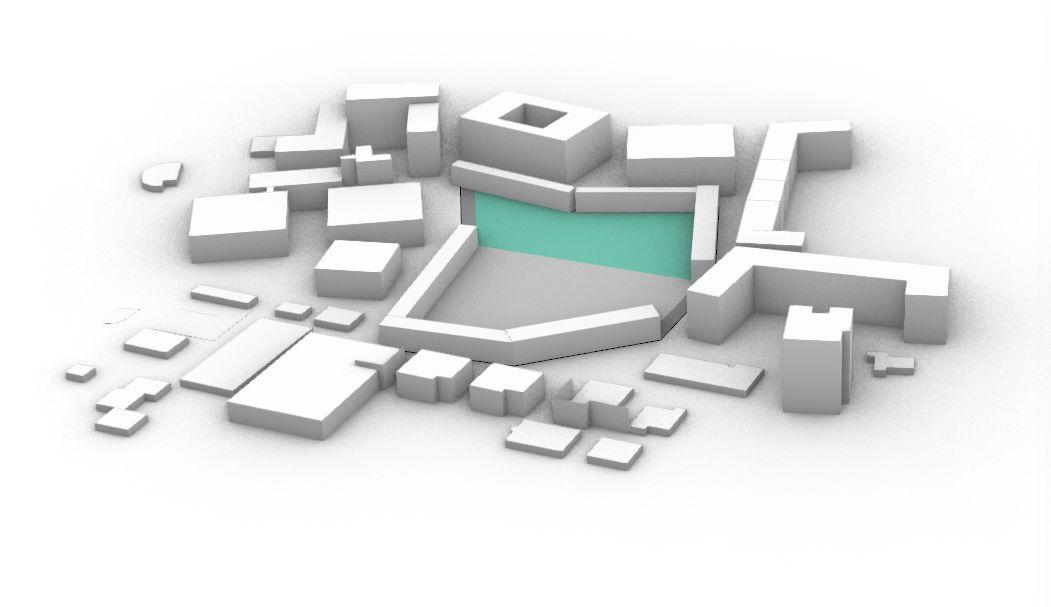
HOPS Parametric Space ML Prediction
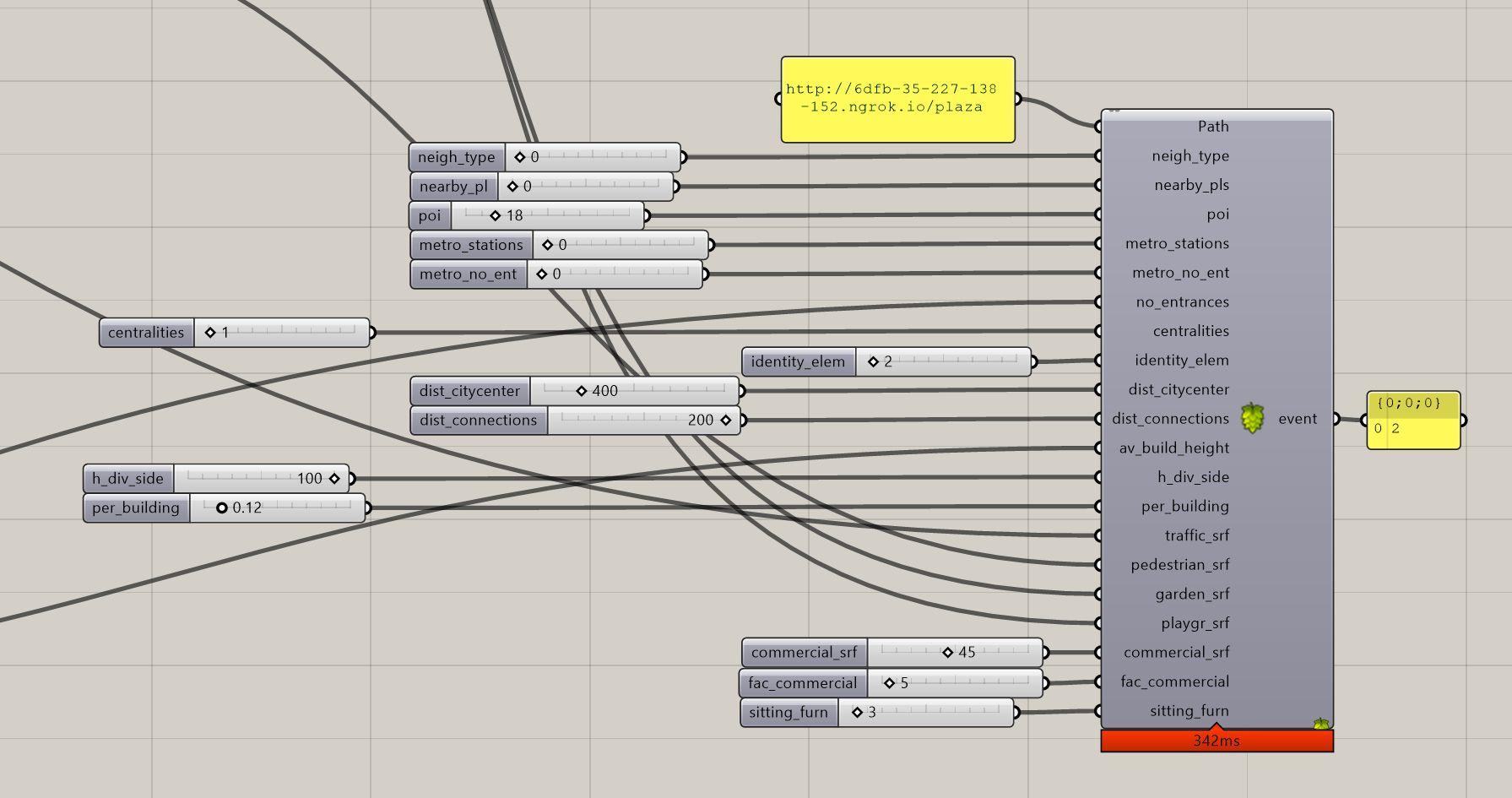
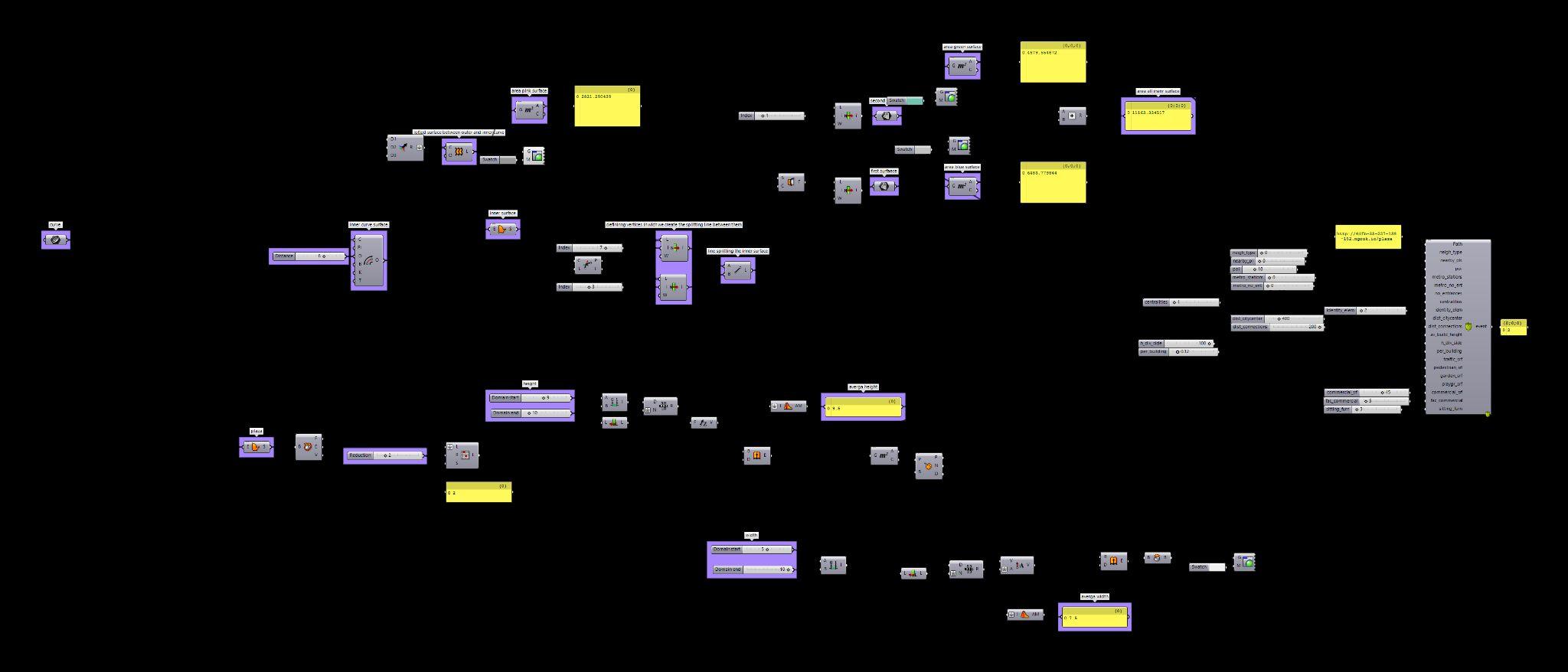
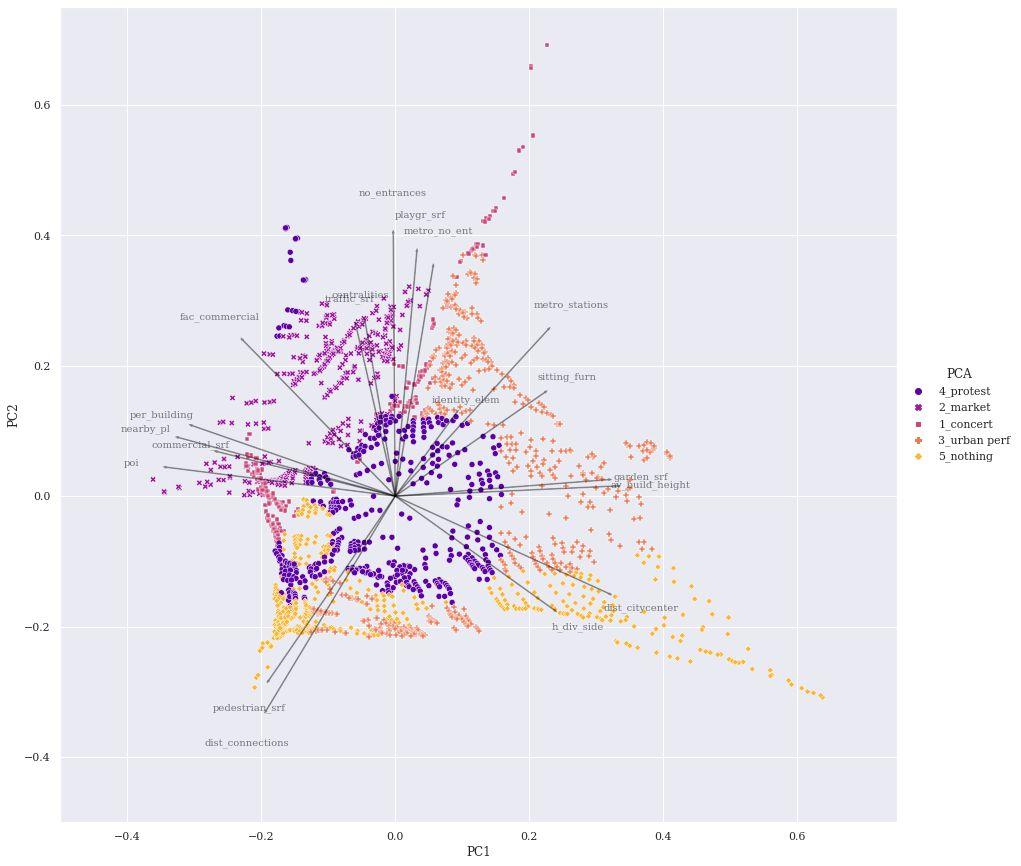
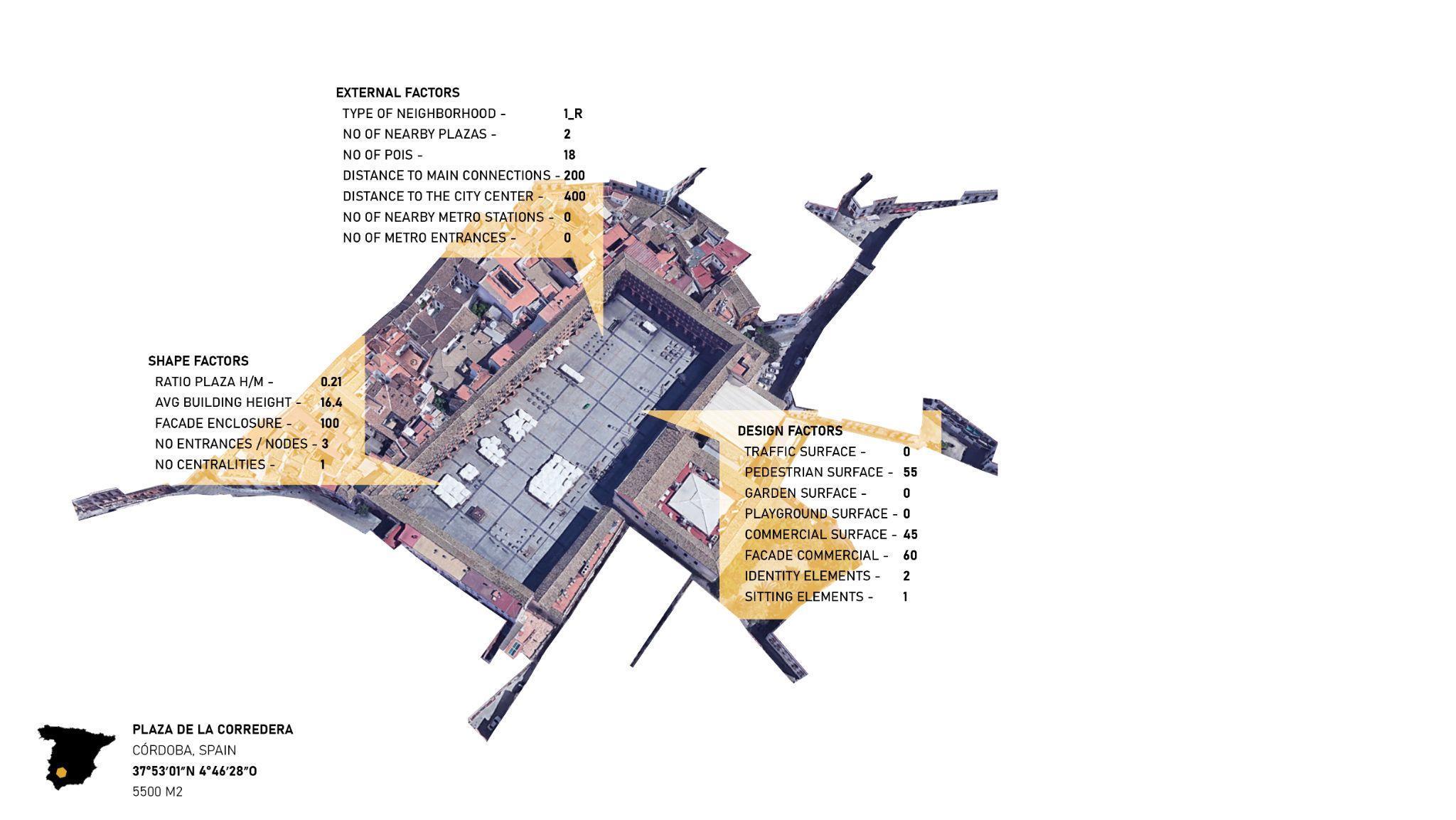
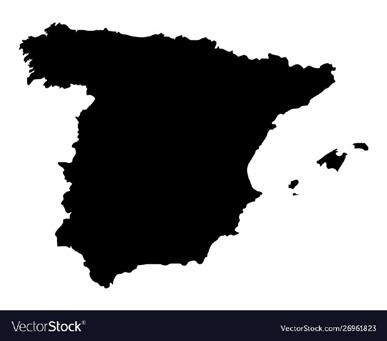
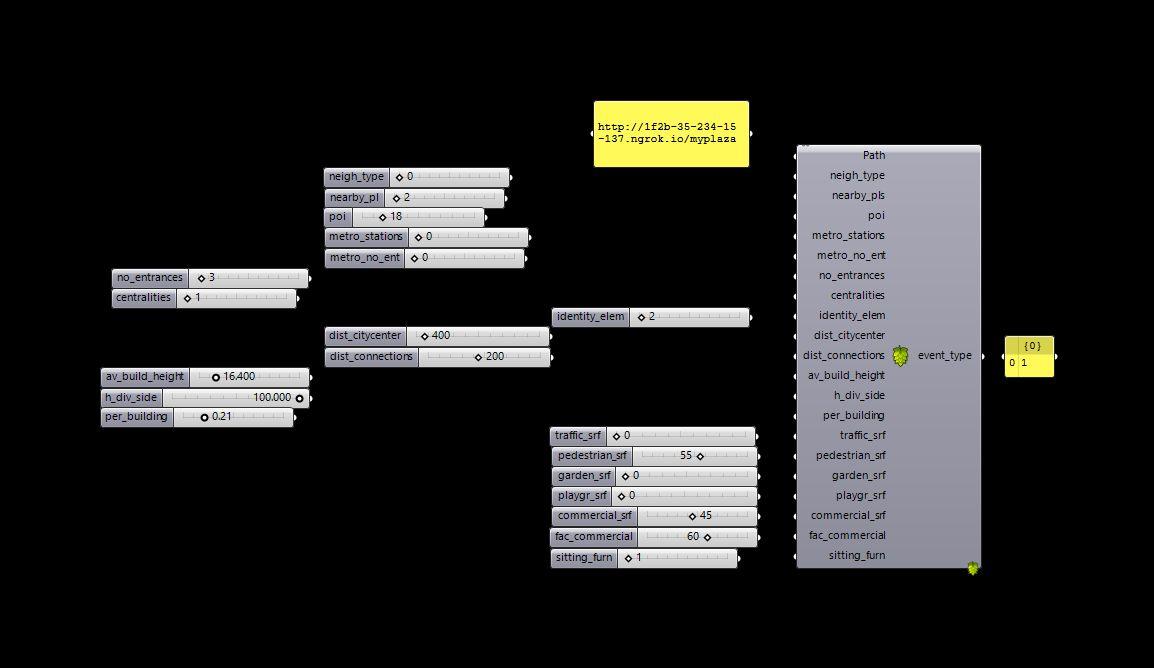
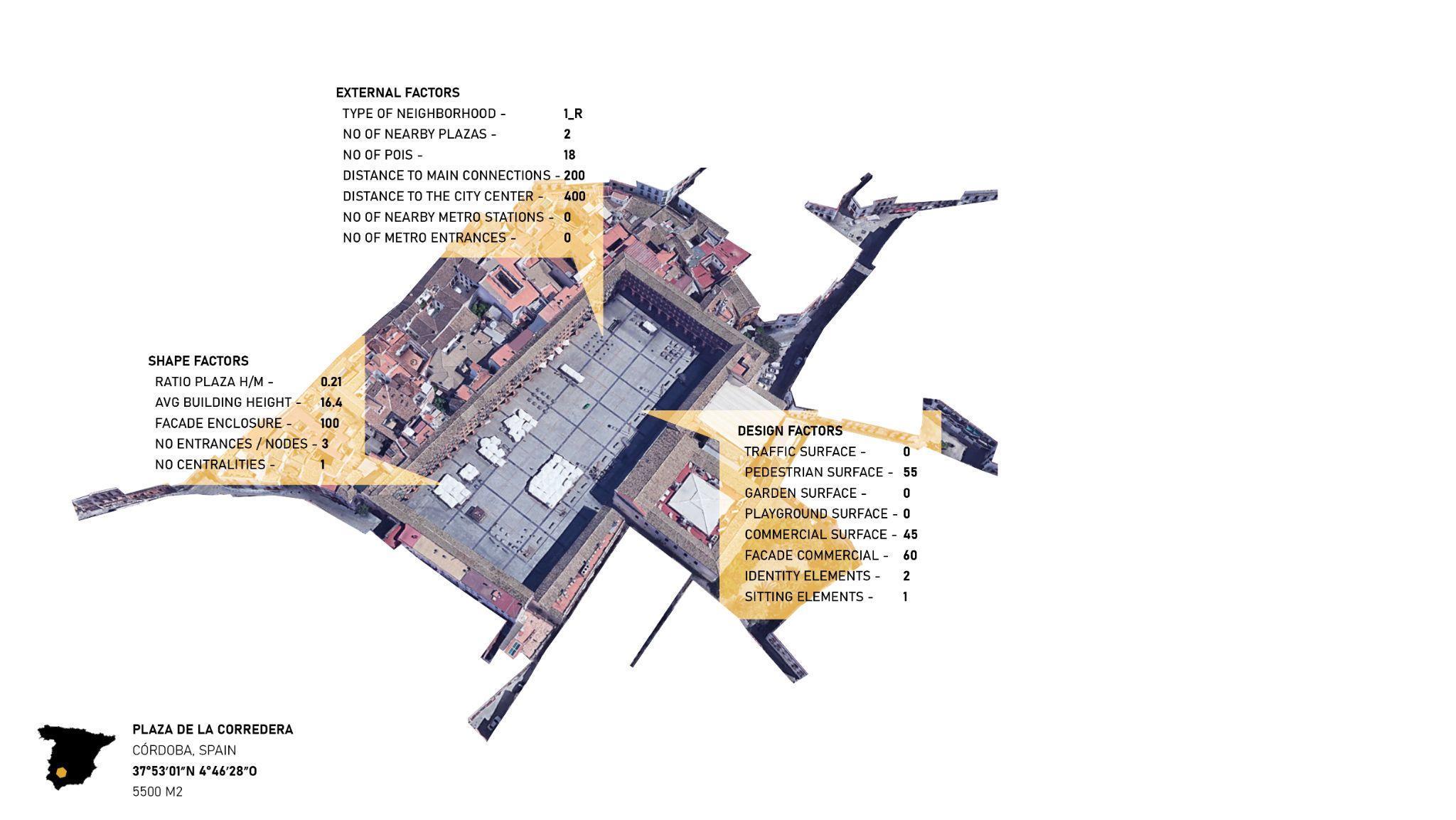
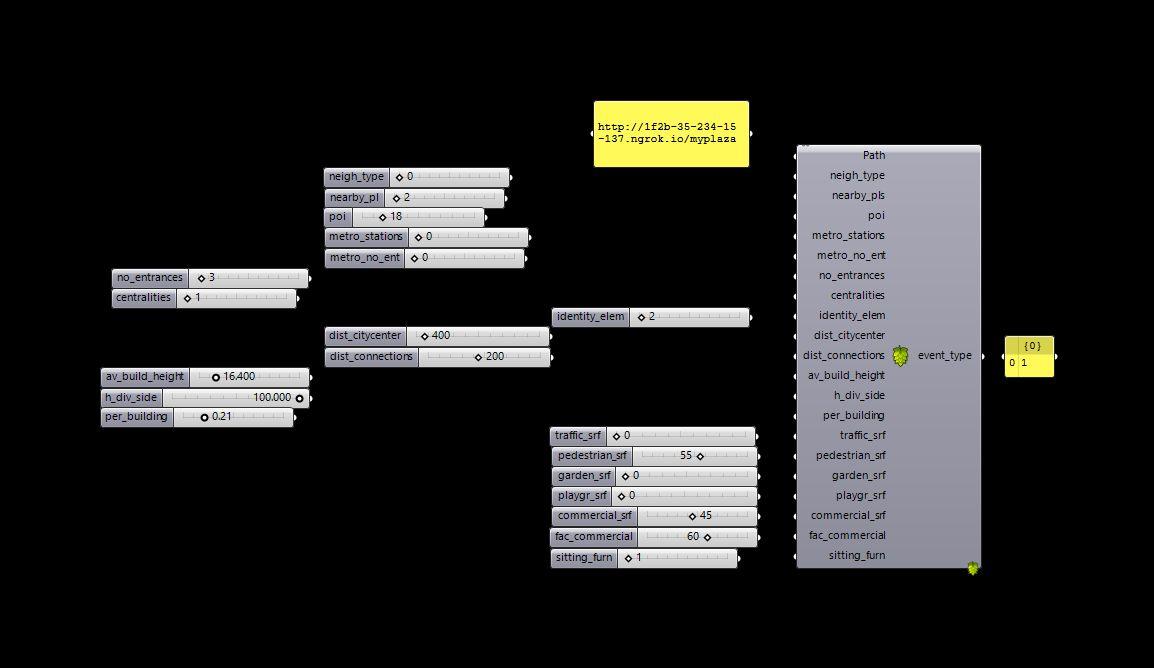
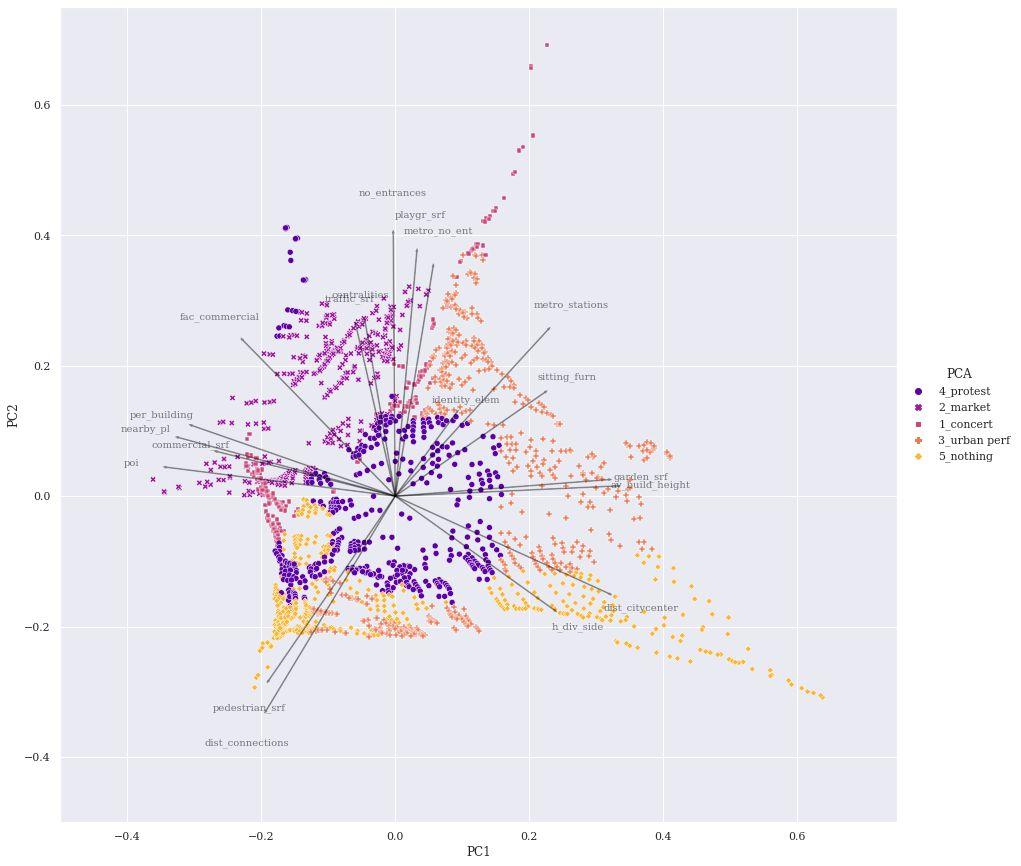
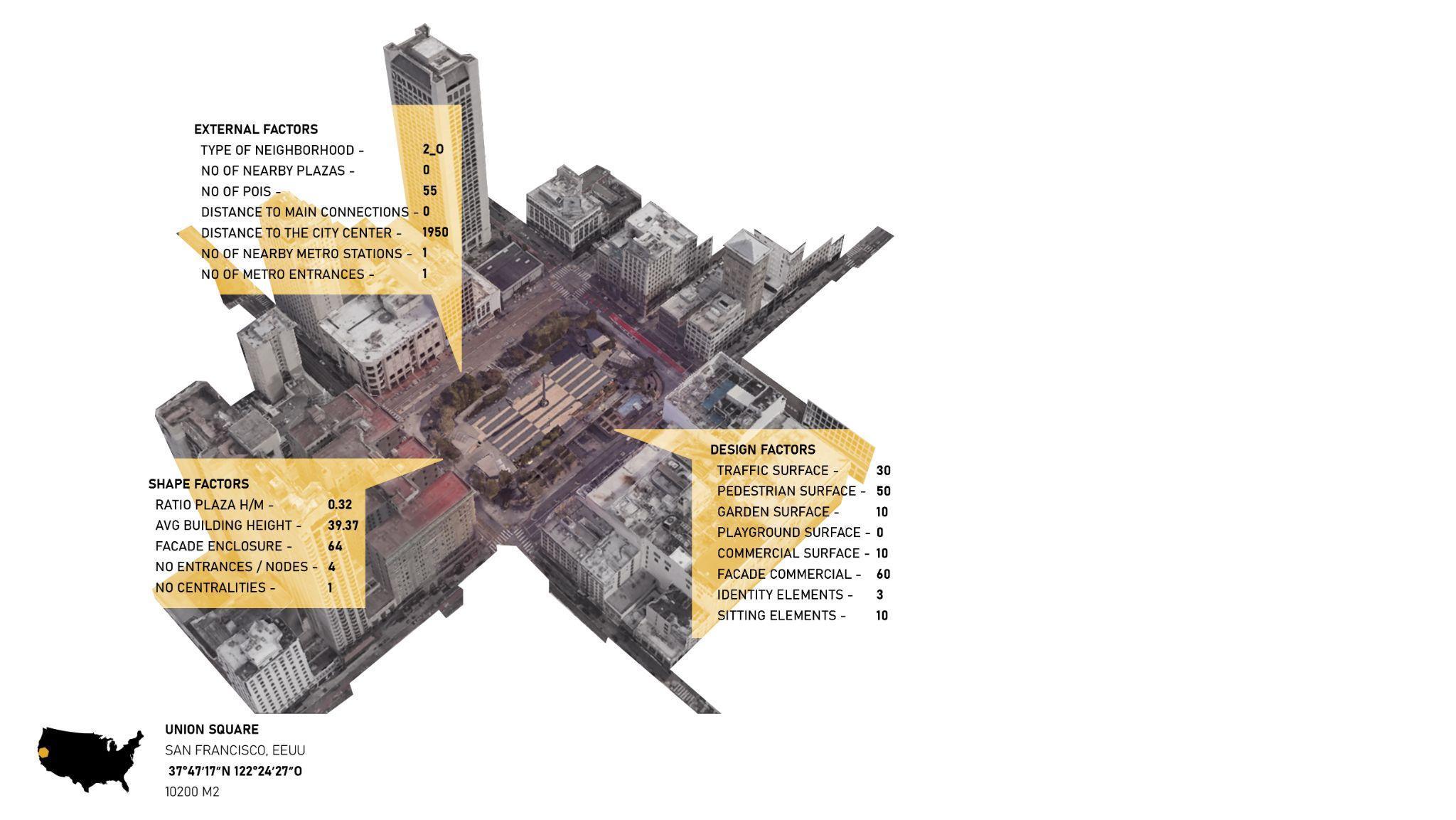
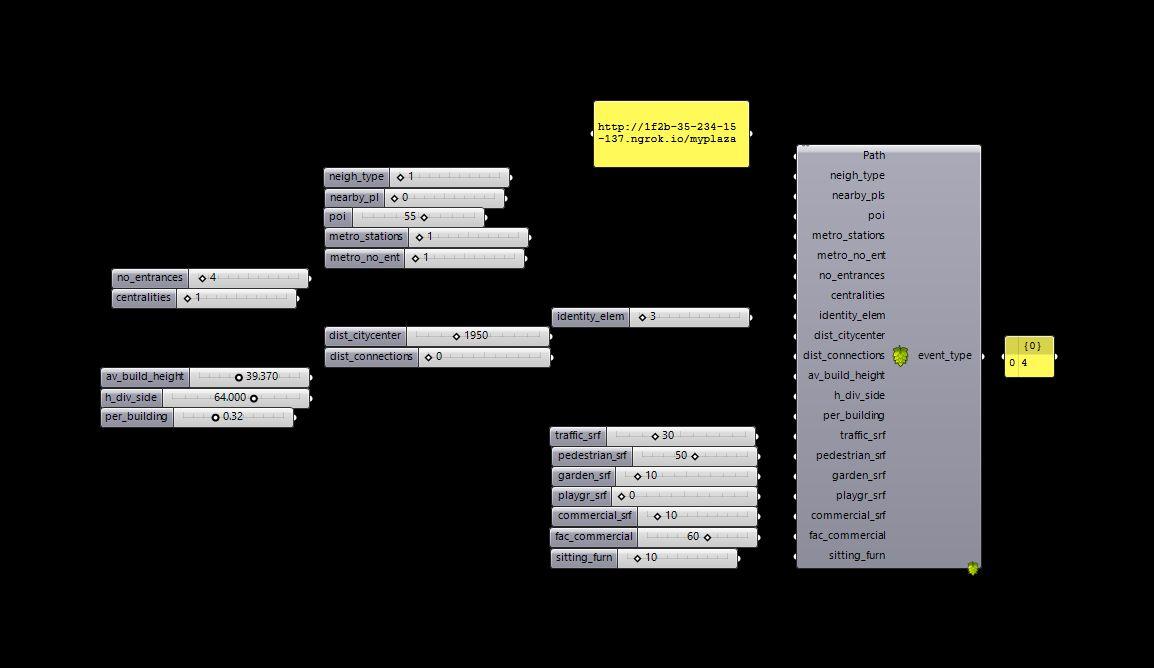
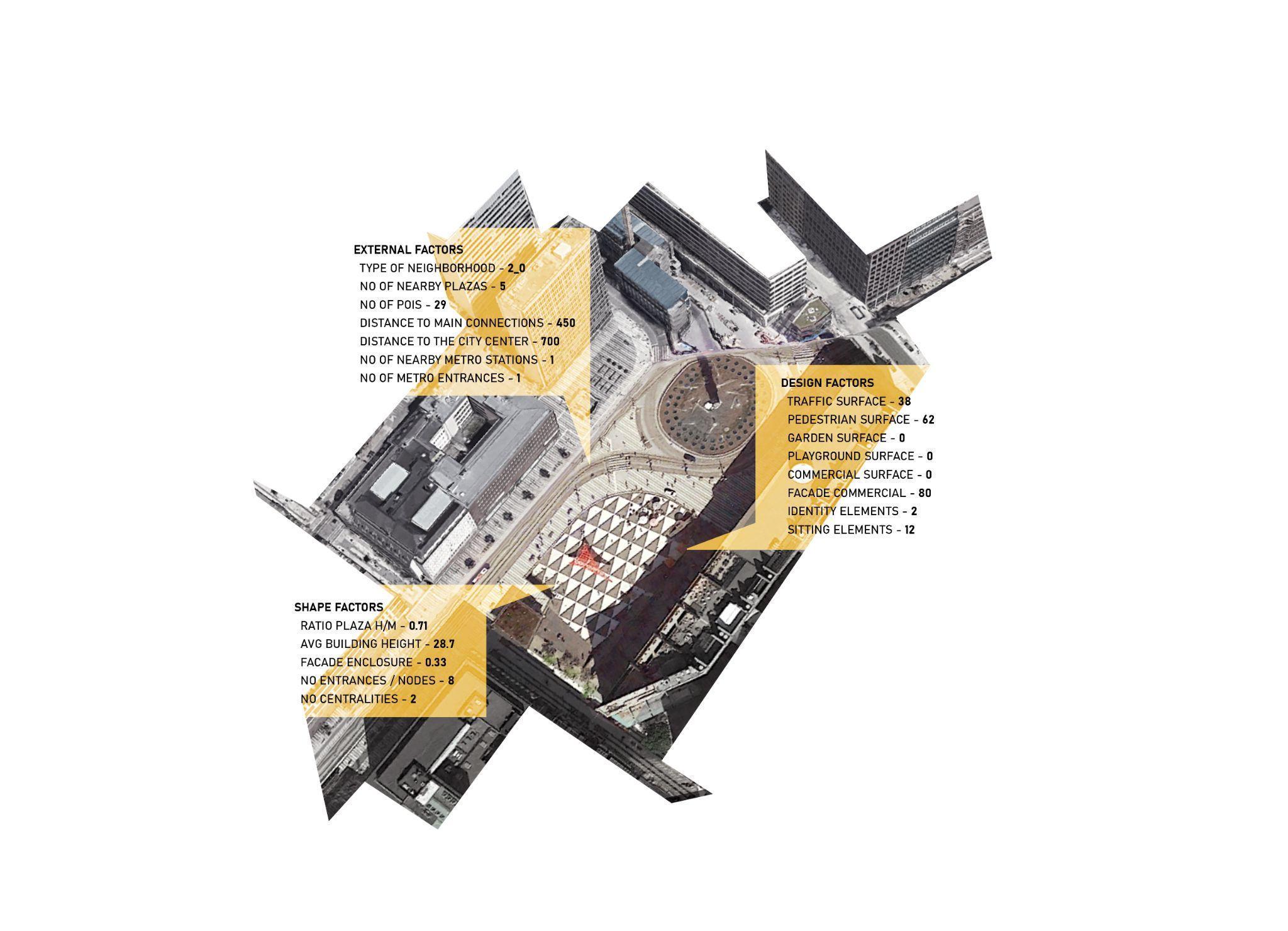
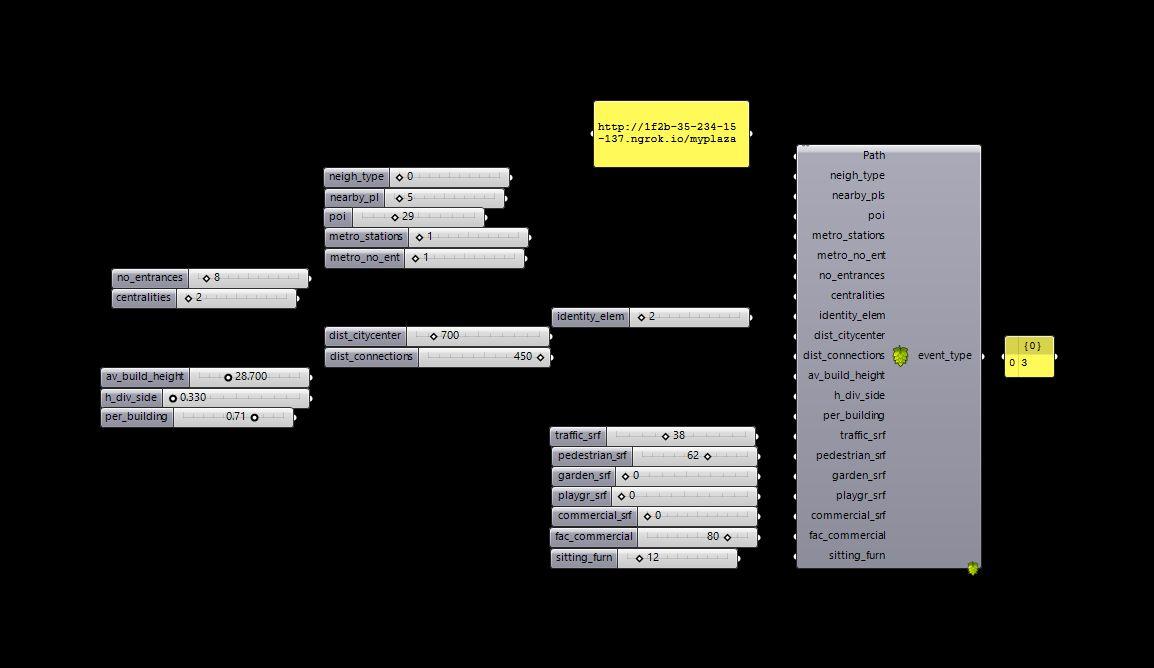
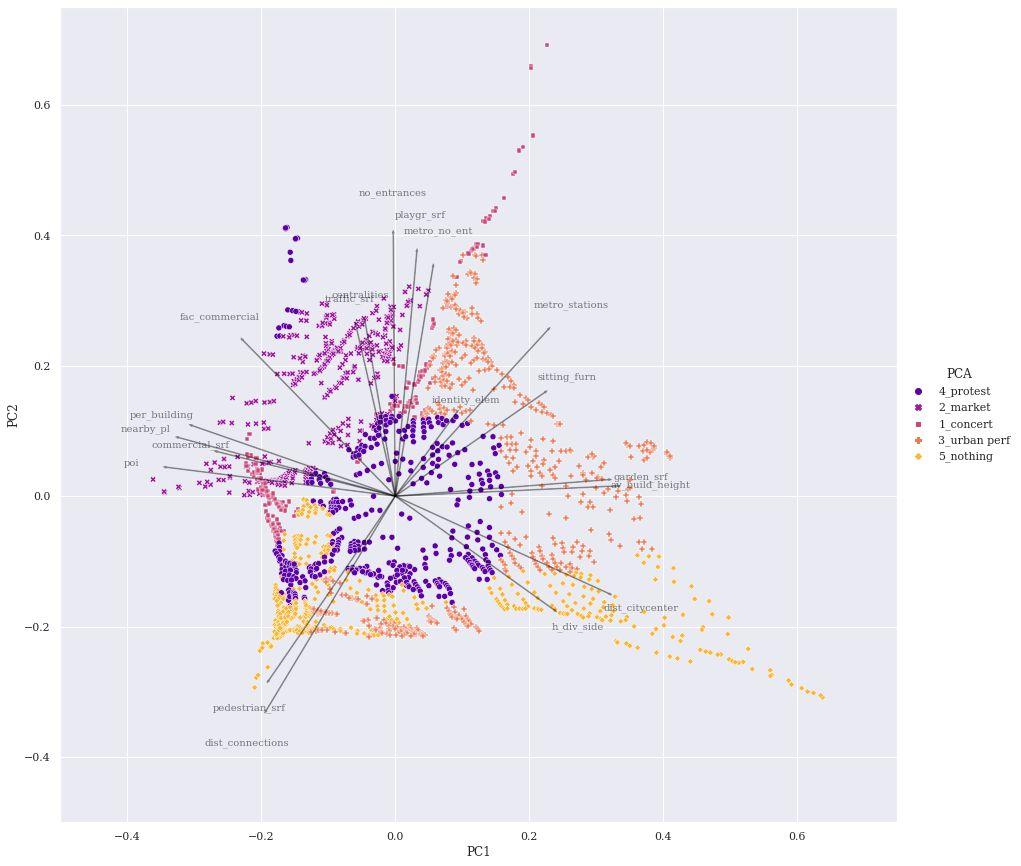
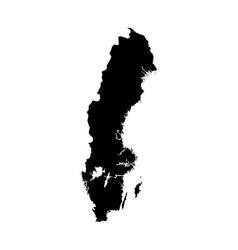
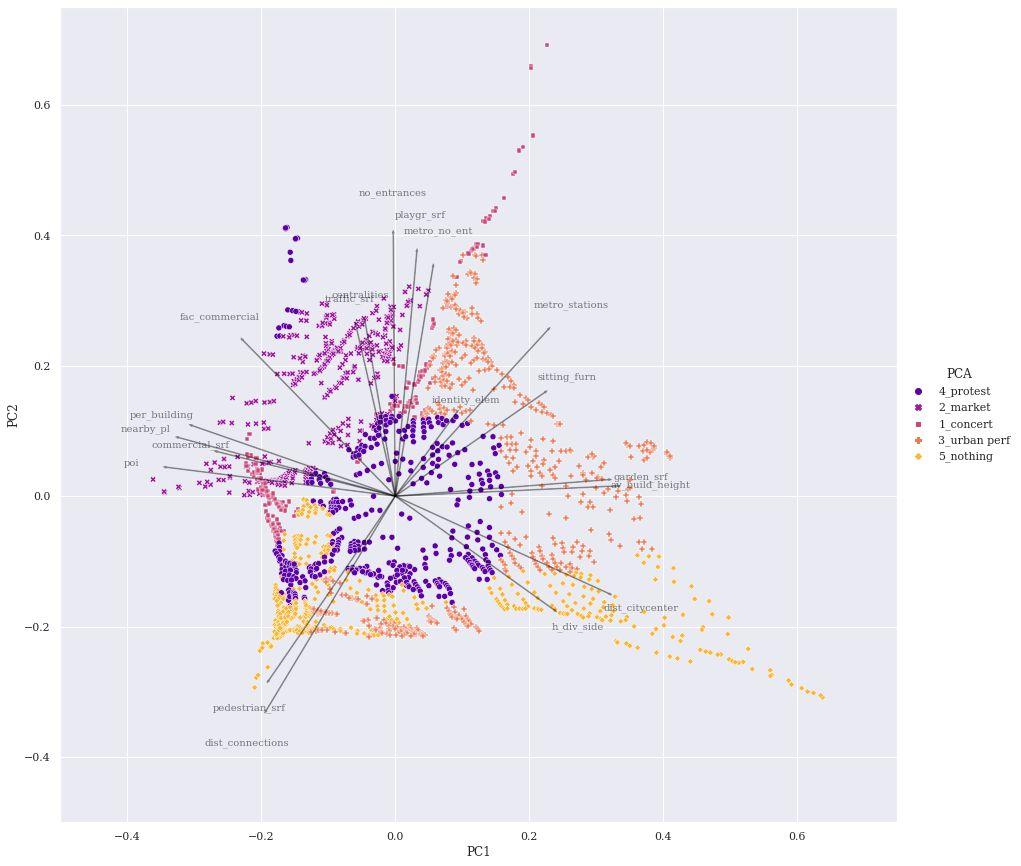
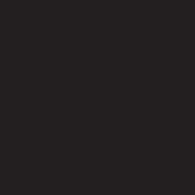
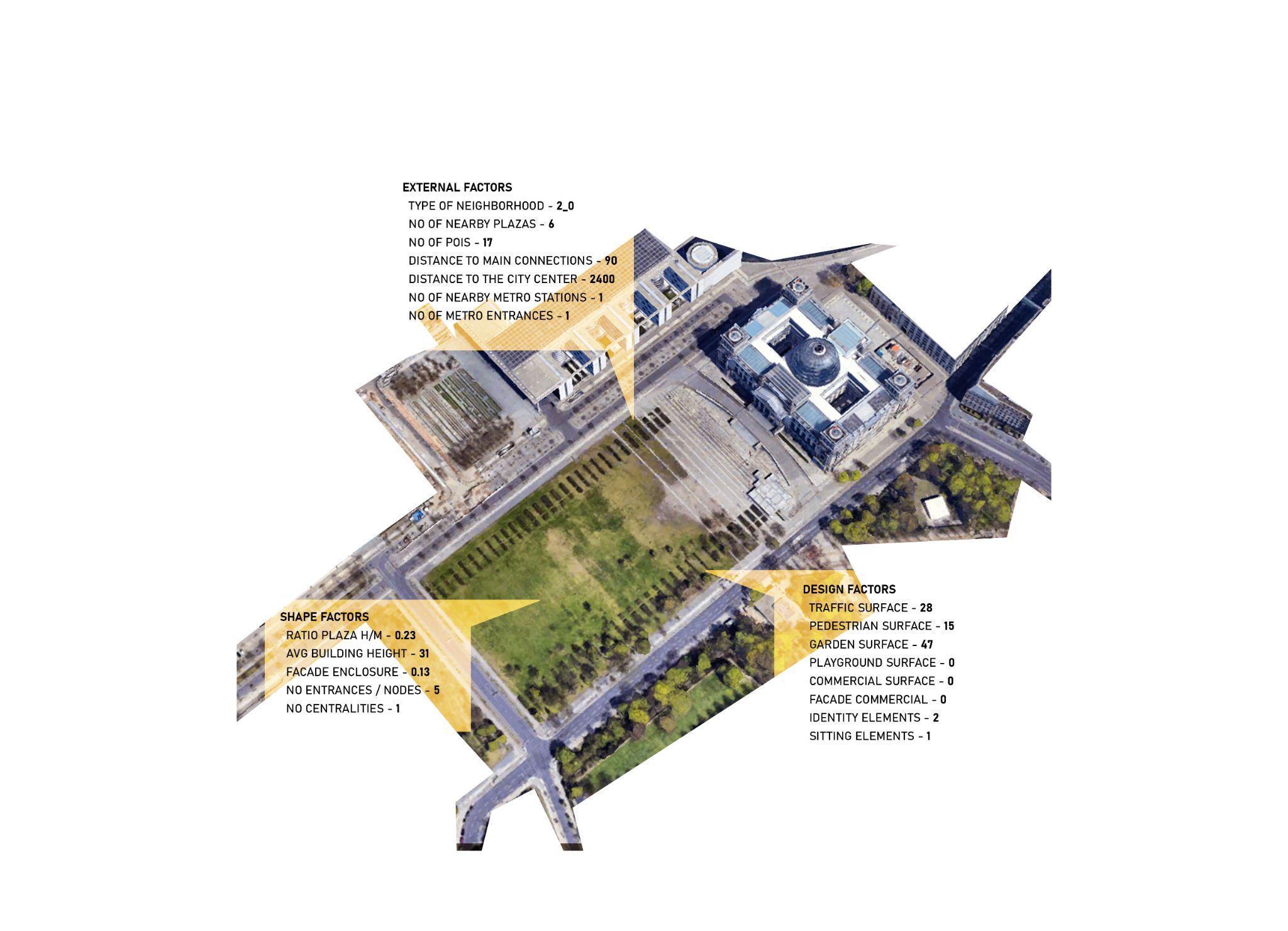
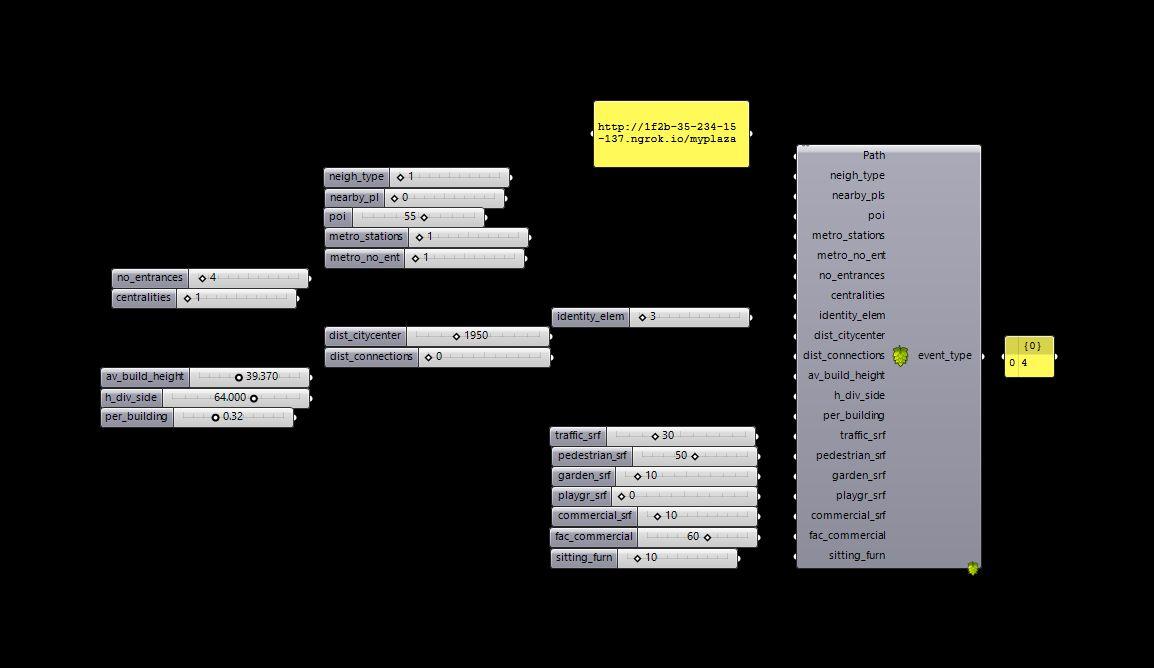
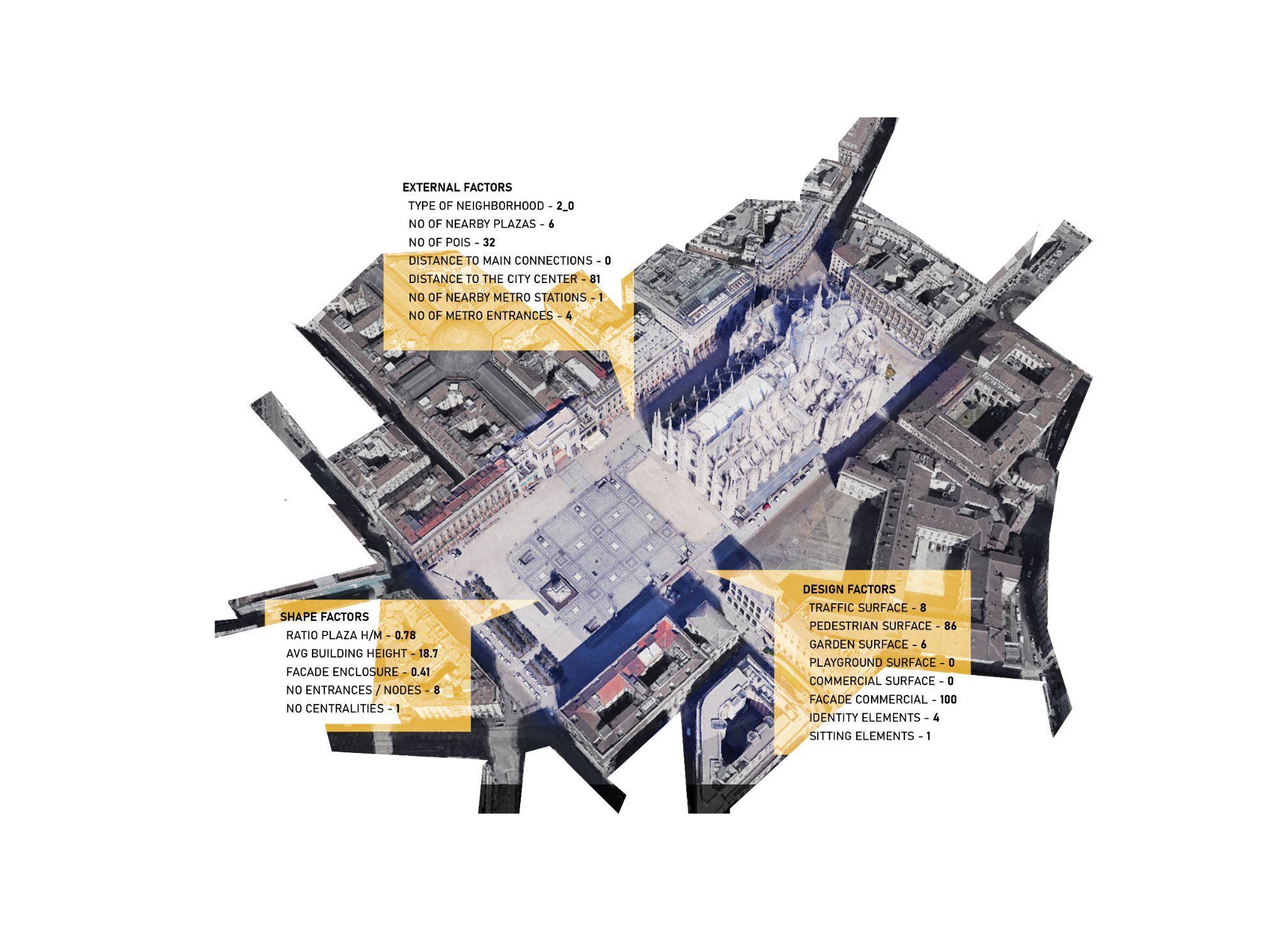
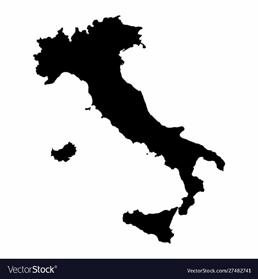
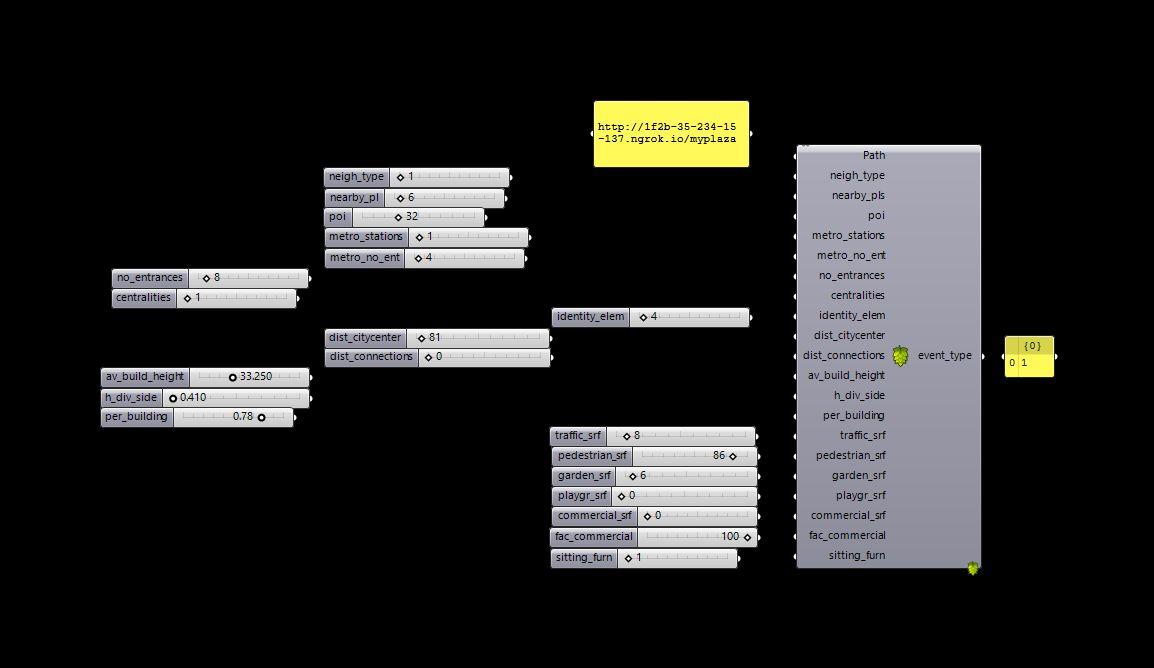
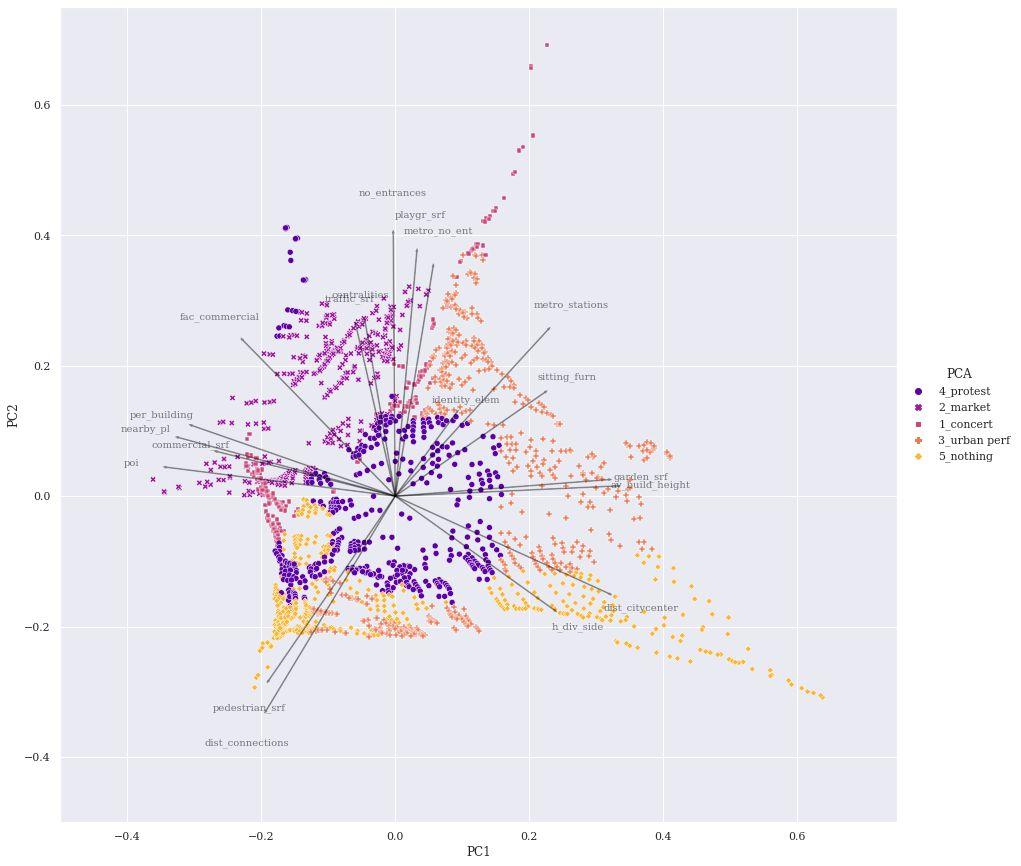
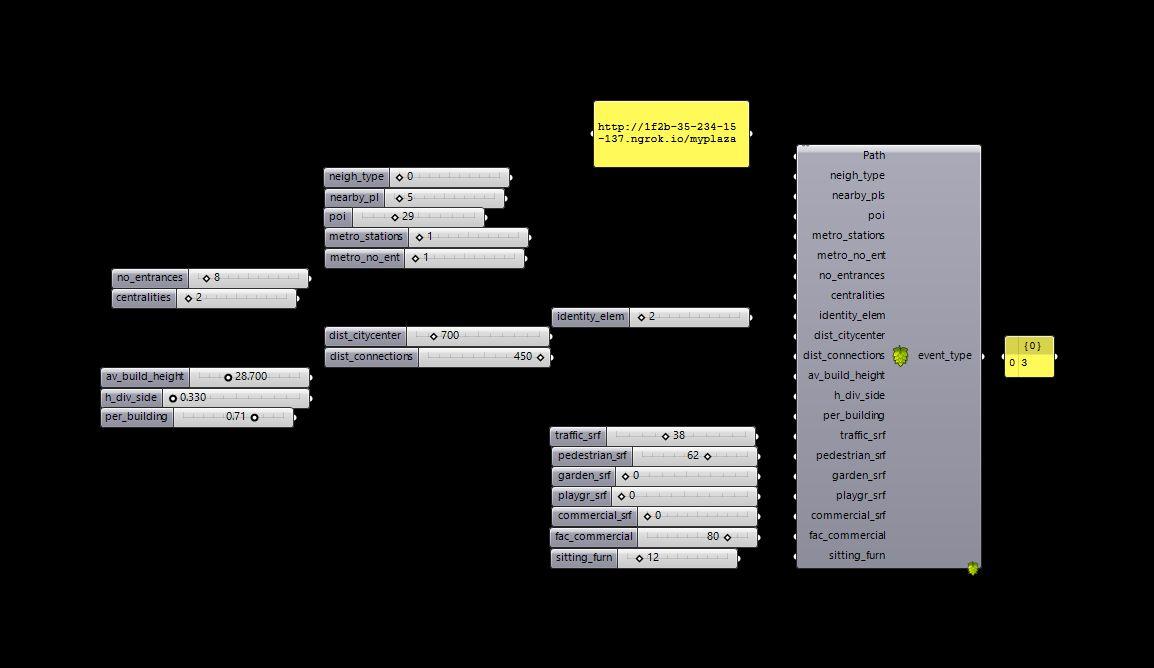
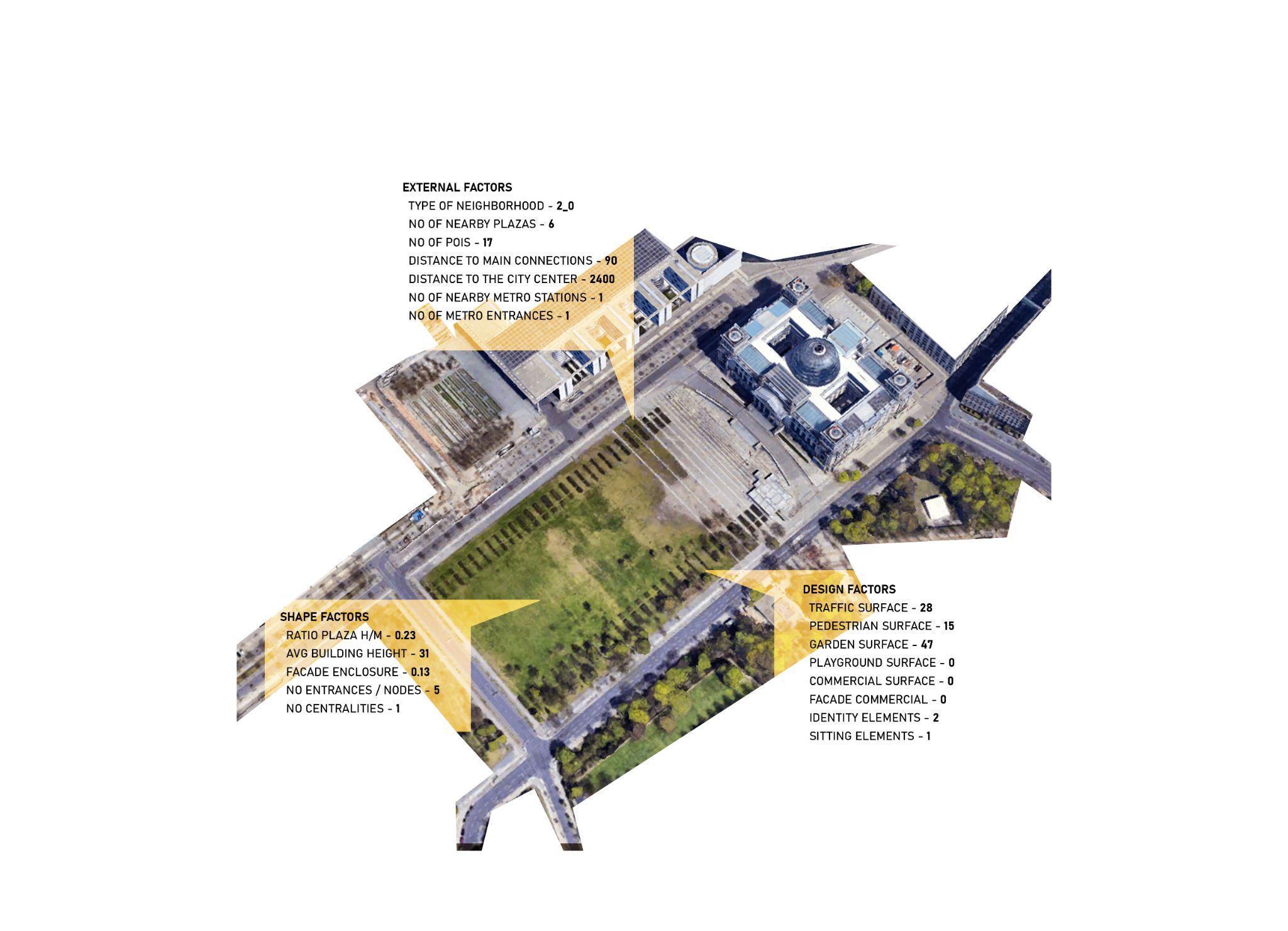
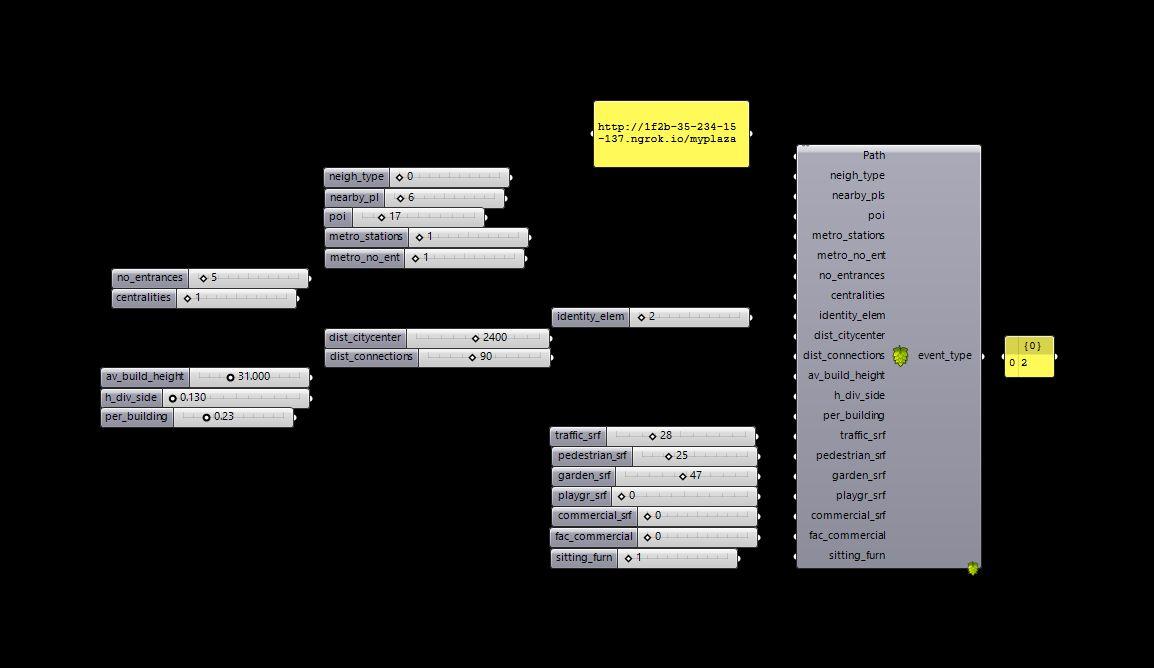
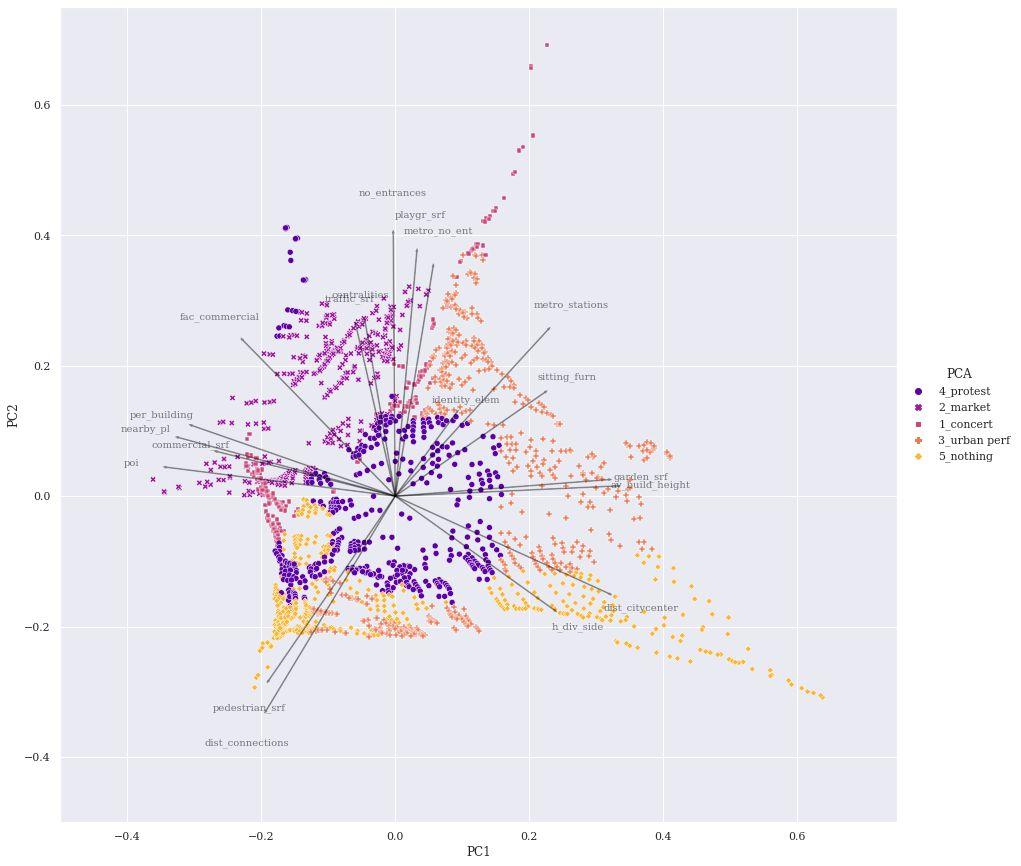
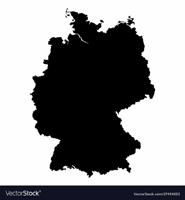
DEPTH CITY
16 Date: 2021 Collaborators: Jumana Hamdani Pablo Antuña Molina Salvador Calgua Instructor: Oana Taut Project Type: Academic at IAAC Tools: Rhino,Grasshopper, Point Cloud, Python, Google Colab, DLT model, MiDaS, PyTorch

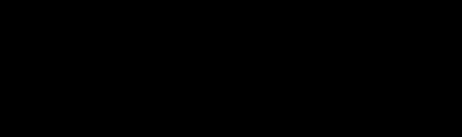
Design Intention
To create 3D space from 2D image
Method: Depth estimation is a crucial step towards inferring scene geometry from 2D images. The goal in monocular depth estimation is to predict the depth value of each pixel or inferring depth information, given only a single RGB image as input.
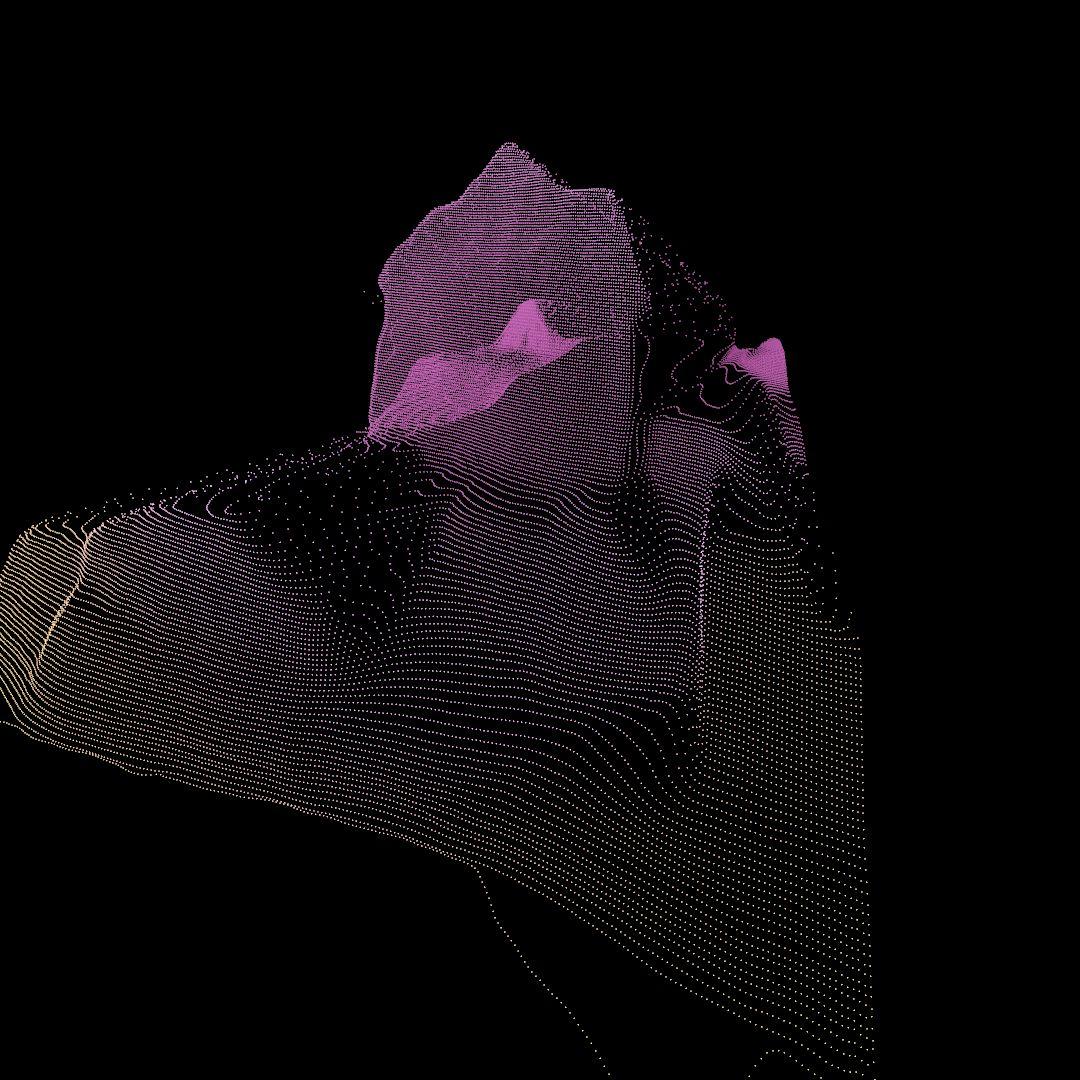
DEPTH CITY
Estimate Depth using DLT model
WORKFLOW Mode Dataset
Various datasets containing depth information are not compatible in terms of scale and bias. This is due to the diversity of measuring tools, including stereo cameras, laser scanners, and light sensors. Midas introduces a new loss function that absorbs these diversities, thereby eliminating compatibility issues and allowing multiple data sets to be used for training simultaneously.
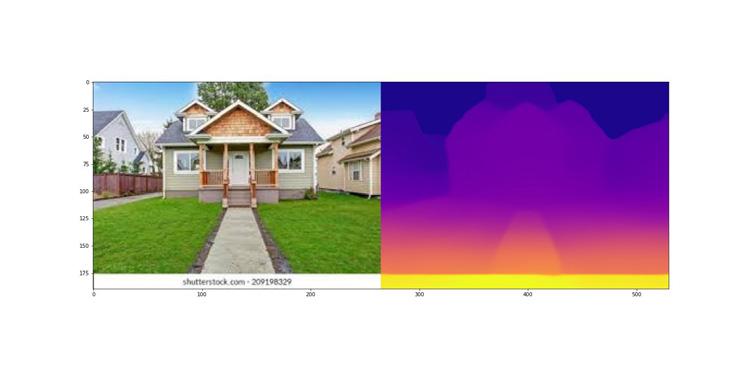
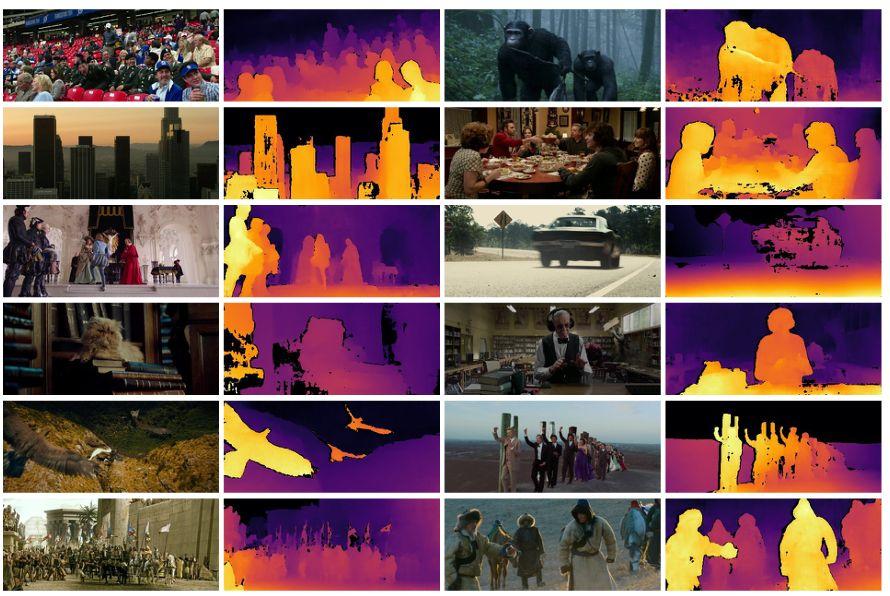
Develop tools that enable mixing diverse datasets to train a general model
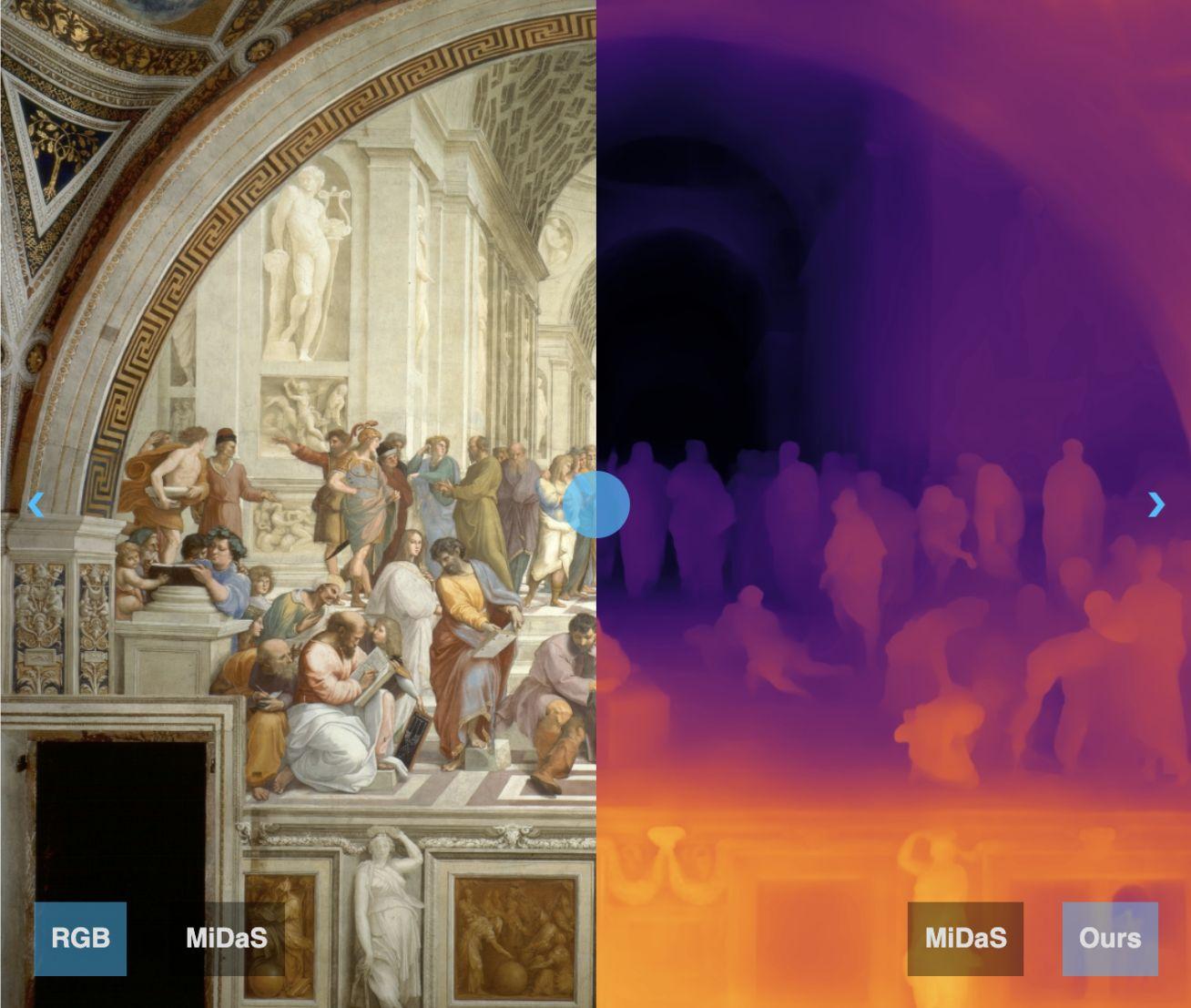
[-1, 256, 2, 2] 0 GroupNormAct-144 [-1, 256, 2, 2] 512 StdConv2dSame-145 [-1, 1024, 2, 2] 262,144 Identity-146 [-1, 1024, 2, 2] 0 GroupNormAct-147 [-1, 1024, 2, 2] 2,048 Identity-148 [-1, 1024, 2, 2] 0 ReLU-149 [-1, 1024, 2, 2] 0 Bottleneck-150 [-1, 1024, 2, 2] 0 StdConv2dSame-151 [-1, 256, 2, 2] 262,144 ReLU-152 [-1, 256, 2, 2] 0 GroupNormAct-153 [-1, 256, 2, 2] 512 StdConv2dSame-154 [-1, 256, 2, 2] 589,824 ReLU-155 [-1, 256, 2, 2] 0 GroupNormAct-156 [-1, 256, 2, 2] 512 StdConv2dSame-157 [-1, 1024, 2, 2] 262,144 Identity-158 [-1, 1024, 2, 2] 0 GroupNormAct-159 [-1, 1024, 2, 2] 2,048 Identity-160 [-1, 1024, 2, 2] 0 ReLU-161 [-1, 1024, 2, 2] 0 Bottleneck-162 [-1, 1024, 2, 2] 0 StdConv2dSame-163 [-1, 256, 2, 2] 262,144 ReLU-164 [-1, 256, 2, 2] 0 GroupNormAct-165 [-1, 256, 2, 2] 512 StdConv2dSame-166 [-1, 256, 2, 2] 589,824 ReLU-167 [-1, 256, 2, 2] 0 GroupNormAct-168 [-1, 256, 2, 2] 512 StdConv2dSame-169 [-1, 1024, 2, 2] 262,144 Identity-170 [-1, 1024, 2, 2] 0 GroupNormAct-171 [-1, 1024, 2, 2] 2,048 Identity-172 [-1, 1024, 2, 2] 0 ReLU-173 [-1, 1024, 2, 2] 0 Bottleneck-174 [-1, 1024, 2, 2] 0 StdConv2dSame-175 [-1, 256, 2, 2] 262,144 ReLU-176 [-1, 256, 2, 2] 0 GroupNormAct-177 [-1, 256, 2, 2] 512 StdConv2dSame-178 [-1, 256, 2, 2] 589,824 ReLU-179 [-1, 256, 2, 2] 0 GroupNormAct-180 [-1, 256, 2, 2] 512 StdConv2dSame-181 [-1, 1024, 2, 2] 262,144 Identity-182 [-1, 1024, 2, 2] 0 GroupNormAct-183 [-1, 1024, 2, 2] 2,048 Identity-184 [-1, 1024, 2, 2] 0 ReLU-185 [-1, 1024, 2, 2] 0 Bottleneck-186 [-1, 1024, 2, 2] 0 StdConv2dSame-187 [-1, 256, 2, 2] 262,144 ReLU-188 [-1, 256, 2, 2] 0 GroupNormAct-189 [-1, 256, 2, 2] 512 StdConv2dSame-190 [-1, 256, 2, 2] 589,824 ReLU-191 [-1, 256, 2, 2] 0 GroupNormAct-192 [-1, 256, 2, 2] 512 StdConv2dSame-193 [-1, 1024, 2, 2] 262,144 Identity-194 [-1, 1024, 2, 2] 0 GroupNormAct-195 [-1, 1024, 2, 2] 2,048 Identity-196 [-1, 1024, 2, 2] 0 ReLU-197 [-1, 1024, 2, 2] 0 Bottleneck-198 [-1, 1024, 2, 2] 0 StdConv2dSame-199 [-1, 256, 2, 2] 262,144 ReLU-200 [-1, 256, 2, 2] 0 GroupNormAct-201 [-1, 256, 2, 2] 512 StdConv2dSame-202 [-1, 256, 2, 2] 589,824 ReLU-203 [-1, 256, 2, 2] 0 GroupNormAct-204 [-1, 256, 2, 2] 512 StdConv2dSame-205 [-1, 1024,
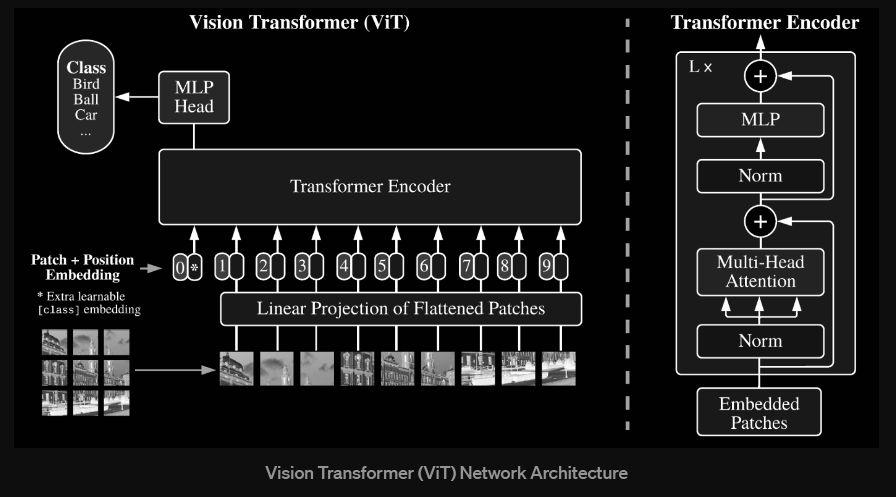
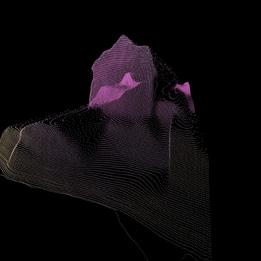
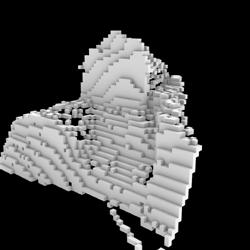
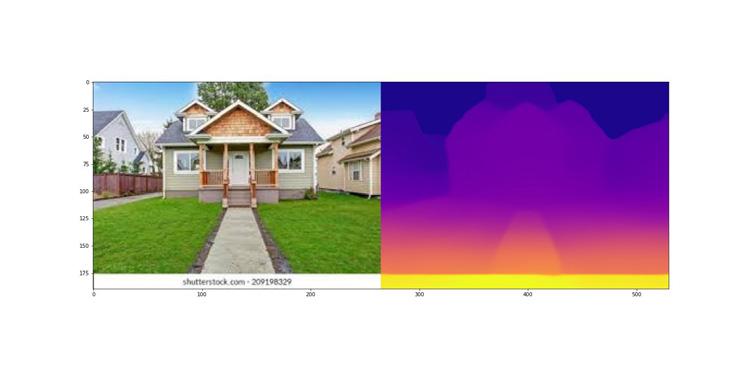
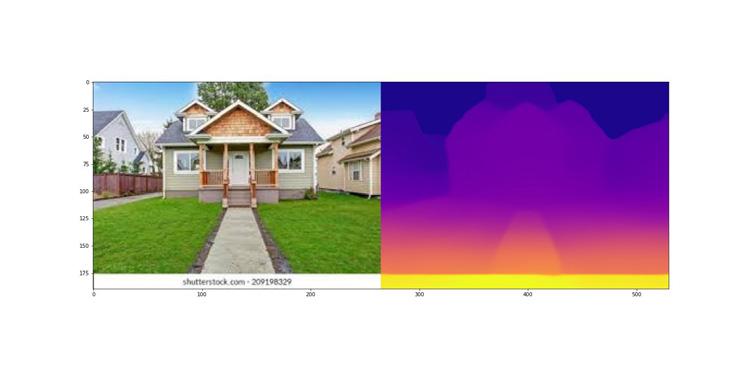
Dropout-266 [-1, 5, 768] 0 Mlp-267 [-1, 5, 768] 0 Identity-268 [-1, 5, 768] 0 Block-269 [-1, 5, 768] 0 LayerNorm-270 [-1, 5, 768] 1,536 Linear-271 [-1, 5, 2304] 1,771,776 Dropout-272 [-1, 12, 5, 5] 0 Linear-273 [-1, 5, 768] 590,592 Dropout-274 [-1, 5, 768] 0 Attention-275 [-1, 5, 768] 0 Identity-276 [-1, 5, 768] 0 LayerNorm-277 [-1, 5, 768] 1,536 Linear-278 [-1, 5, 3072] 2,362,368 GELU-279 [-1, 5, 3072] 0 Dropout-280 [-1, 5, 3072] 0 Linear-281 [-1, 5, 768] 2,360,064 Dropout-282 [-1, 5, 768] 0 Mlp-283 [-1, 5, 768] 0 Identity-284 [-1, 5, 768] 0 Block-285 [-1, 5, 768] 0 LayerNorm-286 [-1, 5, 768] 1,536 Linear-287 [-1, 5, 2304] 1,771,776 Dropout-288 [-1, 12, 5, 5] 0 Linear-289 [-1, 5, 768] 590,592 Dropout-290 [-1, 5, 768] 0 Attention-291 [-1, 5, 768] 0 Identity-292 [-1, 5, 768] 0 LayerNorm-293 [-1, 5, 768] 1,536 Linear-294 [-1, 5, 3072] 2,362,368 GELU-295 [-1, 5, 3072] 0 Dropout-296 [-1, 5, 3072] 0 Linear-297 [-1, 5, 768] 2,360,064 Dropout-298 [-1, 5, 768] 0 Mlp-299 [-1, 5, 768] 0 Identity-300 [-1, 5, 768] 0 Block-301 [-1, 5, 768] 0 LayerNorm-302 [-1, 5, 768] 1,536 Linear-303 [-1, 5, 2304] 1,771,776 Dropout-304 [-1, 12, 5, 5] 0 Linear-305 [-1, 5, 768] 590,592 Dropout-306 [-1, 5, 768] 0 Attention-307 [-1, 5, 768] 0 Identity-308 [-1, 5, 768] 0 LayerNorm-309 [-1, 5, 768] 1,536 Linear-310 [-1, 5, 3072] 2,362,368 GELU-311 [-1, 5, 3072] 0 Dropout-312 [-1, 5, 3072] 0 Linear-313 [-1, 5, 768] 2,360,064 Dropout-314 [-1, 5, 768] 0 Mlp-315 [-1, 5, 768] 0 Identity-316 [-1, 5, 768] 0 Block-317 [-1, 5, 768] 0 LayerNorm-318 [-1, 5, 768] 1,536 Linear-319 [-1, 5, 2304] 1,771,776 Dropout-320 [-1, 12, 5, 5] 0 Linear-321 [-1, 5, 768] 590,592 Dropout-322 [-1, 5, 768] 0 Attention-323 [-1, 5, 768] 0 Identity-324 [-1, 5, 768] 0 LayerNorm-325 [-1, 5, 768] 1,536 Linear-326 [-1, 5, 3072] 2,362,368 GELU-327 [-1, 5, 3072] 0 Dropout-328 [-1, 5, 3072] 0 Linear-329 [-1, 5, 768] 2,360,064 Dropout-330 [-1, 5, 768] 0 Mlp-331 [-1, 5, 768] 0 Identity-332 [-1, 5, 768] 0 Block-333 [-1, 5, 768] 0 LayerNorm-334 [-1, 5, 768] 1,536 Linear-335 [-1, 5, 2304] 1,771,776 Dropout-336 [-1, 12, 5, 5] 0 Linear-337 [-1, 5, 768] 590,592 Dropout-338 [-1, 5, 768] 0 Attention-339 [-1, 5, 768] 0 Identity-340 [-1, 5, 768] 0 LayerNorm-341 [-1, 5, 768] 1,536 Linear-342 [-1, 5, 3072] 2,362,368 GELU-343 [-1, 5, 3072] 0 Dropout-344 [-1, 5, 3072] 0 Linear-345 [-1, 5, 768] 2,360,064 Dropout-346 [-1, 5, 768] 0 Mlp-347 [-1, 5, 768] 0 Identity-348 [-1, 5, 768] 0 Block-349 [-1, 5, 768] 0 LayerNorm-350 [-1, 5, 768] 1,536 Linear-351 [-1, 5, 2304] 1,771,776 Dropout-352 [-1, 12, 5, 5] 0 Linear-353 [-1, 5, 768] 590,592 Dropout-354 [-1, 5, 768] 0 Attention-355 [-1, 5, 768] 0 Identity-356 [-1, 5, 768] 0 LayerNorm-357 [-1, 5, 768] 1,536 Linear-358 [-1, 5, 3072] 2,362,368 GELU-359 [-1, 5, 3072] 0 Dropout-360 [-1, 5, 3072] 0 Linear-361 [-1, 5, 768] 2,360,064 Dropout-362 [-1, 5, 768] 0 Mlp-363 [-1, 5, 768] 0 Identity-364 [-1, 5, 768] 0 Block-365 [-1, 5, 768] 0 LayerNorm-366 [-1, 5, 768] 1,536 Linear-367 [-1, 5, 2304] 1,771,776 Dropout-368 [-1, 12, 5, 5] 0 Linear-369 [-1, 5, 768] 590,592 Dropout-370 [-1, 5, 768] 0 Attention-371
768] 2,360,064 Dropout-410 [-1, 5, 768] 0 Mlp-411 [-1, 5, 768] 0 Identity-412 [-1, 5, 768] 0 Block-413 [-1, 5, 768] 0 LayerNorm-414 [-1, 5, 768] 1,536 Identity-415 [-1, 256, 8, 8] 0 Identity-416 [-1, 256, 8, 8] 0 Identity-417 [-1, 512, 4, 4] 0 Identity-418 [-1, 512, 4, 4] 0 Linear-419 [-1, 4, 768] 1,180,416 GELU-420 [-1, 4, 768] 0 ProjectReadout-421 [-1, 4, 768] 0 Transpose-422 [-1, 768, 4] 0 Linear-423 [-1, 4, 768] 1,180,416 GELU-424 [-1, 4, 768] 0 ProjectReadout-425 [-1, 4, 768] 0 Transpose-426 [-1, 768, 4] 0 Conv2d-427 [-1, 768, 2, 2] 590,592 Conv2d-428 [-1, 768, 2, 2] 590,592 Conv2d-429 [-1, 768, 1, 1] 5,309,184 Conv2d-430 [-1, 256, 8, 8] 589,824 Conv2d-431 [-1, 256, 4, 4] 1,179,648 Conv2d-432 [-1, 256, 2, 2] 1,769,472 Conv2d-433 [-1, 256, 1, 1] 1,769,472 ReLU-434 [-1, 256, 1, 1] 0 ReLU-435 [-1, 256, 1, 1] 0 Conv2d-436 [-1, 256, 1, 1] 590,080 ReLU-437 [-1, 256, 1, 1] 0 ReLU-438 [-1, 256, 1, 1] 0 Conv2d-439 [-1, 256, 1, 1] 590,080 Identity-440 [-1, 256, 1, 1] 0 ResidualConvUnit_custom-441 [-1, 256, 1, 1] 0 Conv2d-442 [-1, 256, 2, 2] 65,792 FeatureFusionBlock_custom-443 [-1, 256, 2, 2] 0 ReLU-444 [-1, 256, 2, 2] 0 ReLU-445 [-1, 256, 2, 2] 0 Conv2d-446 [-1, 256, 2, 2] 590,080 ReLU-447 [-1, 256, 2, 2] 0 ReLU-448 [-1, 256, 2, 2] 0 Conv2d-449 [-1, 256, 2, 2] 590,080 Identity-450 [-1, 256, 2, 2] 0 ResidualConvUnit_custom-451 [-1, 256, 2, 2] 0 Identity-452 [-1, 256, 2, 2] 0 ReLU-453 [-1, 256, 2, 2] 0 ReLU-454 [-1, 256, 2, 2] 0 Conv2d-455 [-1, 256, 2, 2] 590,080 ReLU-456 [-1, 256, 2, 2] 0 ReLU-457 [-1, 256, 2, 2] 0 Conv2d-458 [-1, 256, 2, 2] 590,080 Identity-459 [-1, 256, 2, 2] 0
DEPTH CITY
2D RGB IMAGES
Side by side comparison of image and depth map
An approach to Dense Depth estimation from a single image
Depth to Point Cloud
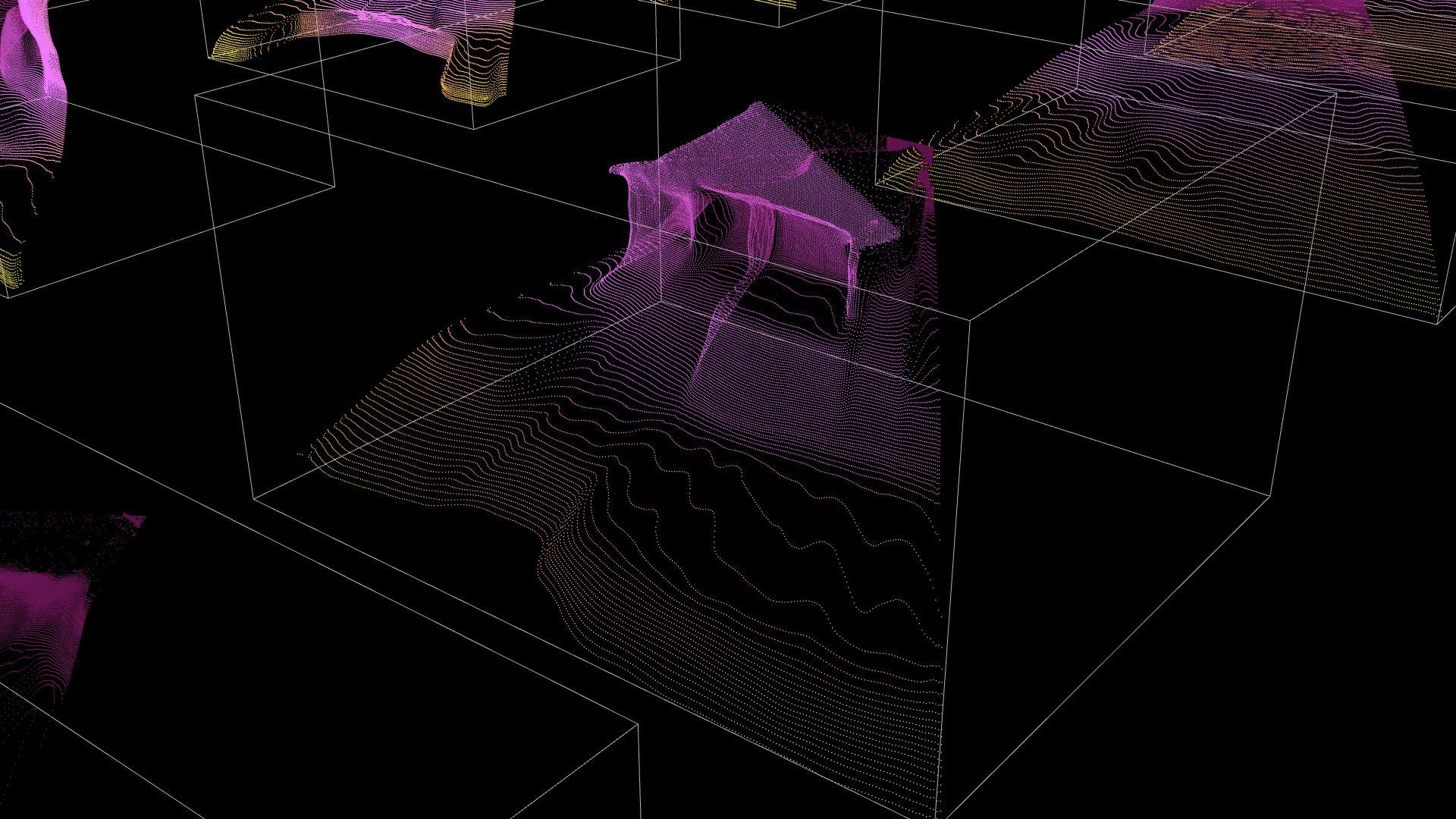
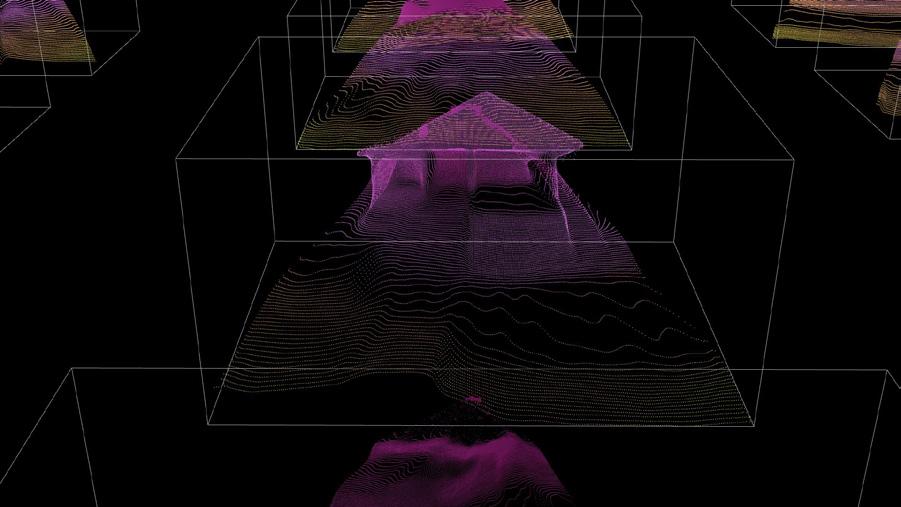
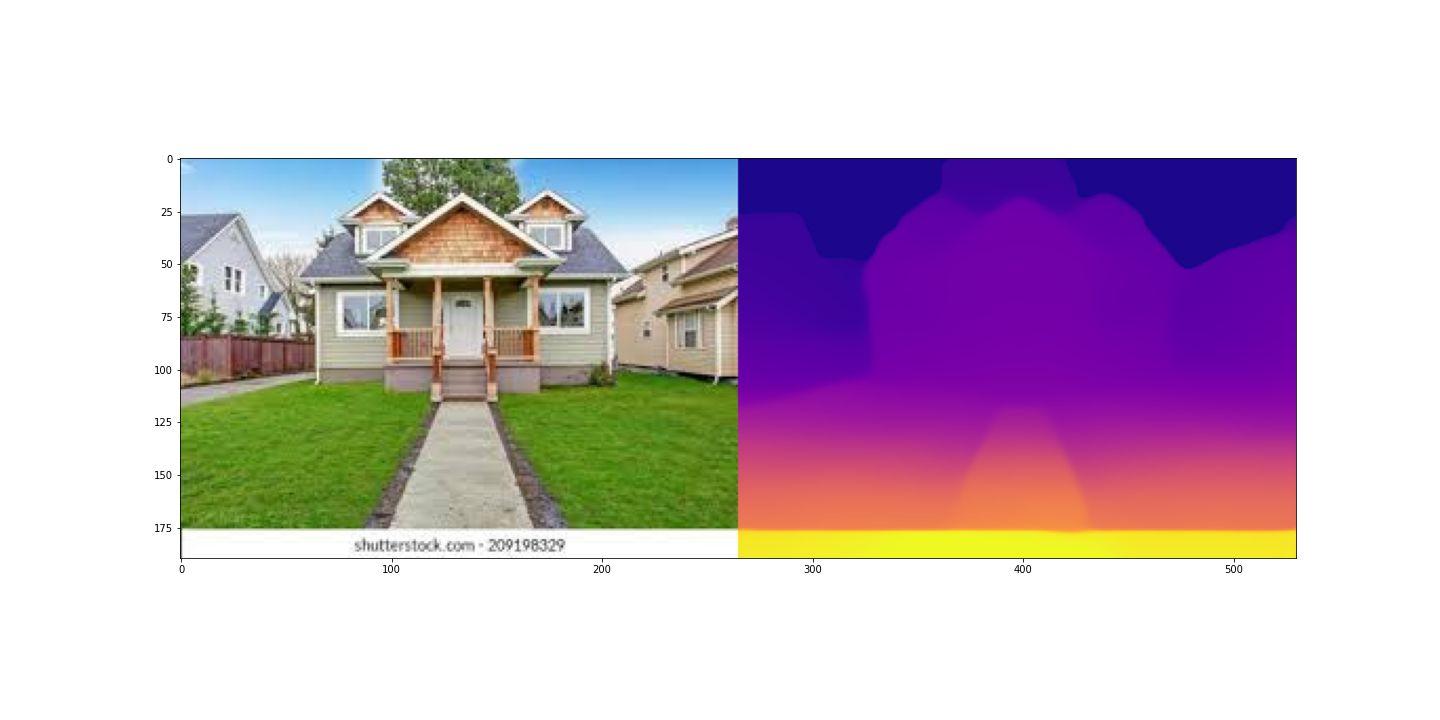
Side by side comparison of image and depth map
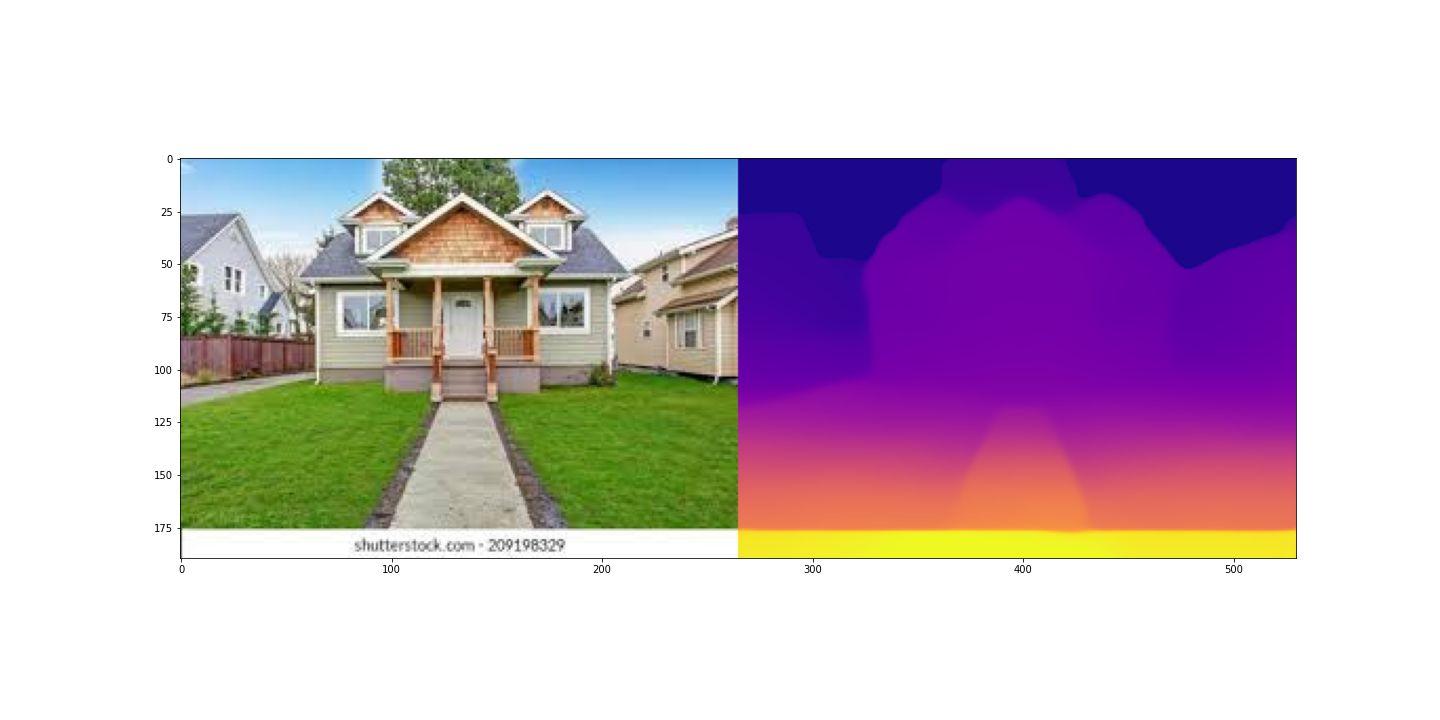
Depth Map to Point Cloud
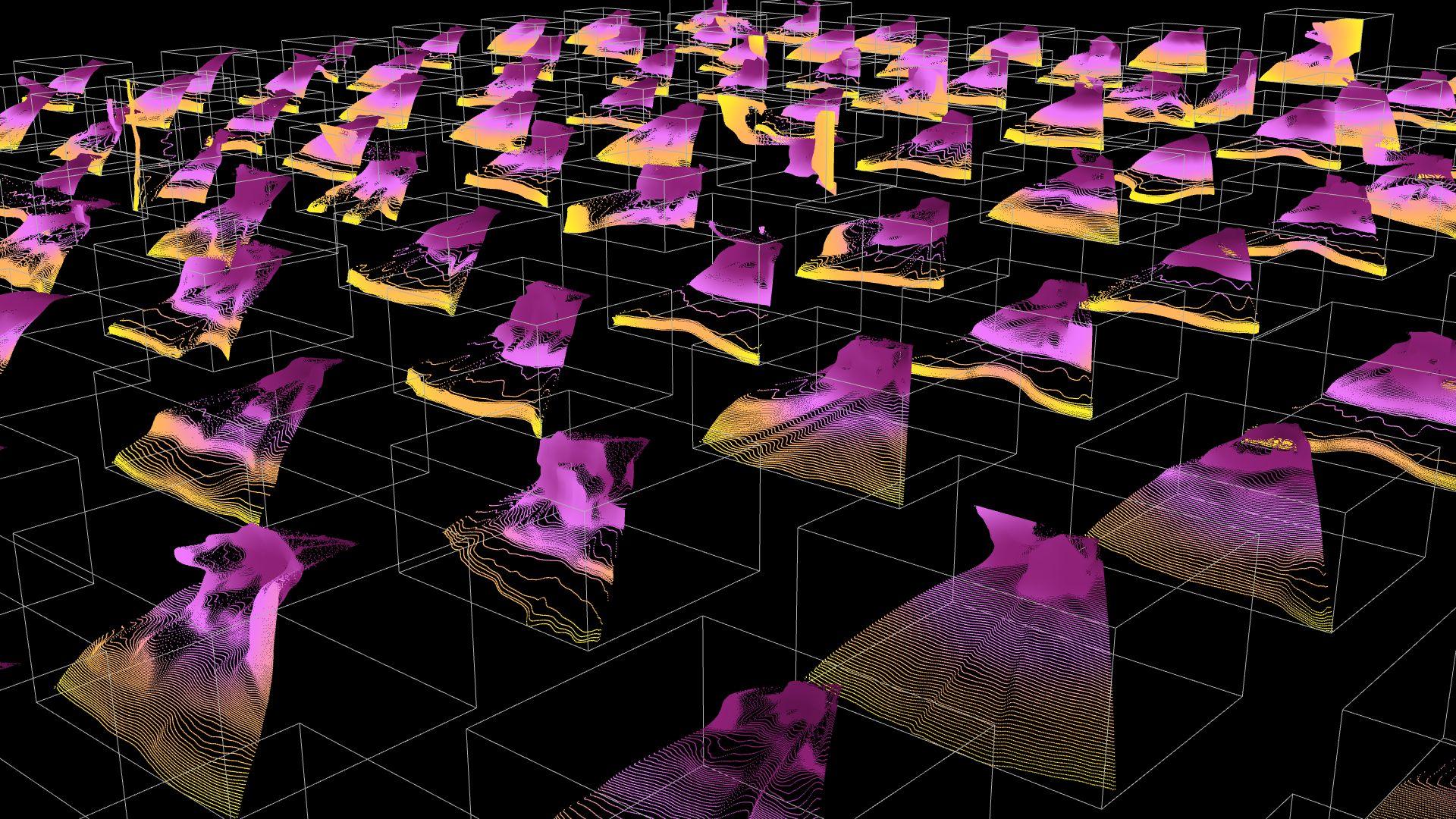
DEPTH CITY
convert depth to 3d point cloud convert 3d point cloud to voxels
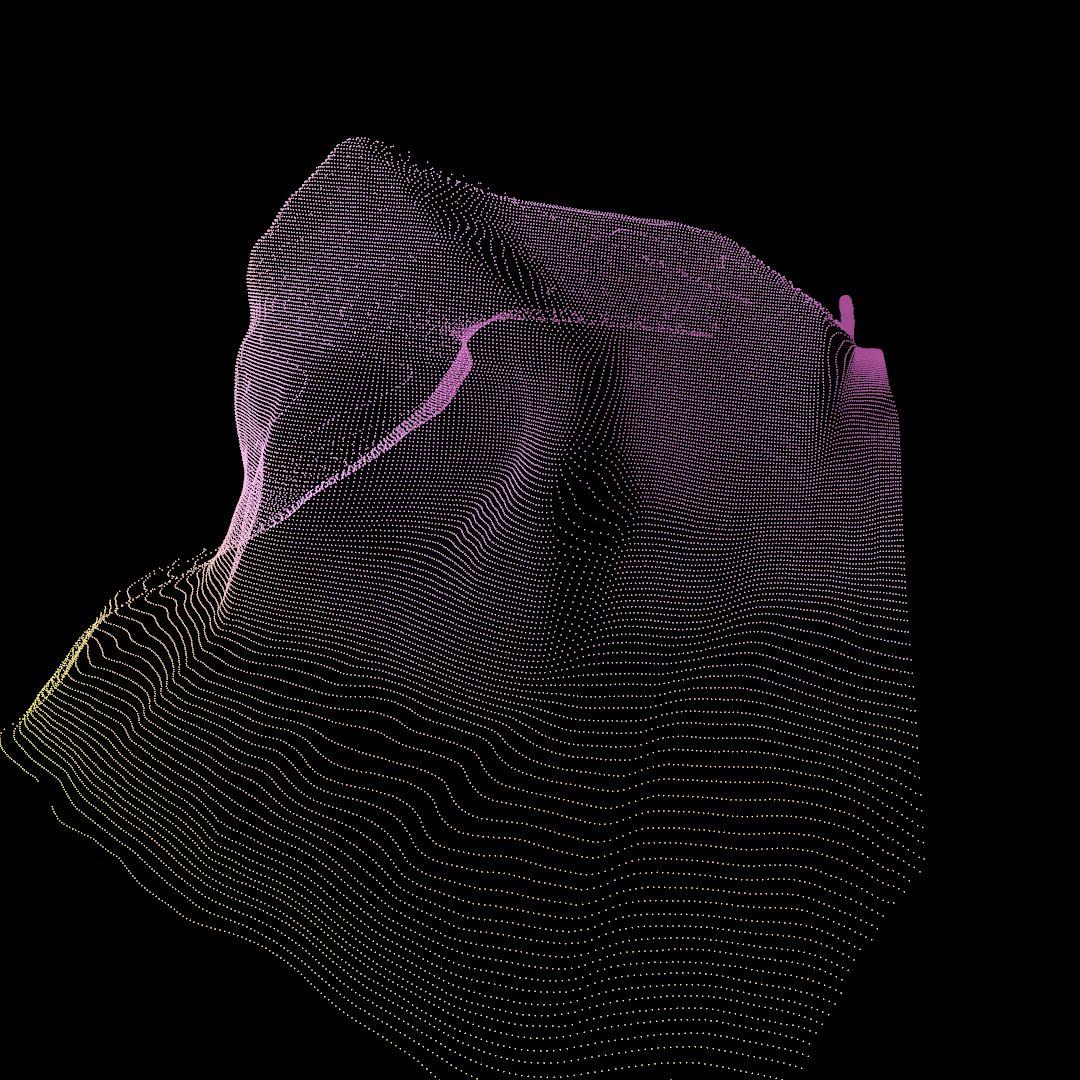
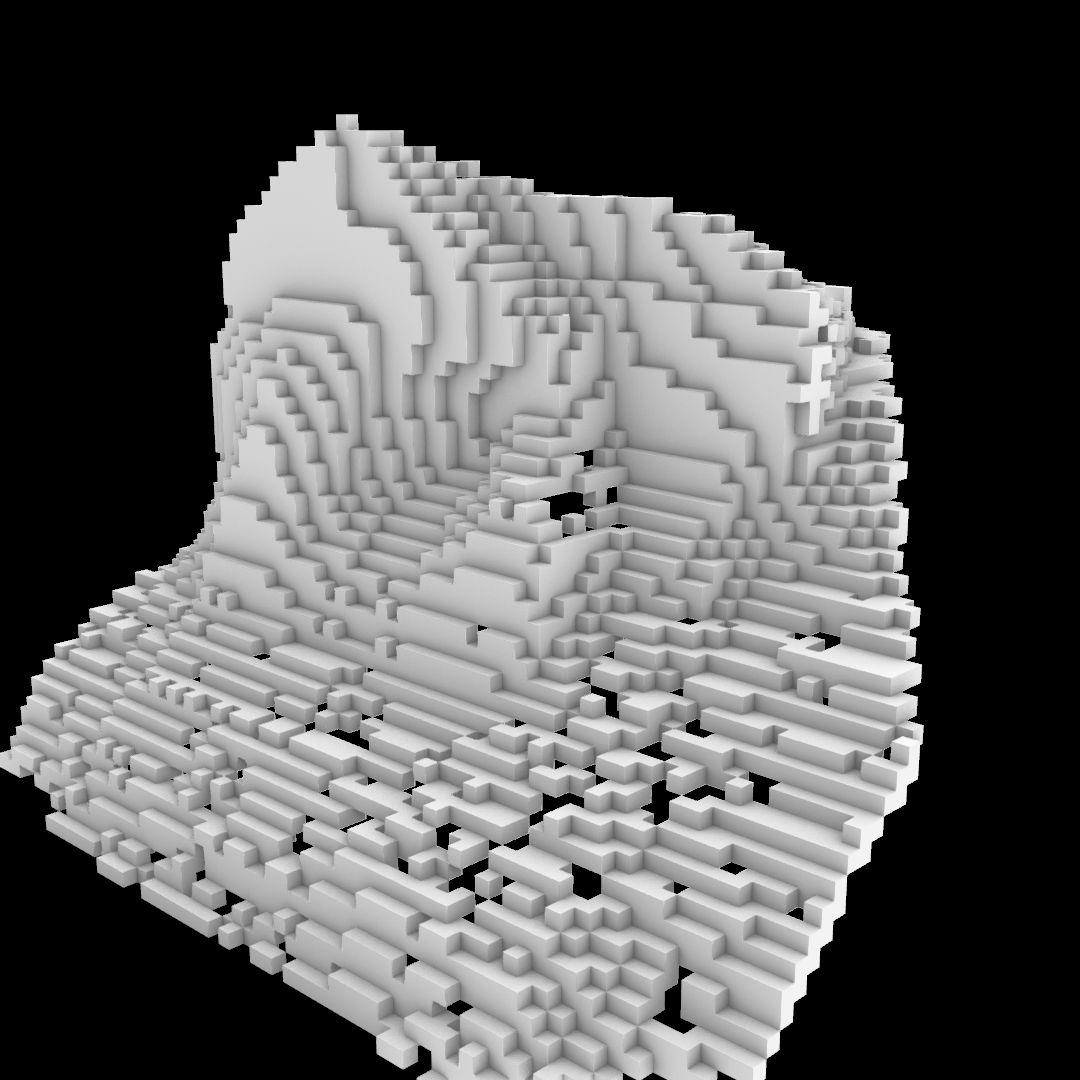
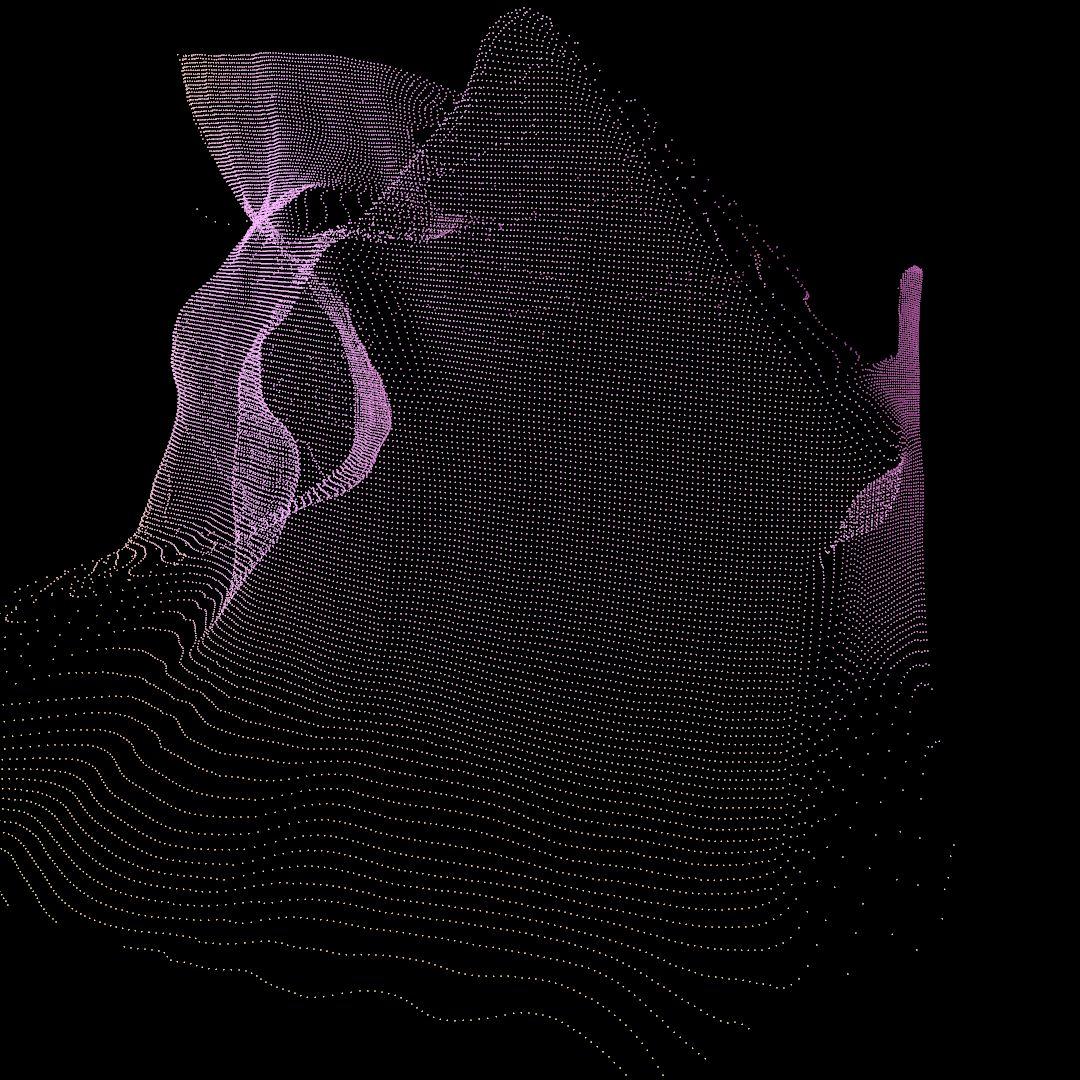
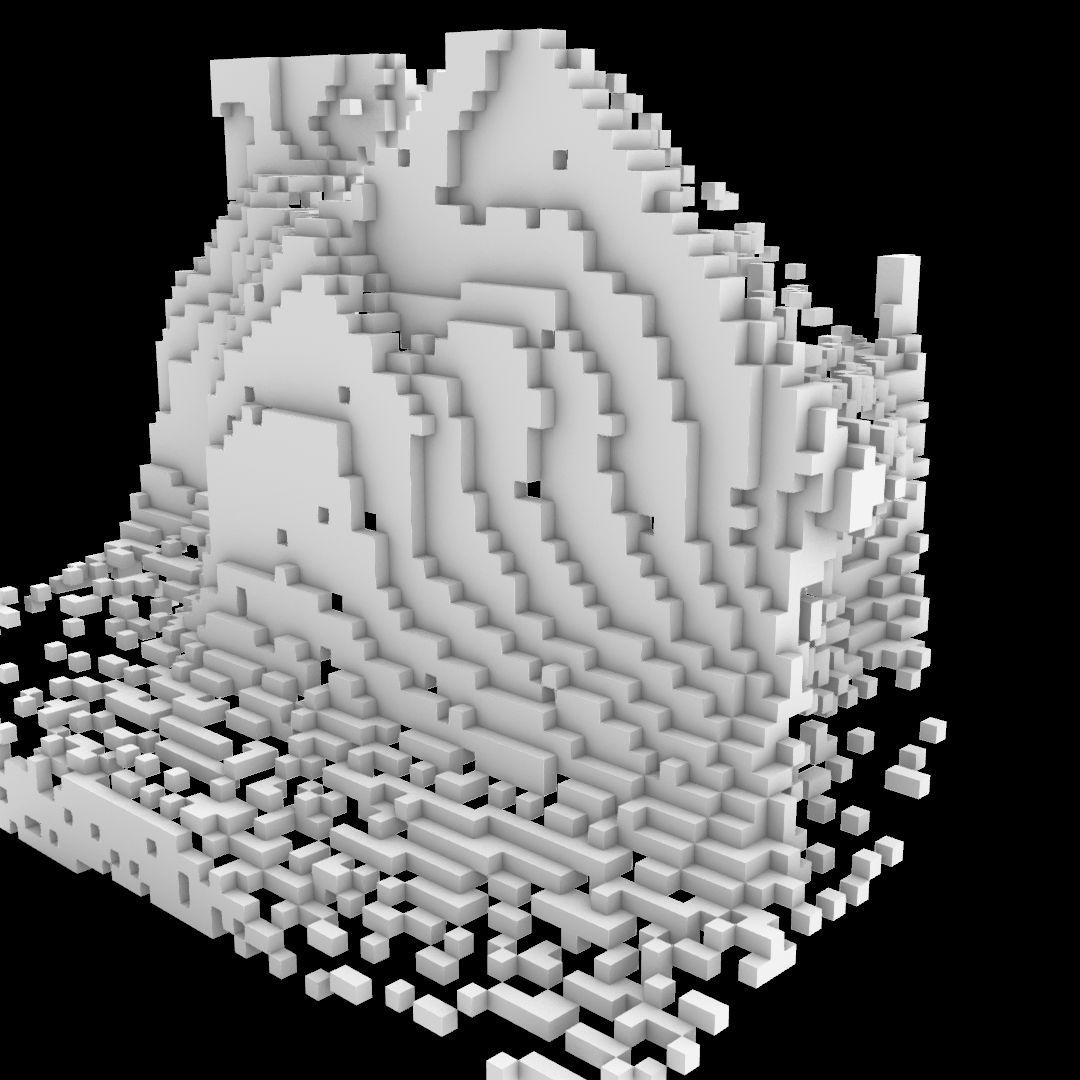
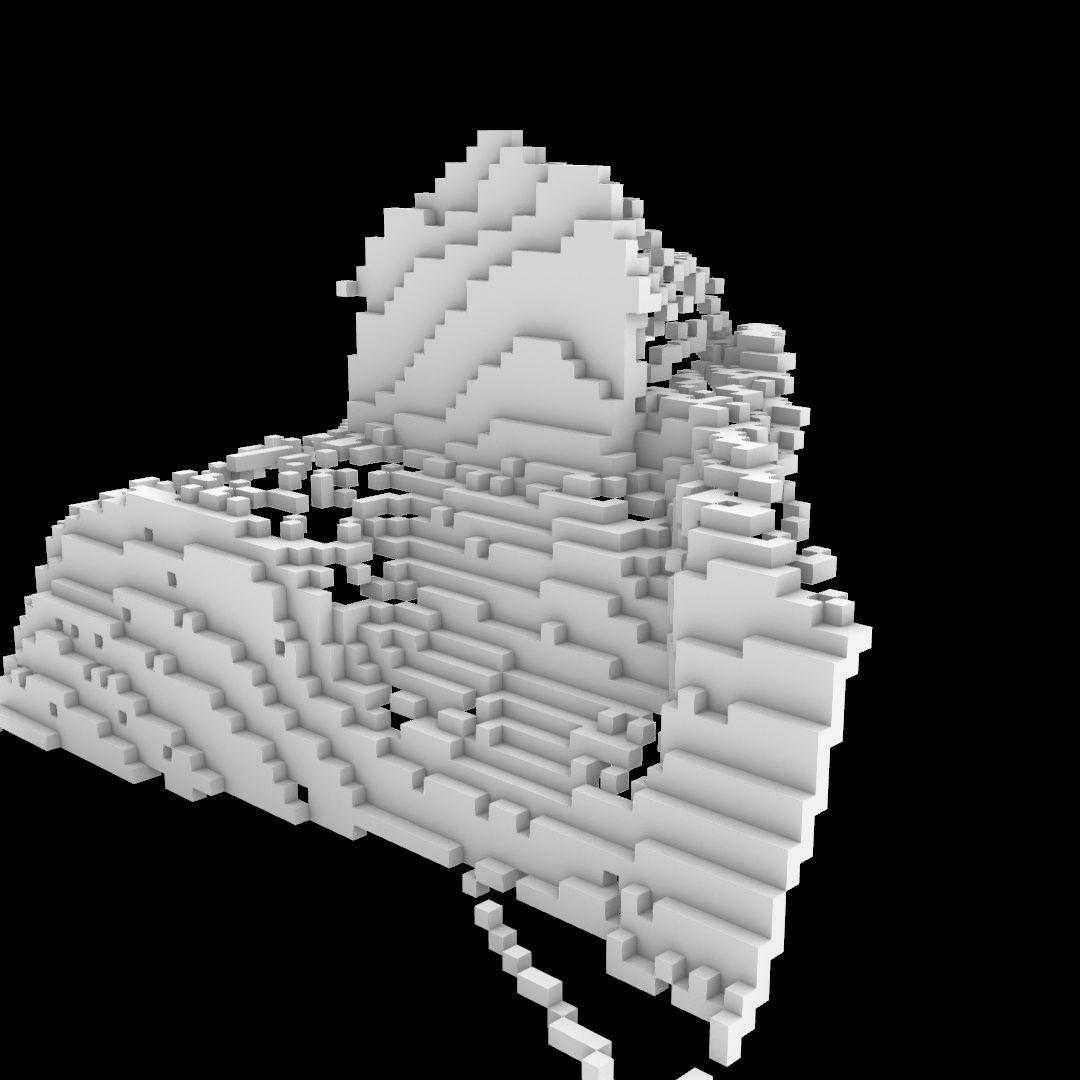
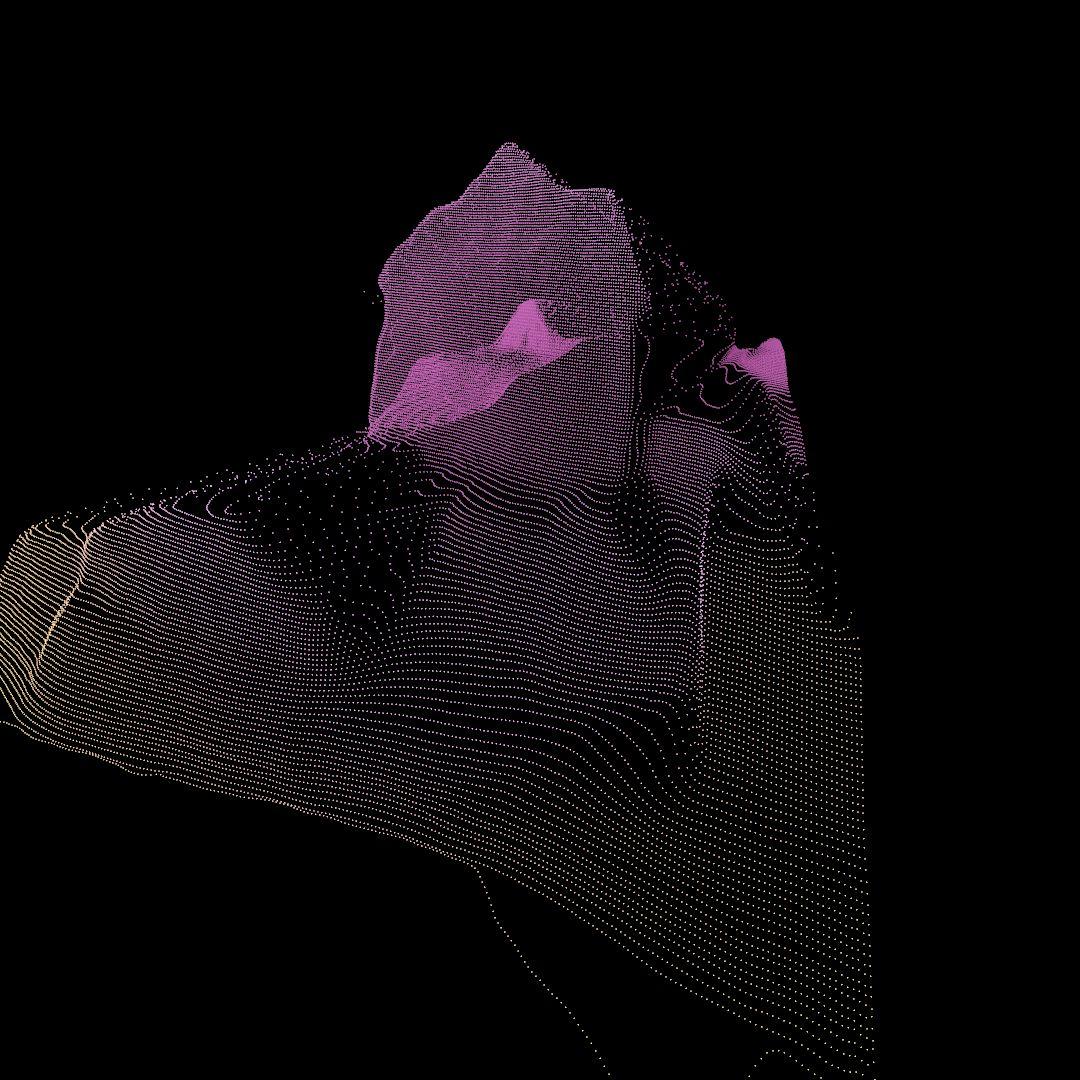
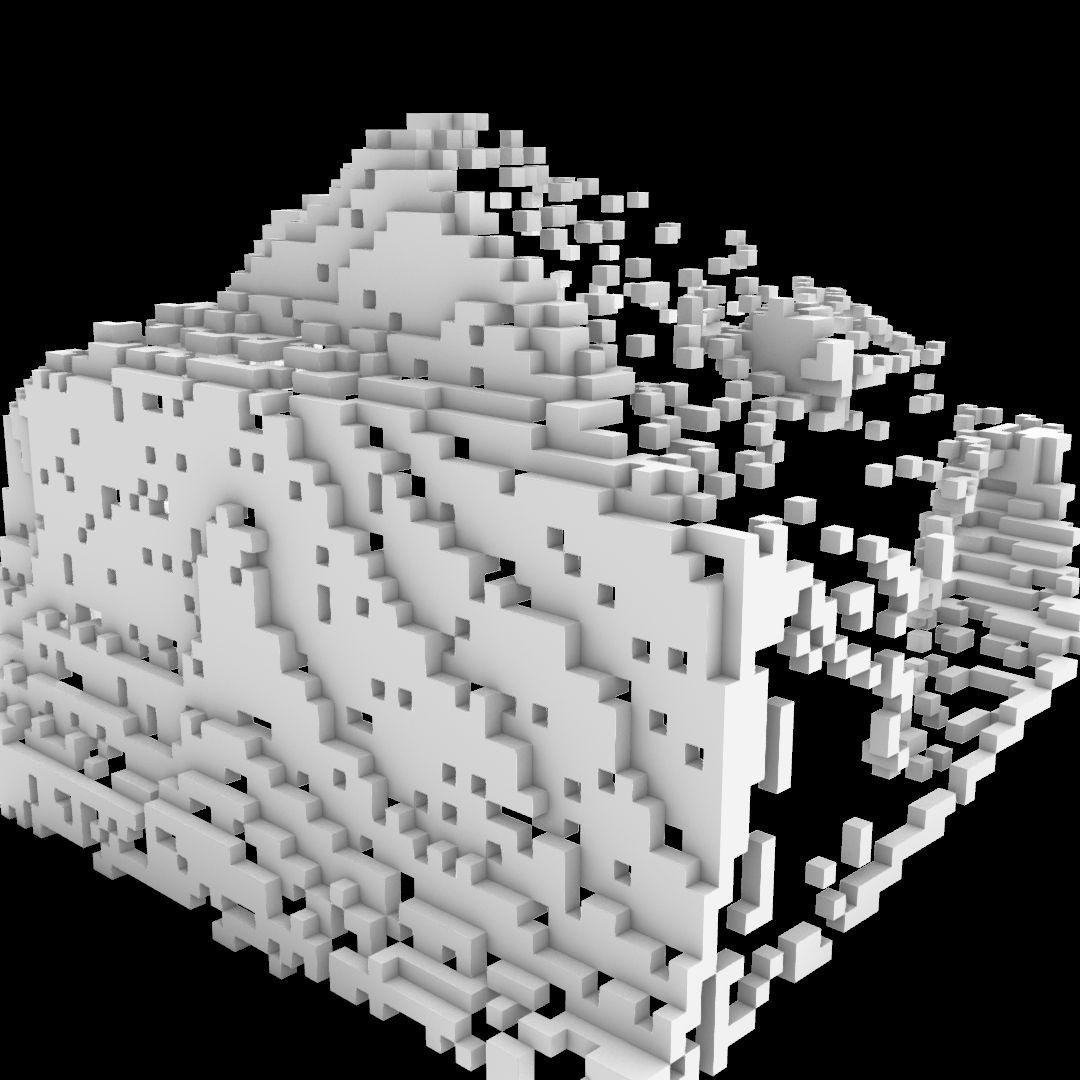
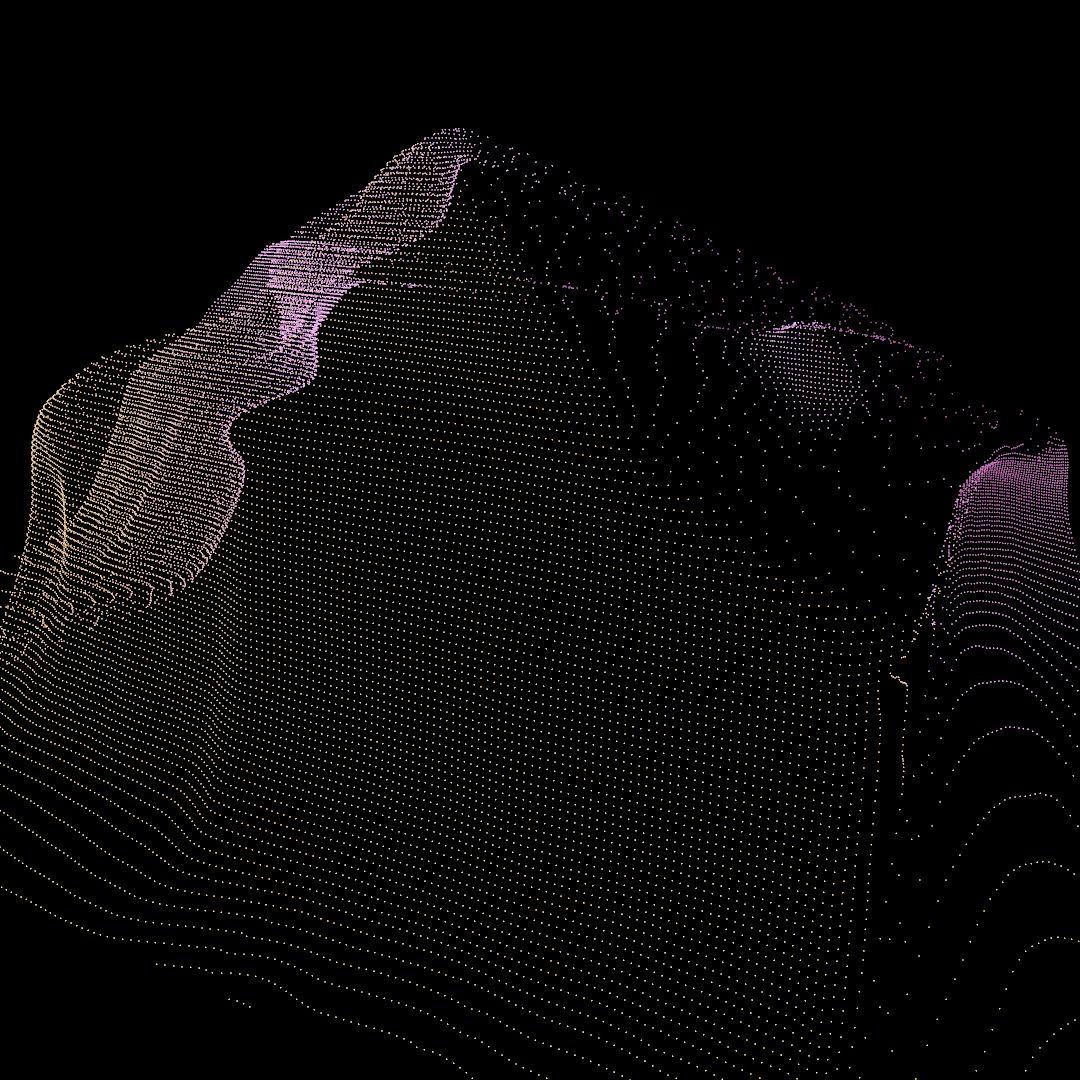
DEPTH
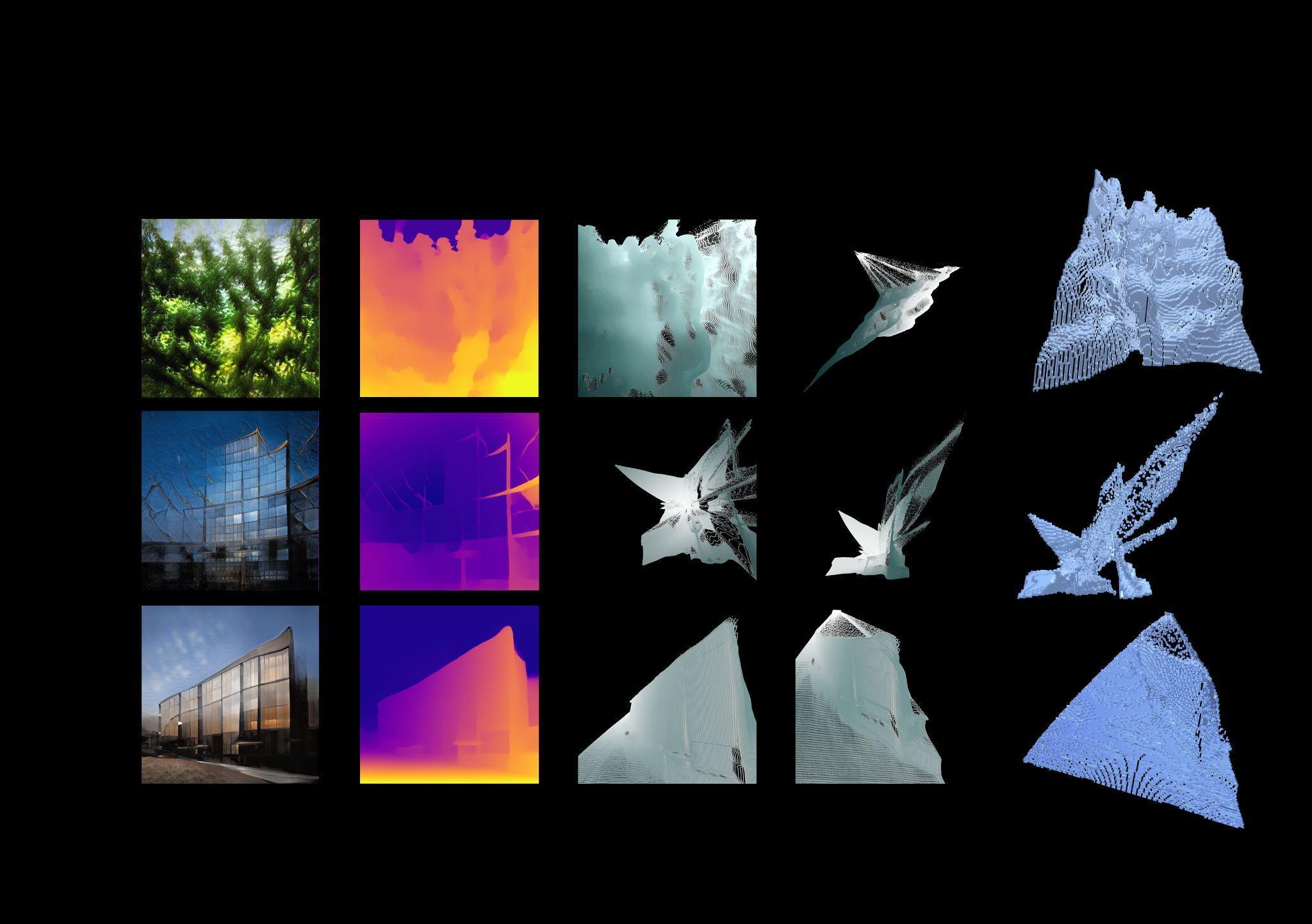
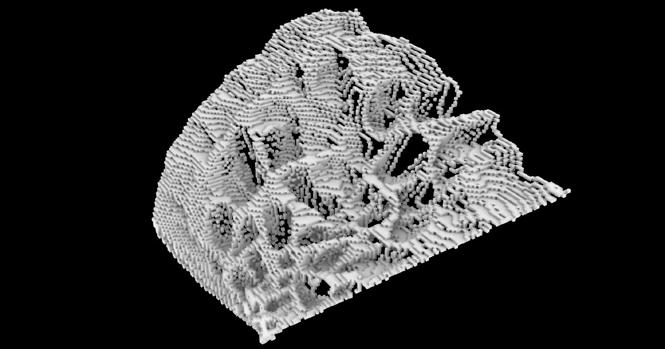
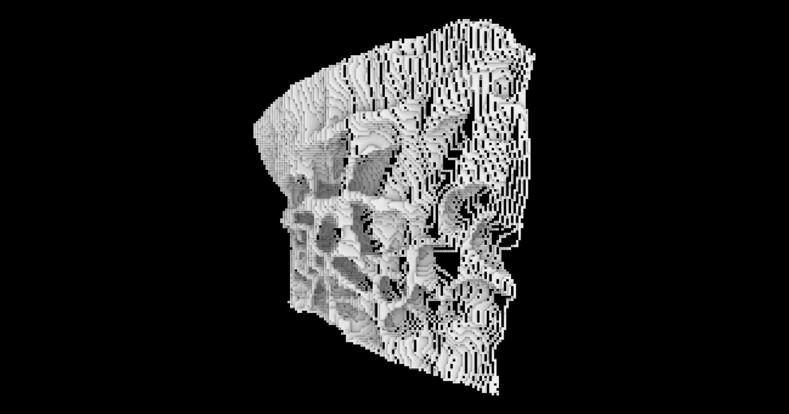
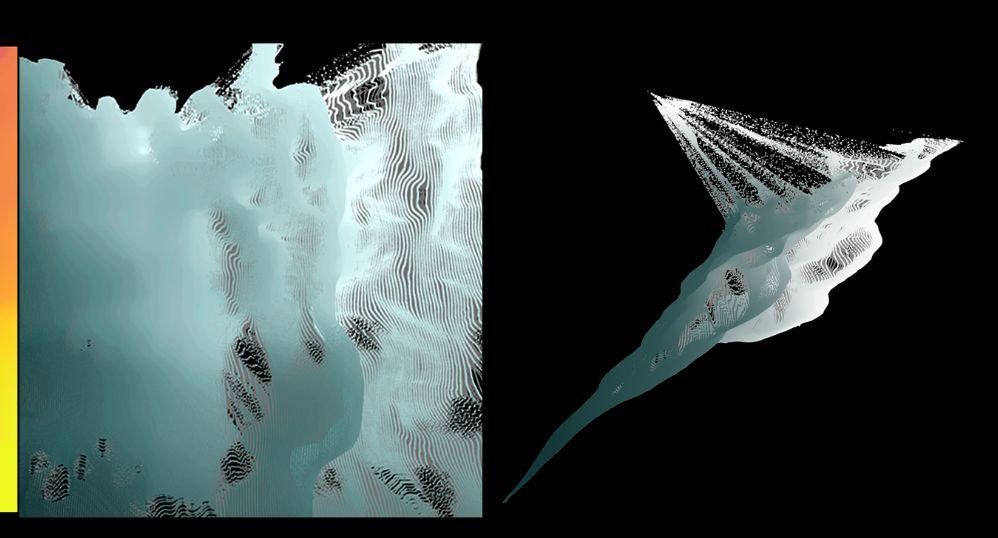
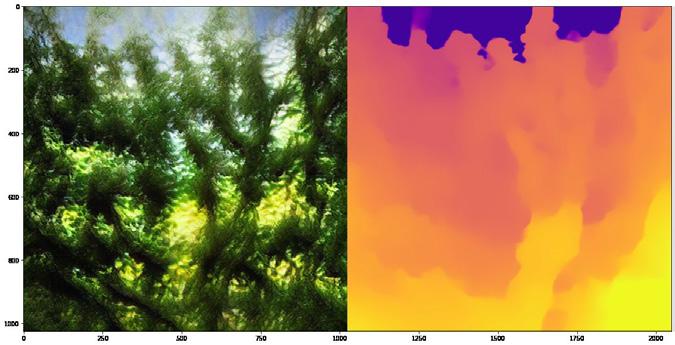
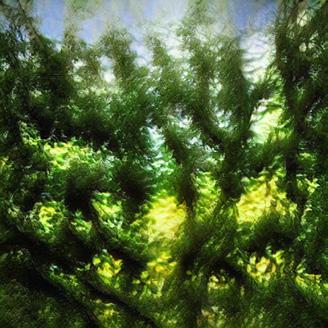
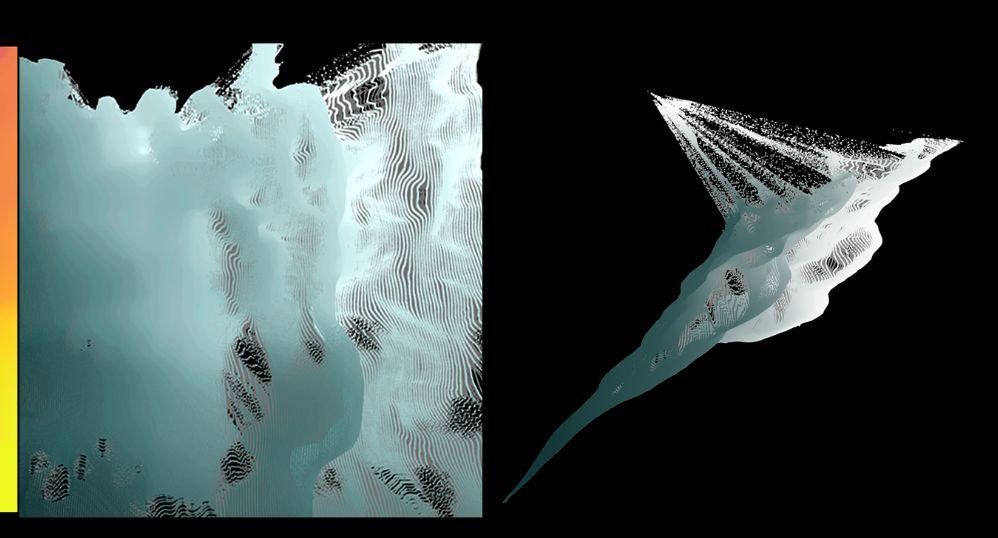
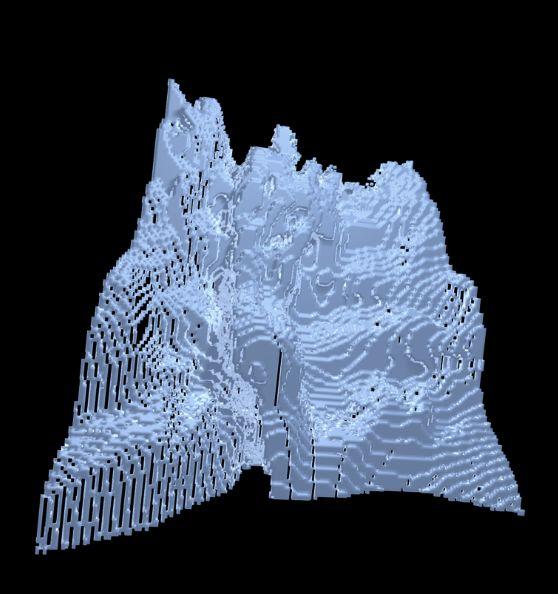
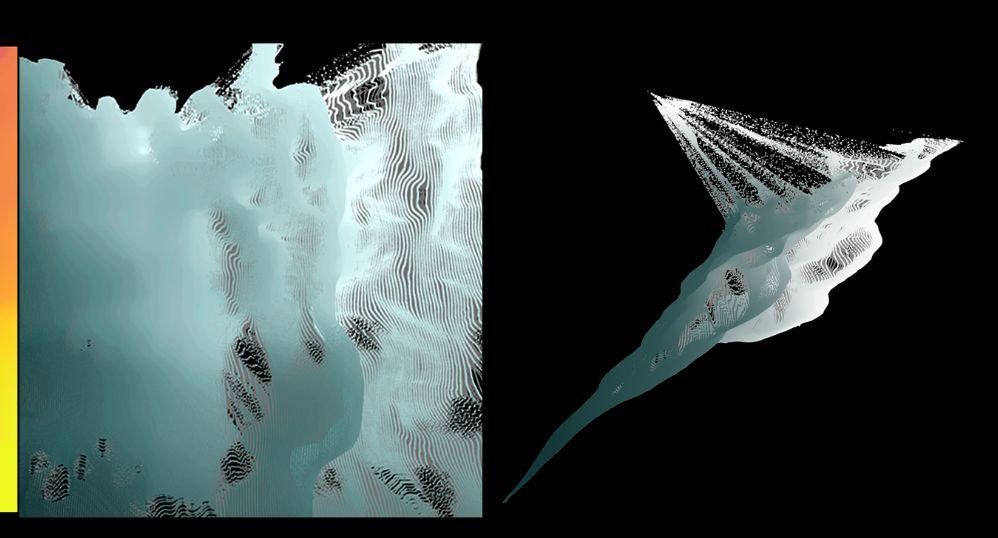
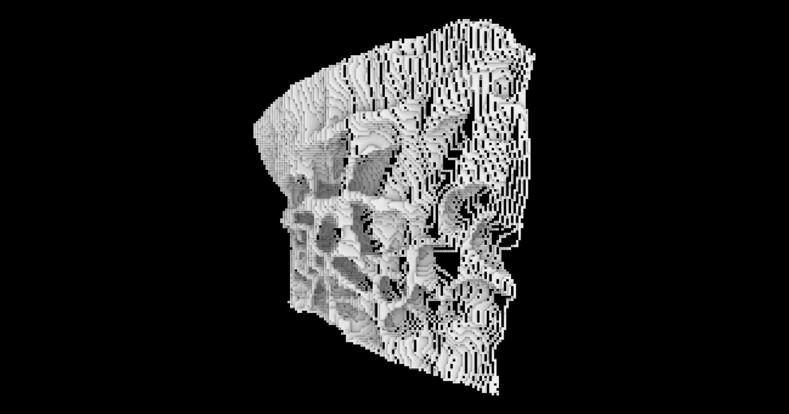
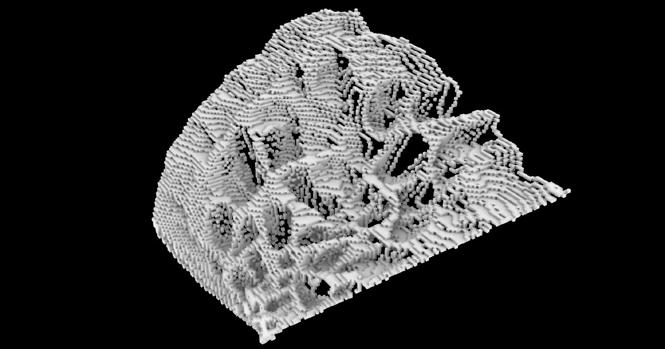
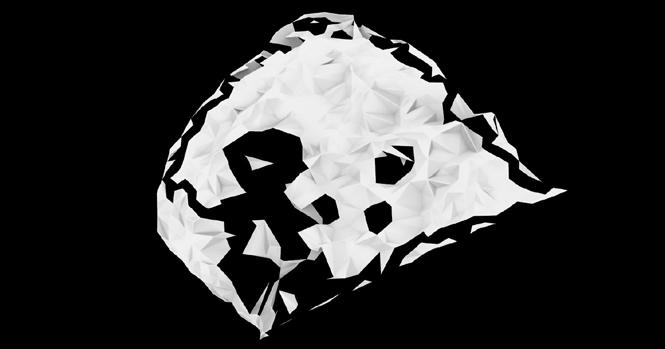
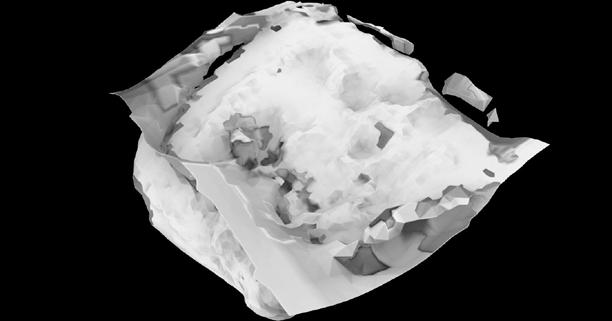
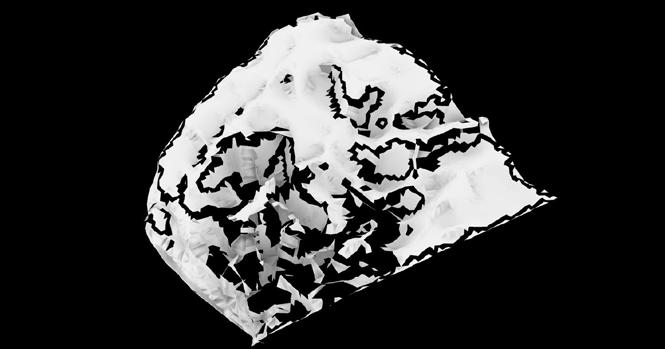
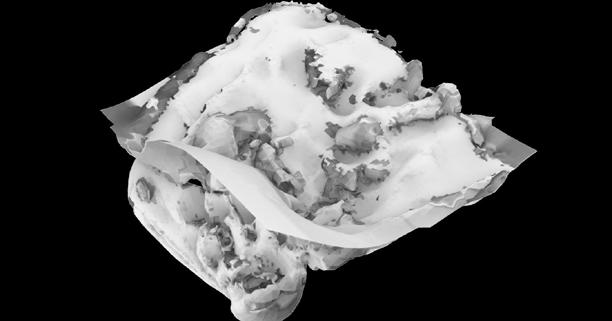
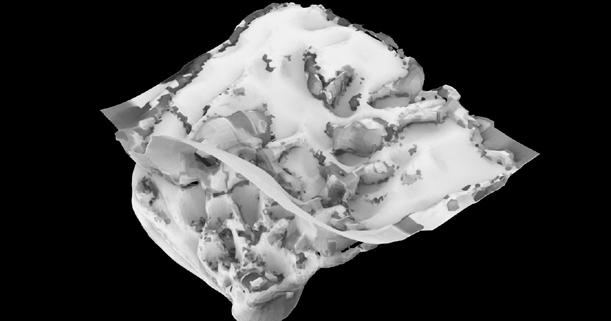
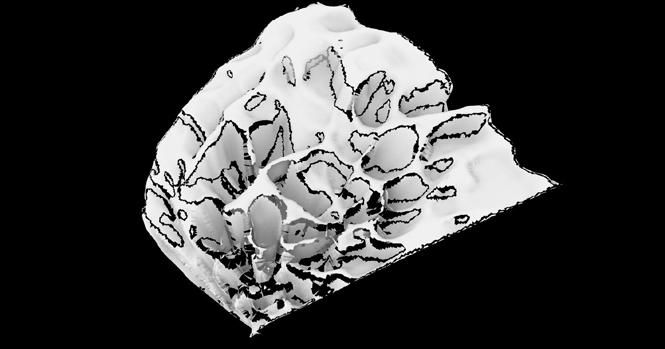
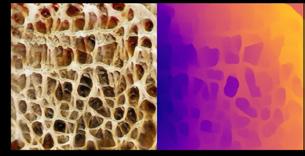
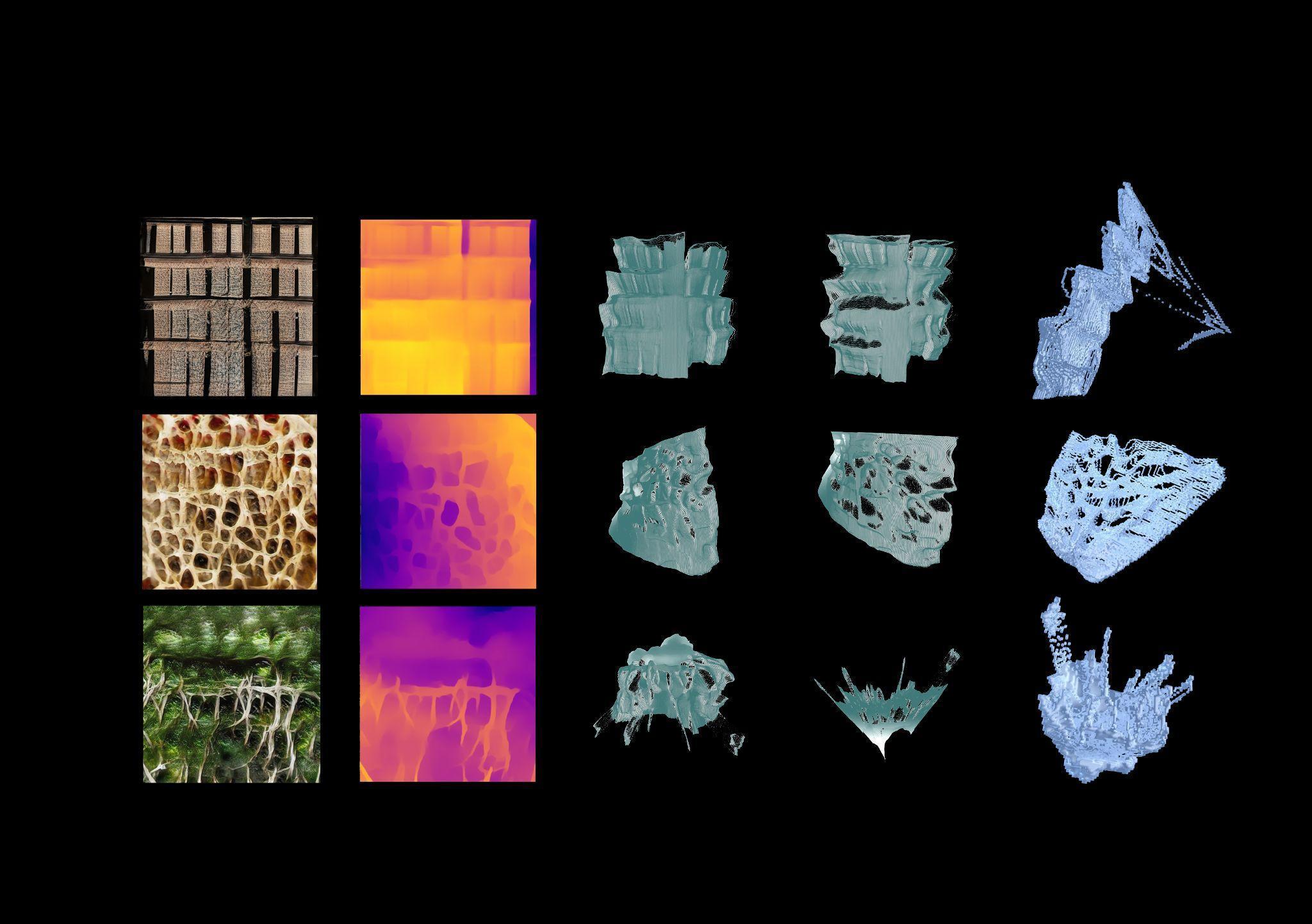
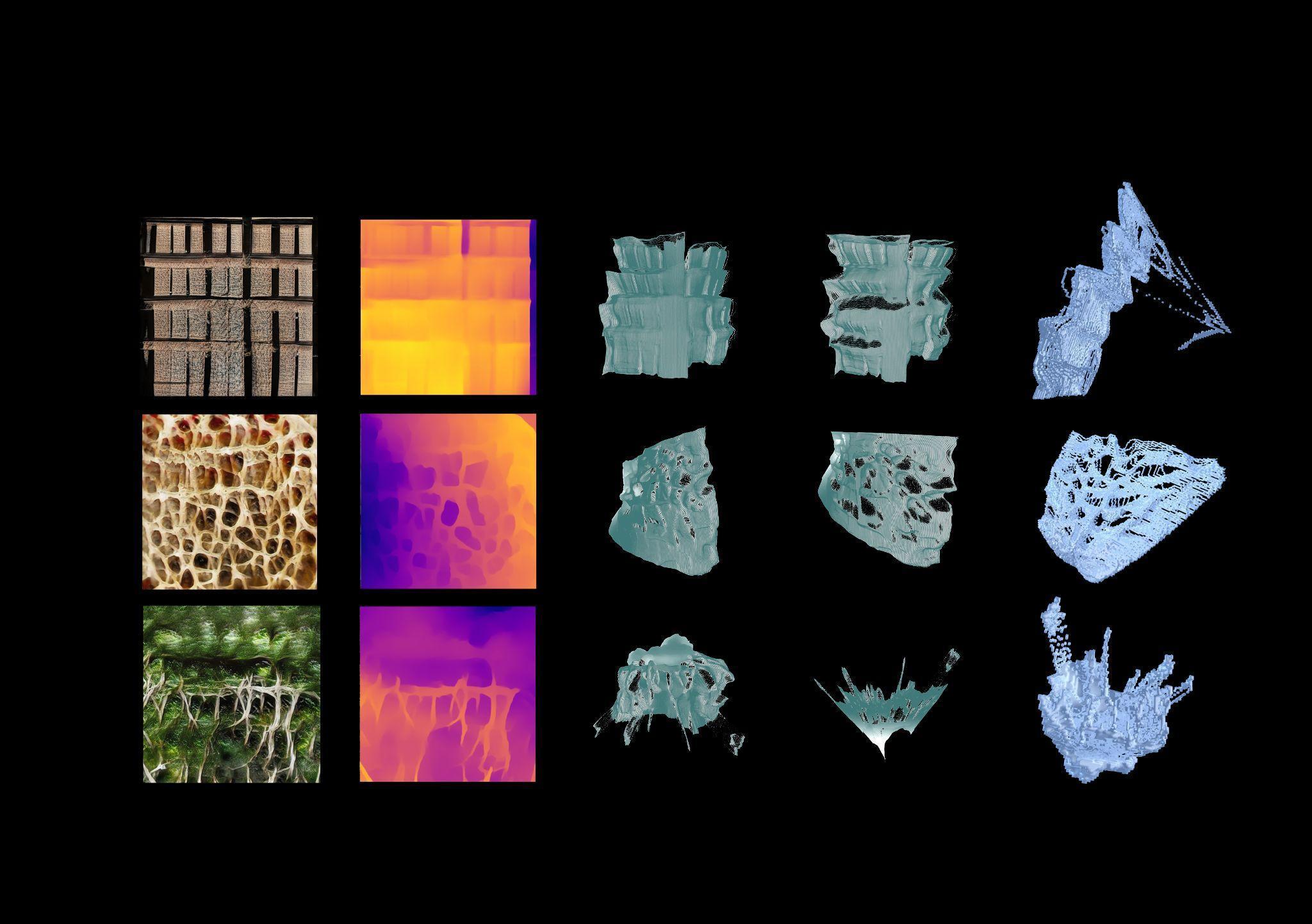
GAME OF GREEN
17
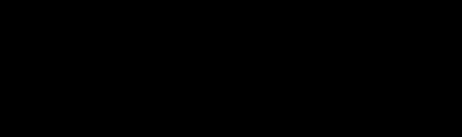
Date: 2022
Location: Vienna, Austria
Collaborators: Charbel Baliss, Jacinto Jesús Moros Montañés, Jumana Hamdani, Lucía Leva
Instructor: Angelos Chronis
Project Type: Academic at IAAC
Tools: Python, Google Colab, Open Street Maps, Carto, Mapbox, QGIS, Open Data Austria, Nasa Power

GAME OF GREEN responds to the lack of Greeneries in the city of Vienna specially the central area. Designed for the decision makers including urban planners, architects, politicians and even inhabitants to participate with their opinions for a better future. Game of Green will propose the optimal locations of planting trees, displaying a score map where adding a tree can make an environmental change
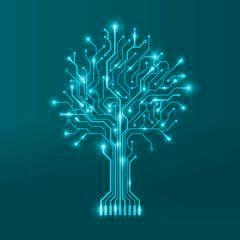
Street of Vienna 824,957 study points VIENNA
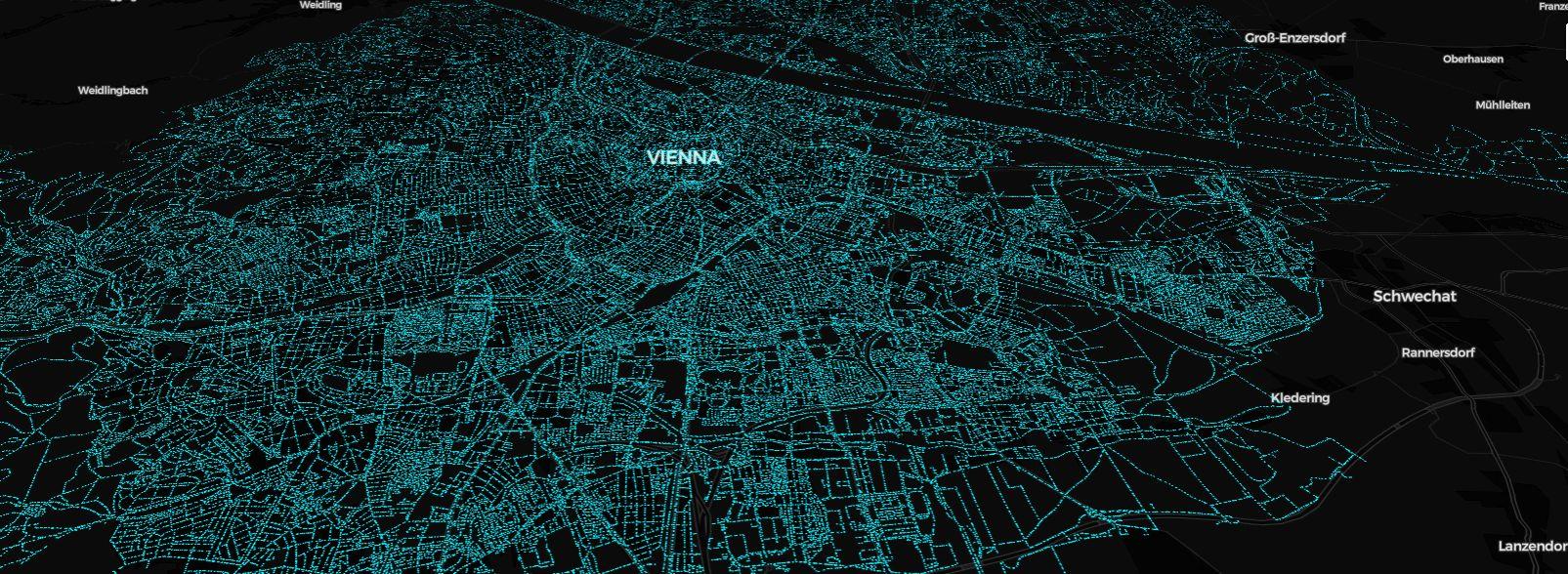





























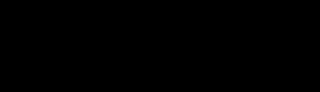
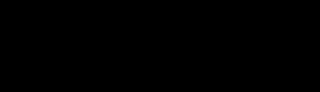


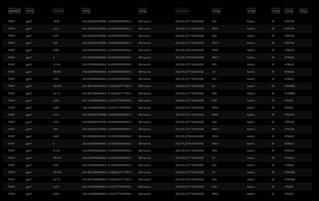
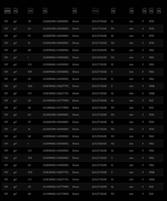
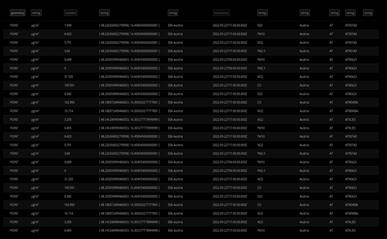
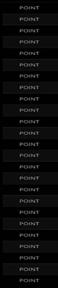


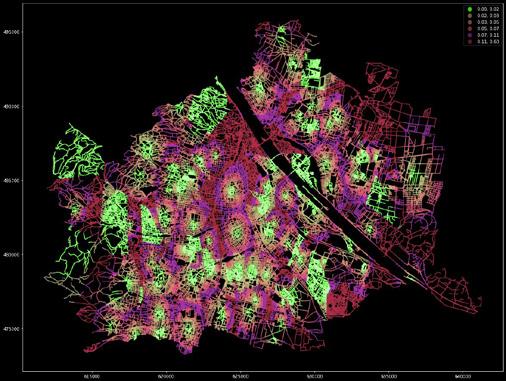

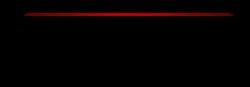
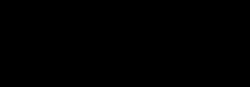
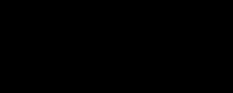
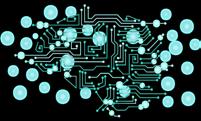
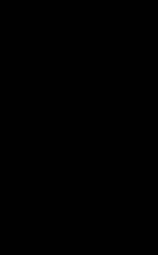

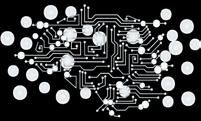



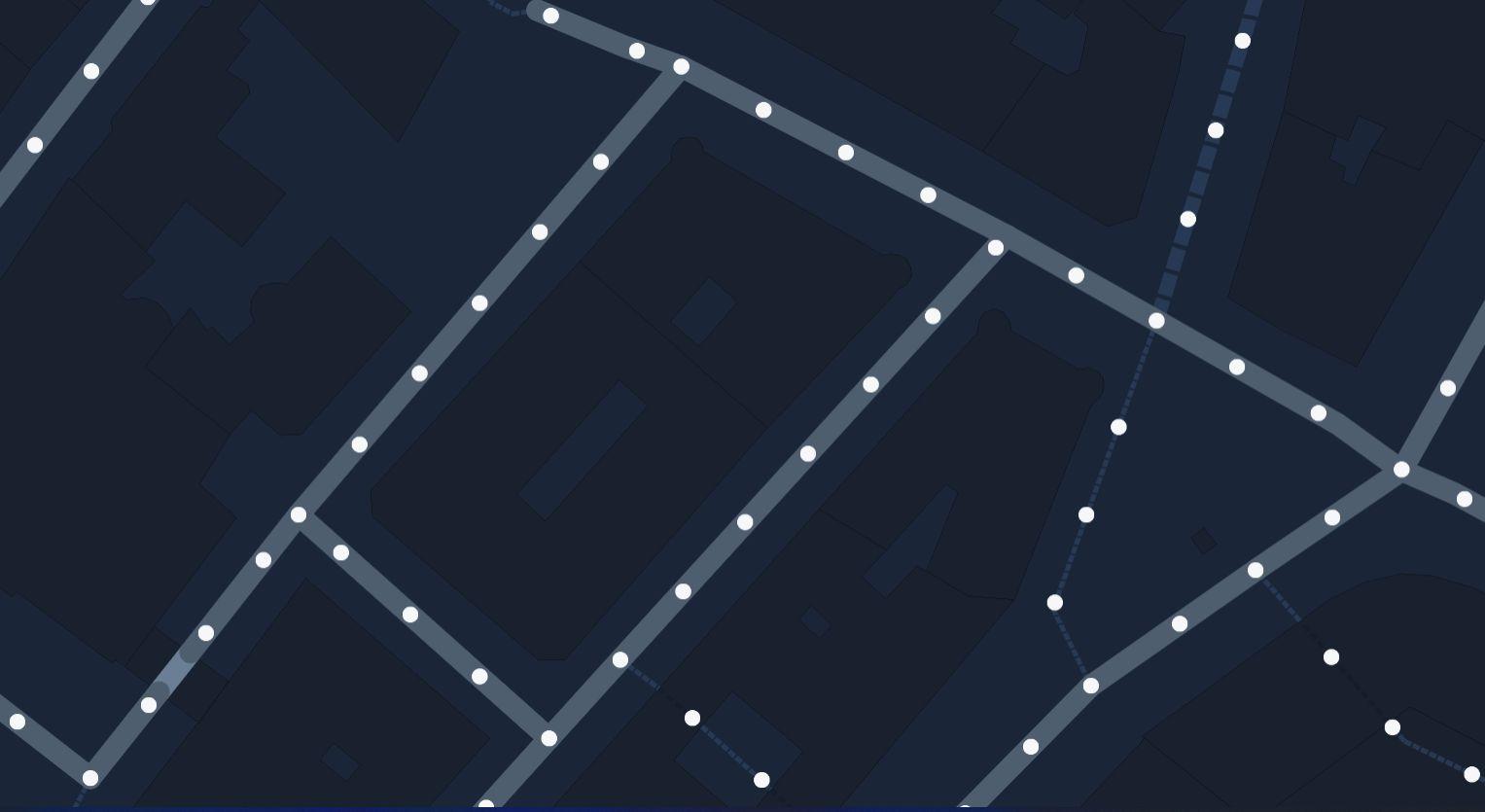
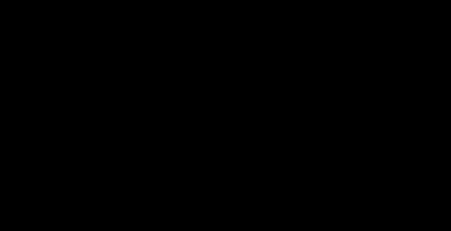
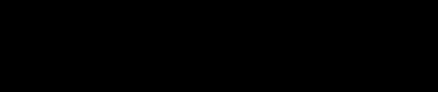
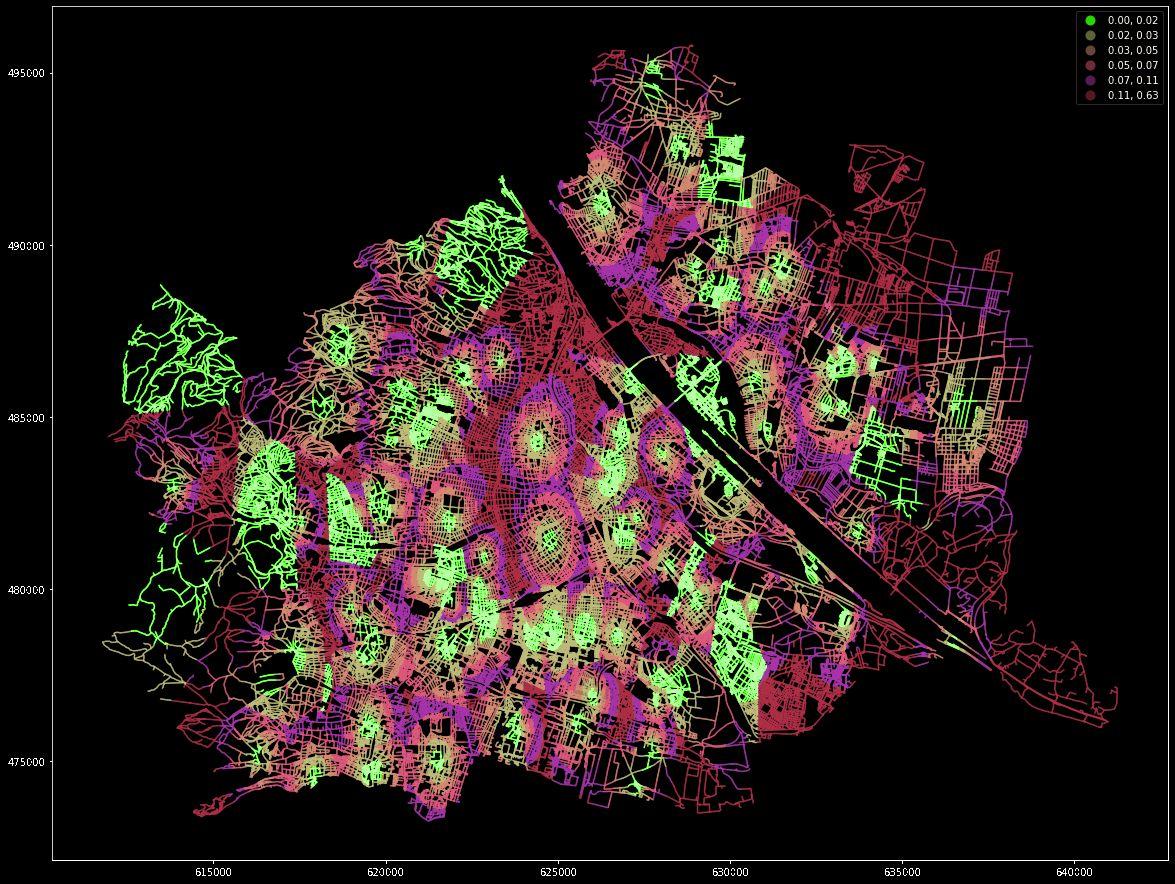




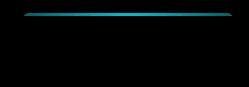
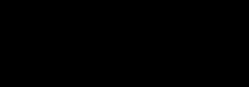
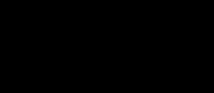
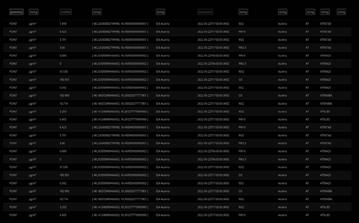
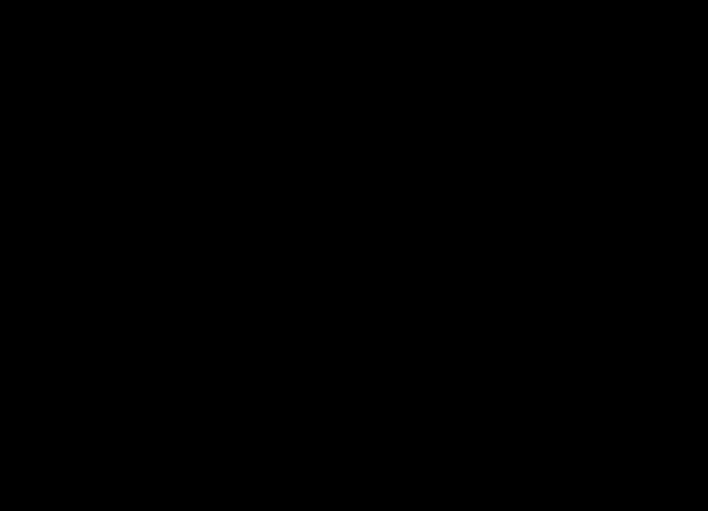
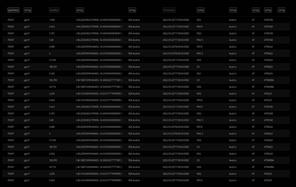
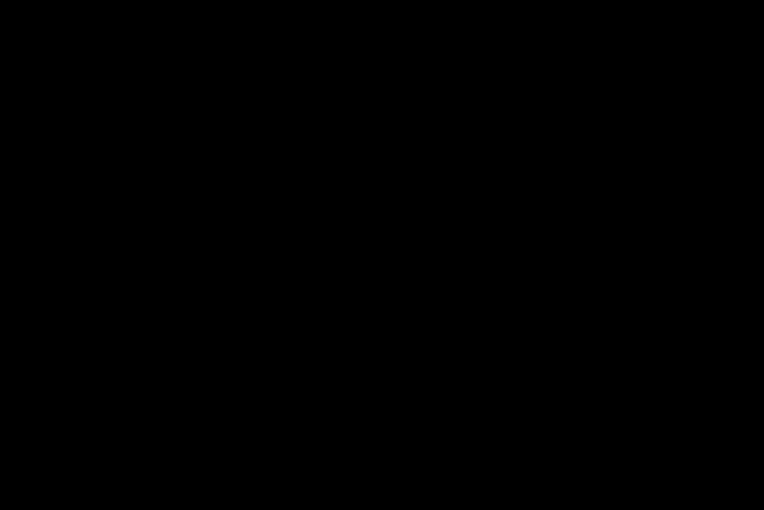



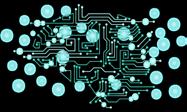
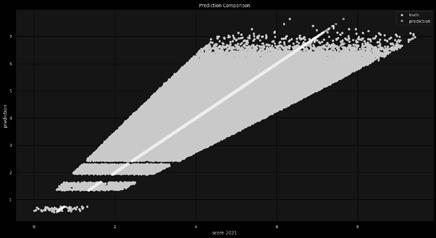
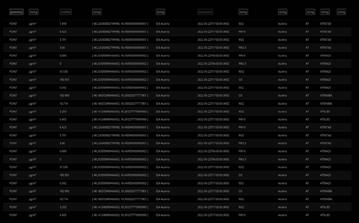
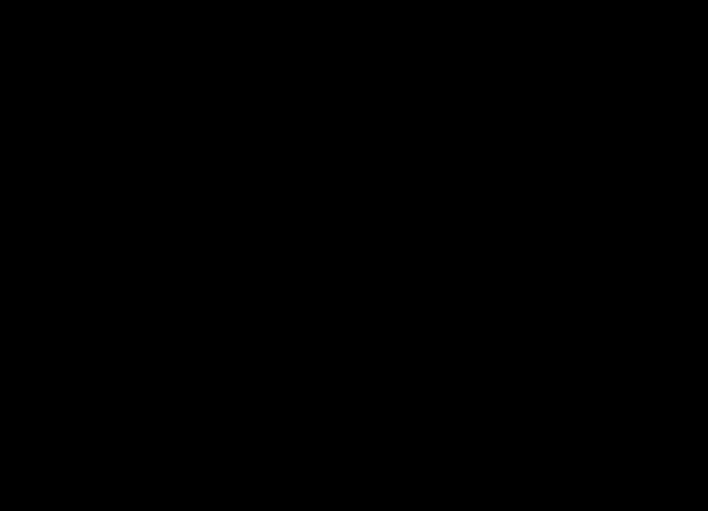

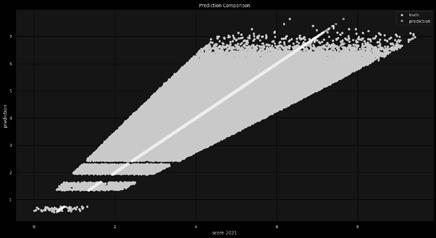
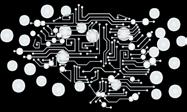








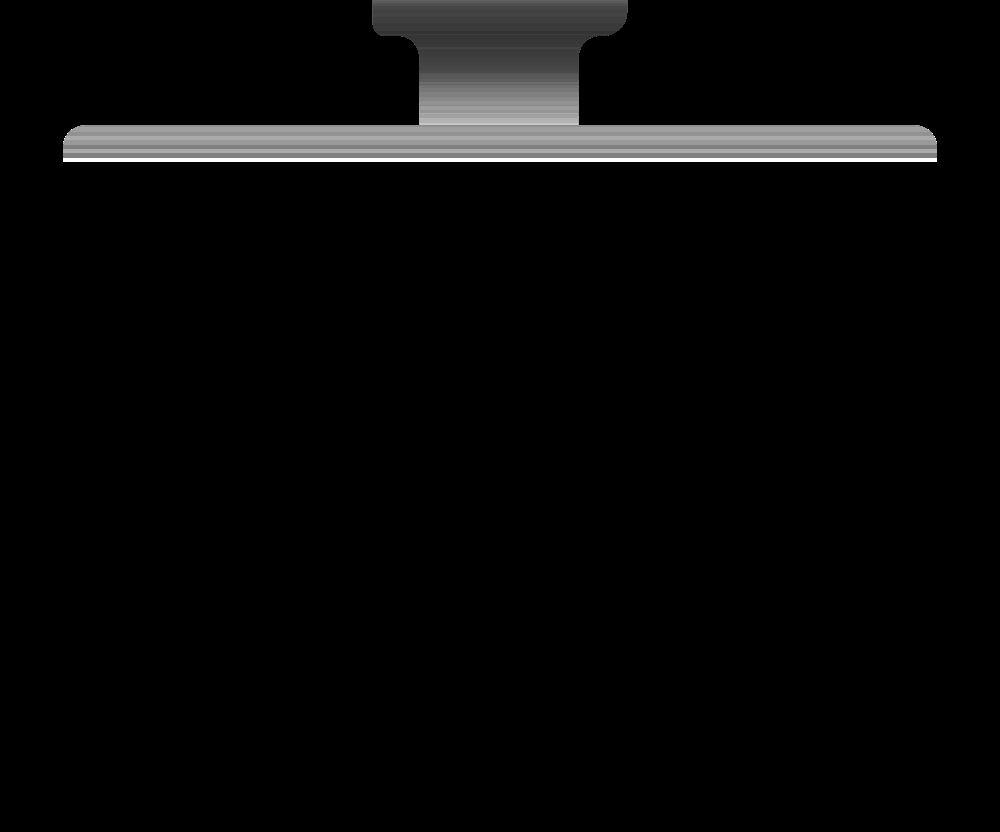
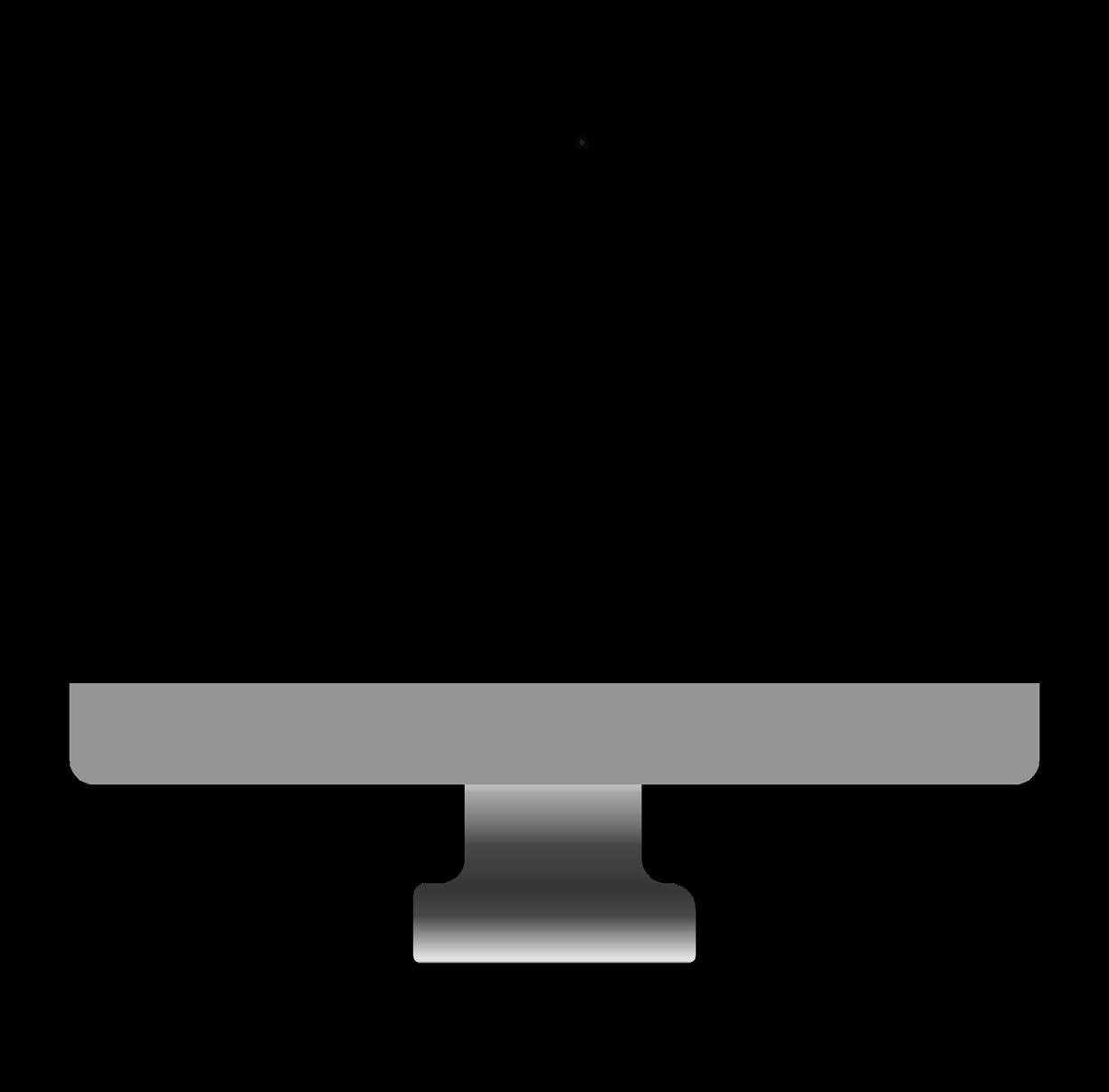




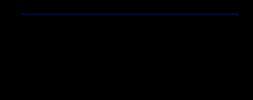
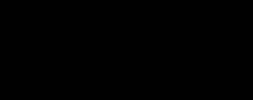
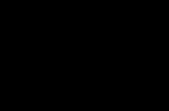
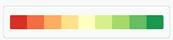

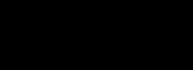
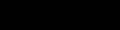
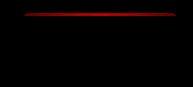
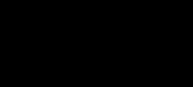
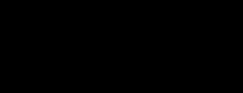
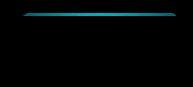
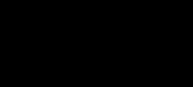
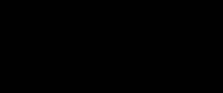
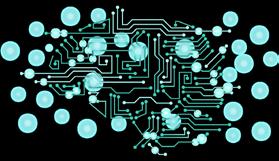

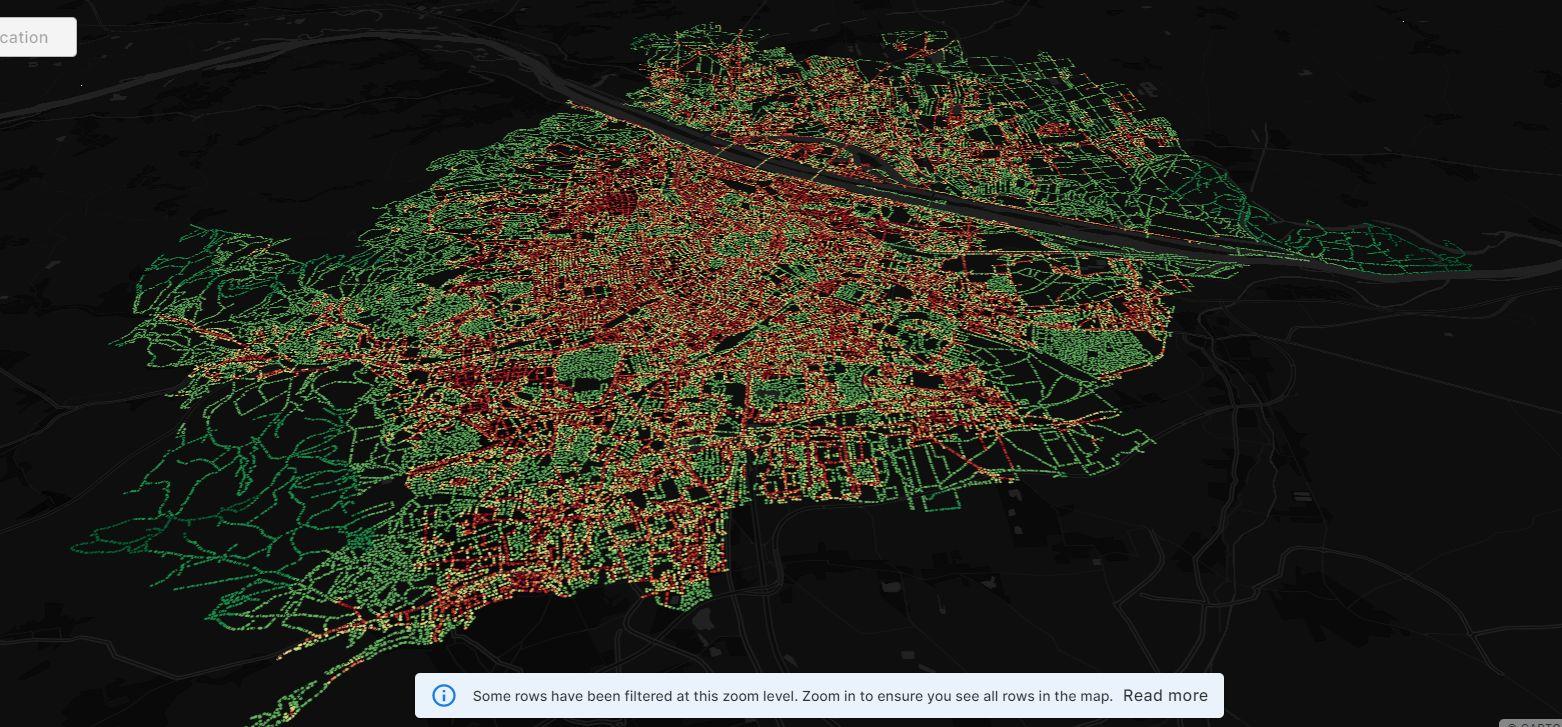
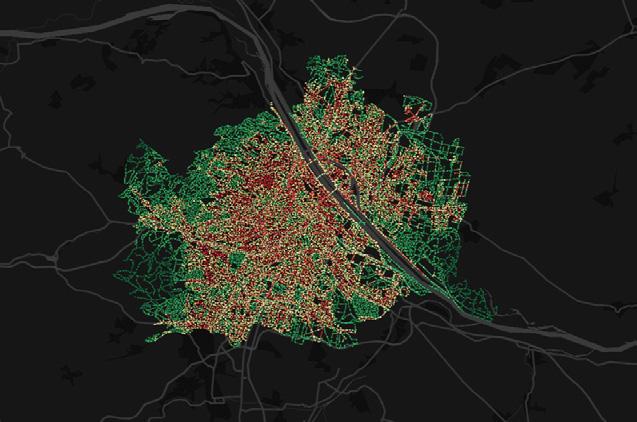
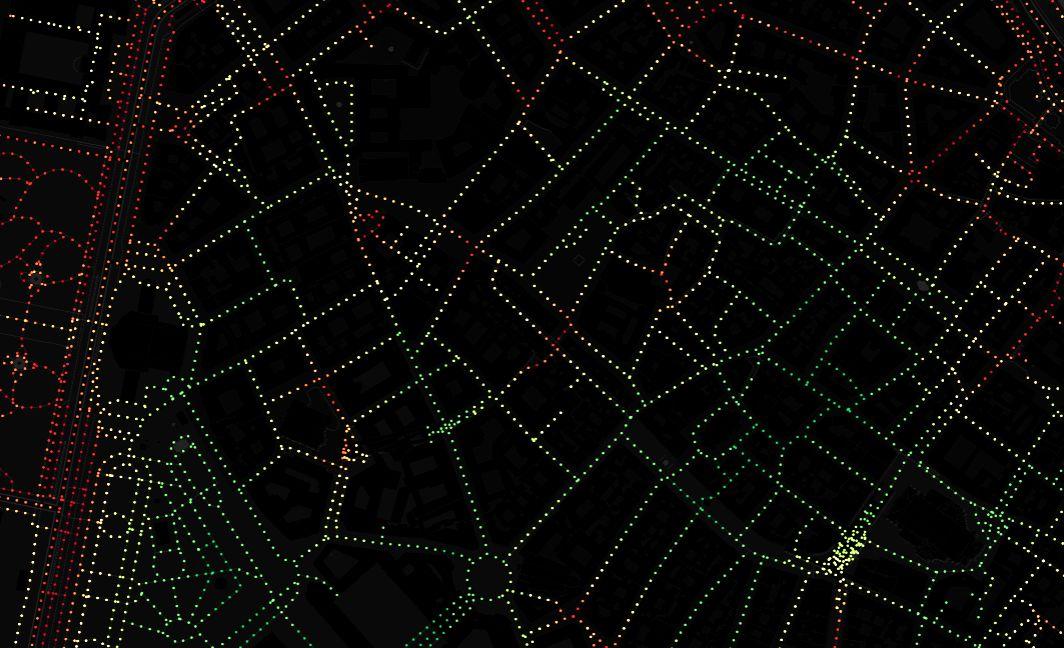
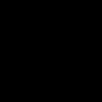
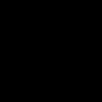
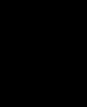
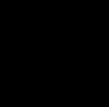
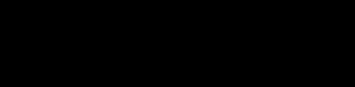

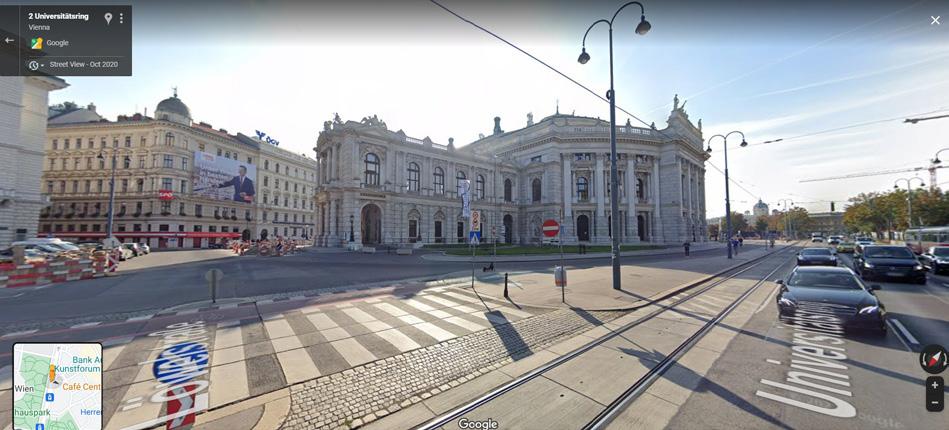
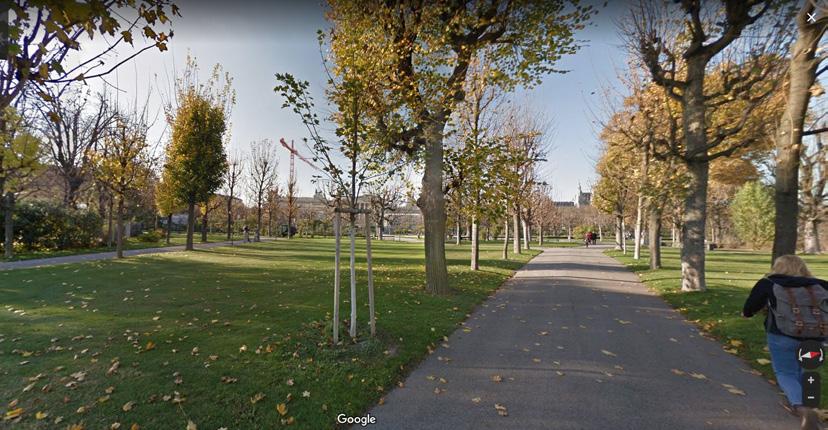

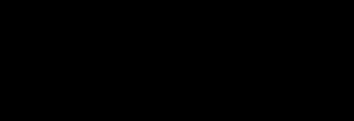
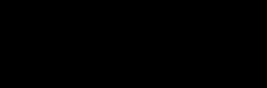
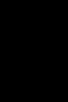
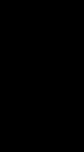

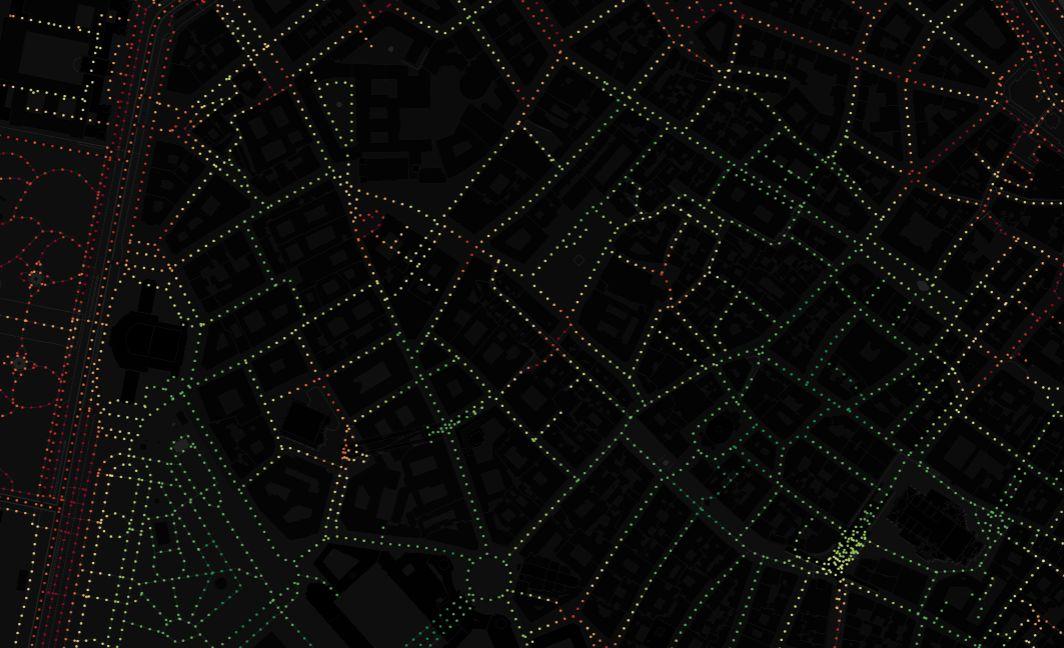
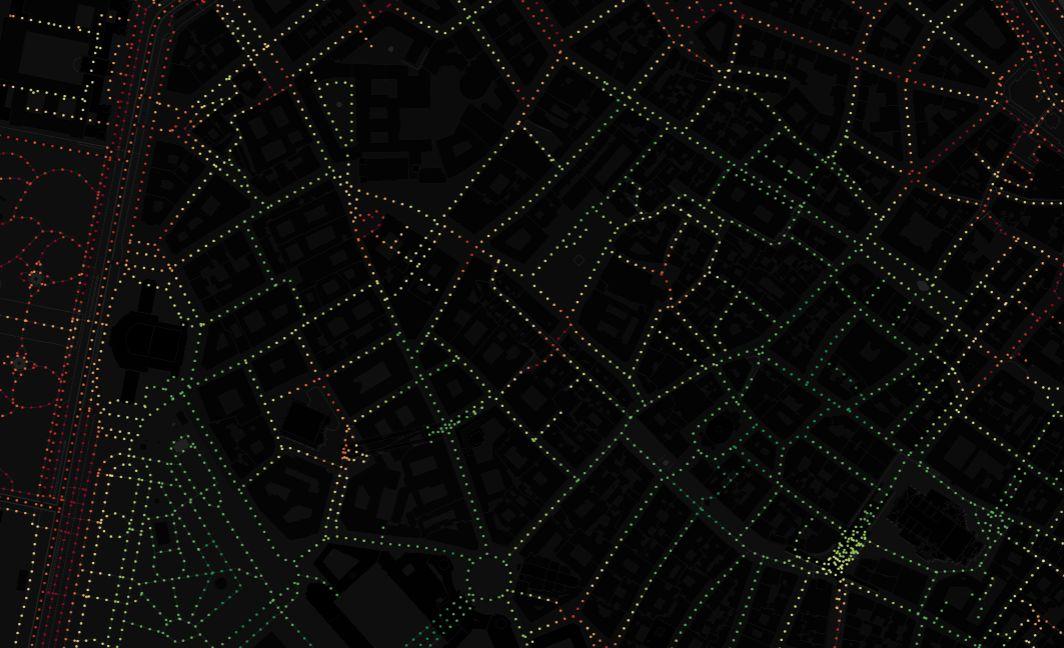


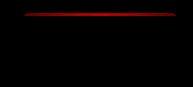
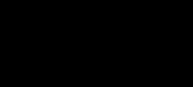
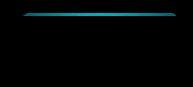
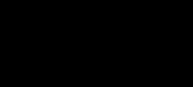



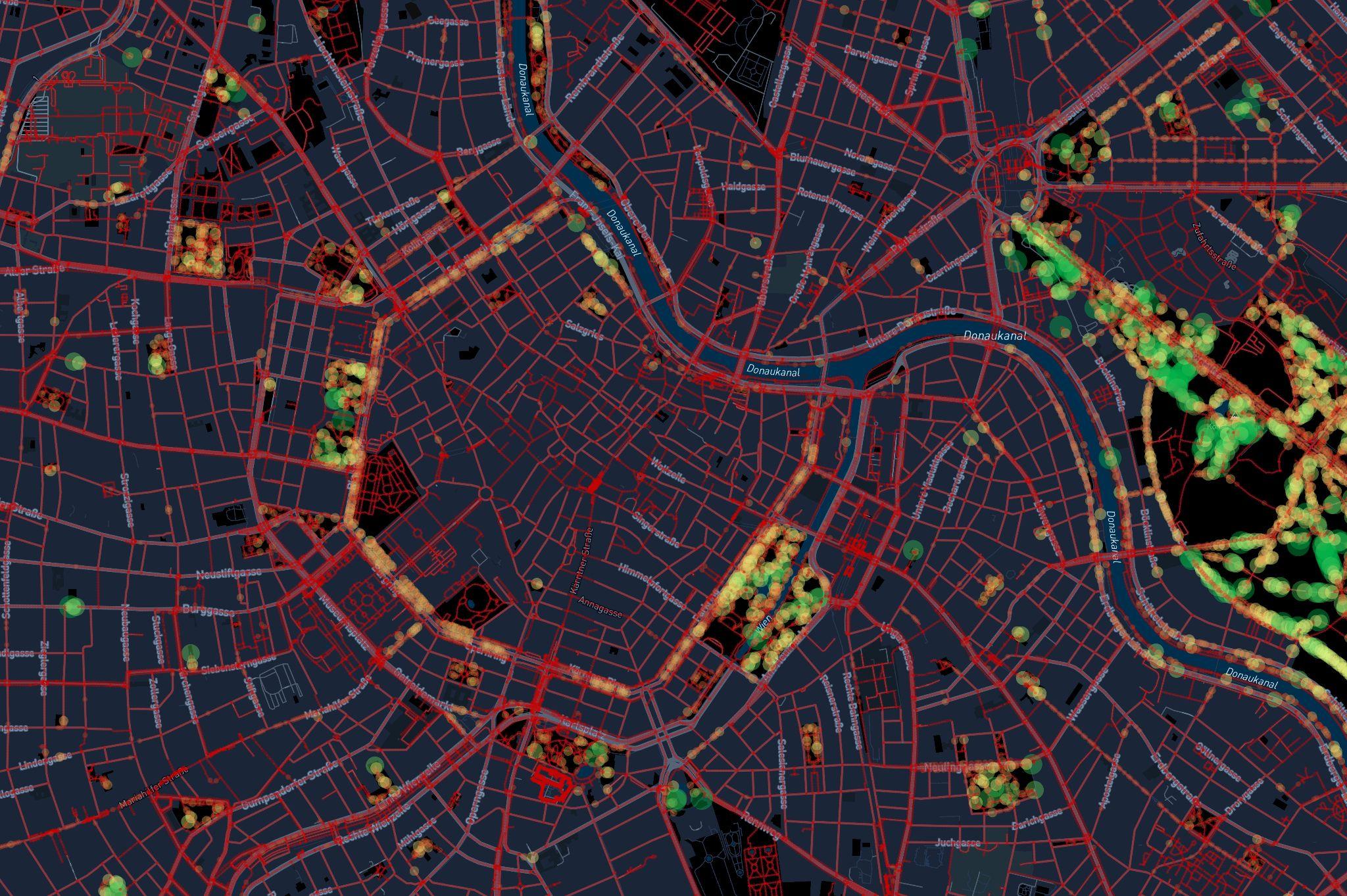
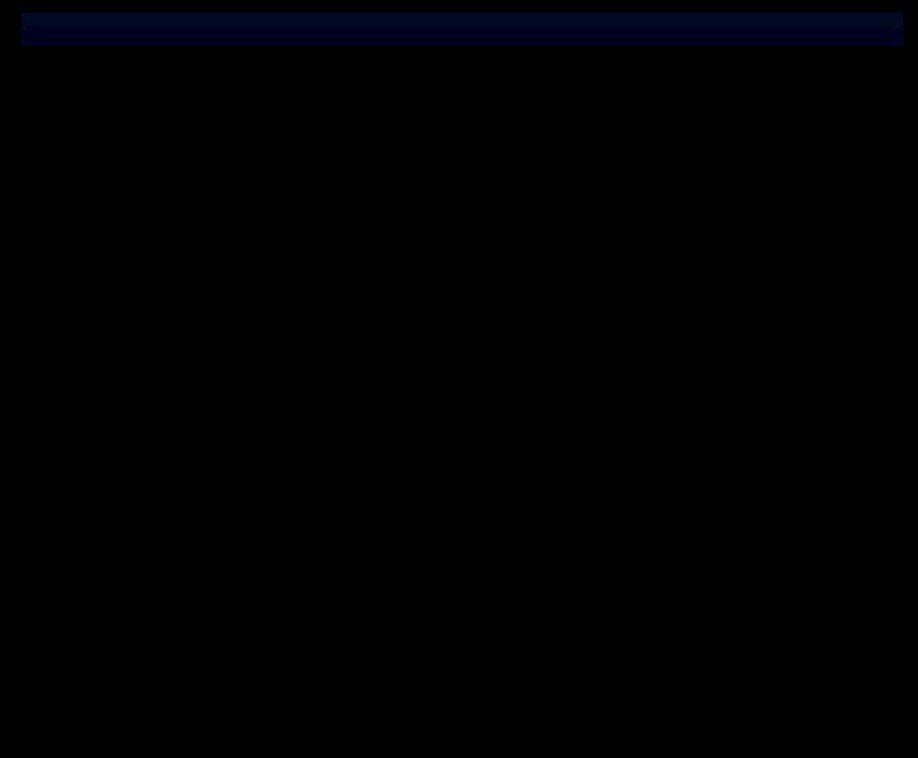
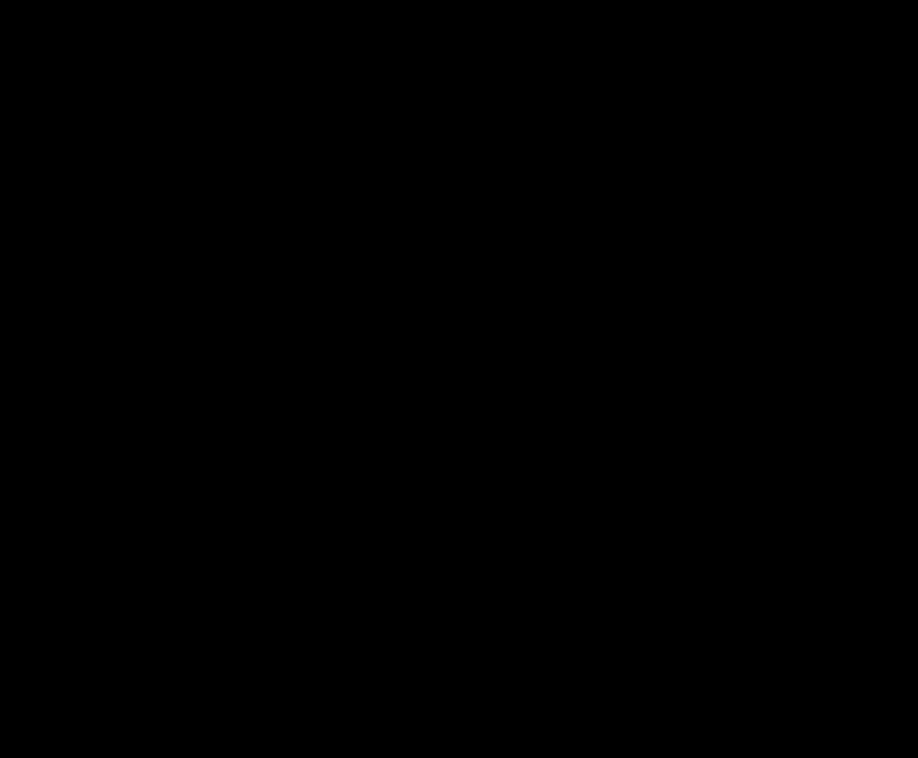
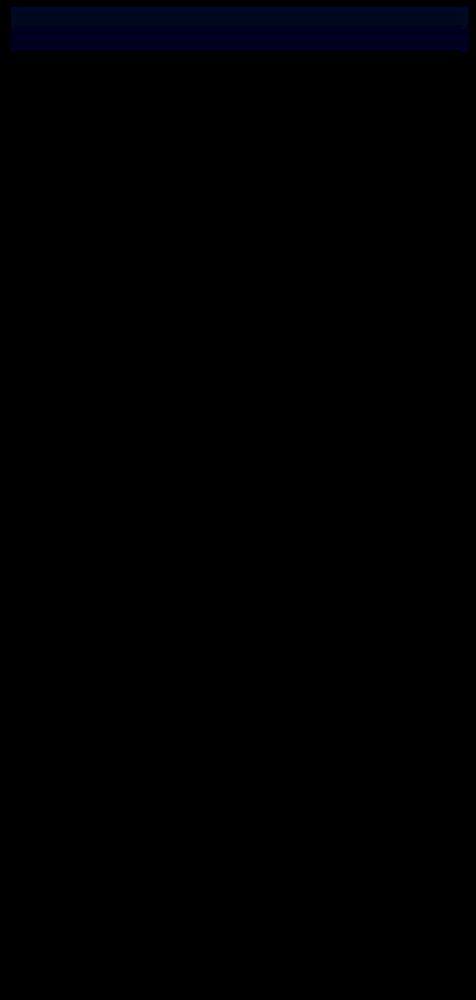
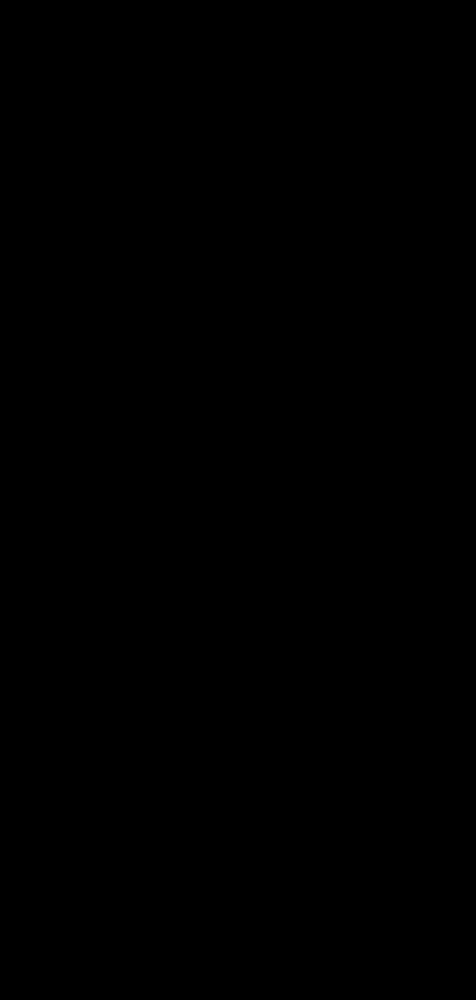
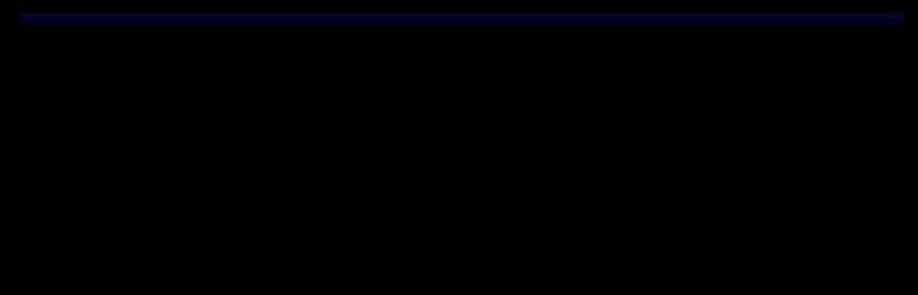
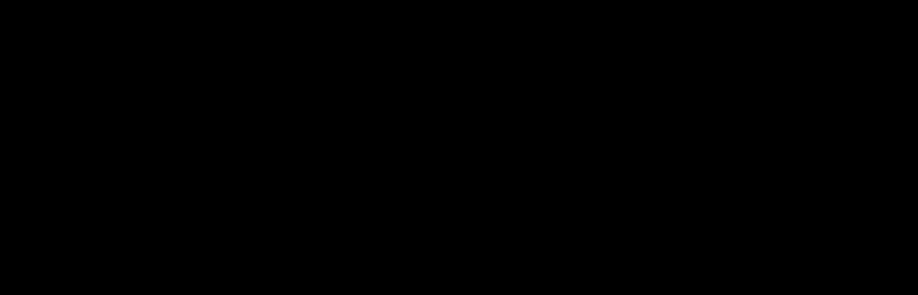
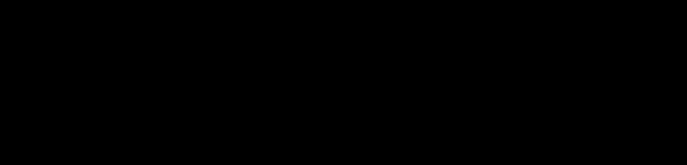

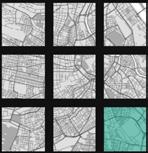




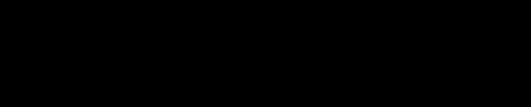


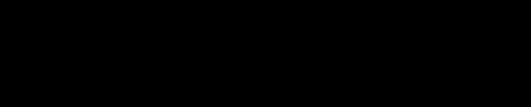
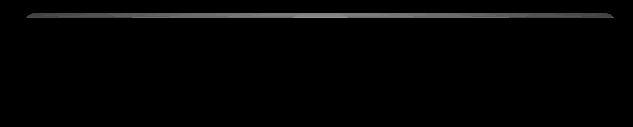
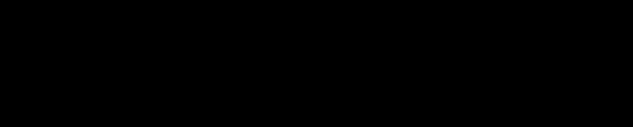
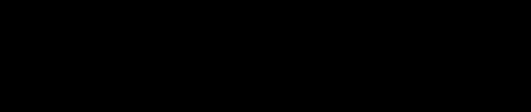

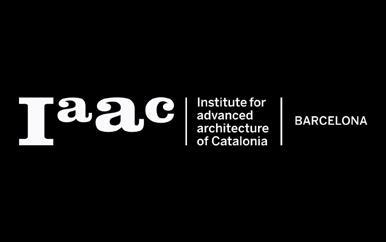
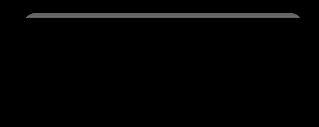
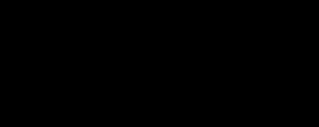
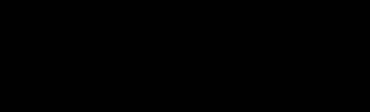
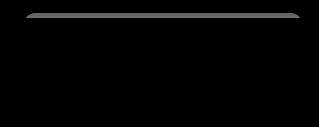
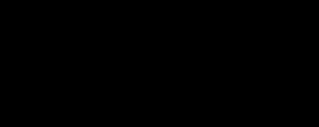
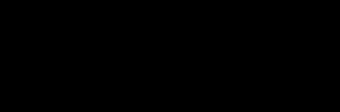
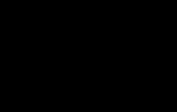
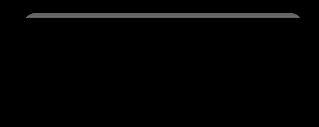
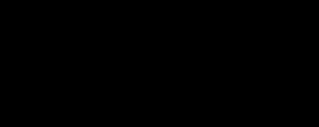
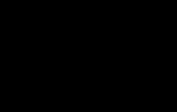
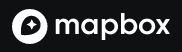
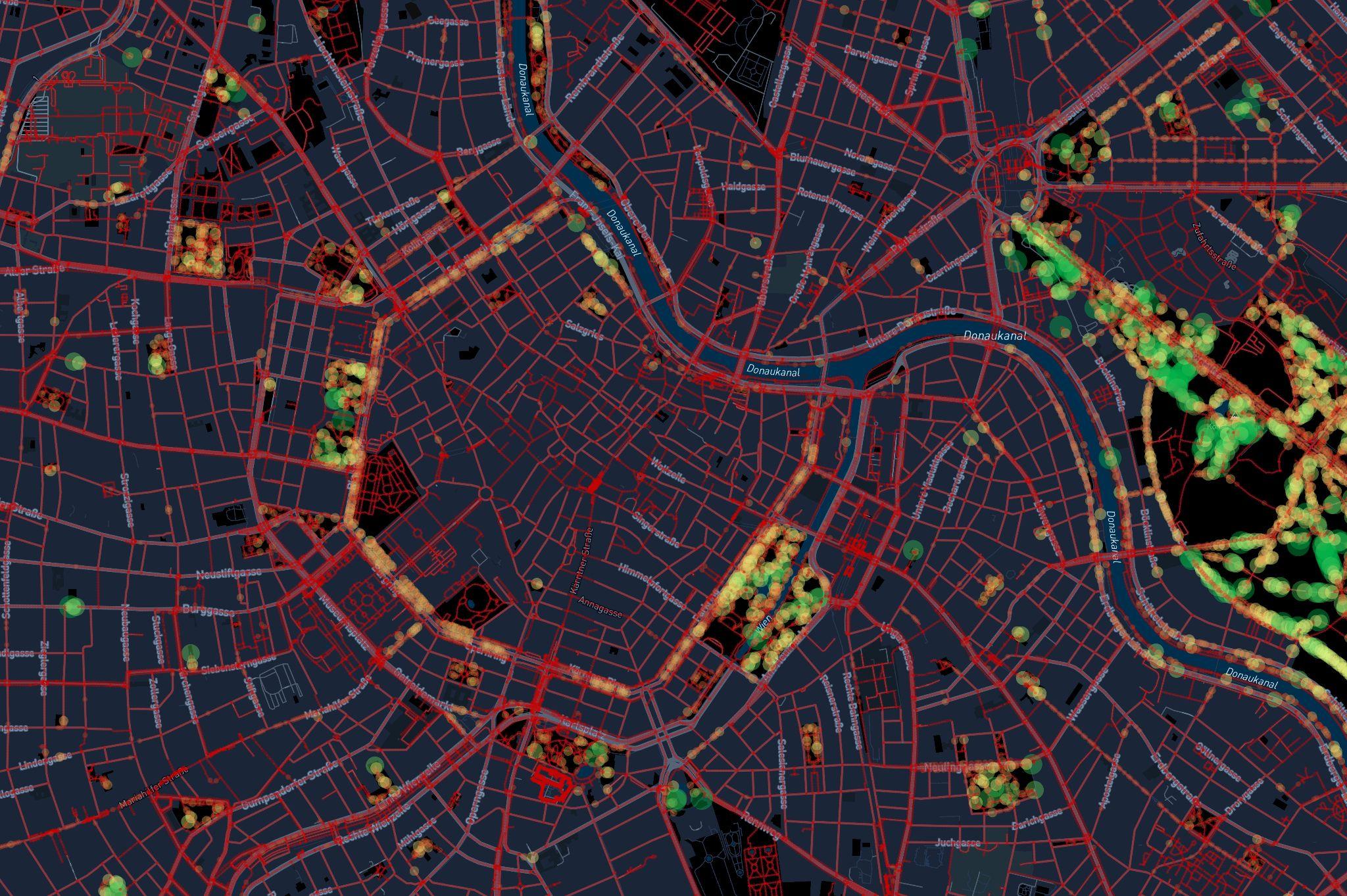
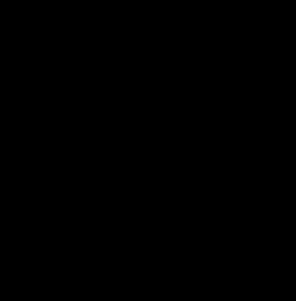
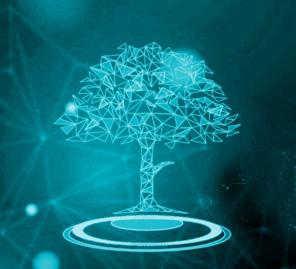
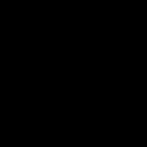
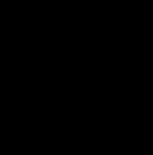
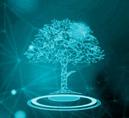
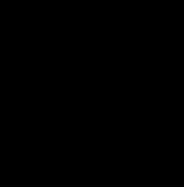
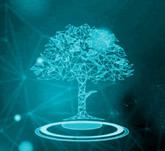
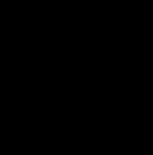
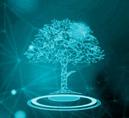
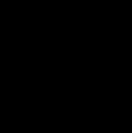
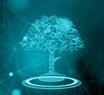
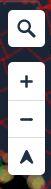
SUBWAY CITY
18
Date: 2022
Location: Stockholm, Munich, Prague
Collaborators: Amanda Gioia, Jumana Hamdani, Sophie Moore
Instructor: David Andrés León
Project Type: Academic at IAAC

Tools: Rhino,Grasshopper, Hops, Python, Graph Theory, Flask, Google Colab
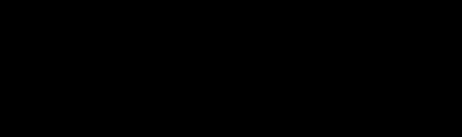
Subway Cities is an urban analysis of the subway network.
In this project we investigated the subway networks of 3 case study cities: Munich, Stockholm and Prague. We often hear citizens complain about how the subway network in their cities could be better. We wanted to challenge that theory by asking our classmates and ourselves to redraw the subway connections to propose better connectivity.
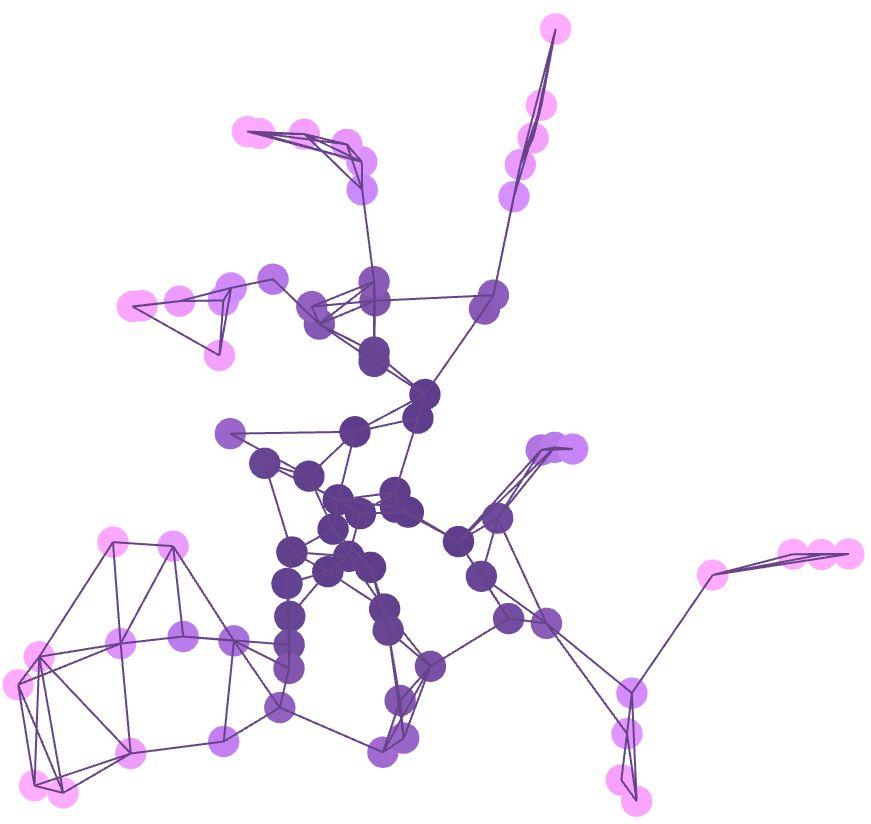
SUBWAY CITY
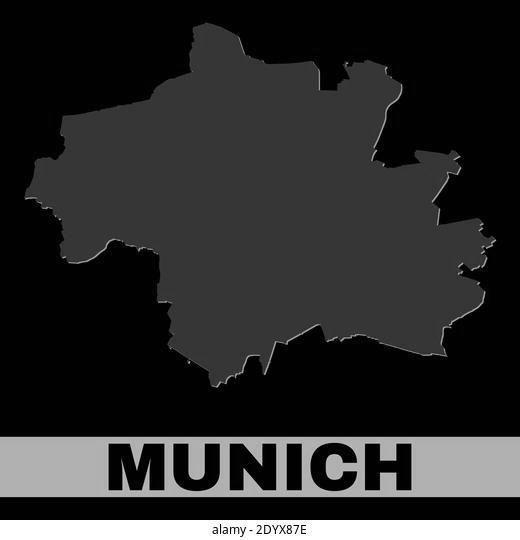
SUBWAY MAPS
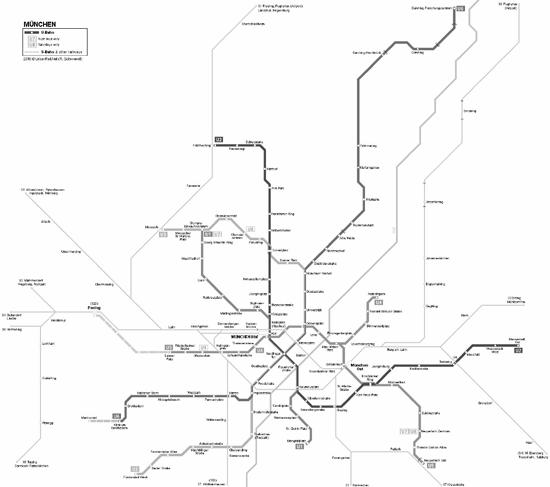
SUBWAY NETWORK

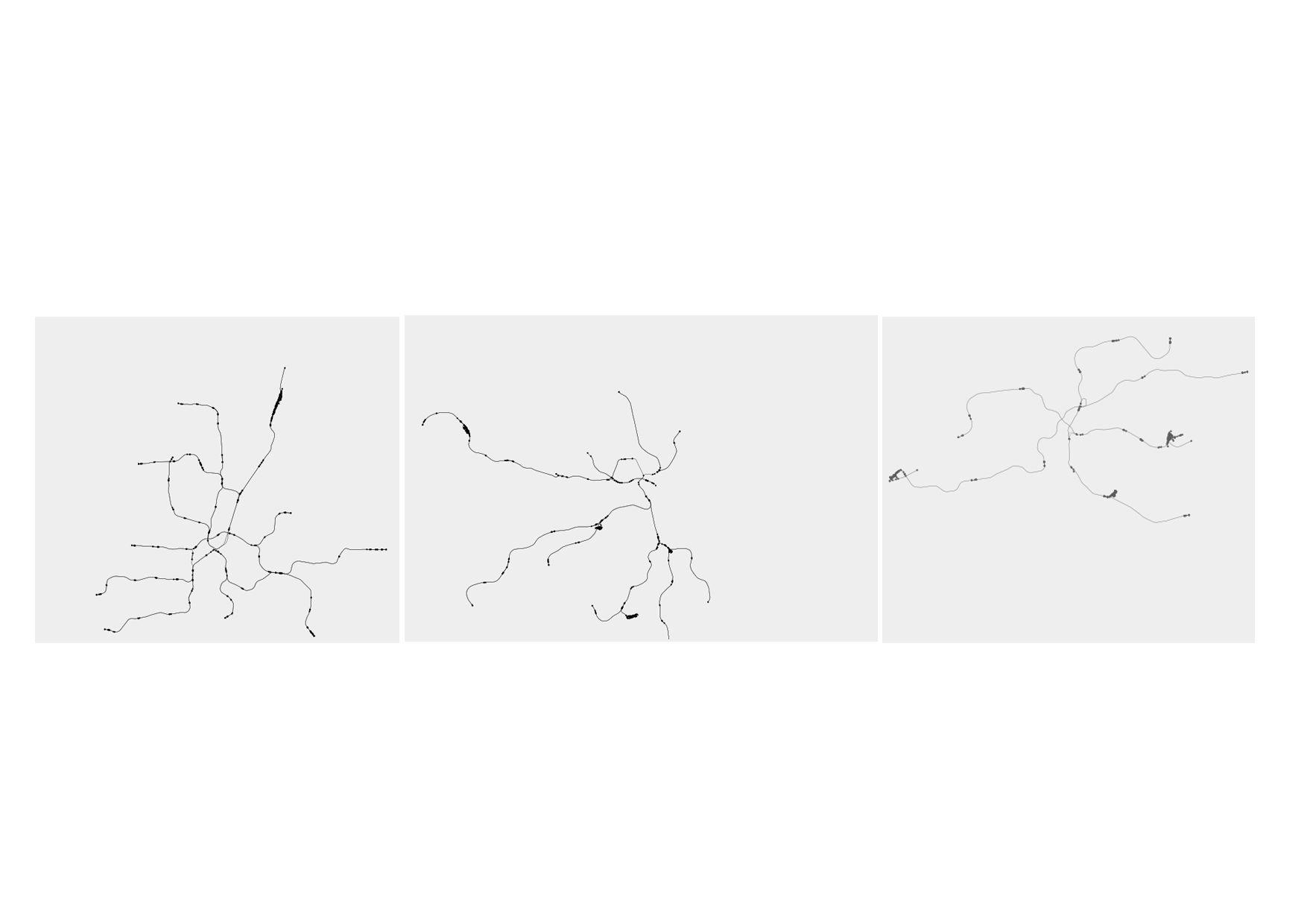
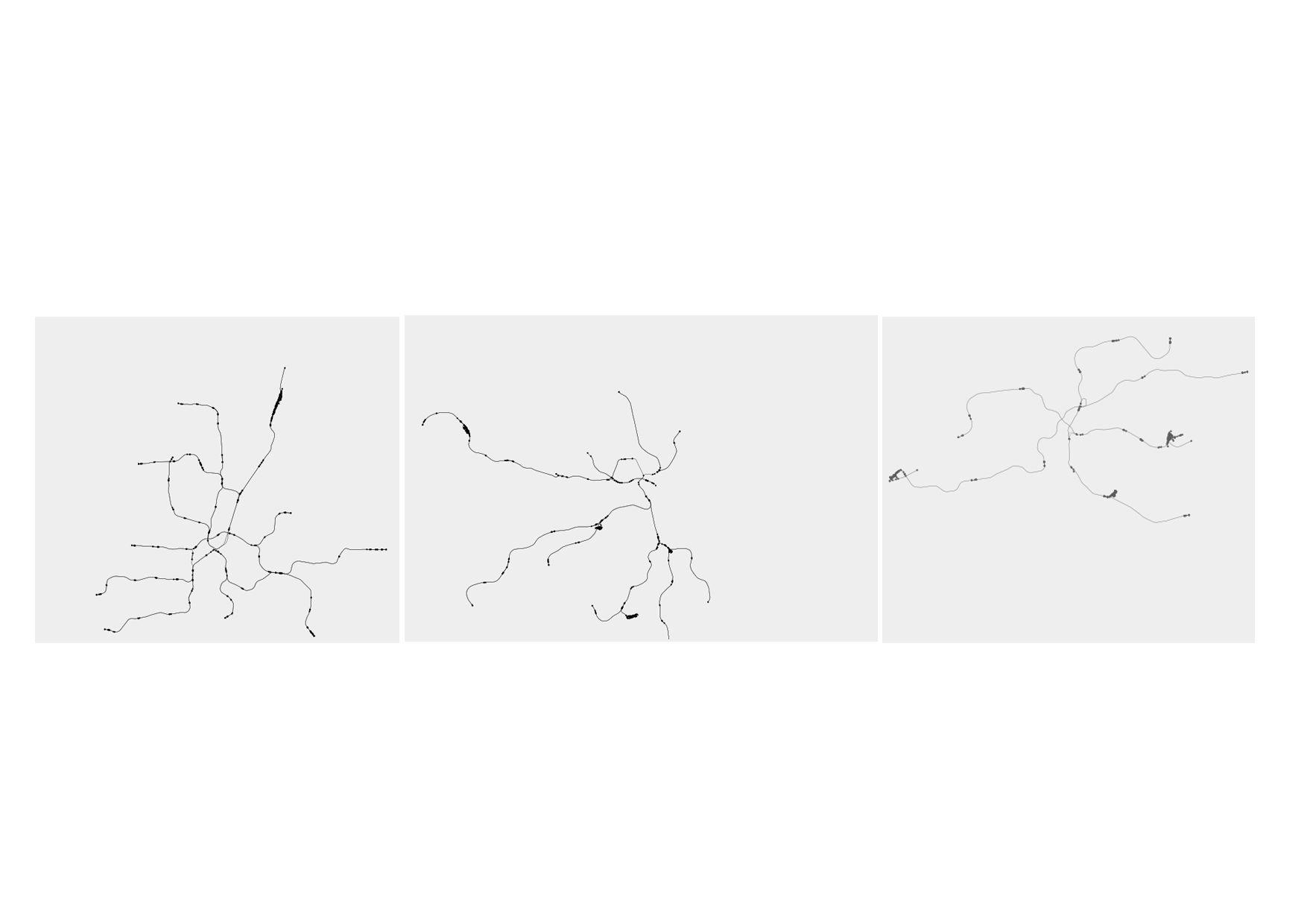
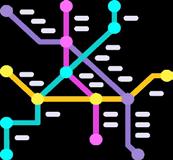
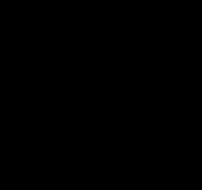
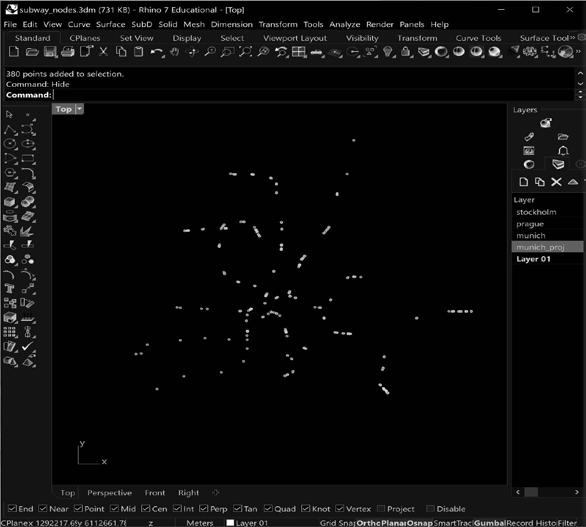
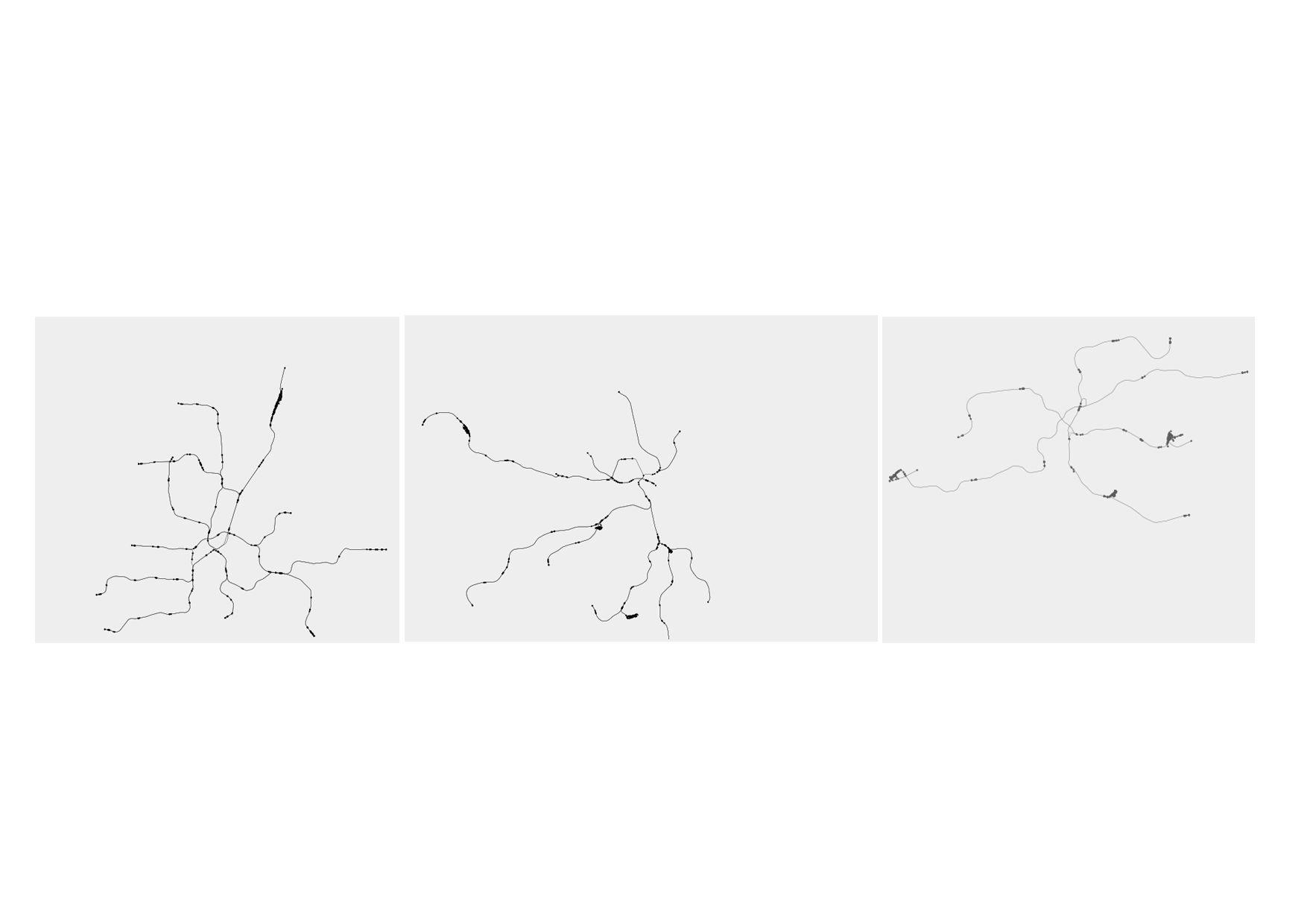
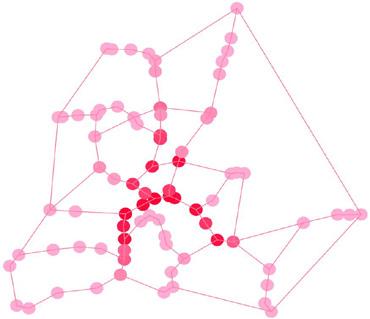
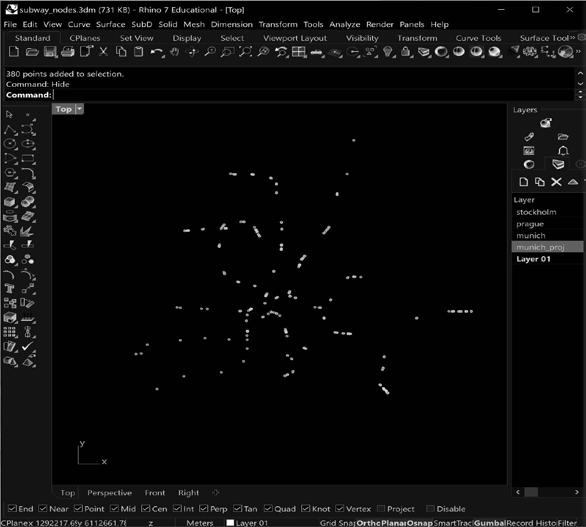
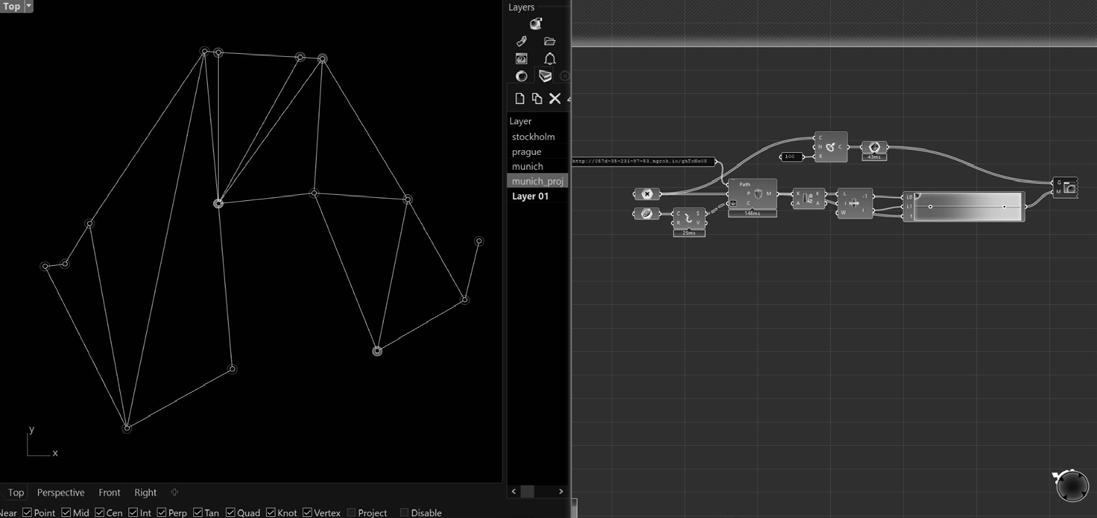
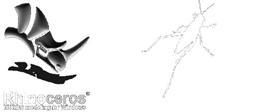
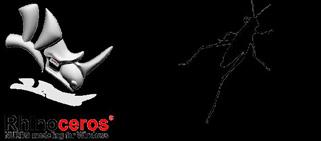

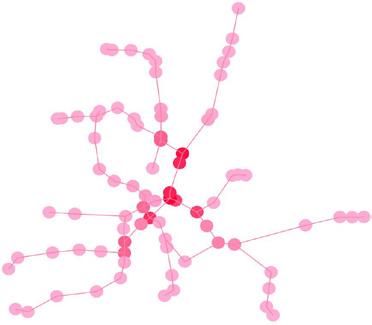
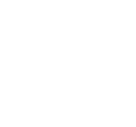
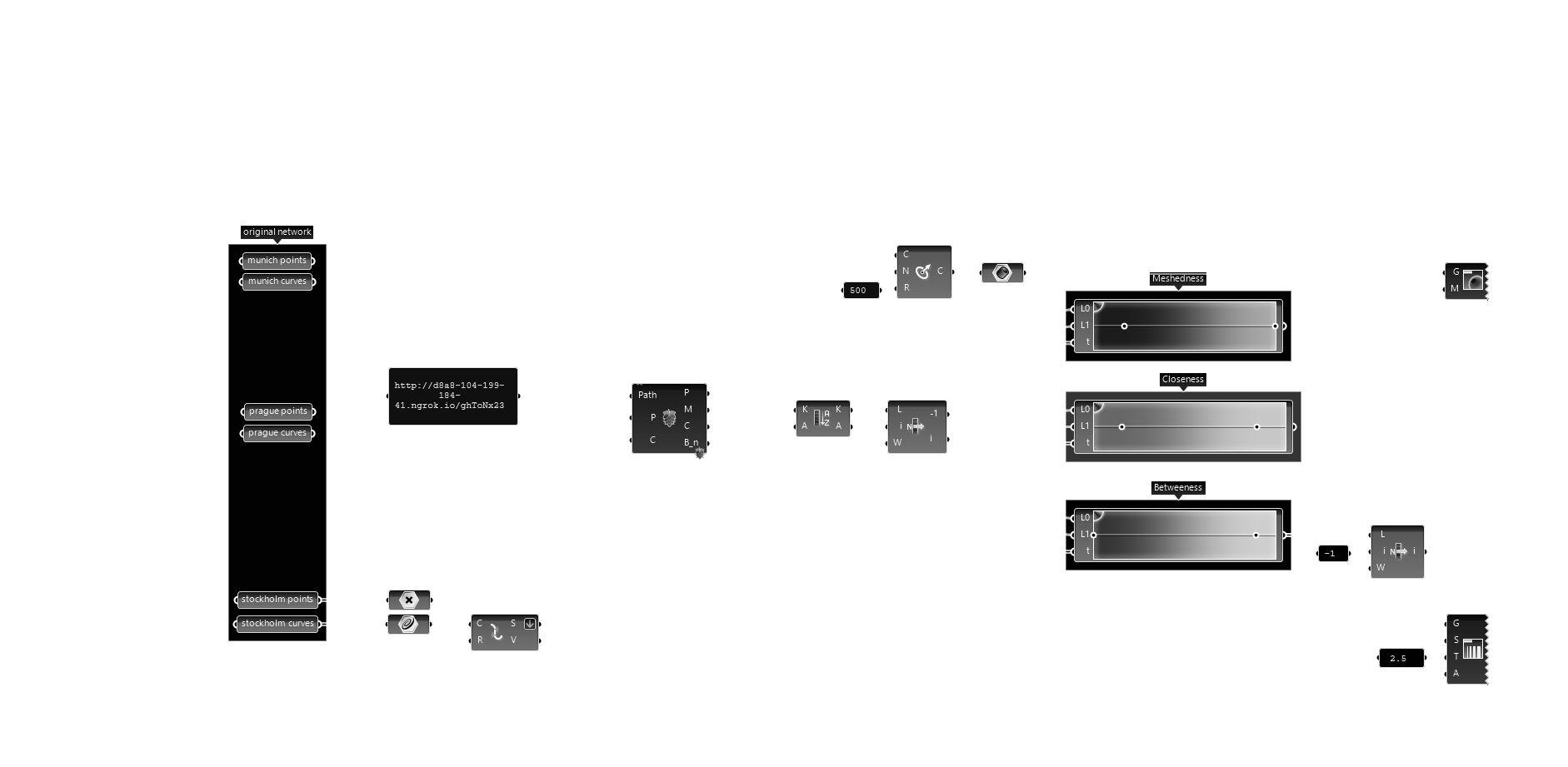
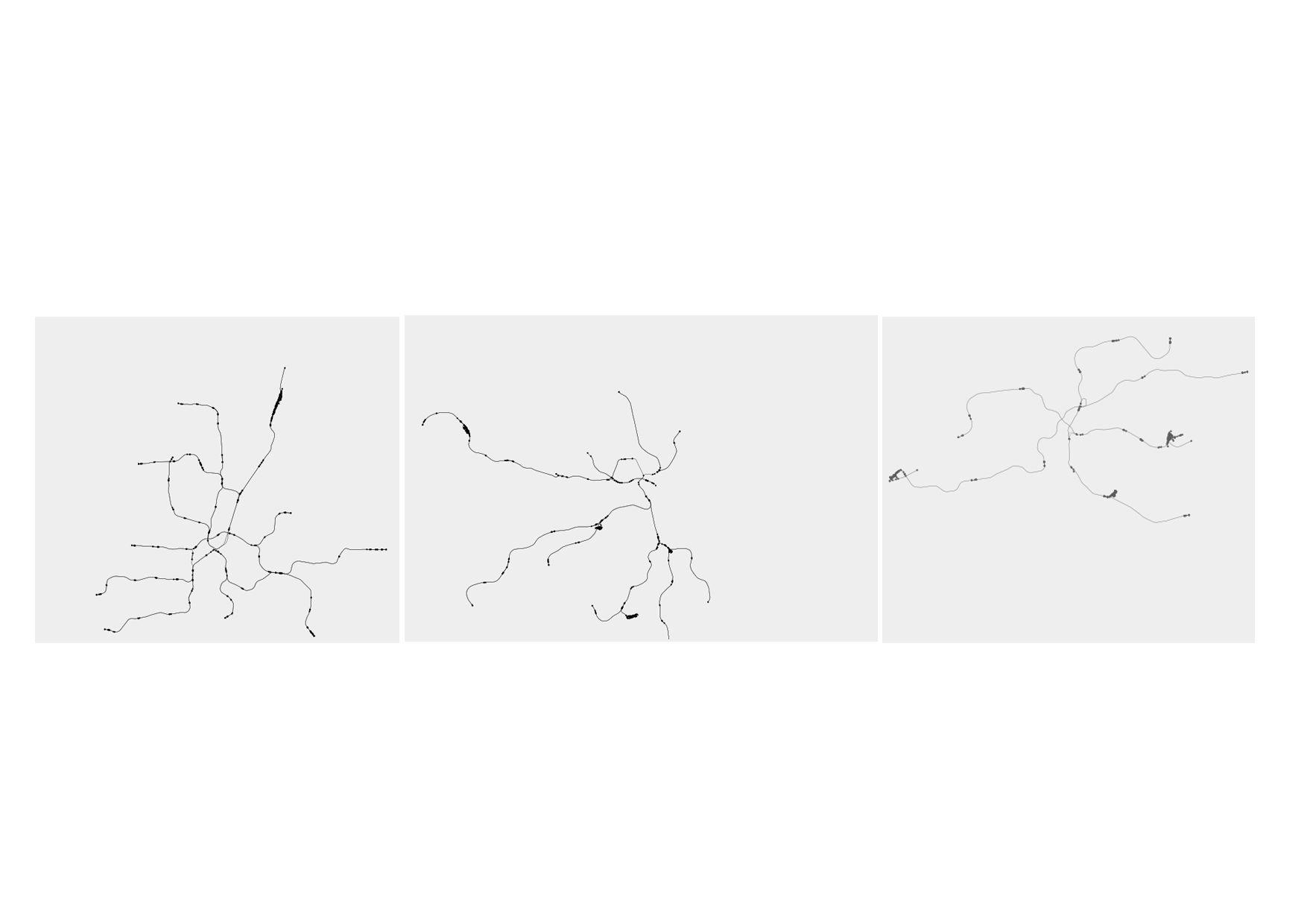
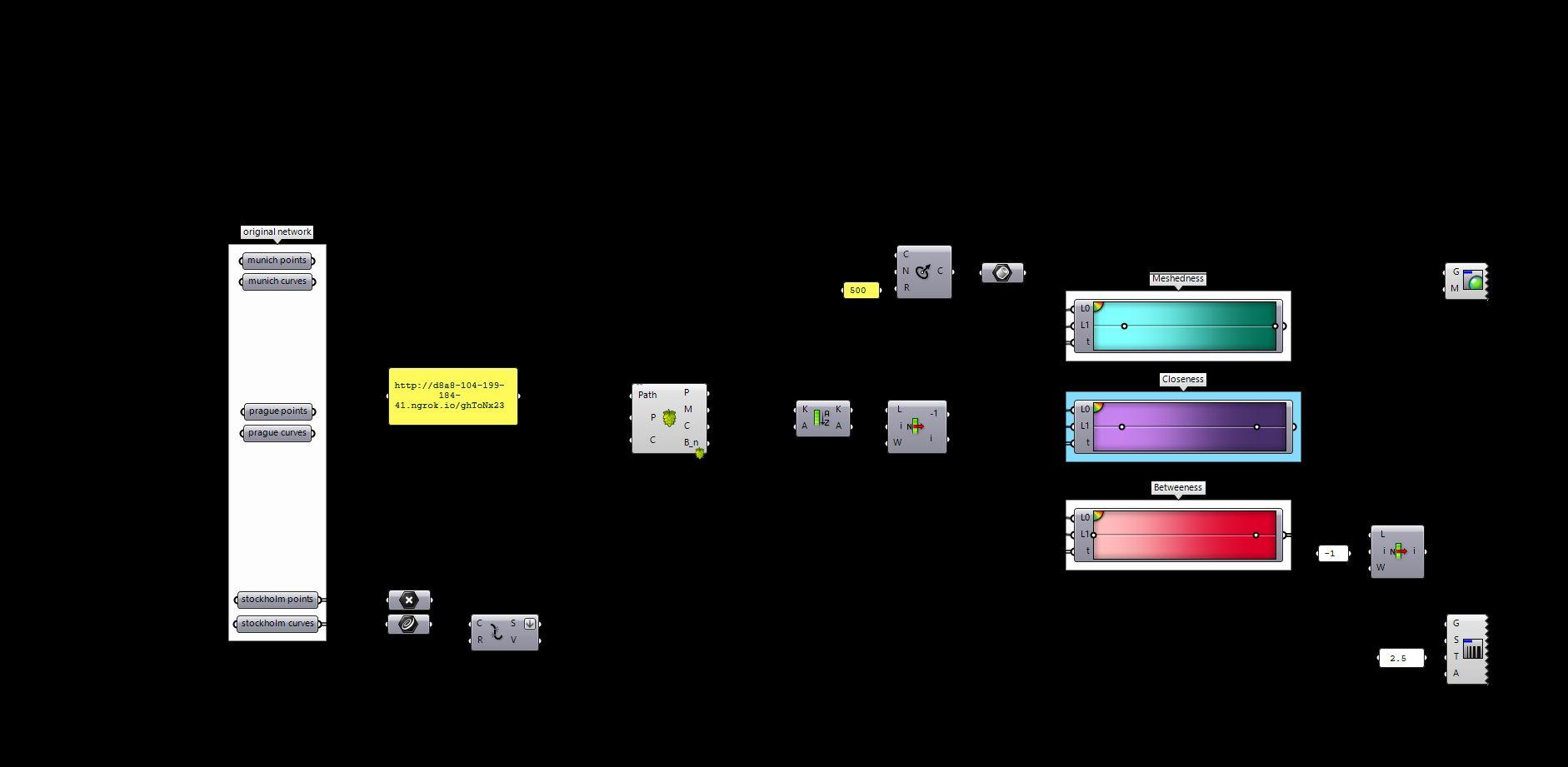
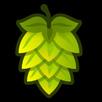
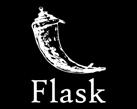
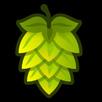

SUBWAY CITY
OBSERVATIONS
SUBWAY NETWORKS
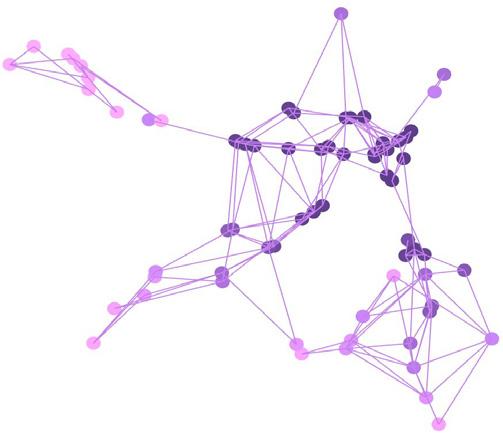
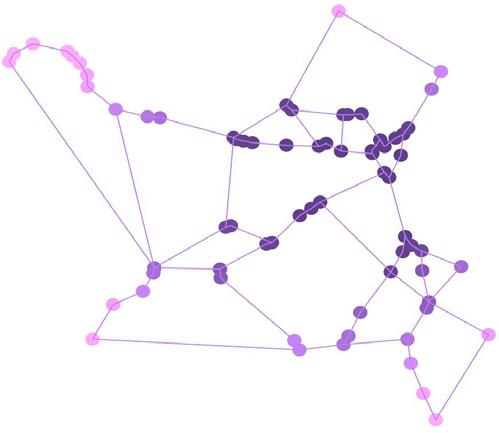
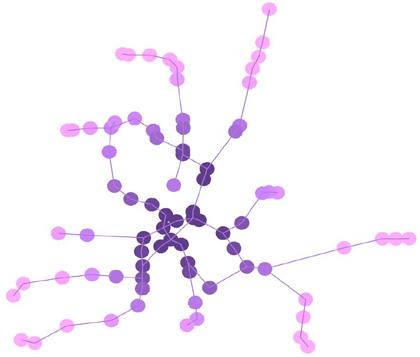
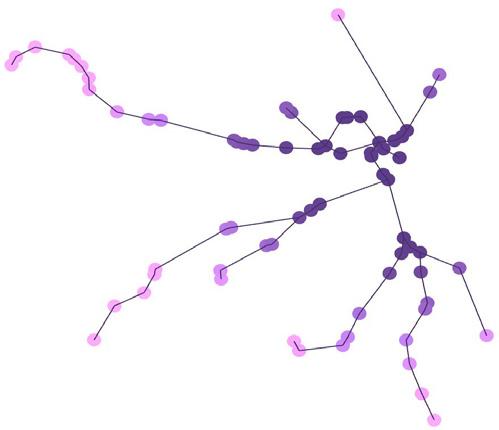
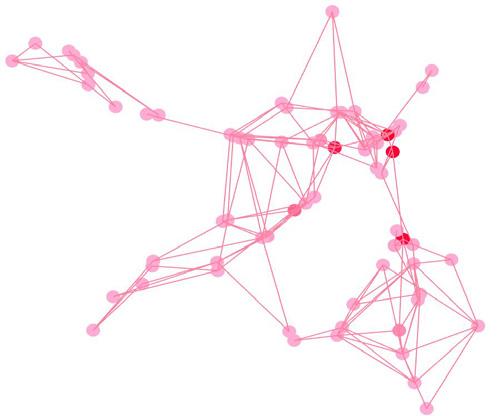
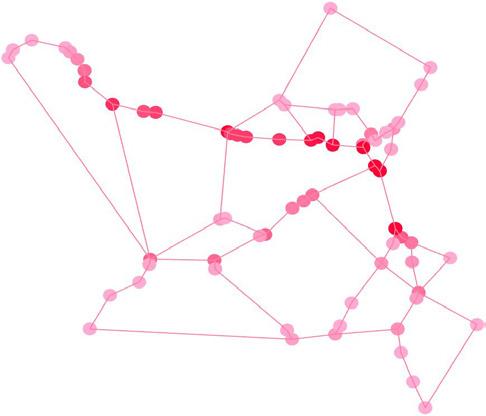

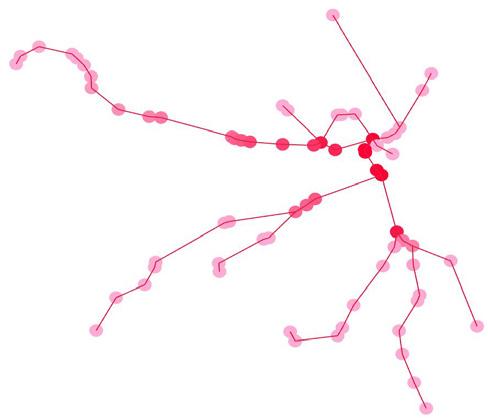
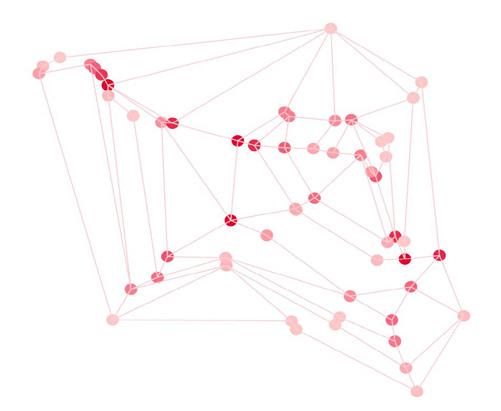
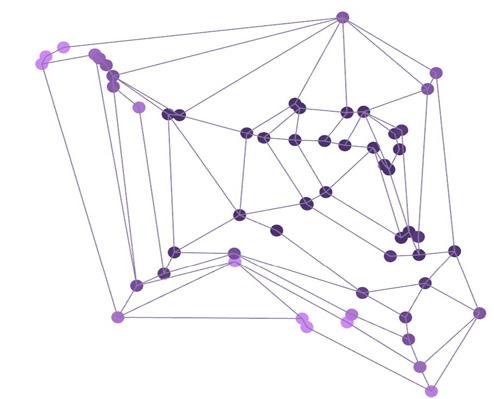
HIGHER INDEX VALUE
BETWEENNESS CENTRALITY CLOSENESS
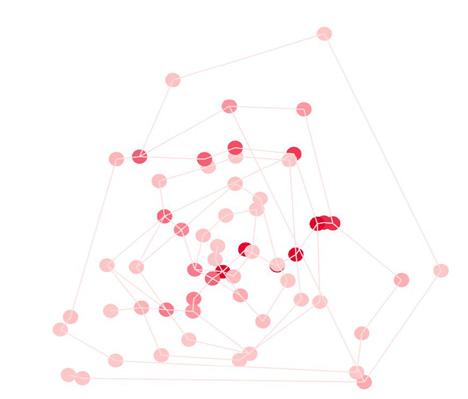
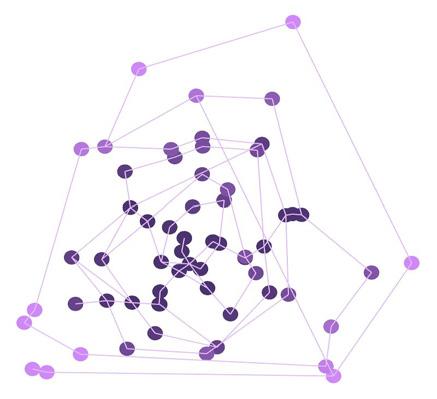
EXISTING SUBWAY NETWORK
NETWORK 01
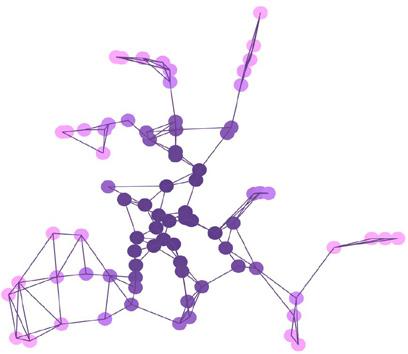
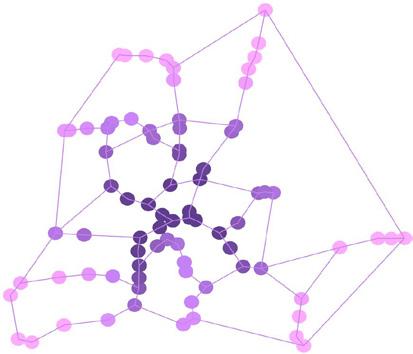
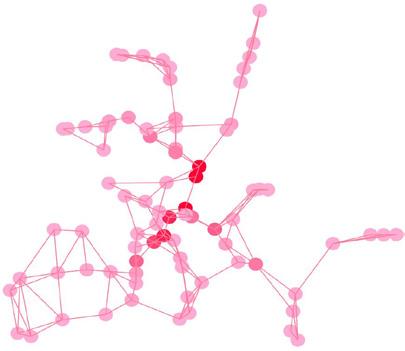
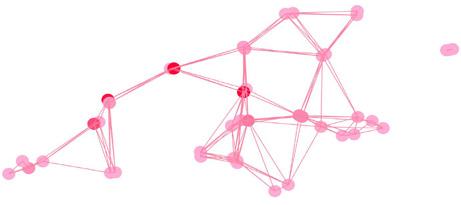
NETWORK 02
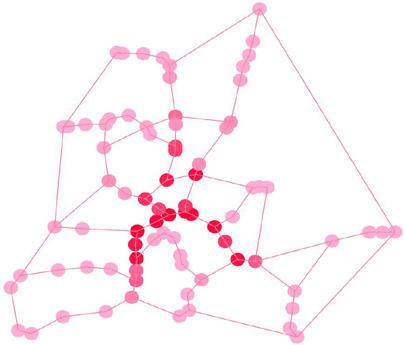
NETWORK 03
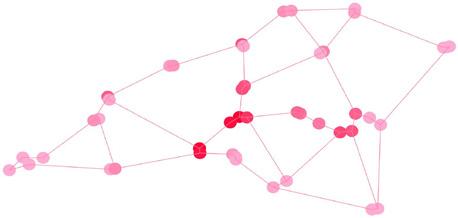
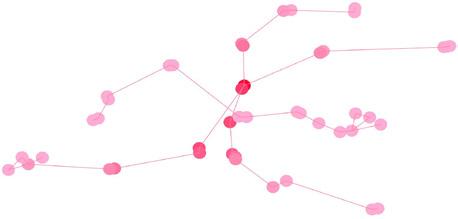
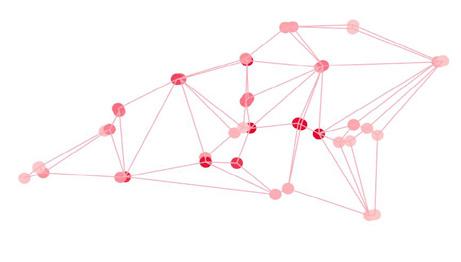
MUNICH SUBWAY
STOCKHOLM SUBWAY
Looking at all the graphs we can make some observations about how the proposed networks compare to the existing network. On average, we can see that the betweenness and closeness features are slightly higher in the proposed networks than in the existing network, while the meshedness is a lot higher. Network 1 has the highest betweenness score, network 2 has the highest closeness score and network 3 has the highest meshedness.
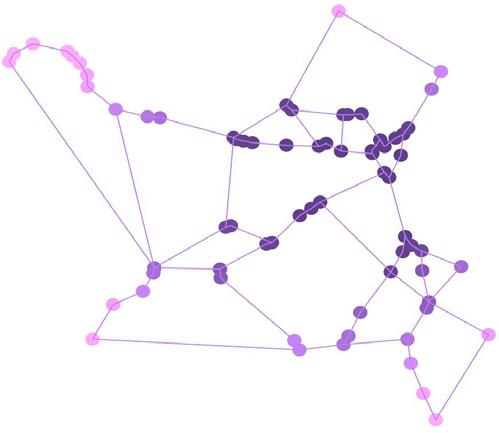
CLOSENESS
CENTRALITY MESHEDNESS

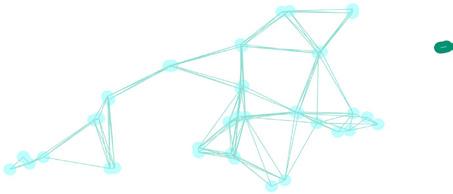
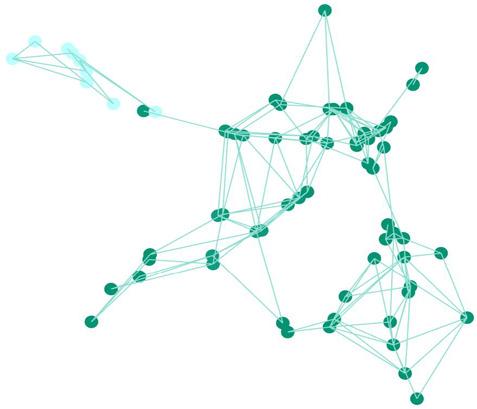
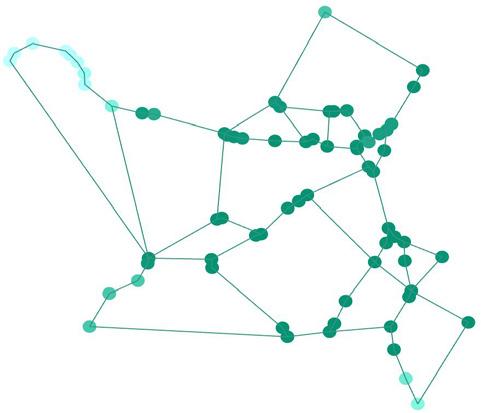
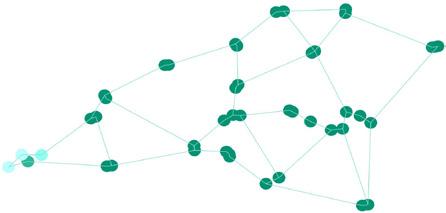
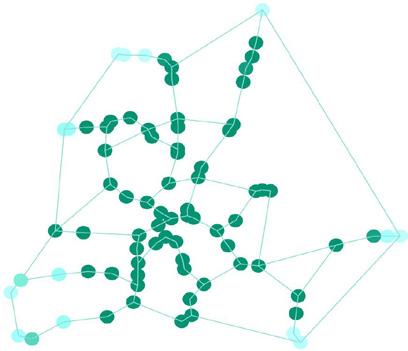
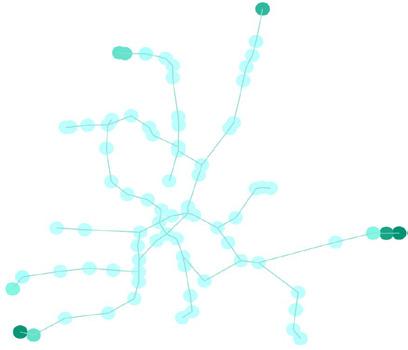
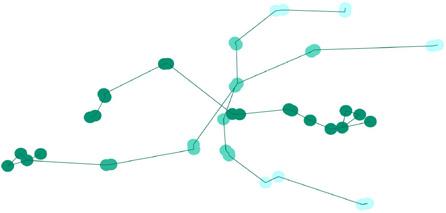
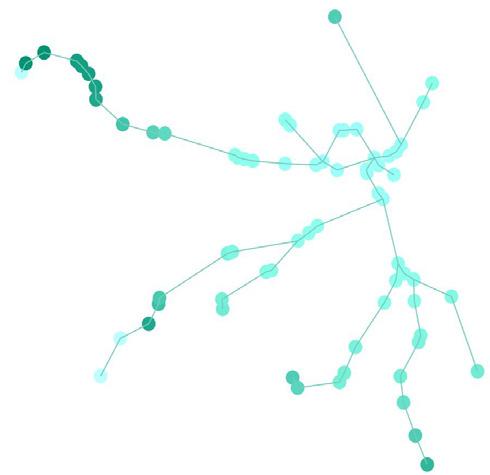
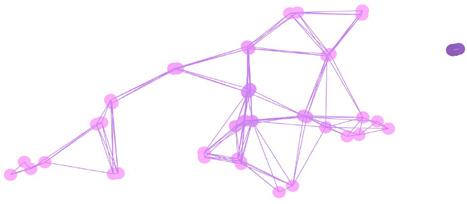
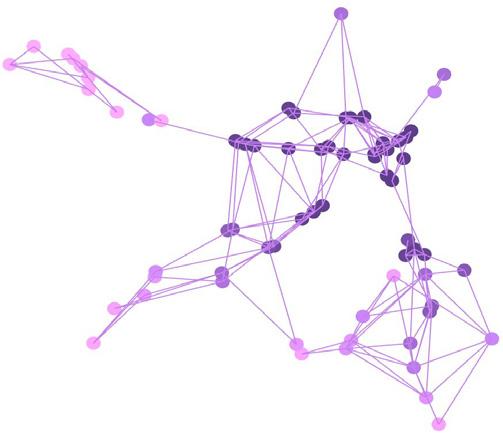
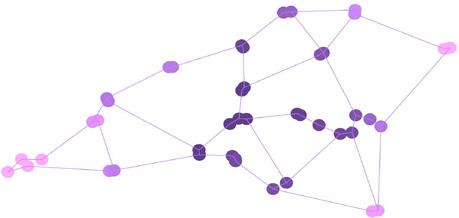
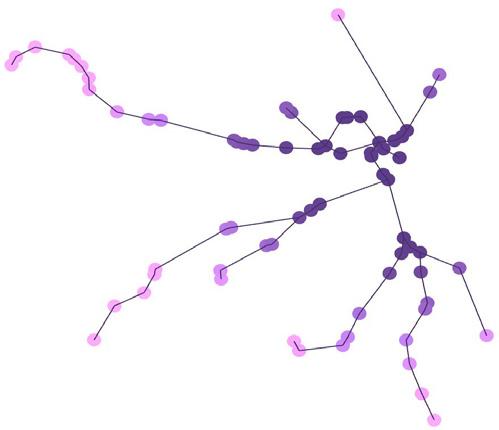
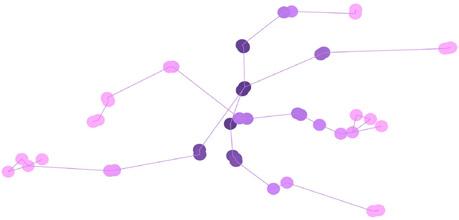
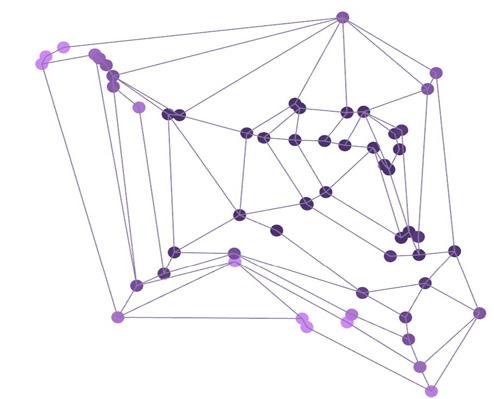
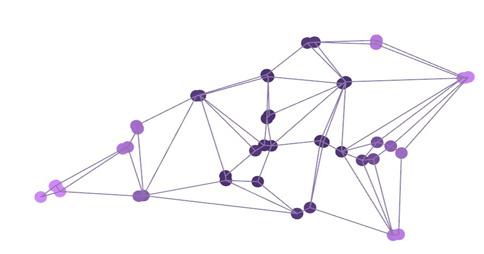
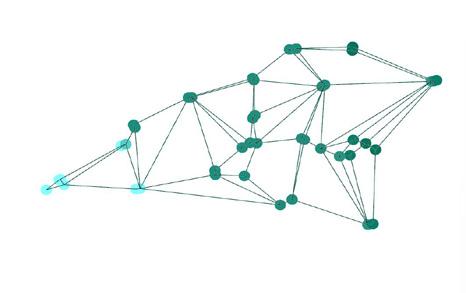
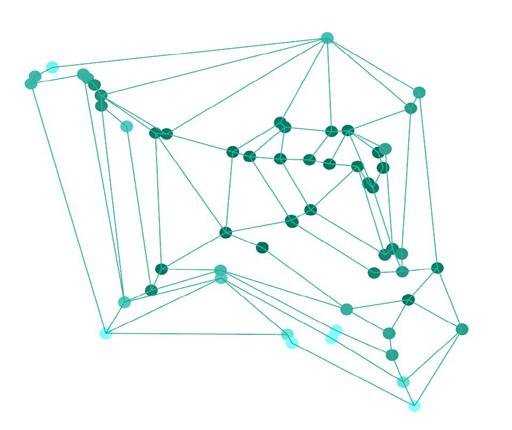
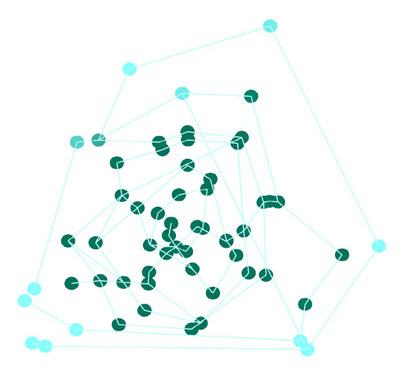
SUBWAY CITY
OBSERVATIONS
SUBWAY NETWORKS
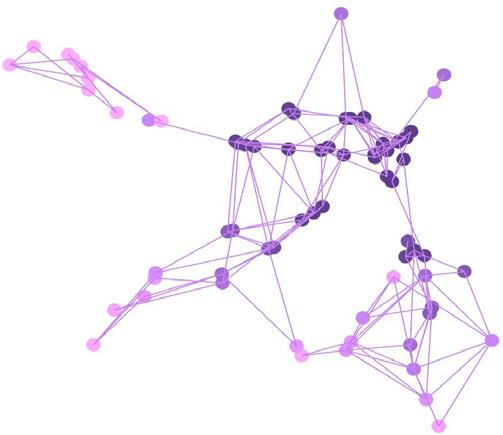
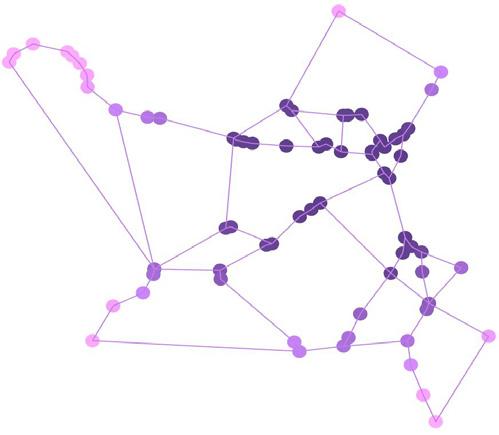
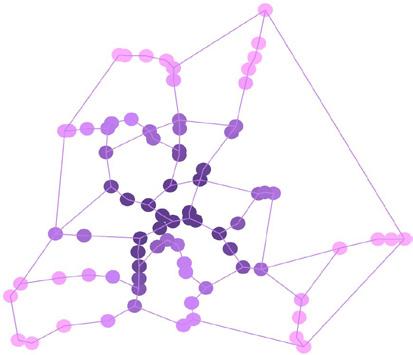
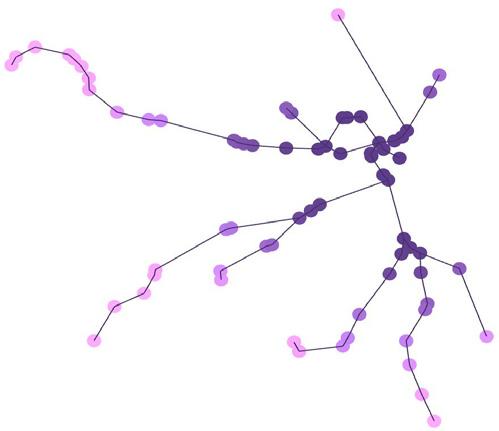
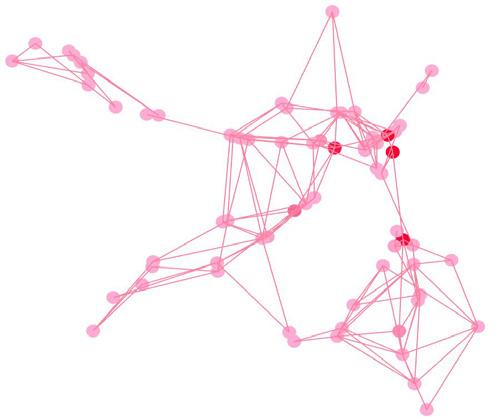
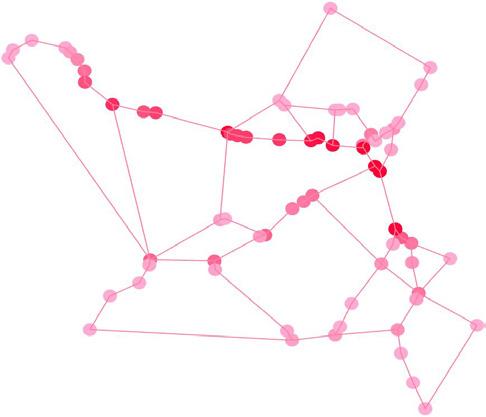
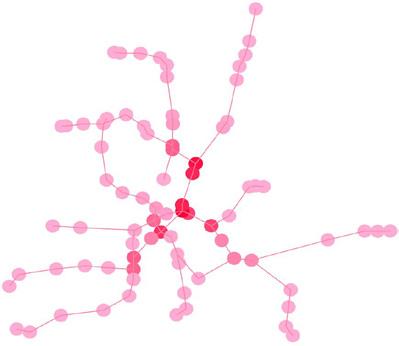
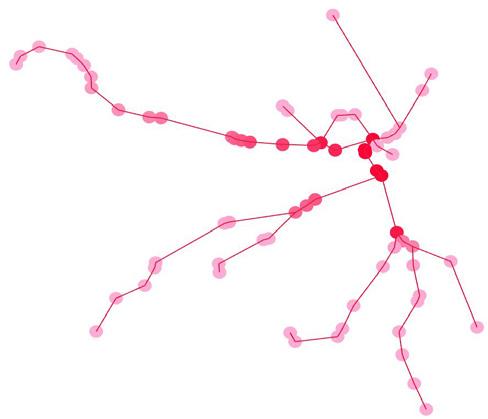
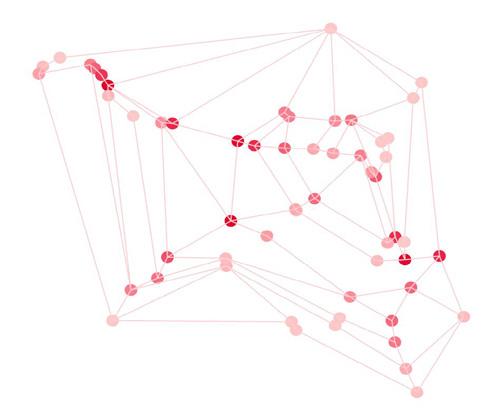
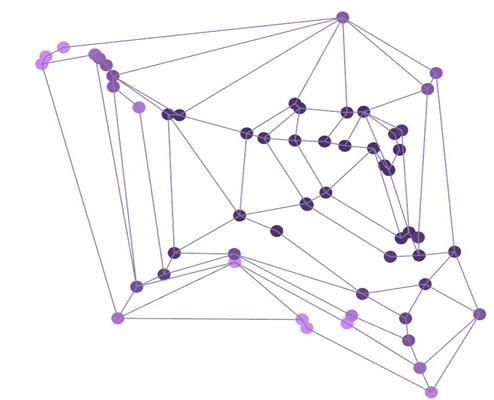
LOWER INDEX VALUE
BETWEENNESS CENTRALITY CLOSENESS
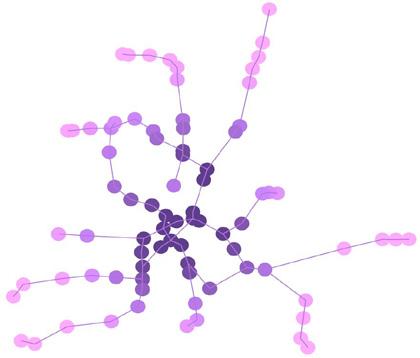
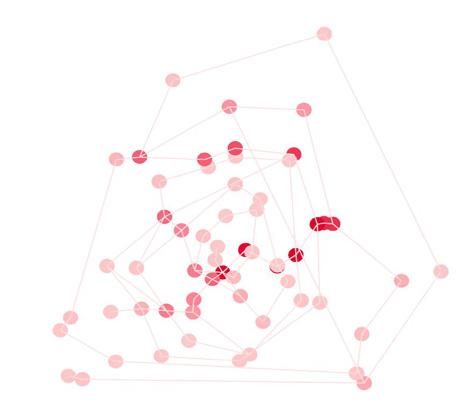
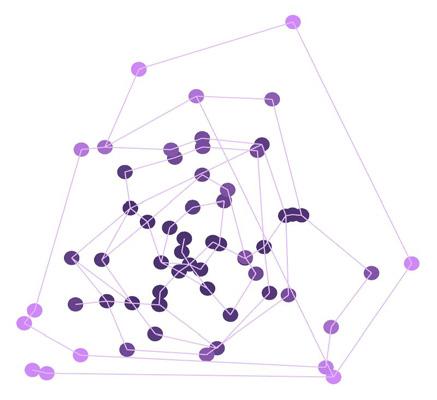
EXISTING SUBWAY NETWORK
NETWORK 01
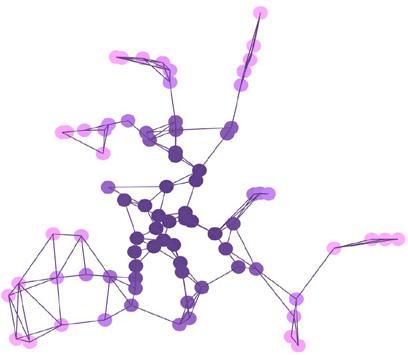
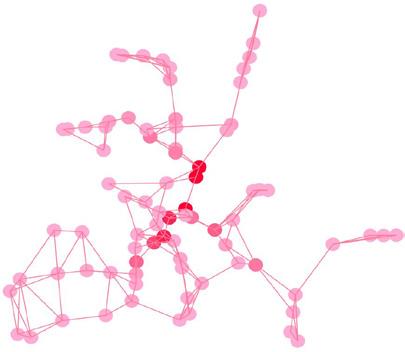
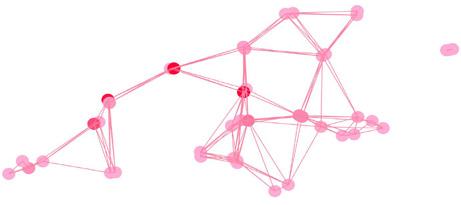
NETWORK 02
NETWORK 03
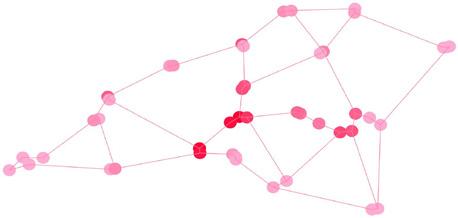
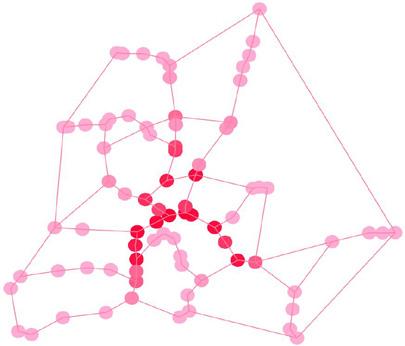
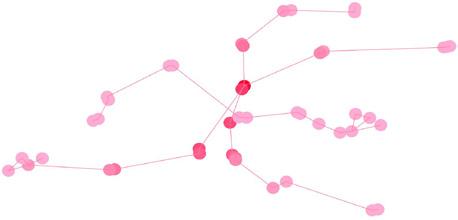
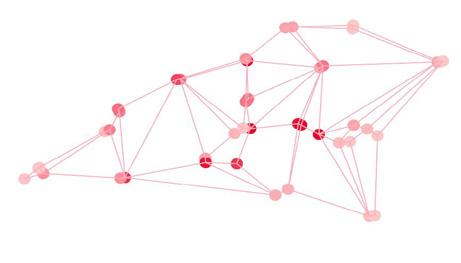
MUNICH SUBWAY
STOCKHOLM SUBWAY
PRAGUE SUBWAY MUNICH SUBWAY
Observing the lower index values, we can see that Network 2 has the lowest betweenness score and meshedness, and network 1 has the lowest closeness score. The original networks have many dead ends and it’s clear that most of the lines converge to a central area, whereas the drawn maps do not display such a distinct central area.
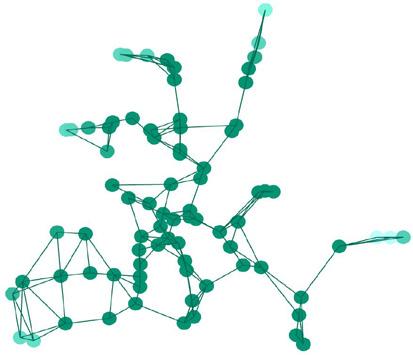
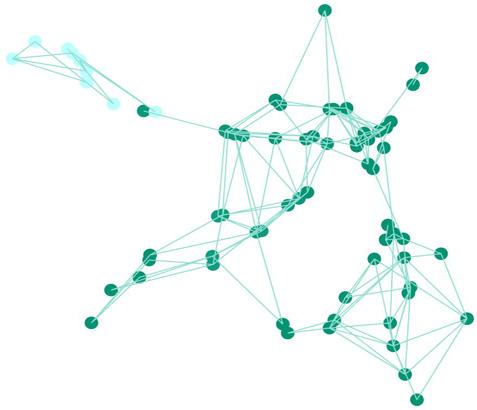
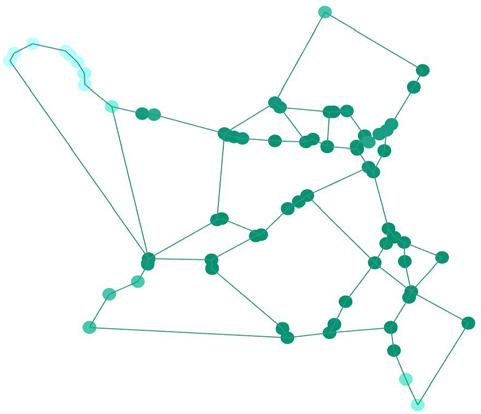
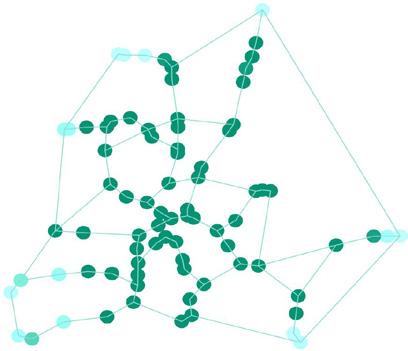
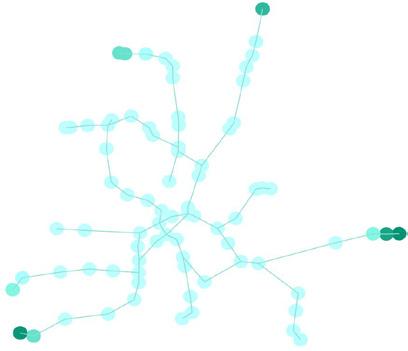
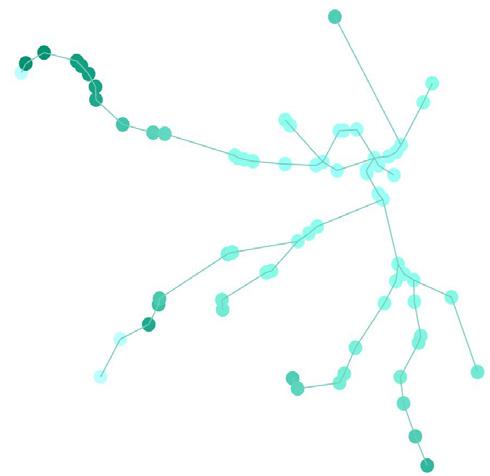
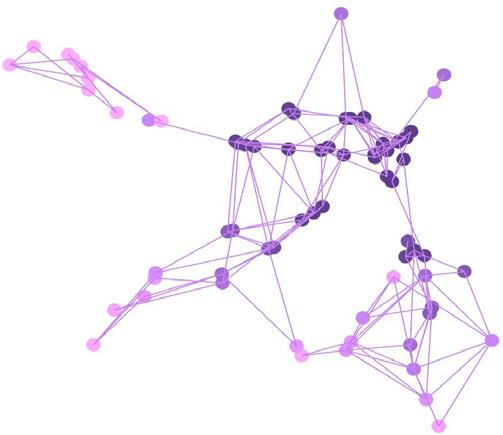
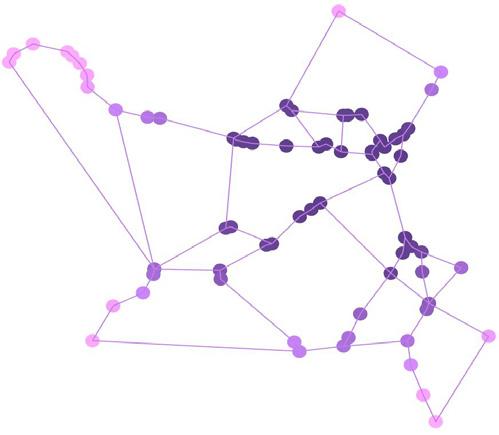
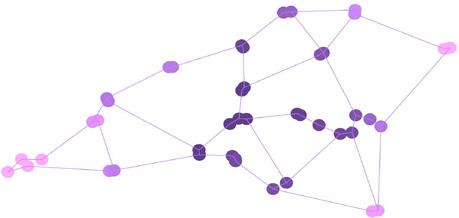
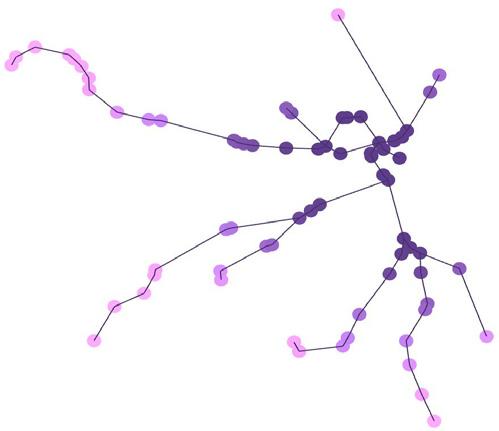
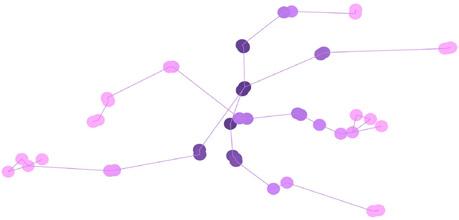
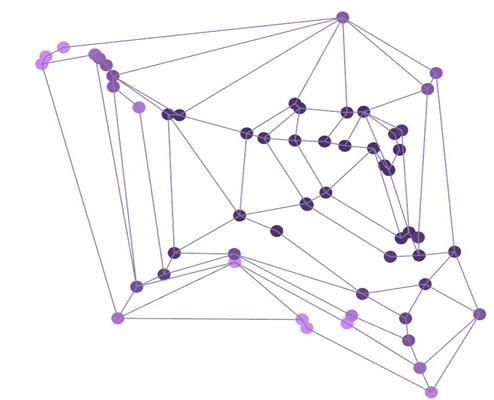
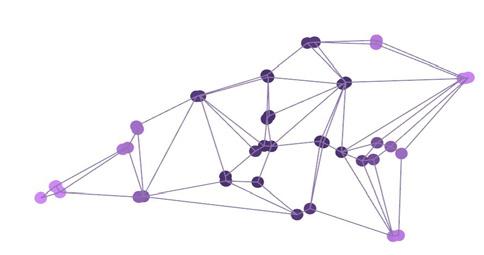
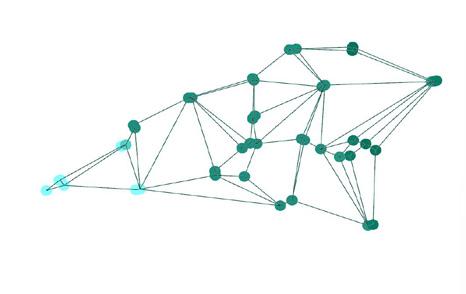
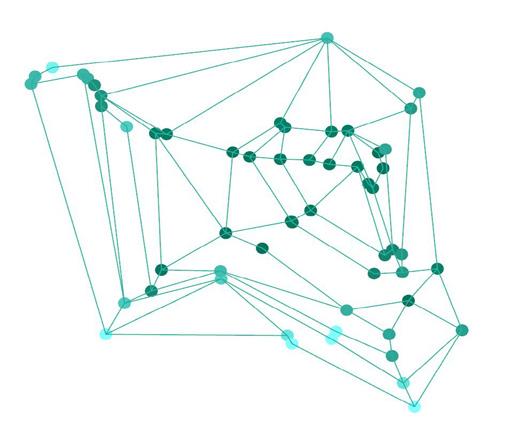
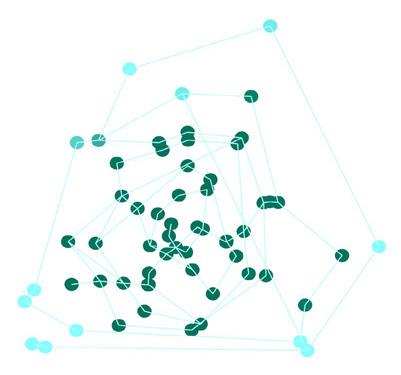
CLOSENESS
CENTRALITY MESHEDNESS
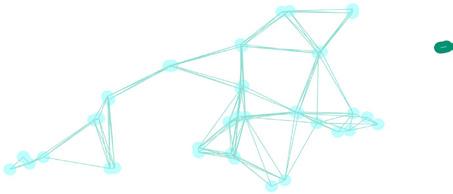
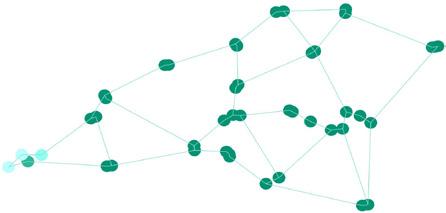
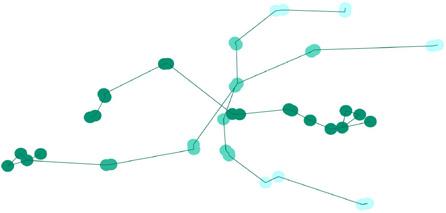
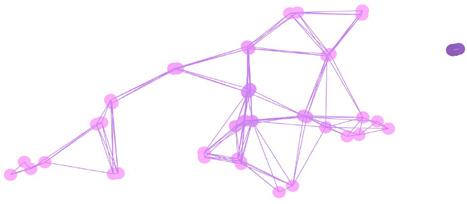
NORTHERN LIGHT PAVILION

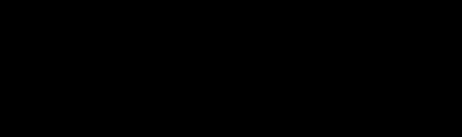
Northern Lights Pavilion is an interactive web-based application to envision a piece of contemporary urban infrastructure made of ice blocks that would activate the areas around northern cities in Sweden. The starting point of this experimental pavilion is based on the quarter-circular Truchet pattern generating in-between maze-like curvature spaces, controlled by width, length, and pattern. The aim is to envision ephemeral spaces made of ice and snow blocks, especially in the season between December-April when many artistic projects are held there.
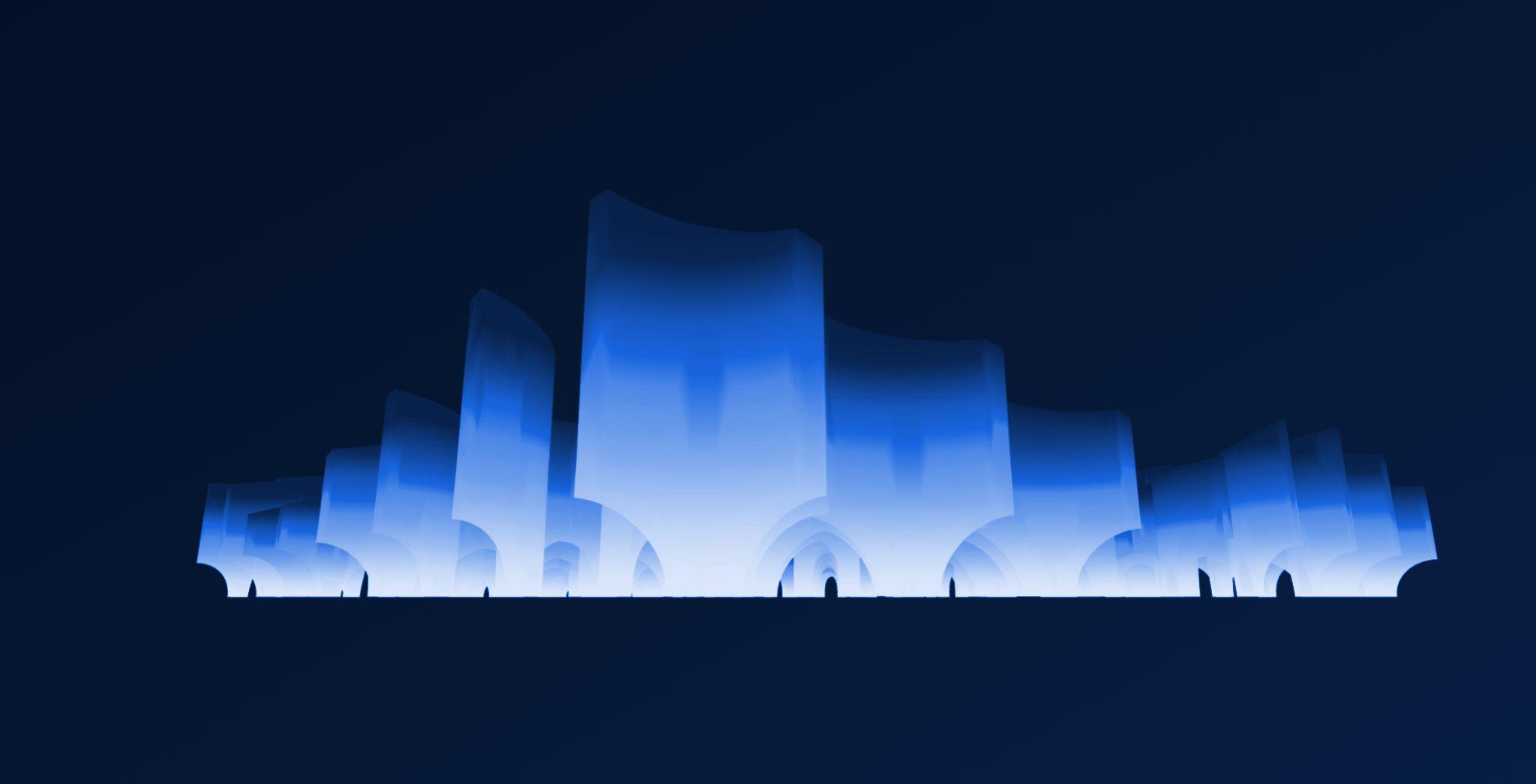
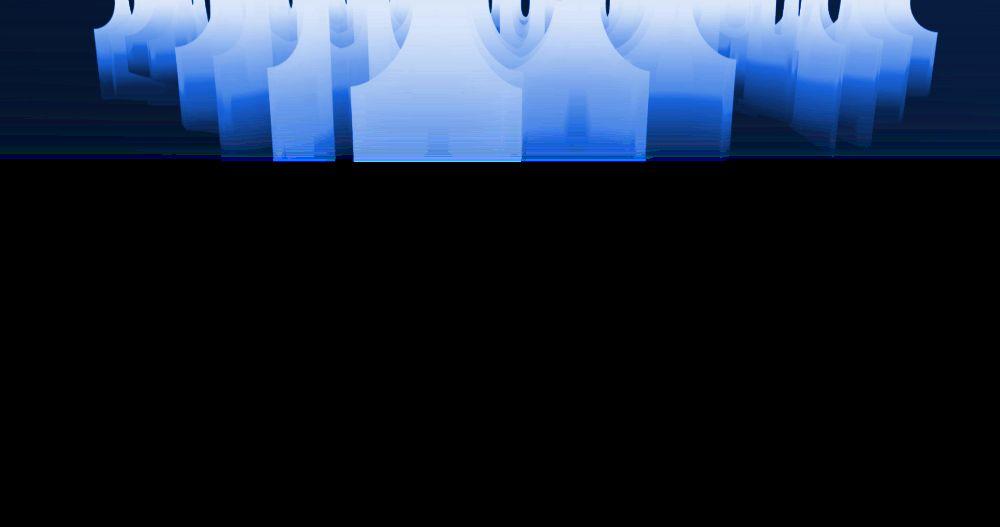
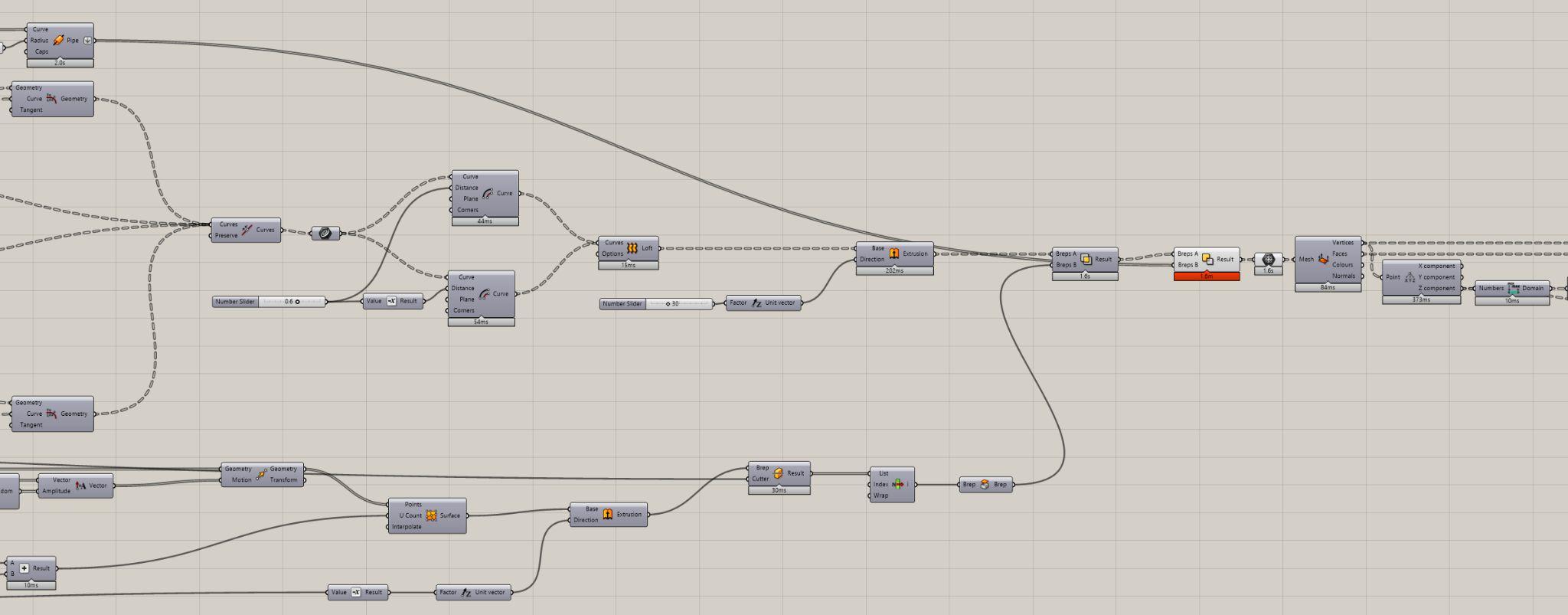
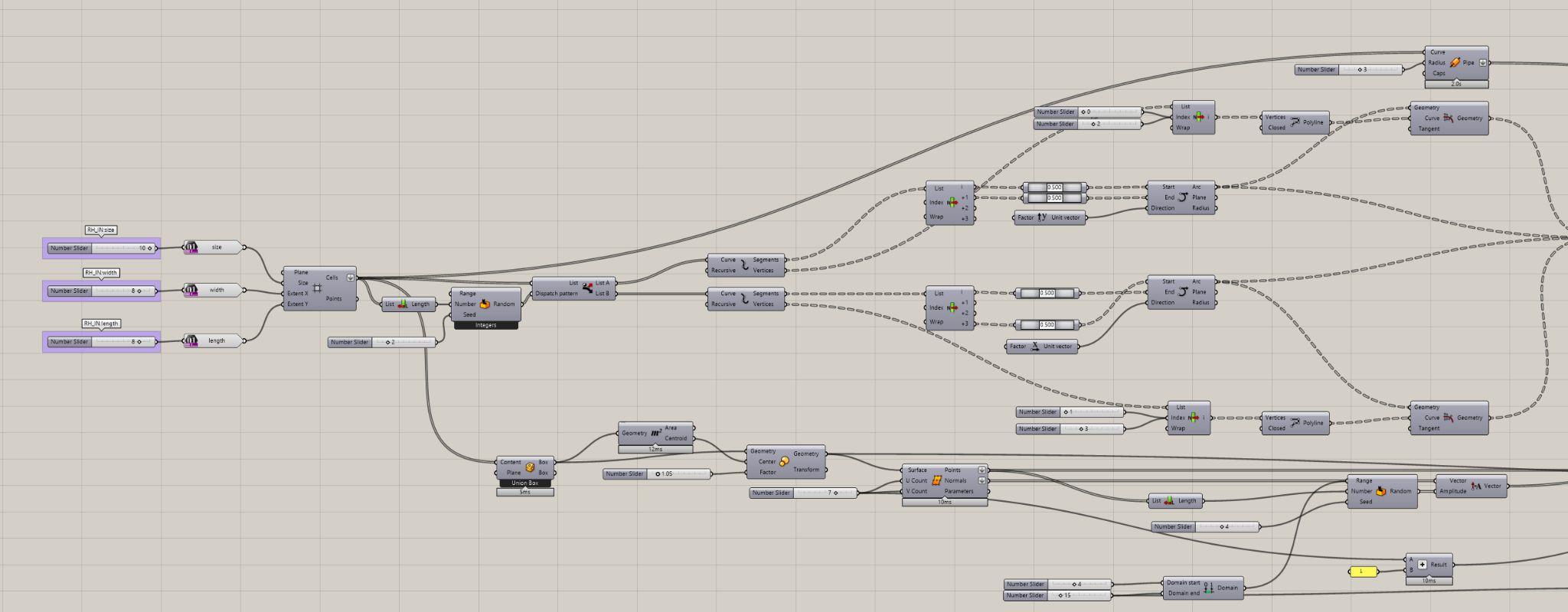

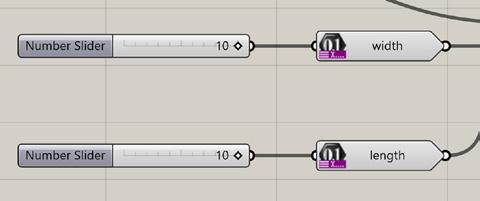

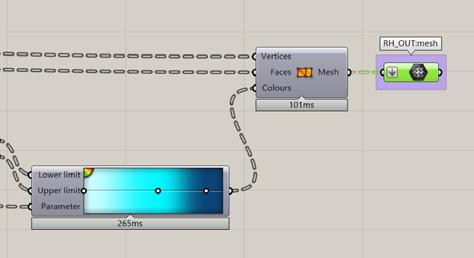
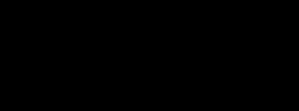













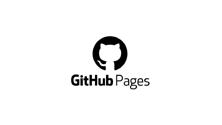

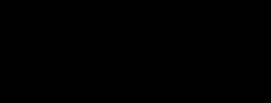
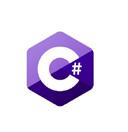





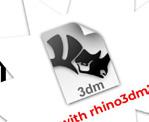
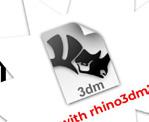
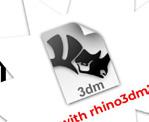
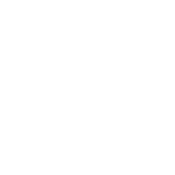
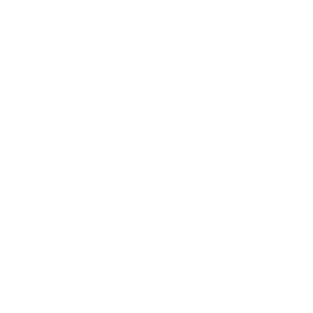
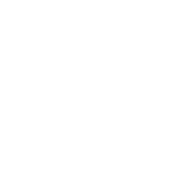
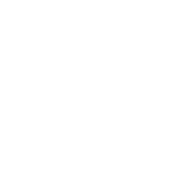
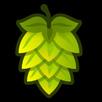
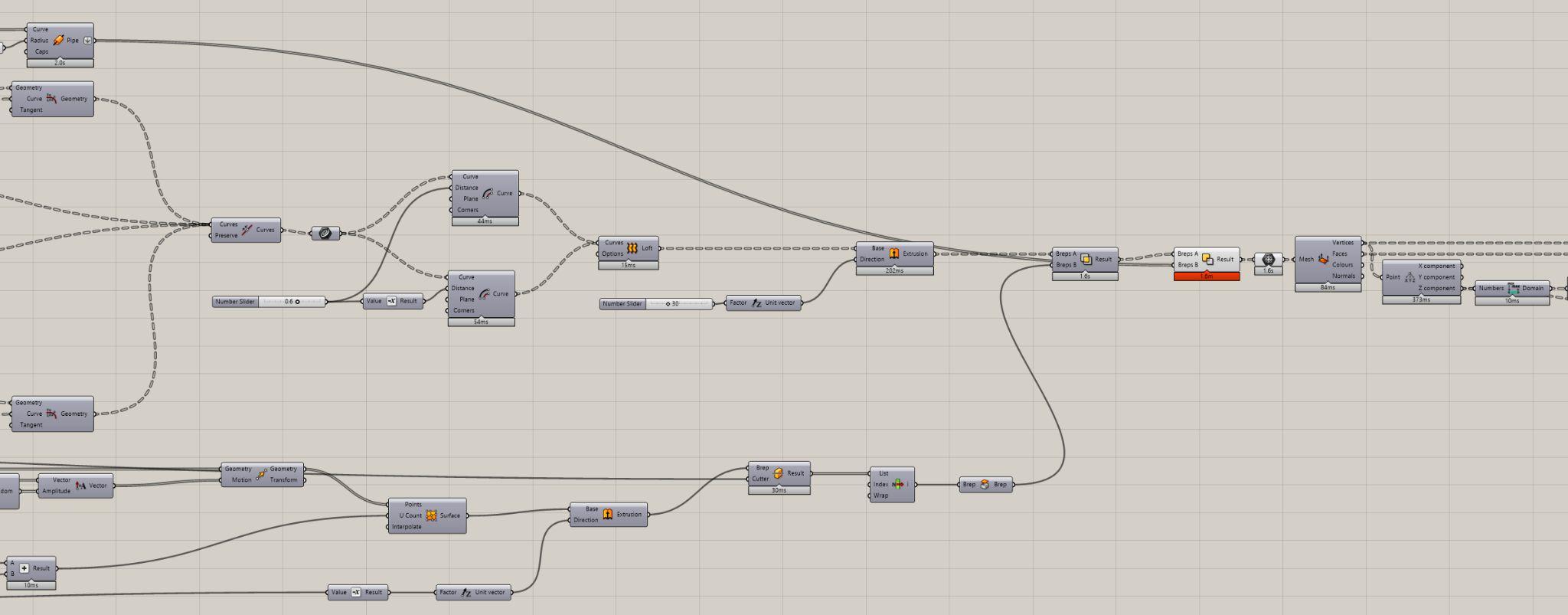
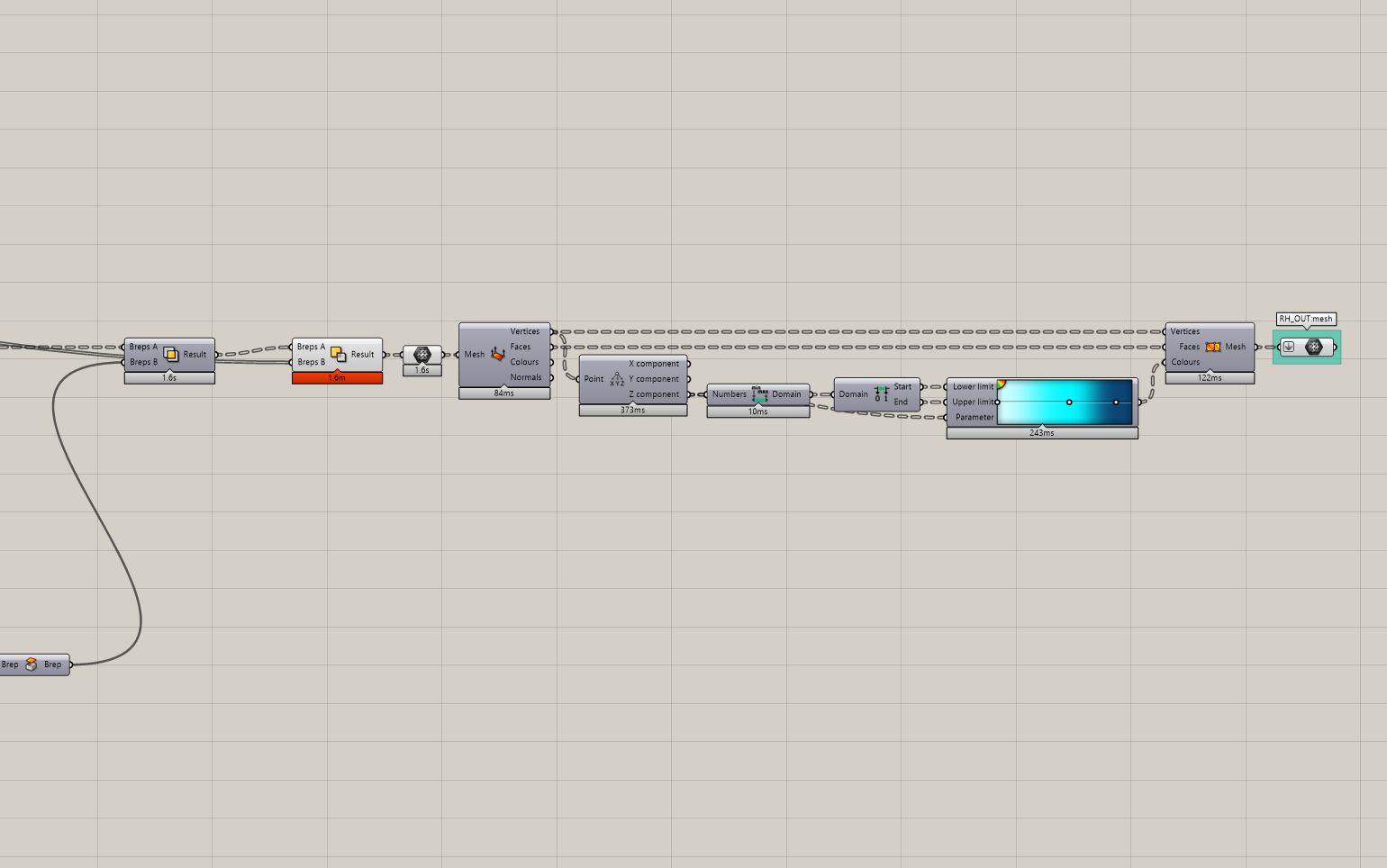
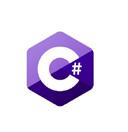



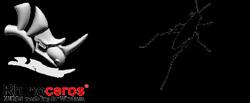
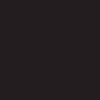
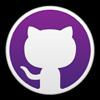
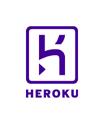
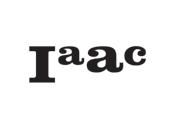

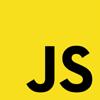


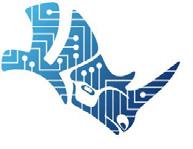
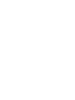

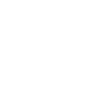
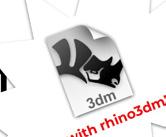
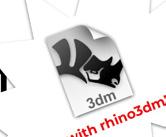
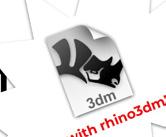
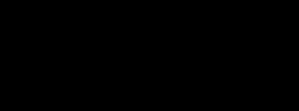
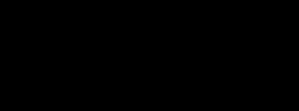
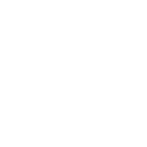
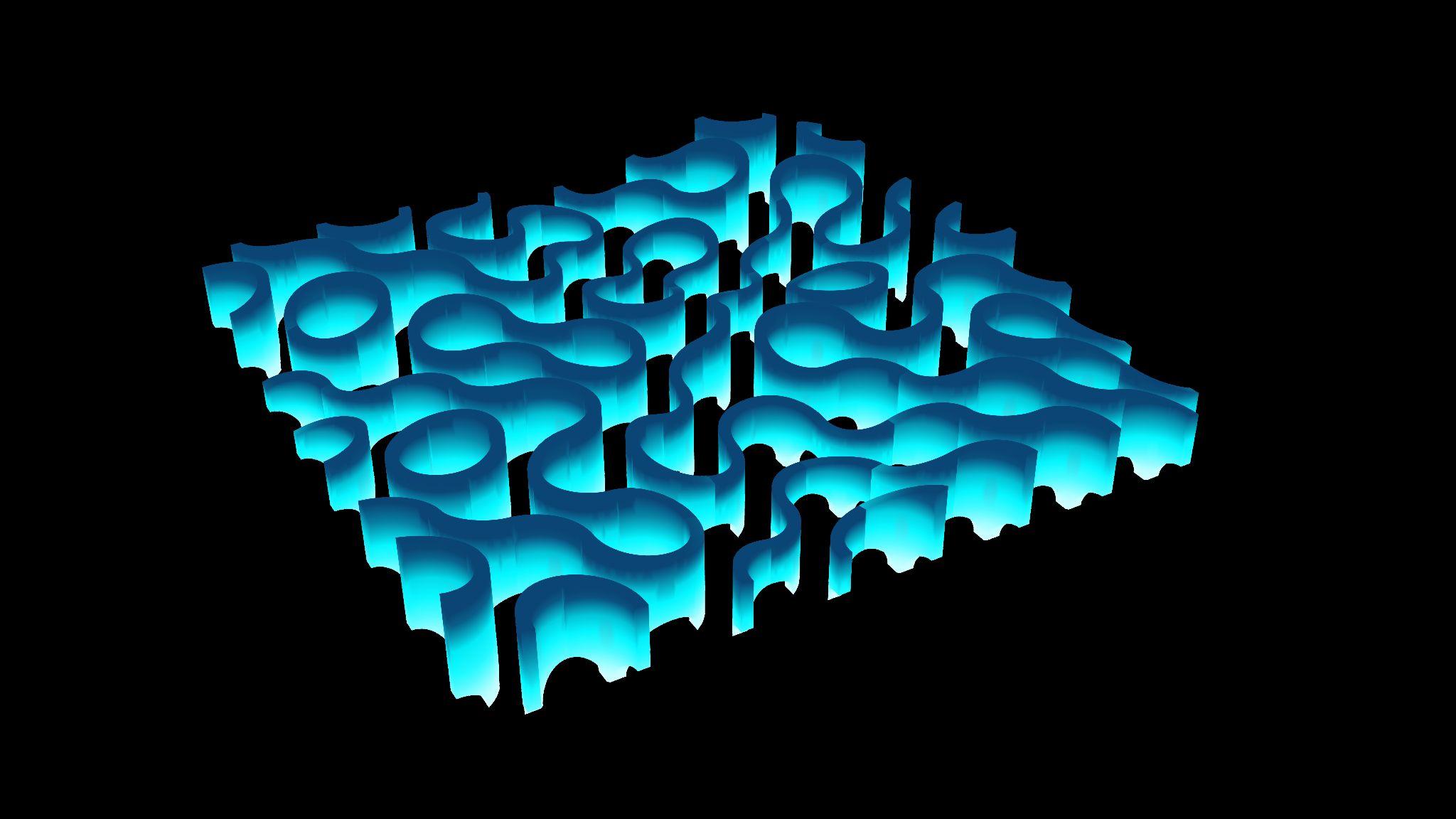
NORTHERN LIGHT PAVILION
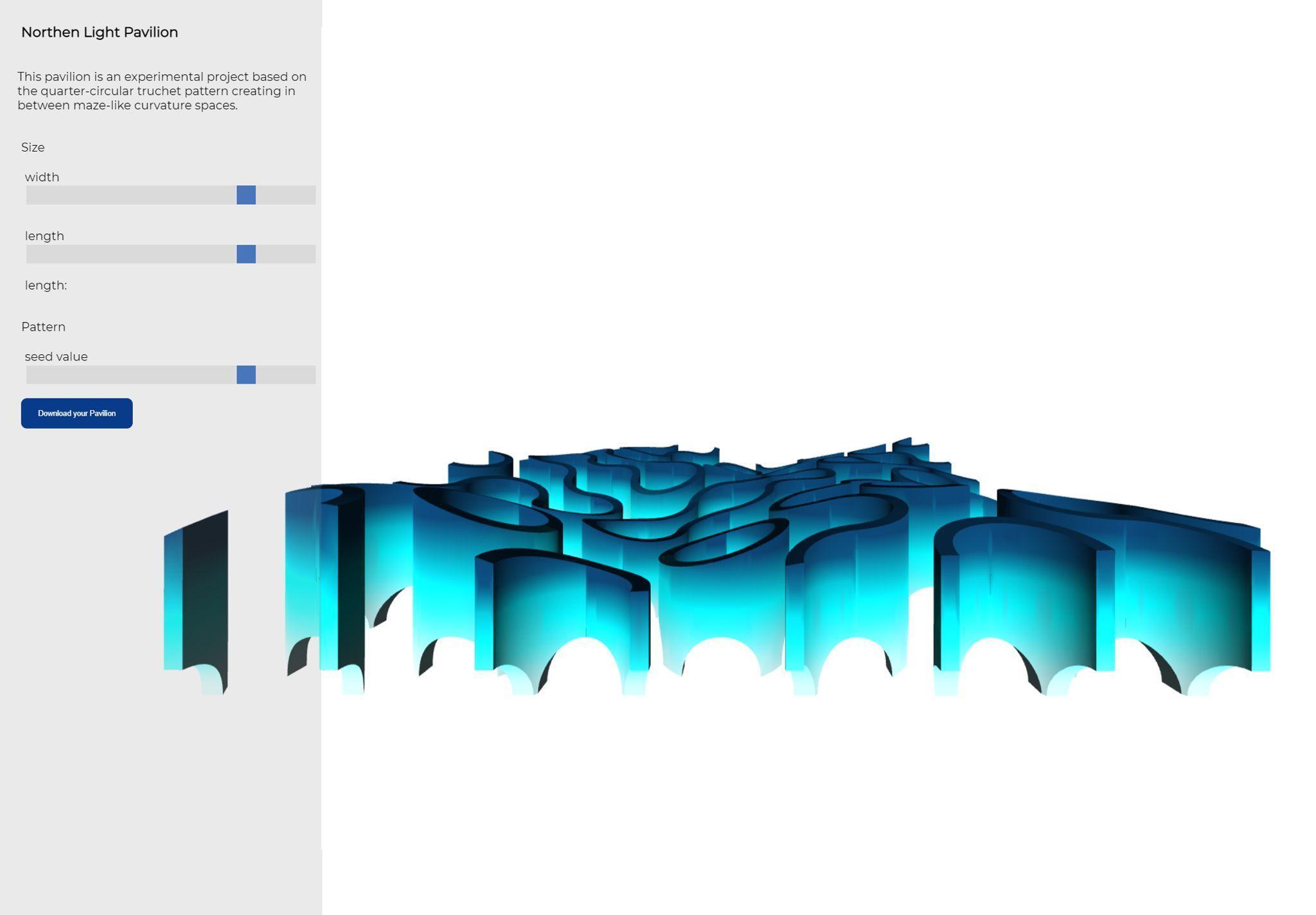
Instructions:
How does it work?
● First, you need to run Rhino. compute,
● Go to the App page at this link: https://jumanahamdani.github.io/pavilion/
● Change the Inputs: Width, and lengths of the pavilion, then you can input the pattern of the pavilion in the seed value based on your aesthetic preferences.
● The OutPuts would be the Geometry of the ice pavilion, where you can download it.
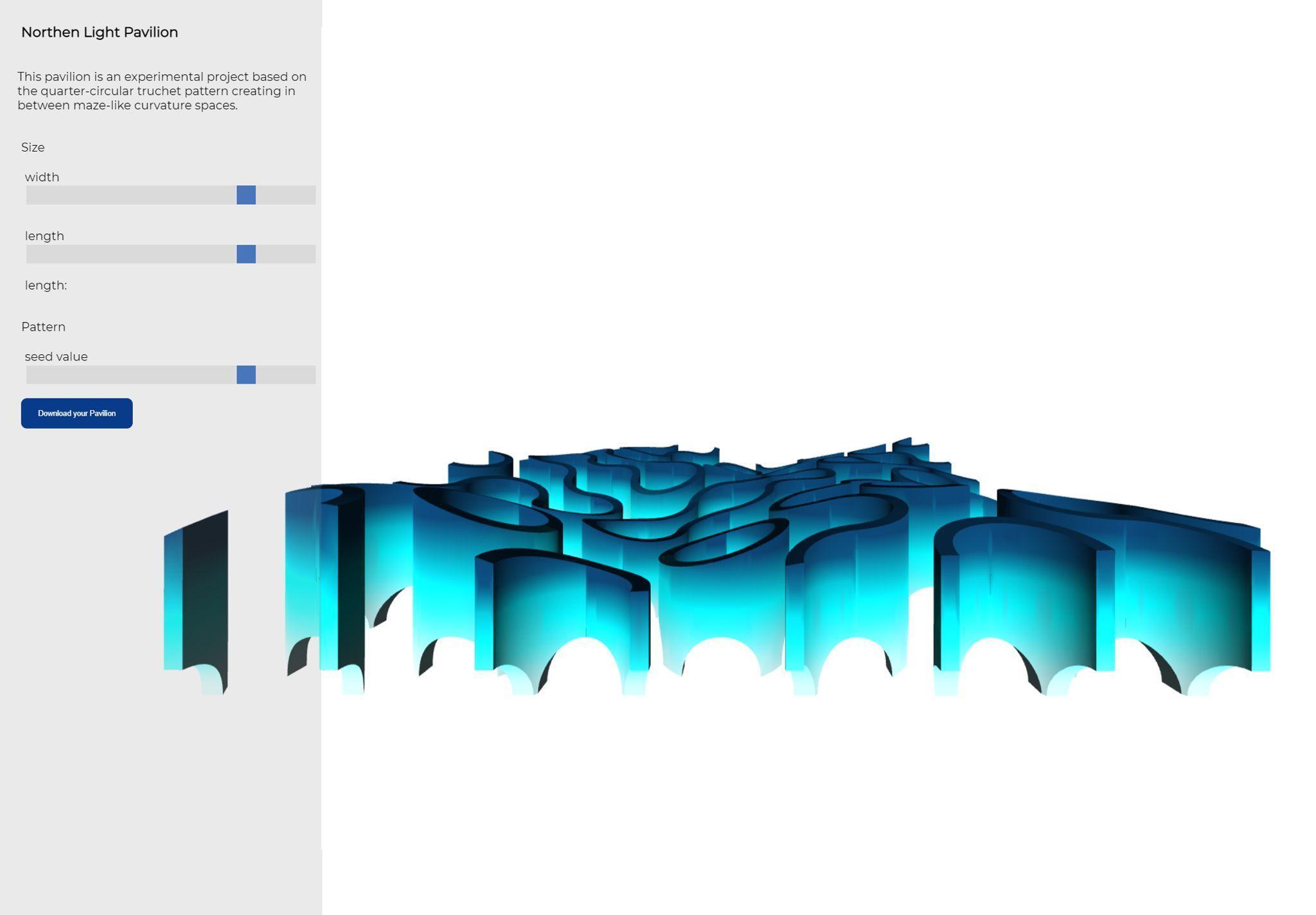
NORTHERN LIGHT PAVILION
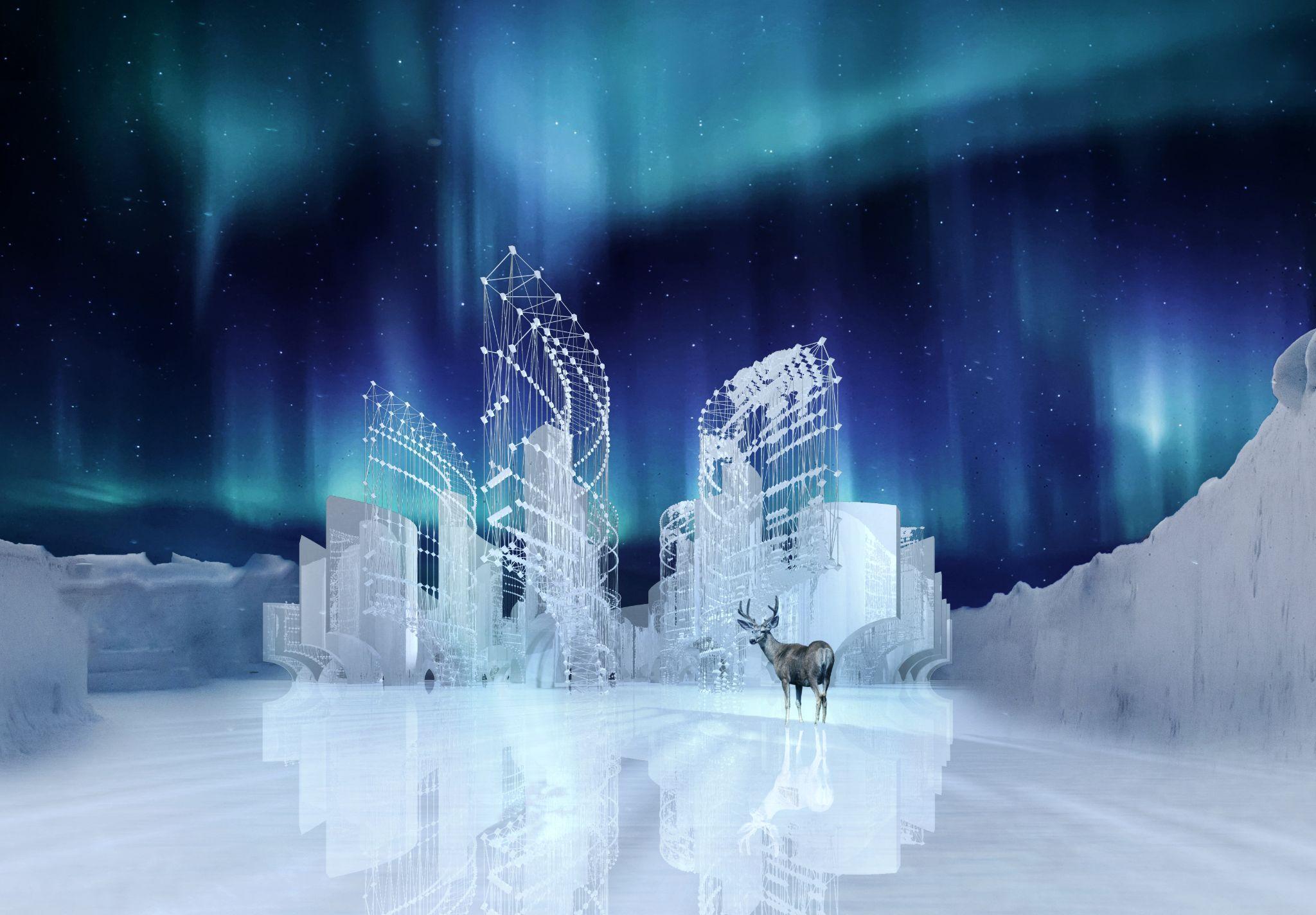
The objective behind creating such an app for the municipalities of the northern area of Sweden is to introduce it to the communities of these cities as a participatory design tool to democratize the decision of creating those public spaces.
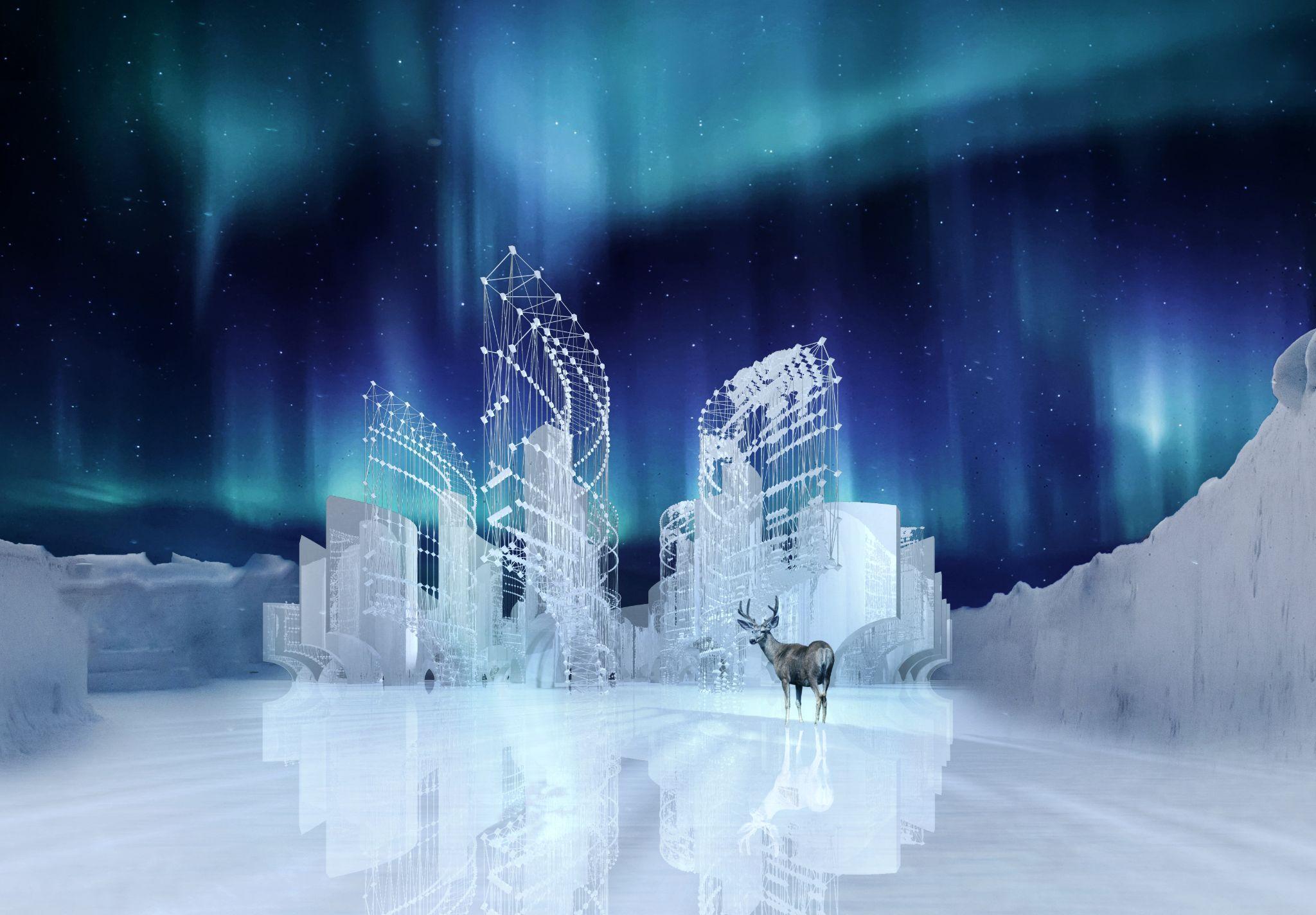
NEBULAE: ORBIT SPACE HABITAT
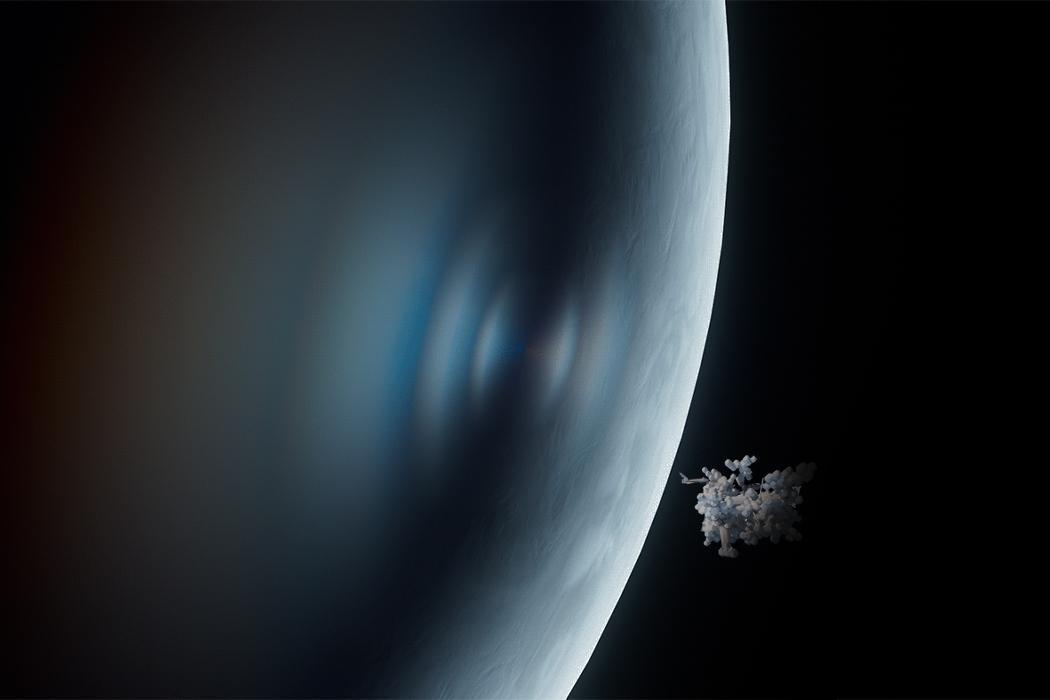
20
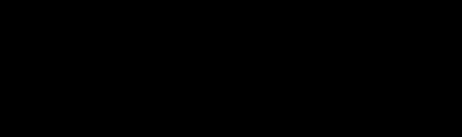
Date: 2022
Location: Low Earth Orbit
Collaborators: Irene Martín, Jumana Hamdani, Pablo Jaramillo Pazmino
Instructor: Xavier De Kestelier, Levent Özruh
Project Type: Academic at IAAC
Tools: Revit, Rhino.inside Revit, Speckle, 3D Max, Photoshop, Rhino,Grasshopper including plugins: Weaverbird, Pufferfish, Human, LunchBox, GeomGym

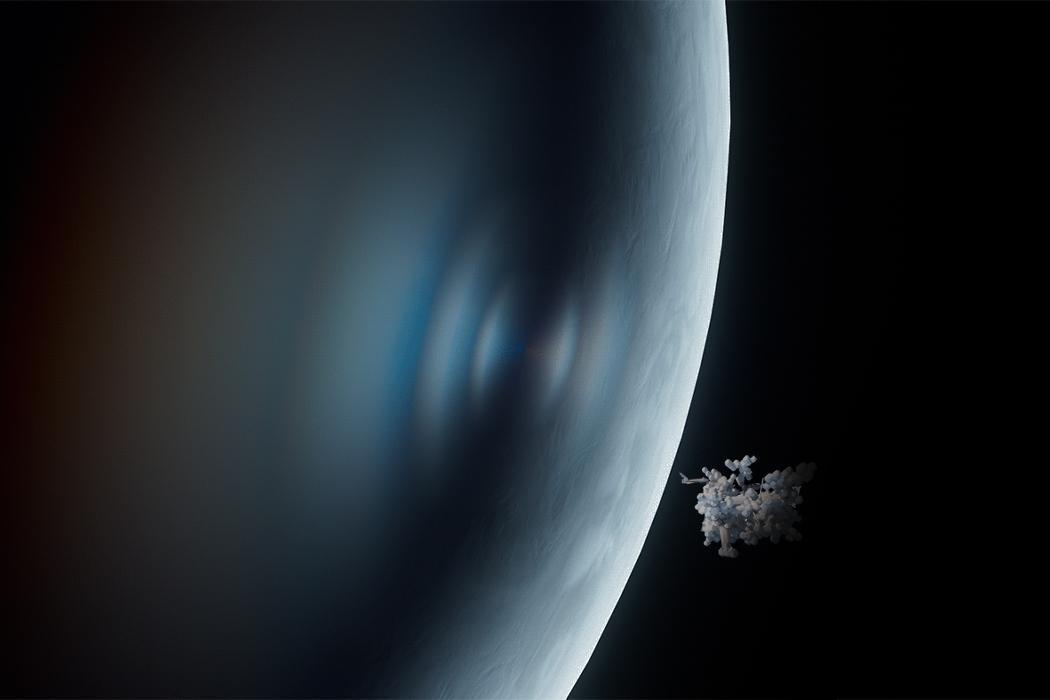
NEBULAE
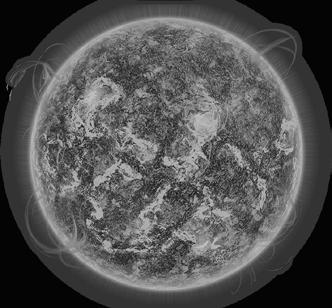
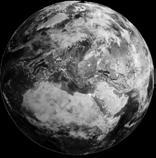
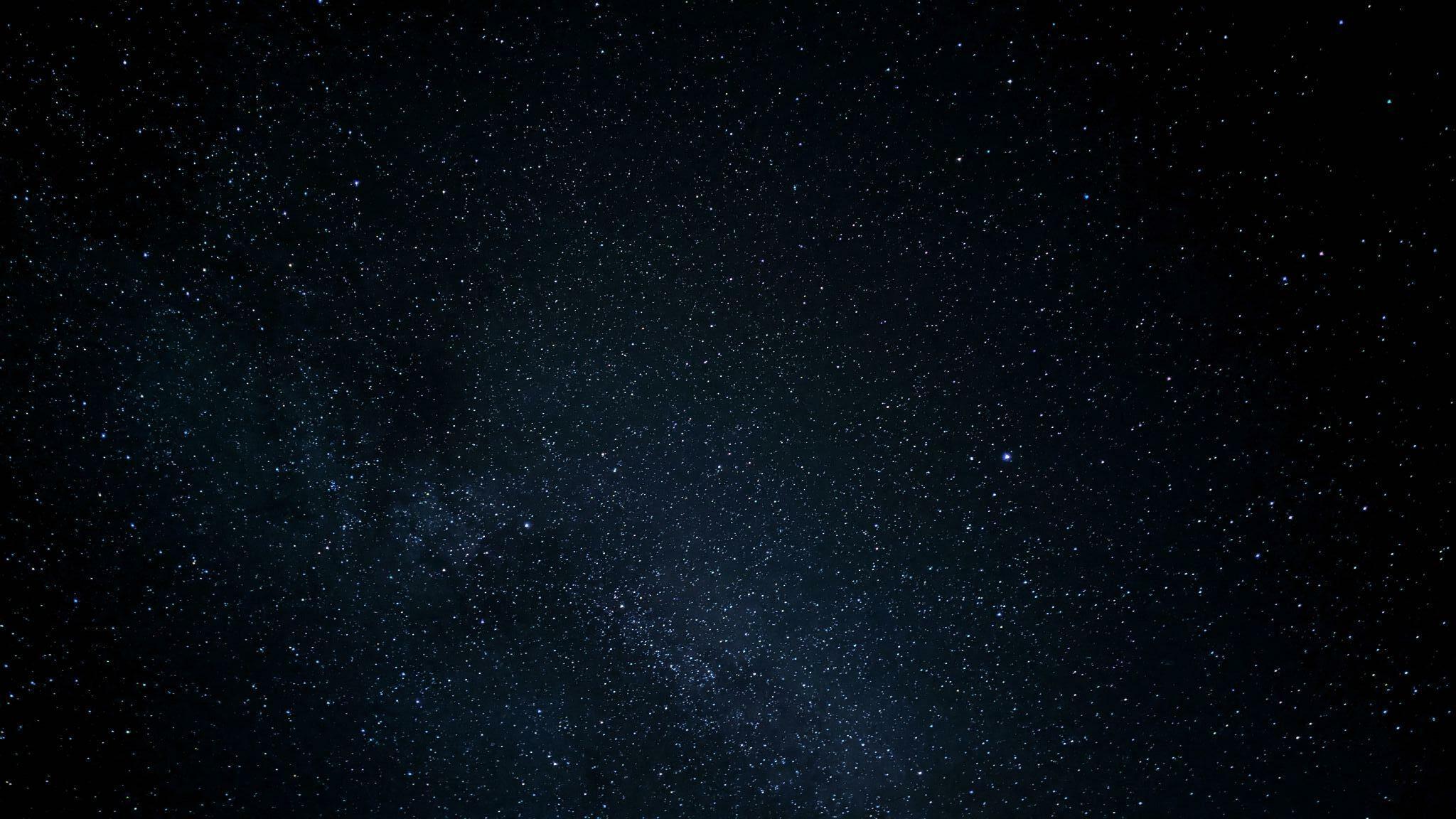
OVERVIEW
The following studio project presents the Sports and Recreation orbital space modules configuration which is part of an orbital space habitat system to be aggregated as a complete sufficient habitat. The development of the project begins with the study of the forms of exercise, recreation, the use of the interior spaces. Consequently, human ergonomics about microgravity was investigated to develop interior spaces suitable transport from the earth and later construction in space.
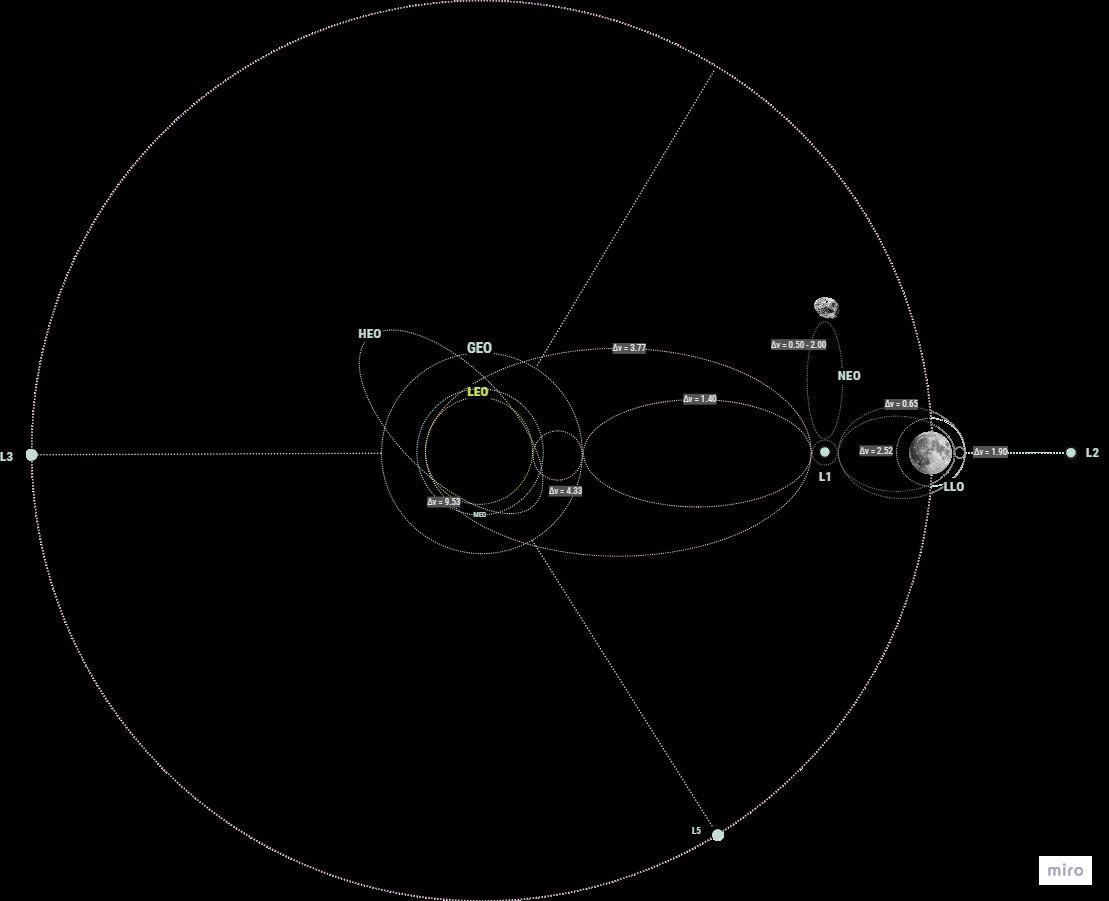
habitat proposal on the Lower Earth Orbit. The project is based on a platonic shape given by the infrastructure orbit group, to create a modular recreation, and sports that are currently practised inside the International Space Station (ISS). Then, alternative ways were studied to extend for the physics of the environment. Finally, the modules’ structural functioning, composition, and design were developed for their possible
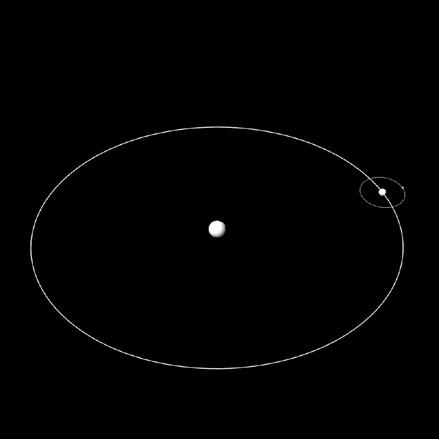
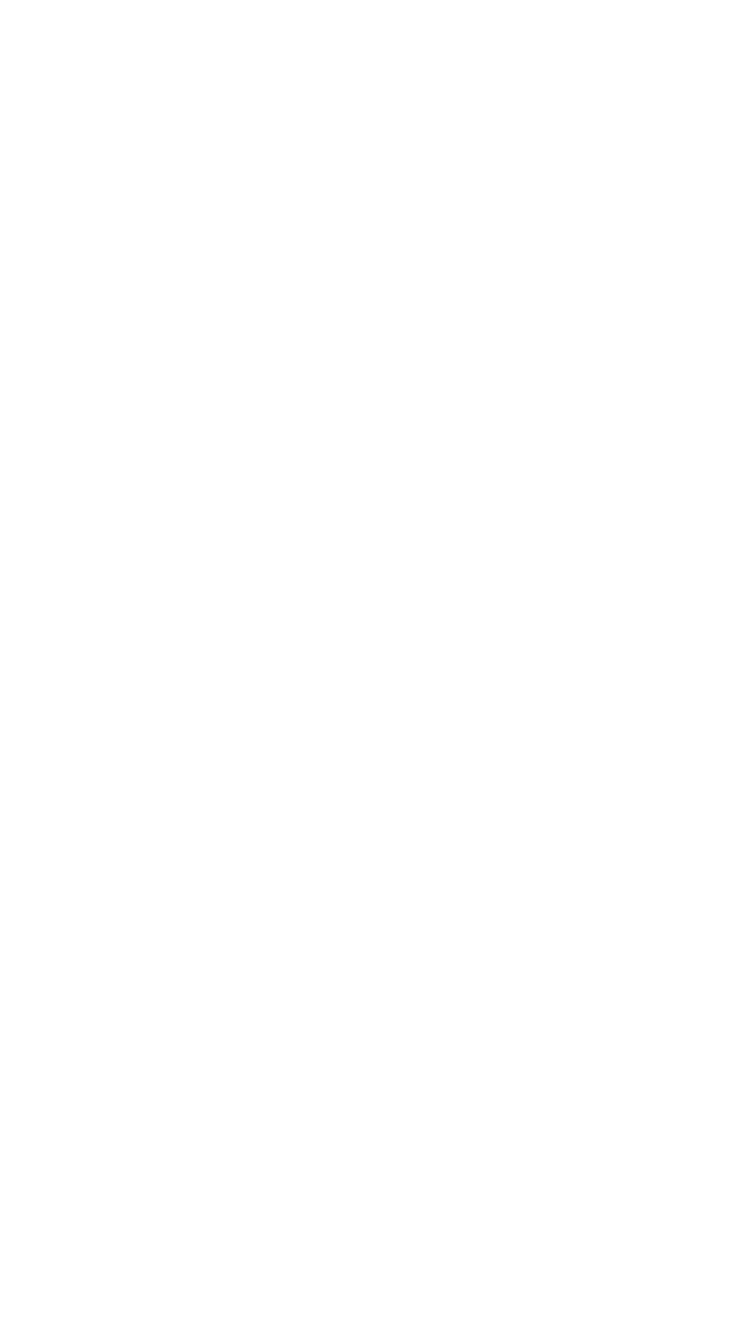
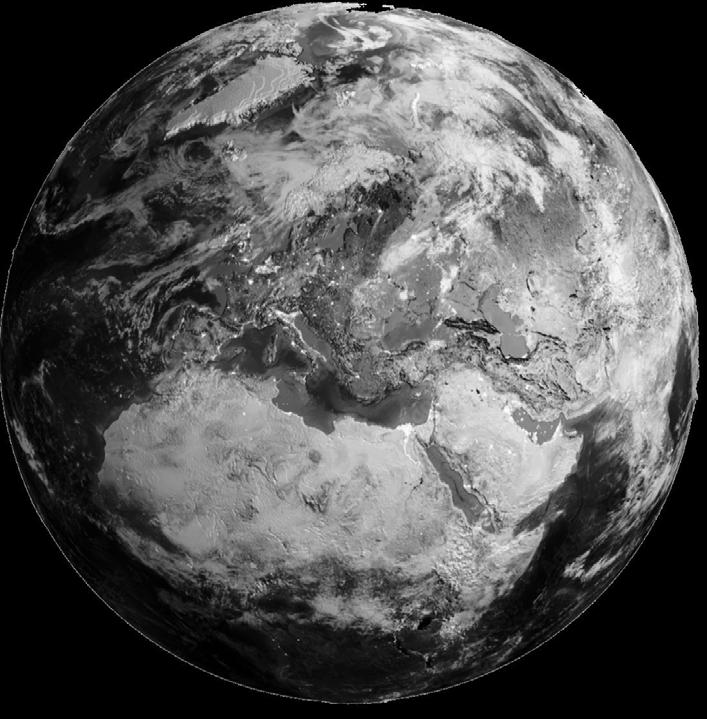
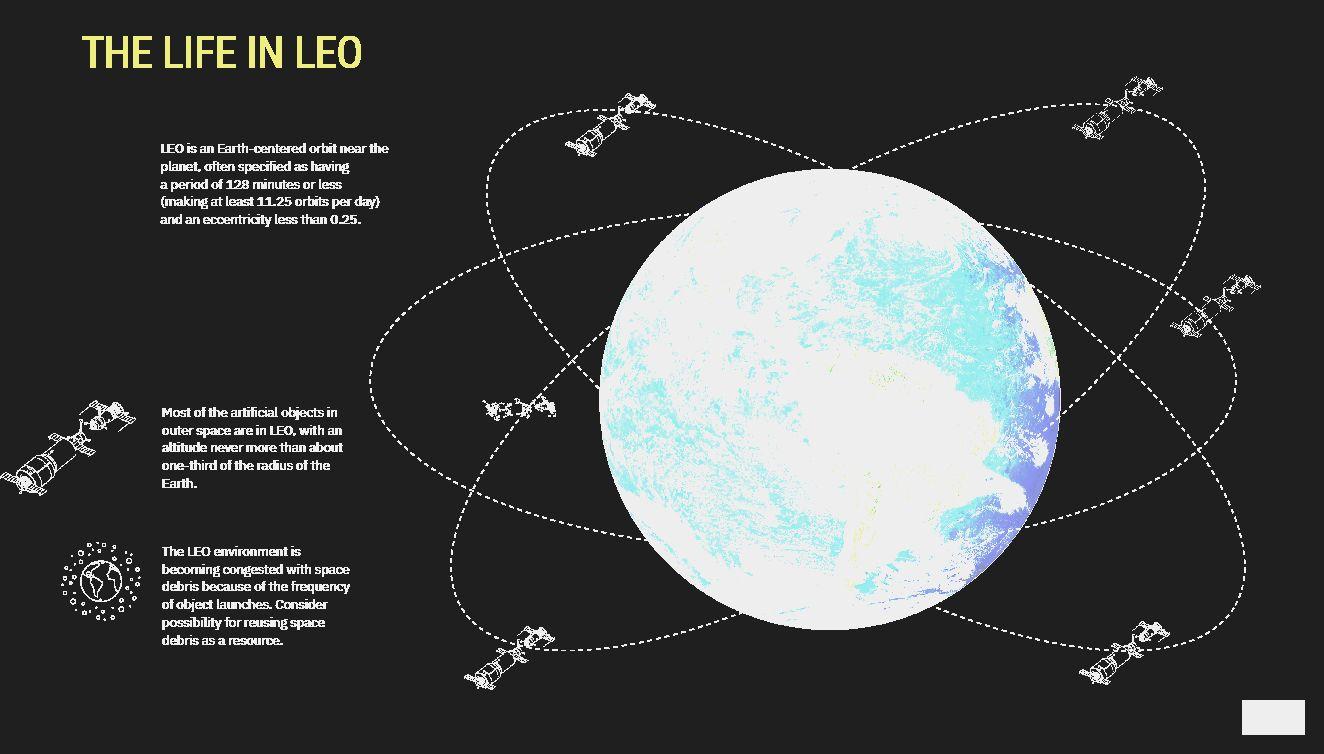
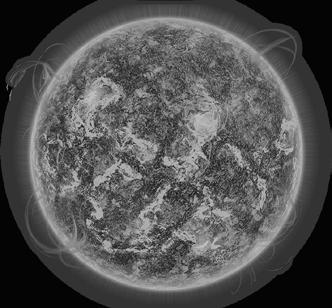
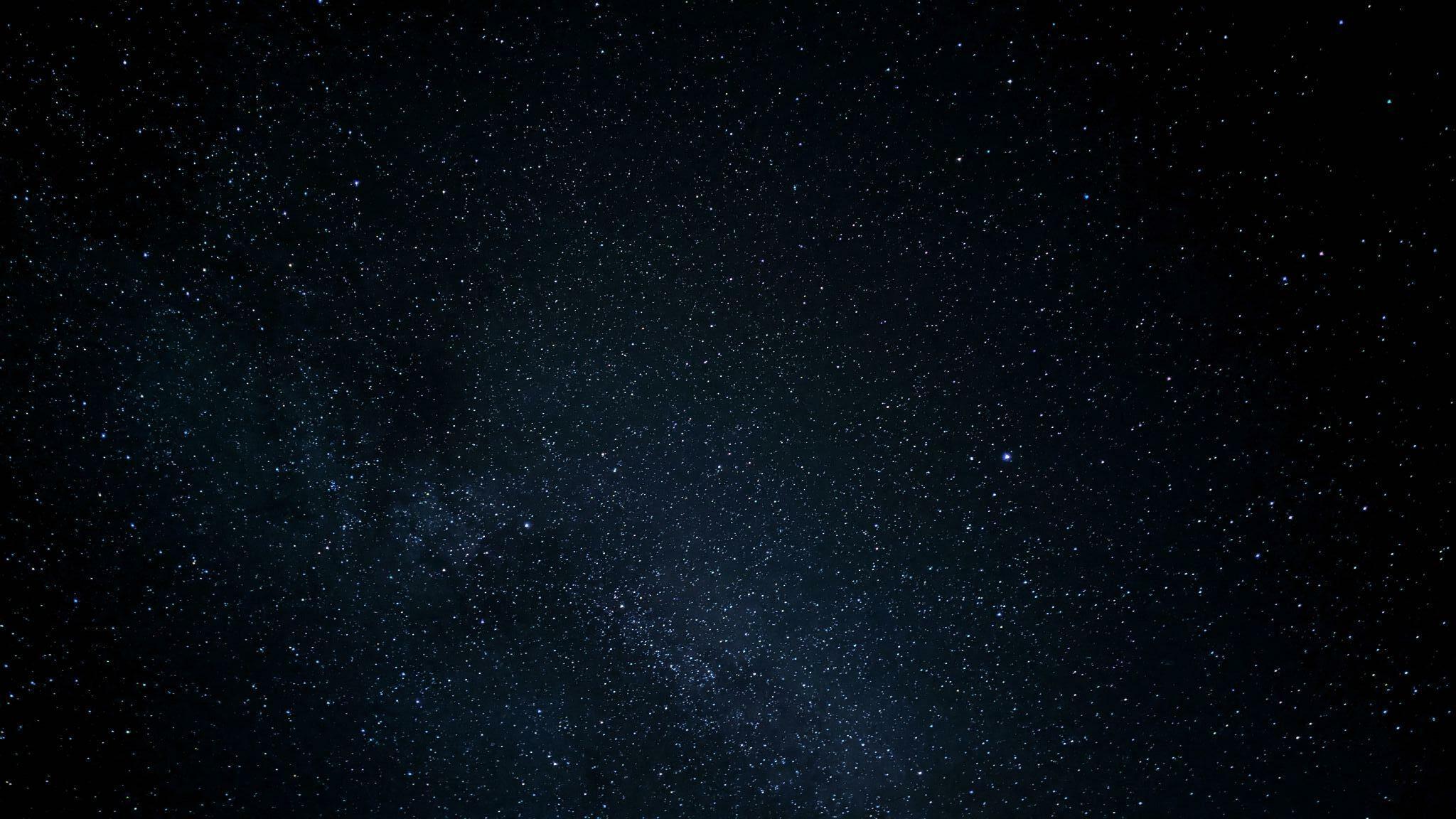
The objective of this workflow in the Orbit Space Habitat is to present and evaluate the collaborative workflow that was followed during the course partners. The project has therefore a particular focus on this digital collaboration across design groups, software platforms, time zones and file formats. industry and in the architectural trade. Two tools that have changed the process of going from implicit to explicit representation, refining the design
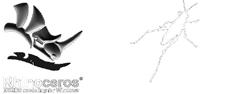
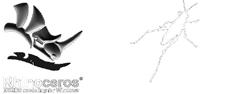
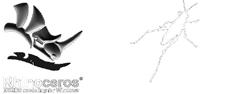
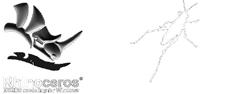
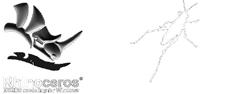
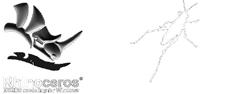
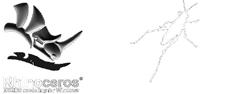
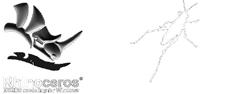
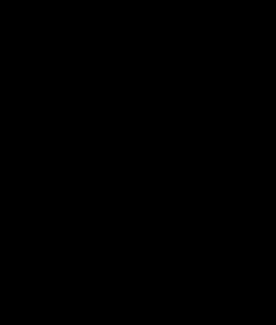
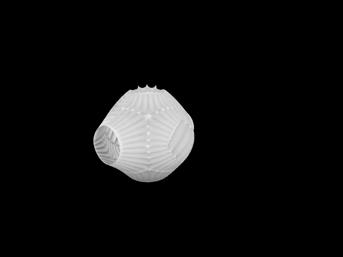






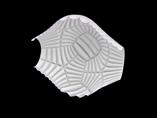
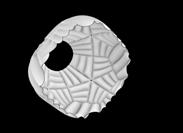

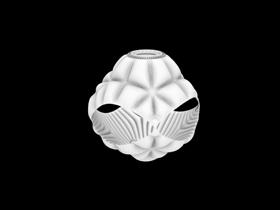
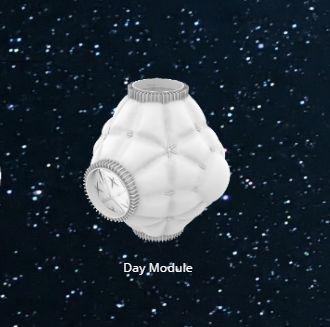
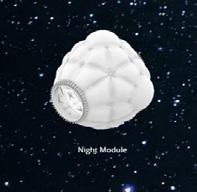
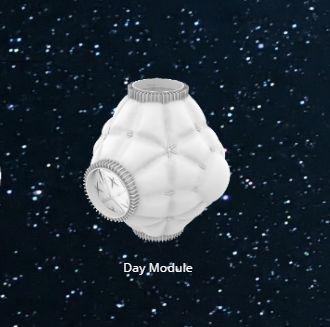
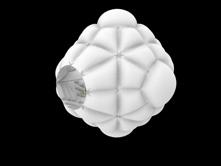
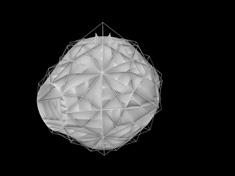
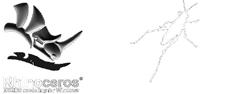
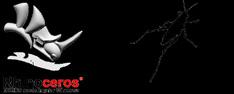
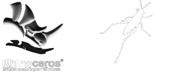
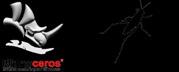
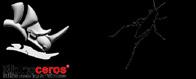
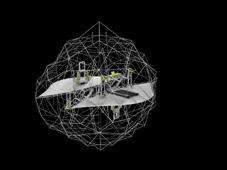
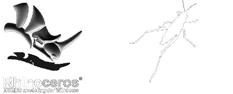
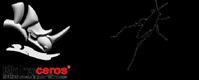
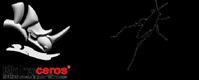





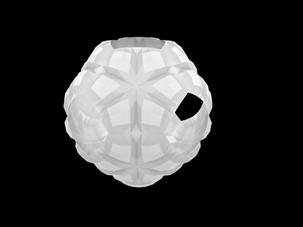
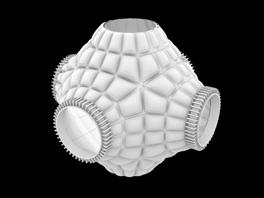
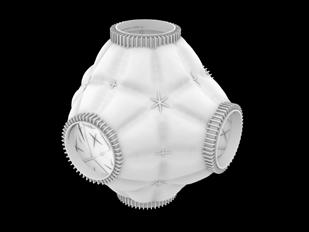

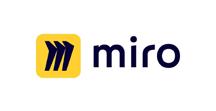
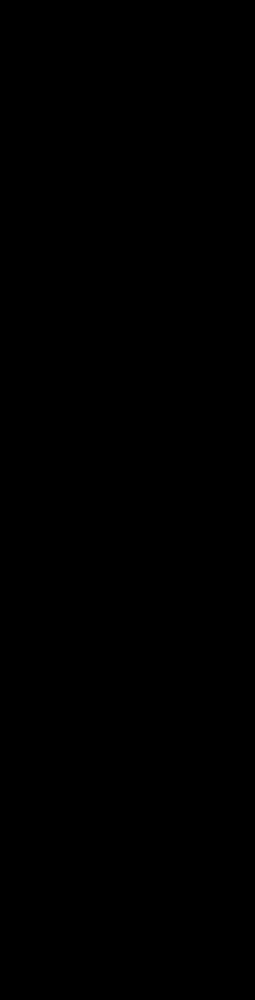




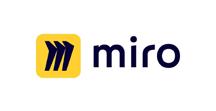


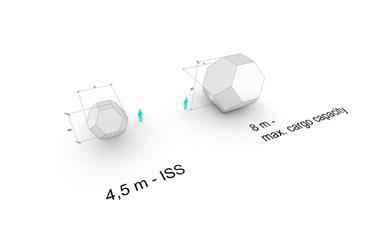
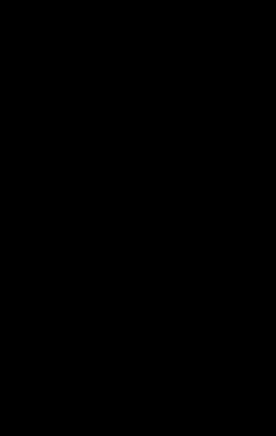

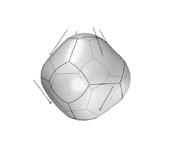
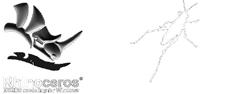
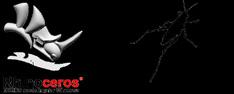
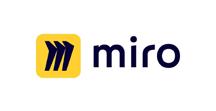

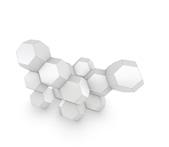
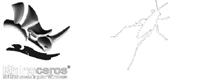
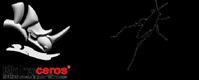
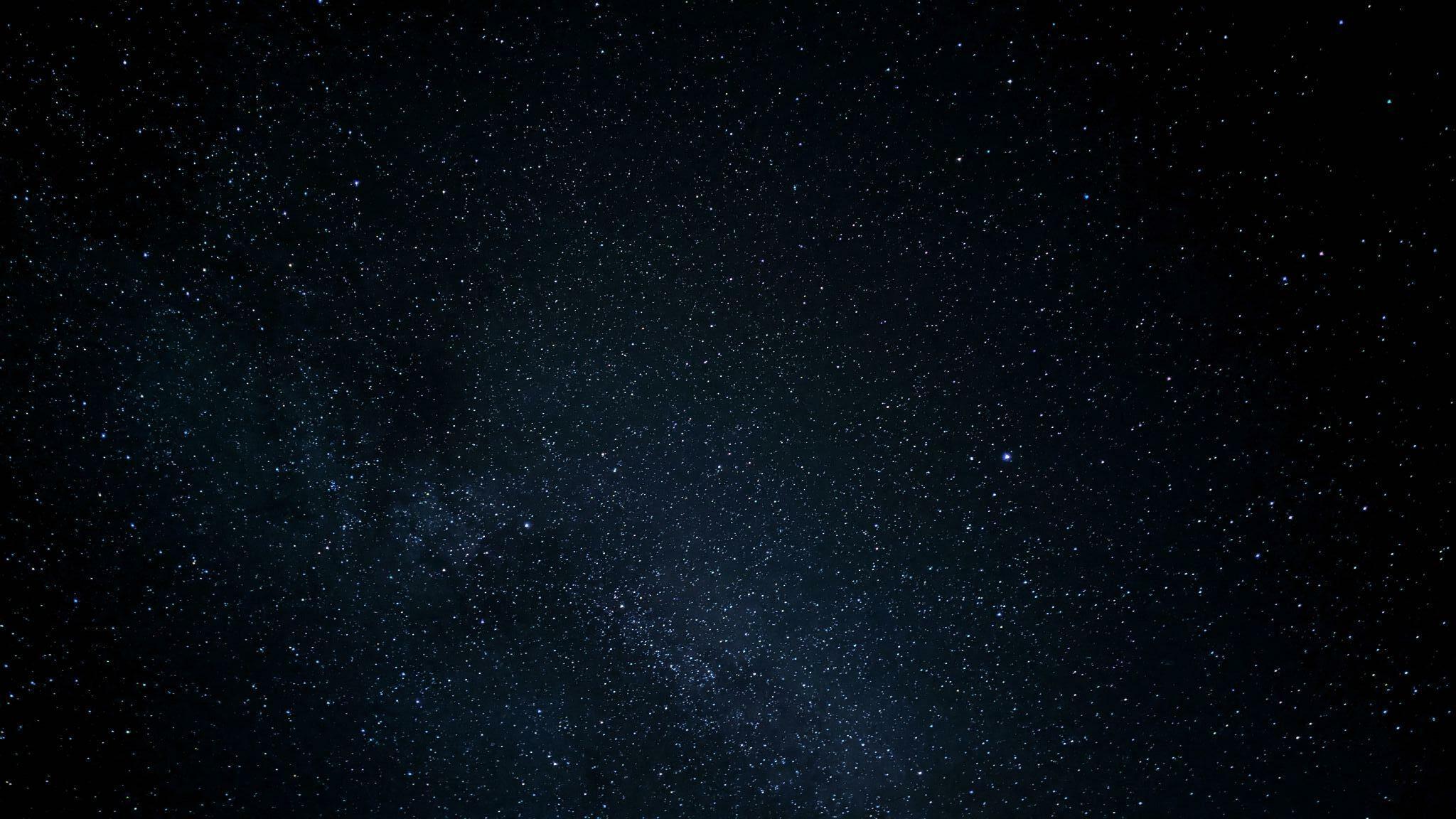
course of the module, in the search of effective patterns and strategies that enables successful data sharing and interoperability between project formats. At the core of the collaborative process are Speckle and BIM, two softwares that are currently at the center of the discussions in the AEC design through continuous input from engineering, environmental, cost and fabrication constraints.
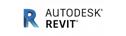

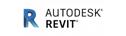




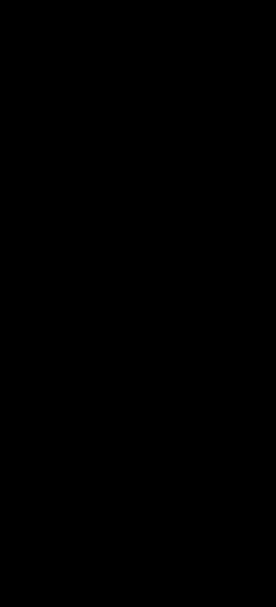
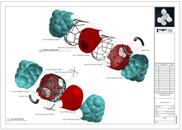
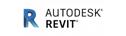

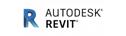


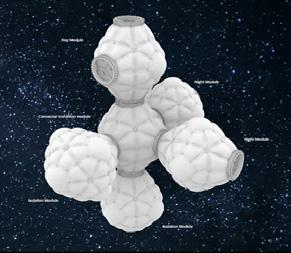
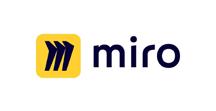
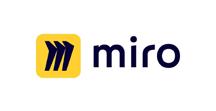
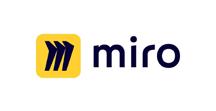
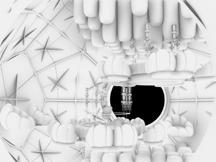
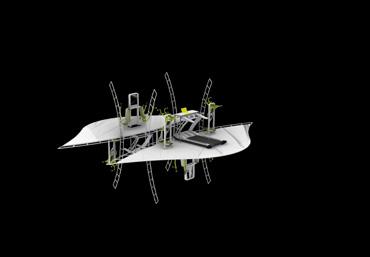

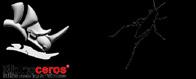
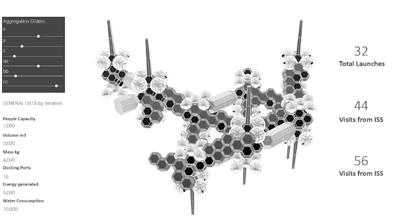
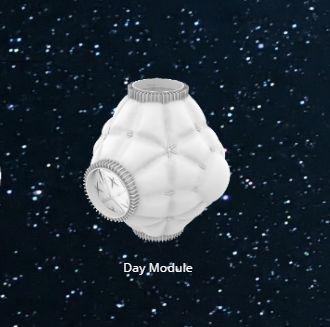
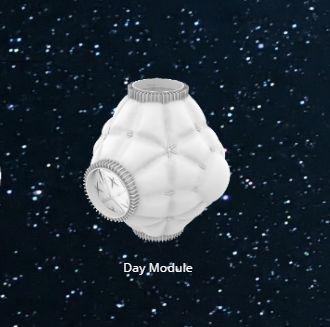
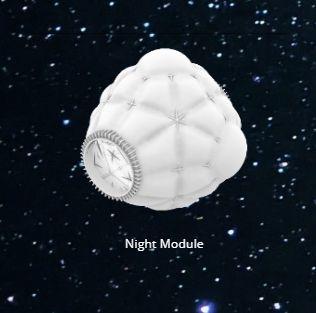
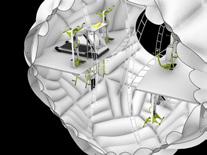
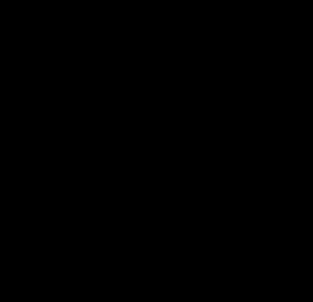
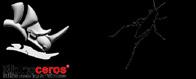
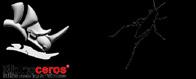
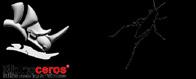

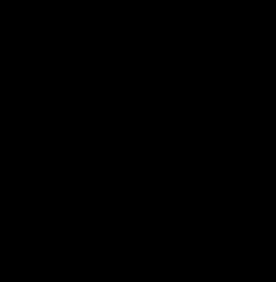

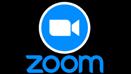
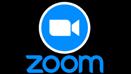
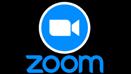
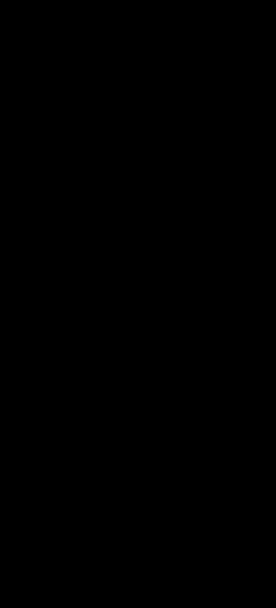
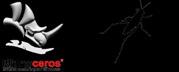
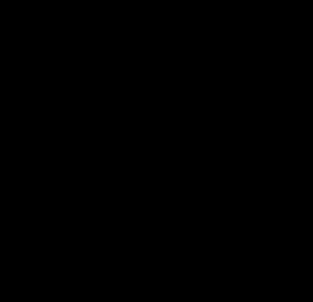
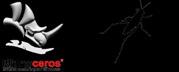
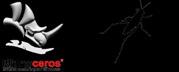
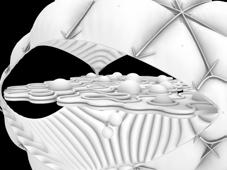
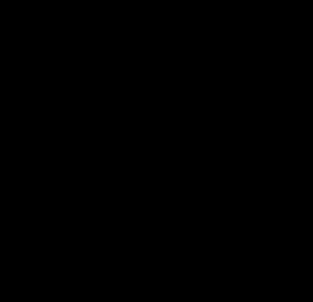
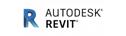



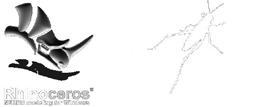
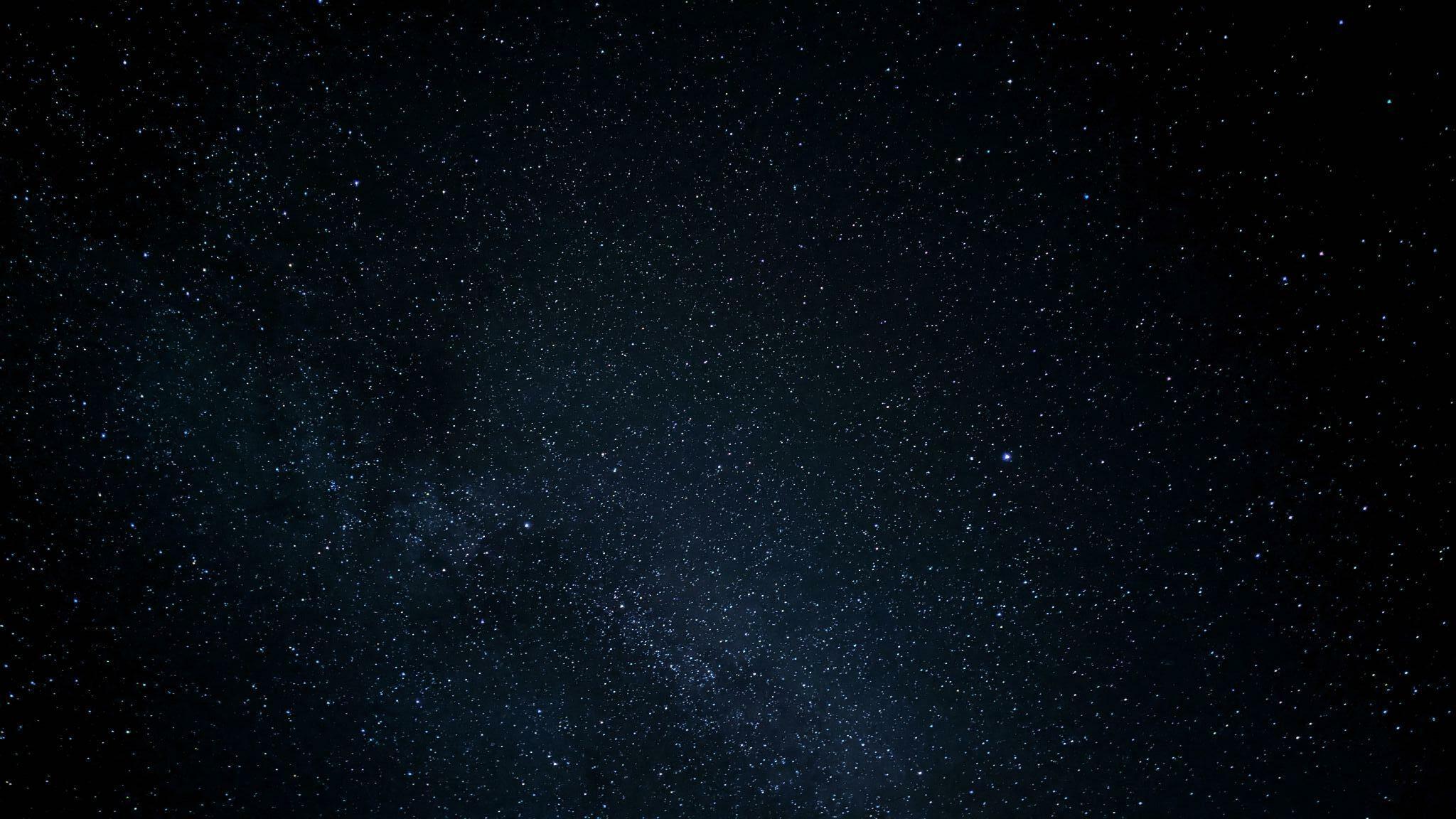
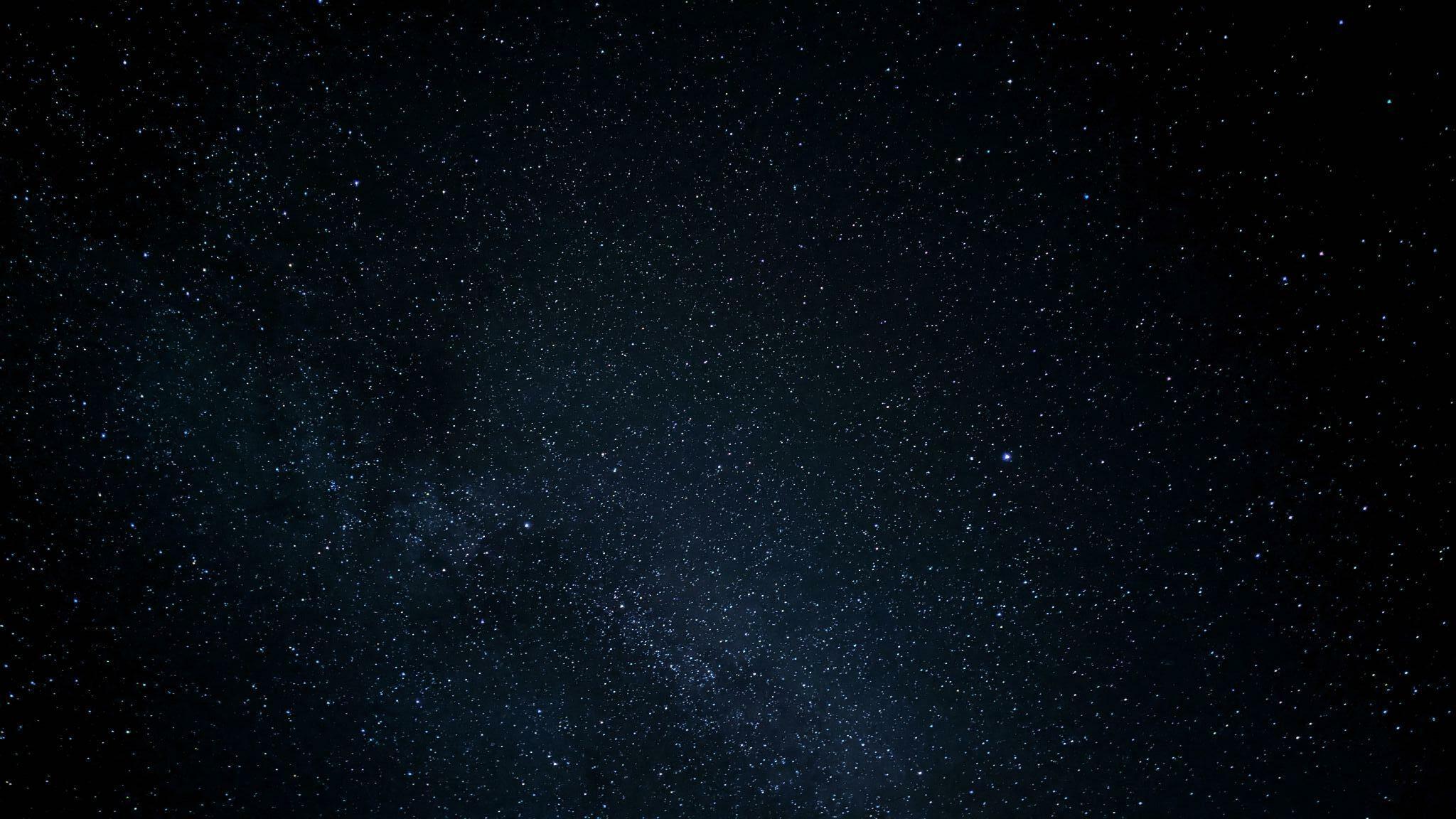
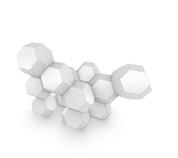
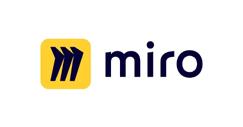
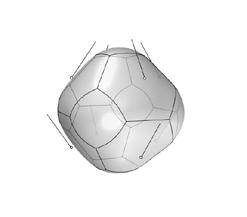

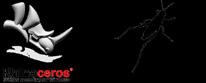
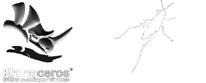
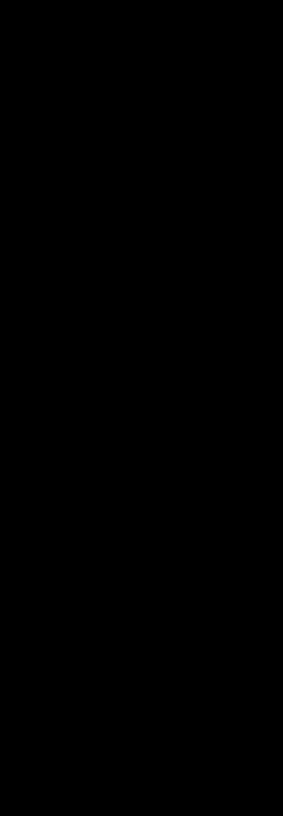


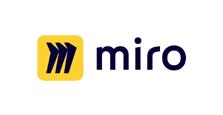
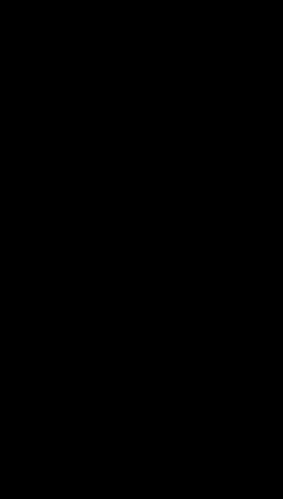
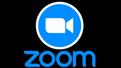
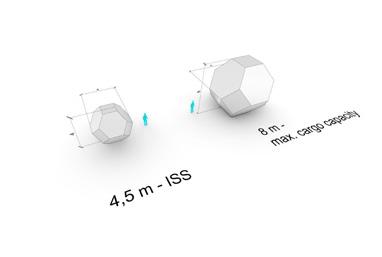

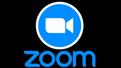
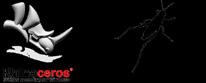
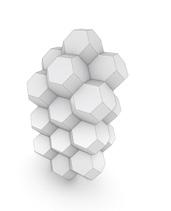
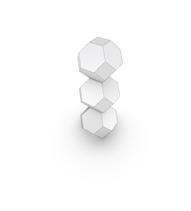
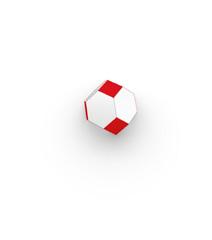
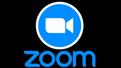
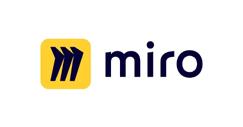
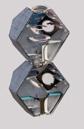
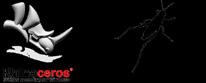
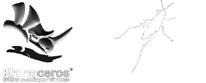


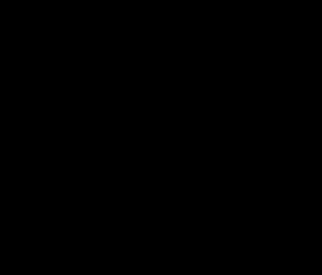
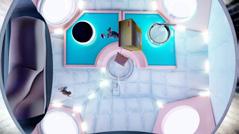
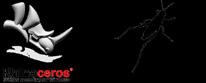
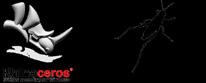
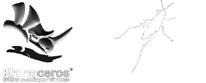

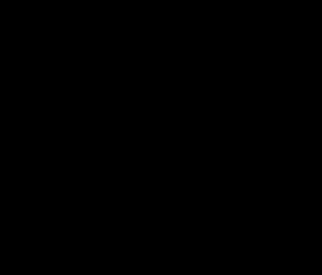

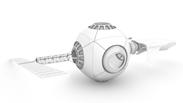
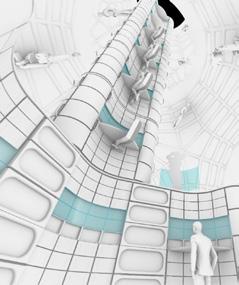

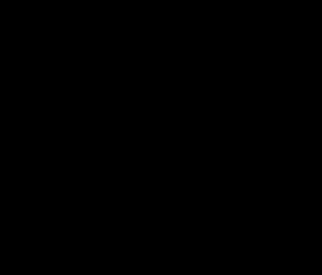

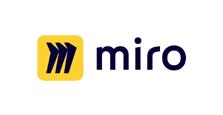
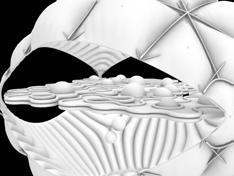
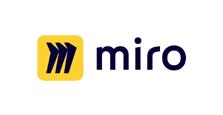
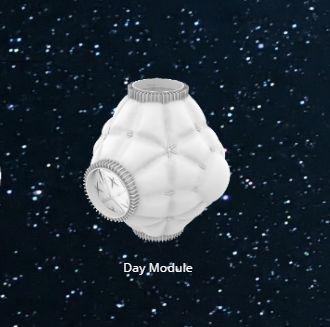

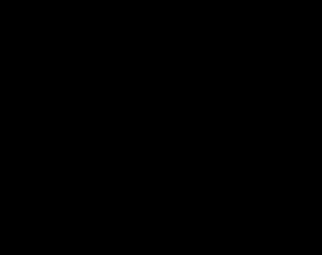

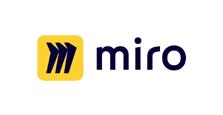
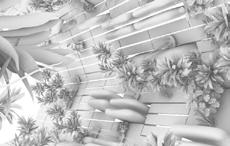
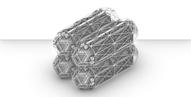


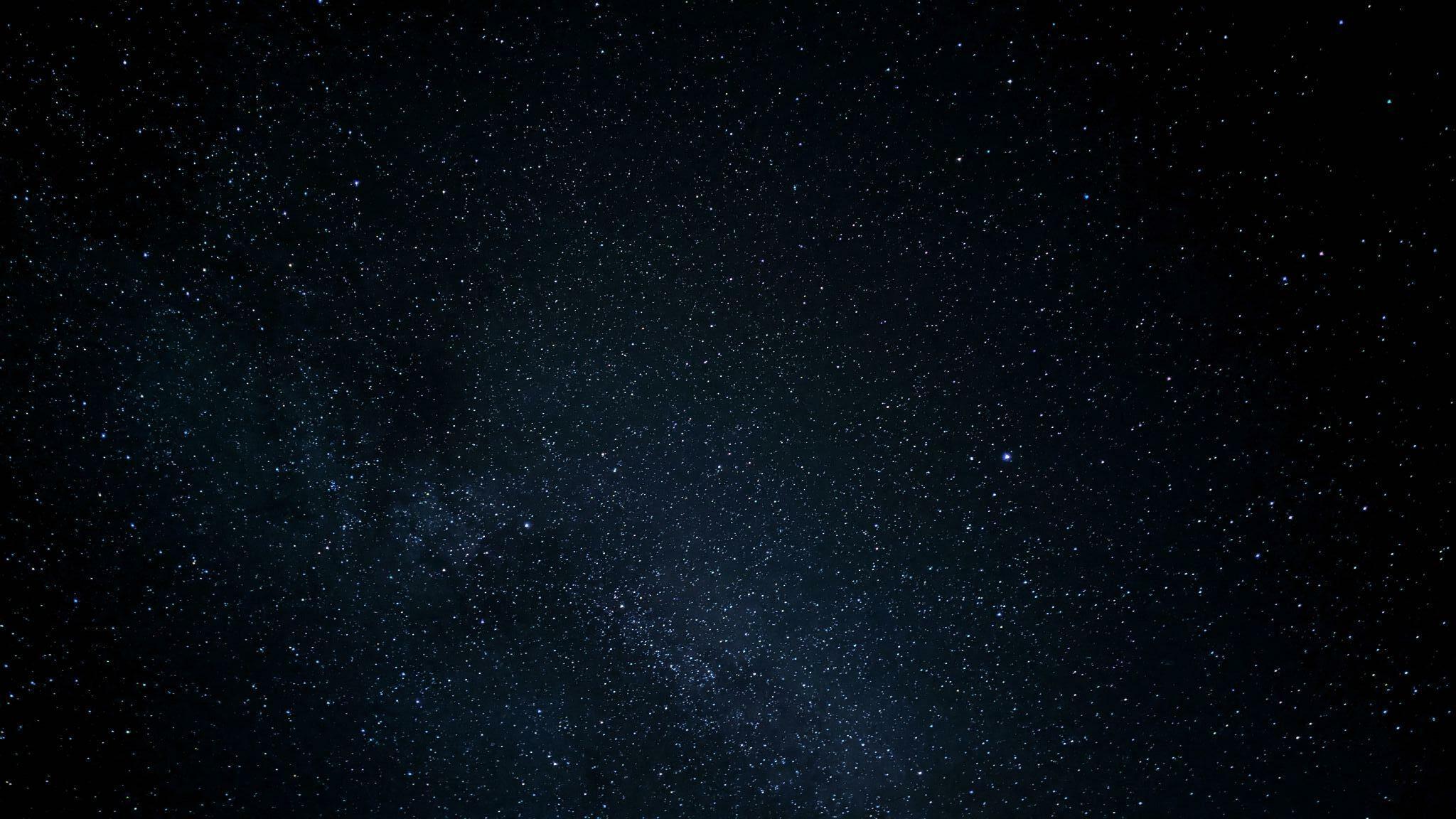
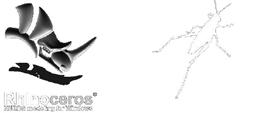

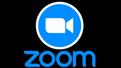
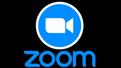
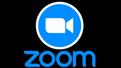
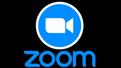


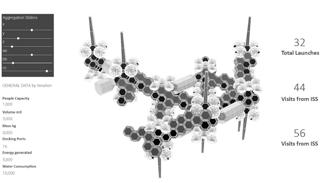

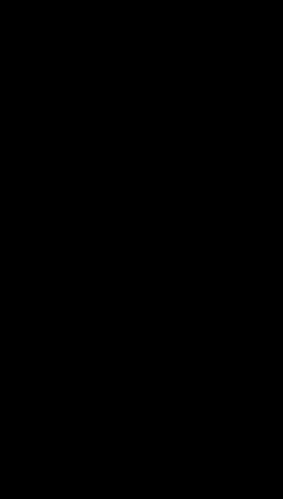













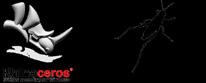

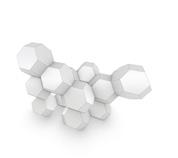
NEBULAE
SLEEP WORK EAT PARTY REPEAT
MODULE FUNCTION PROGRAMME
The system presents the connection between the research and the functionality of each of the modules, and a schedule of activities that can be performed inside them. Similarly to what NASA does for Astronauts, we determined certain activities about time and use. Introducing now, SLEEP – WORK – EAT –PARTY – REPEAT
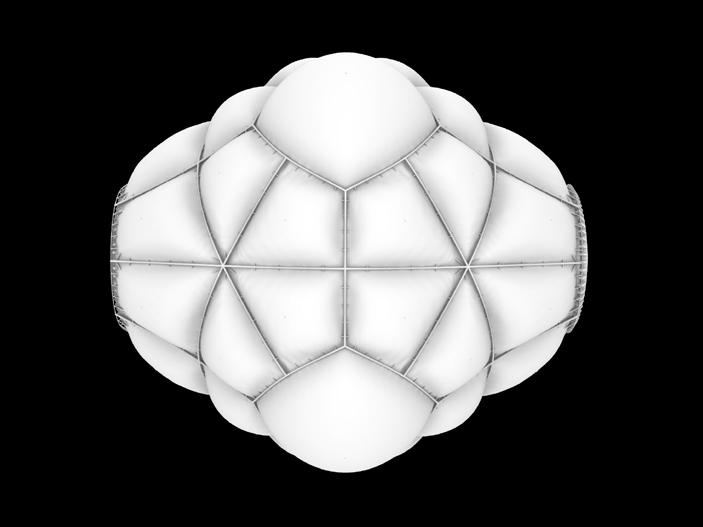
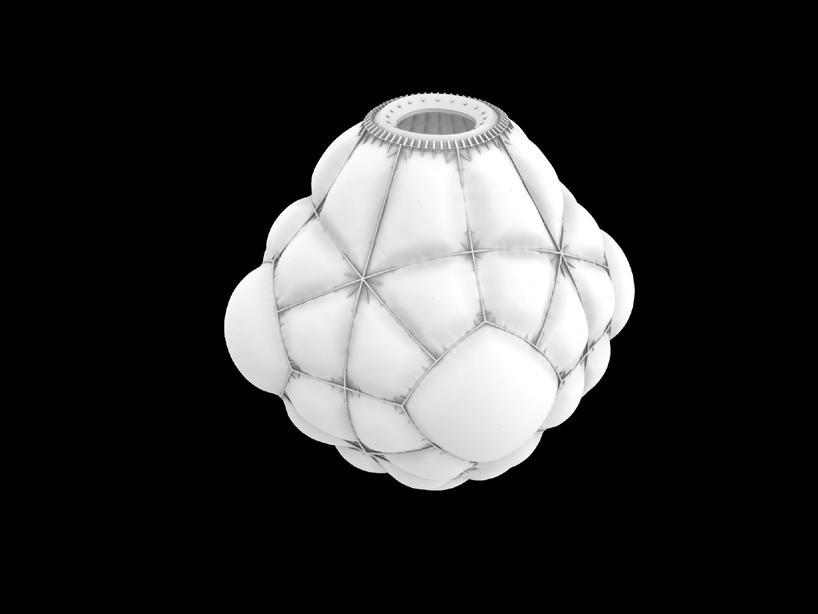
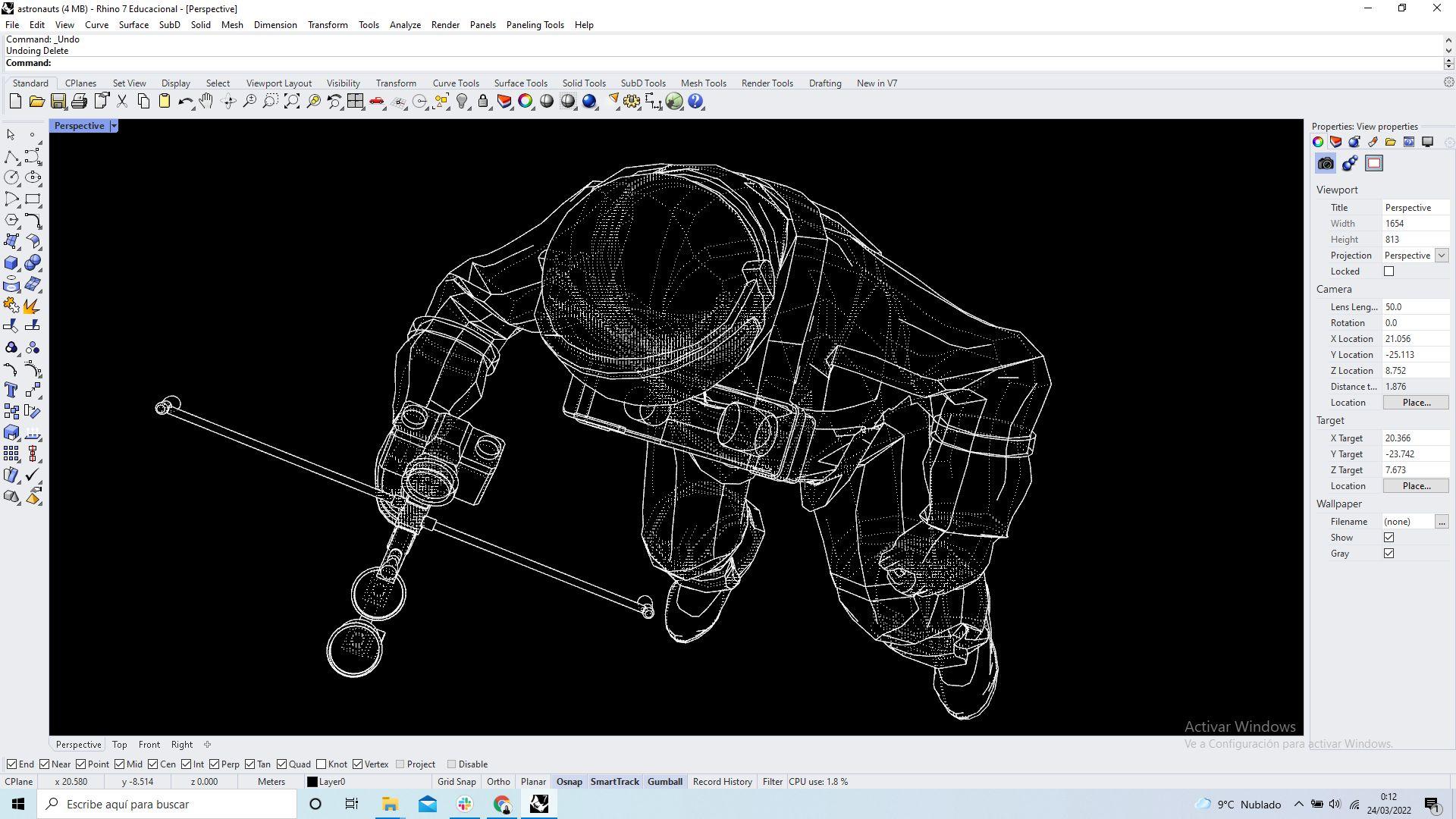
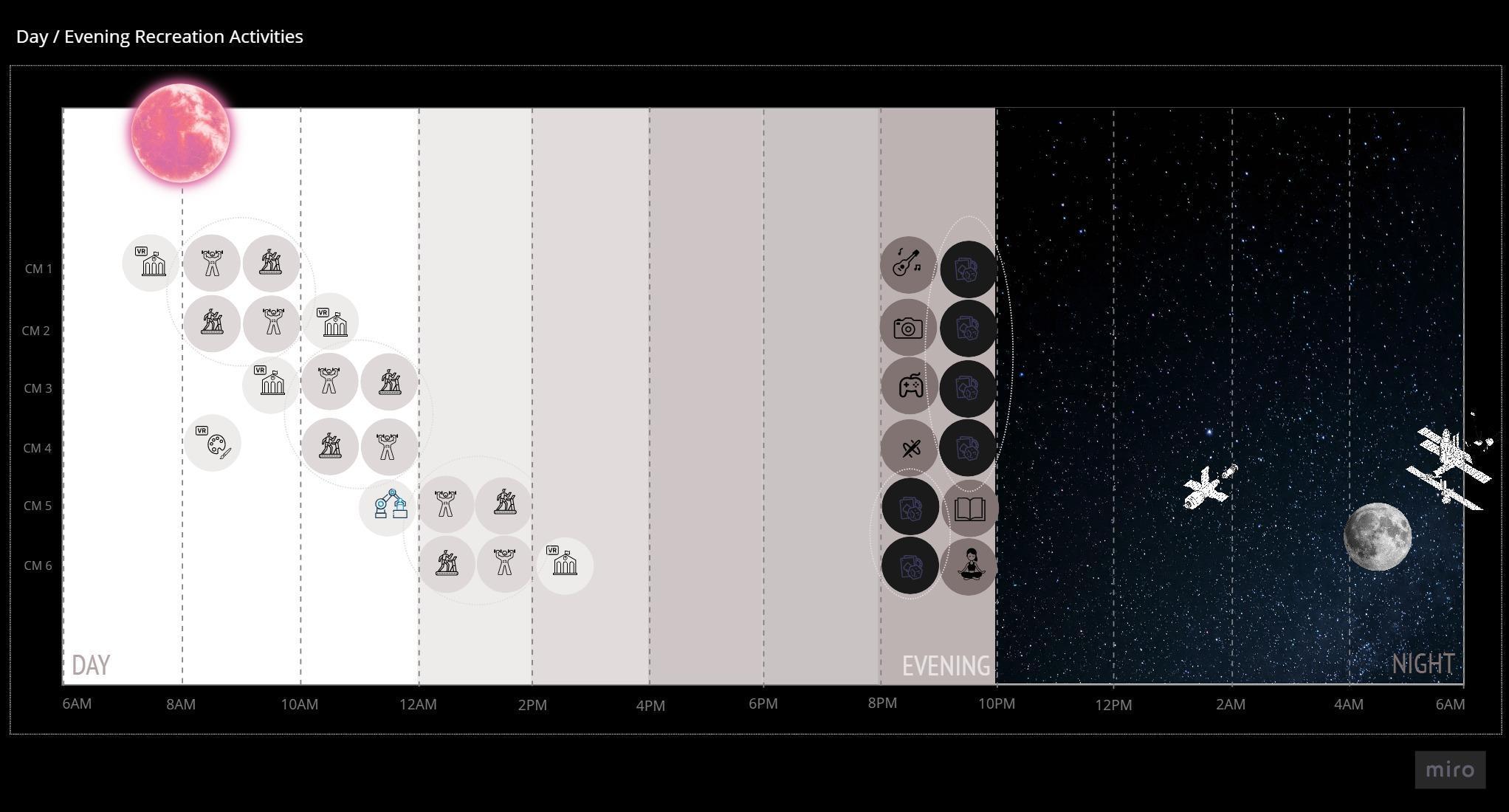
SPORTS YOGA CASINO VR GAMING
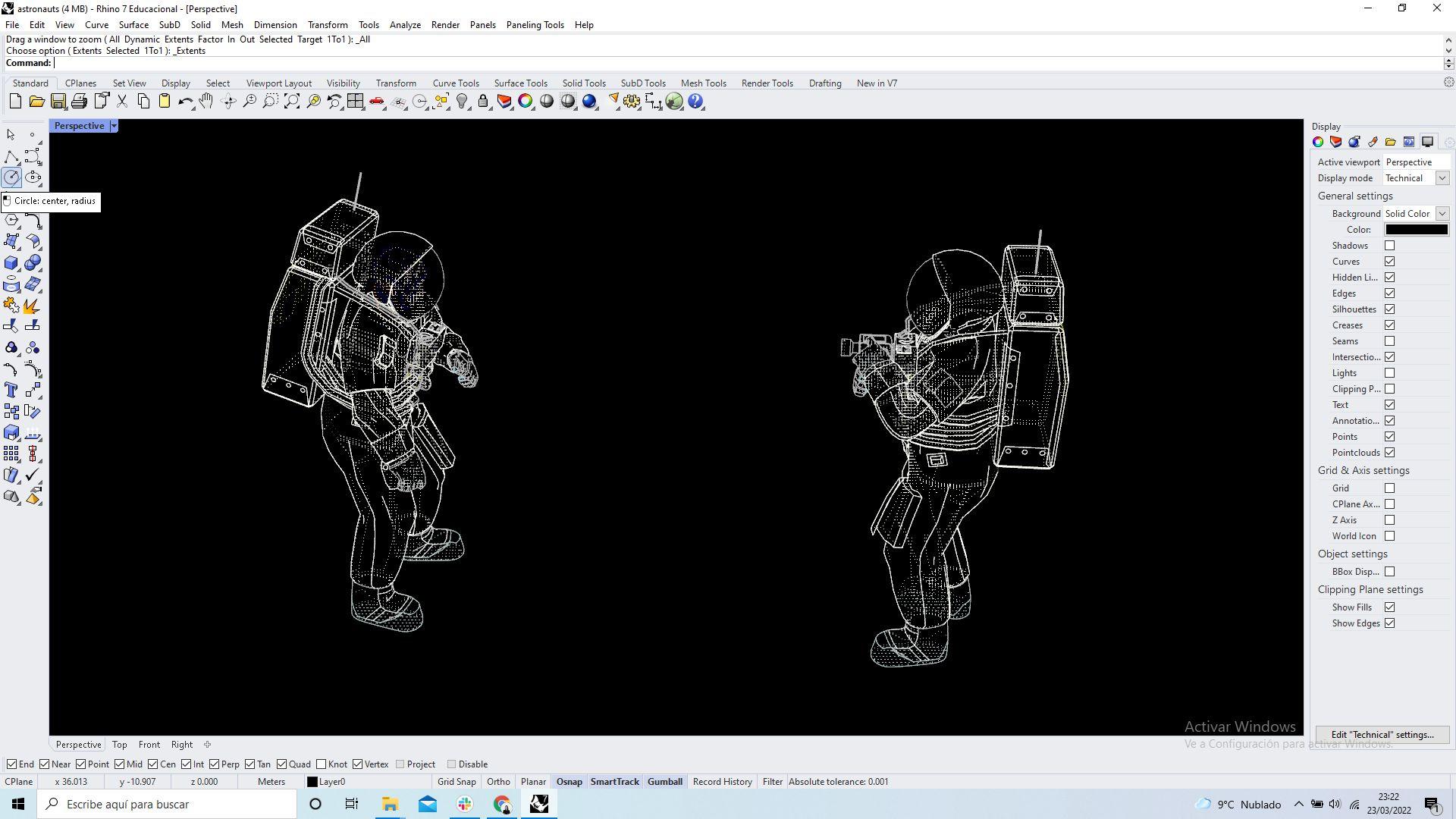
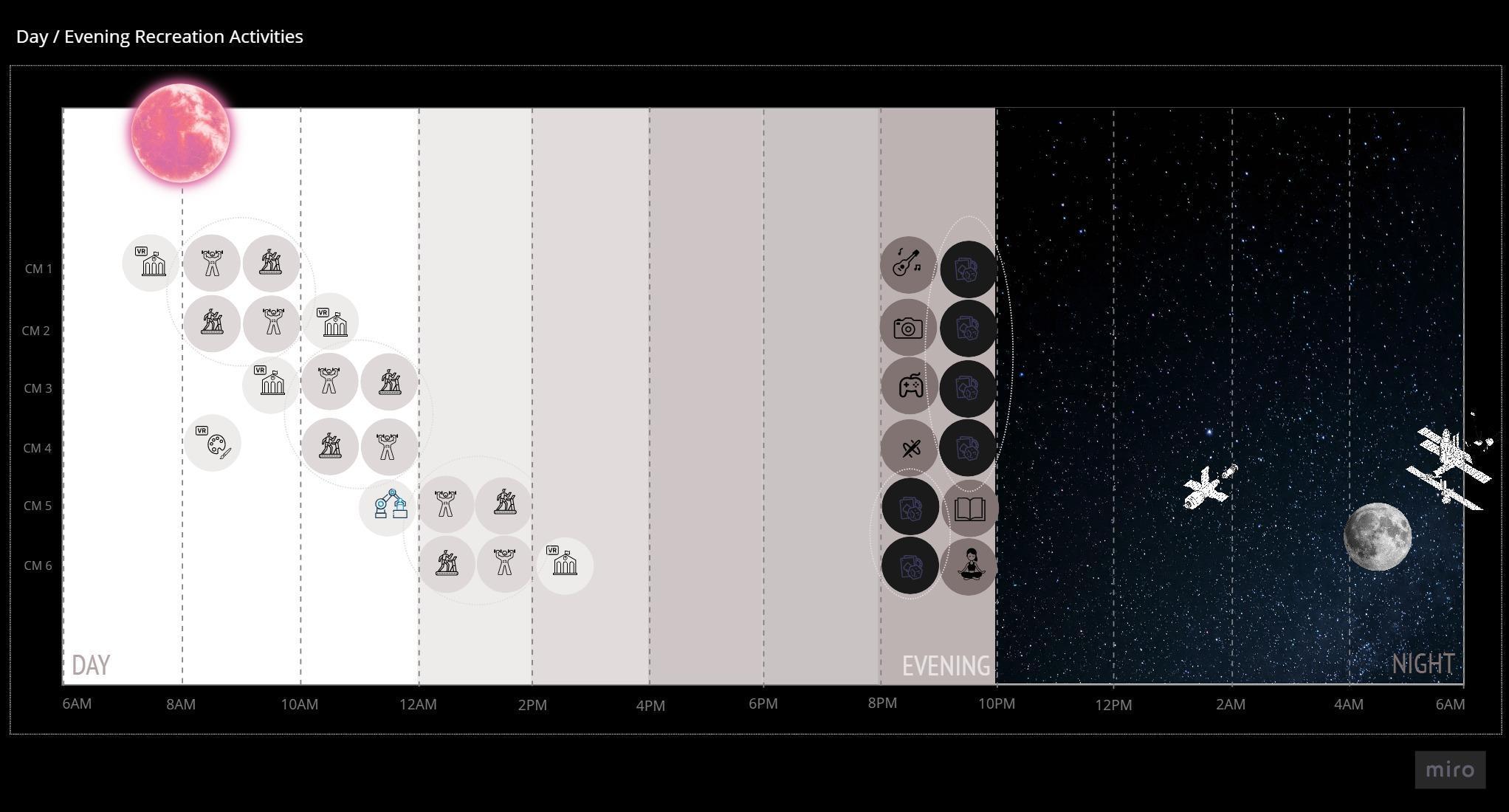
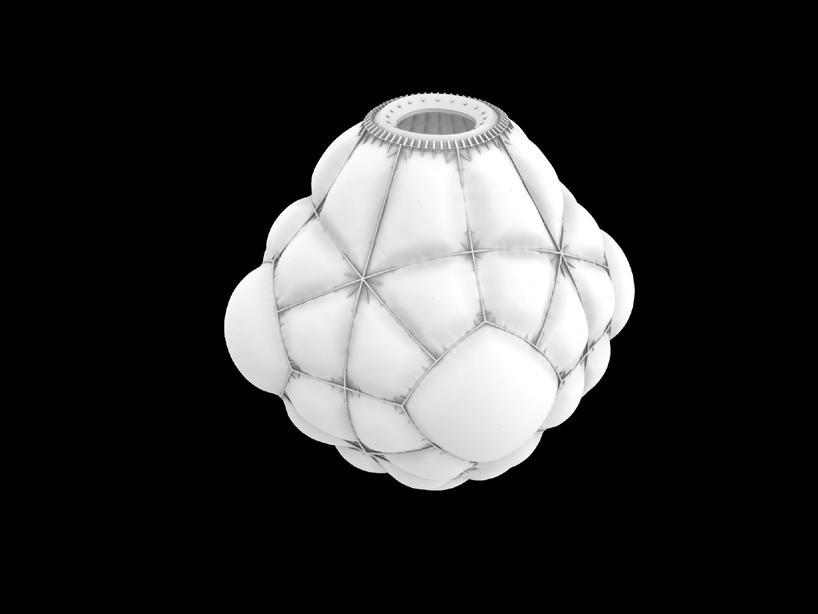
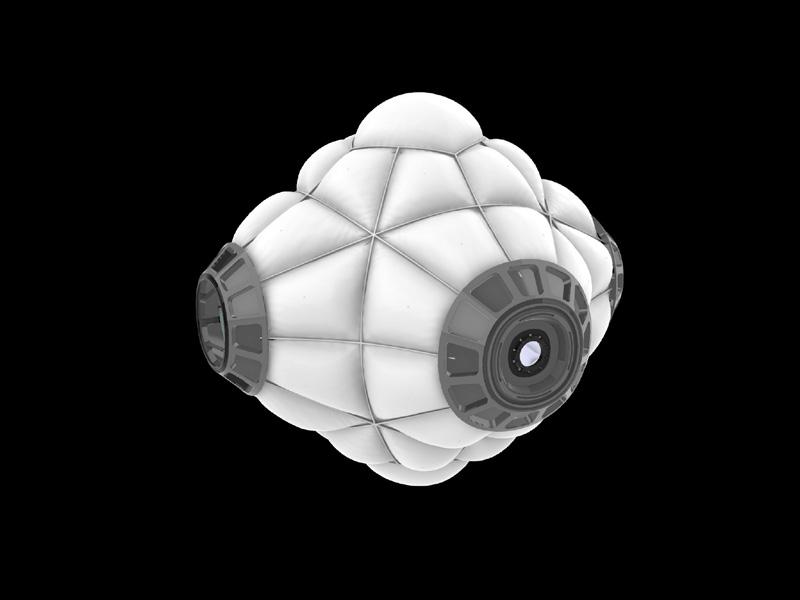
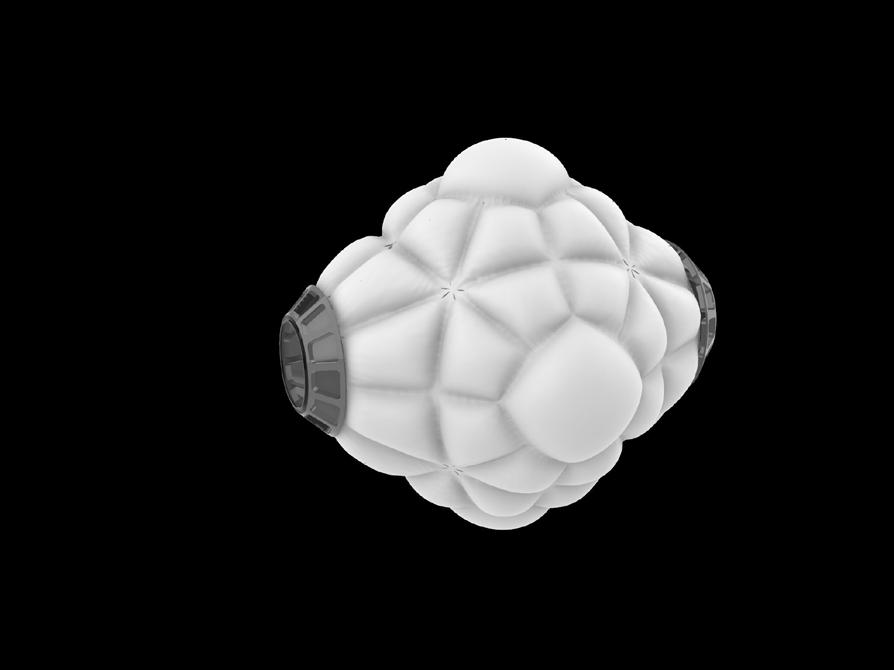
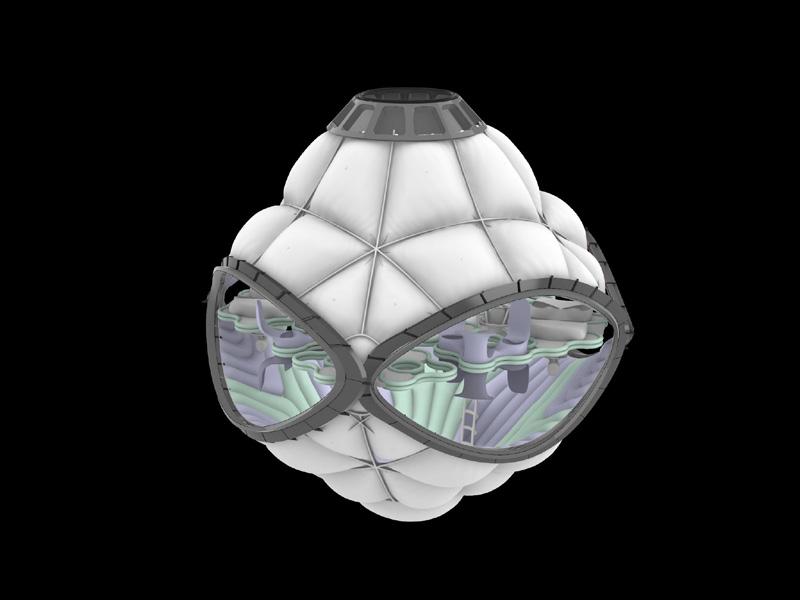
NEBULAE
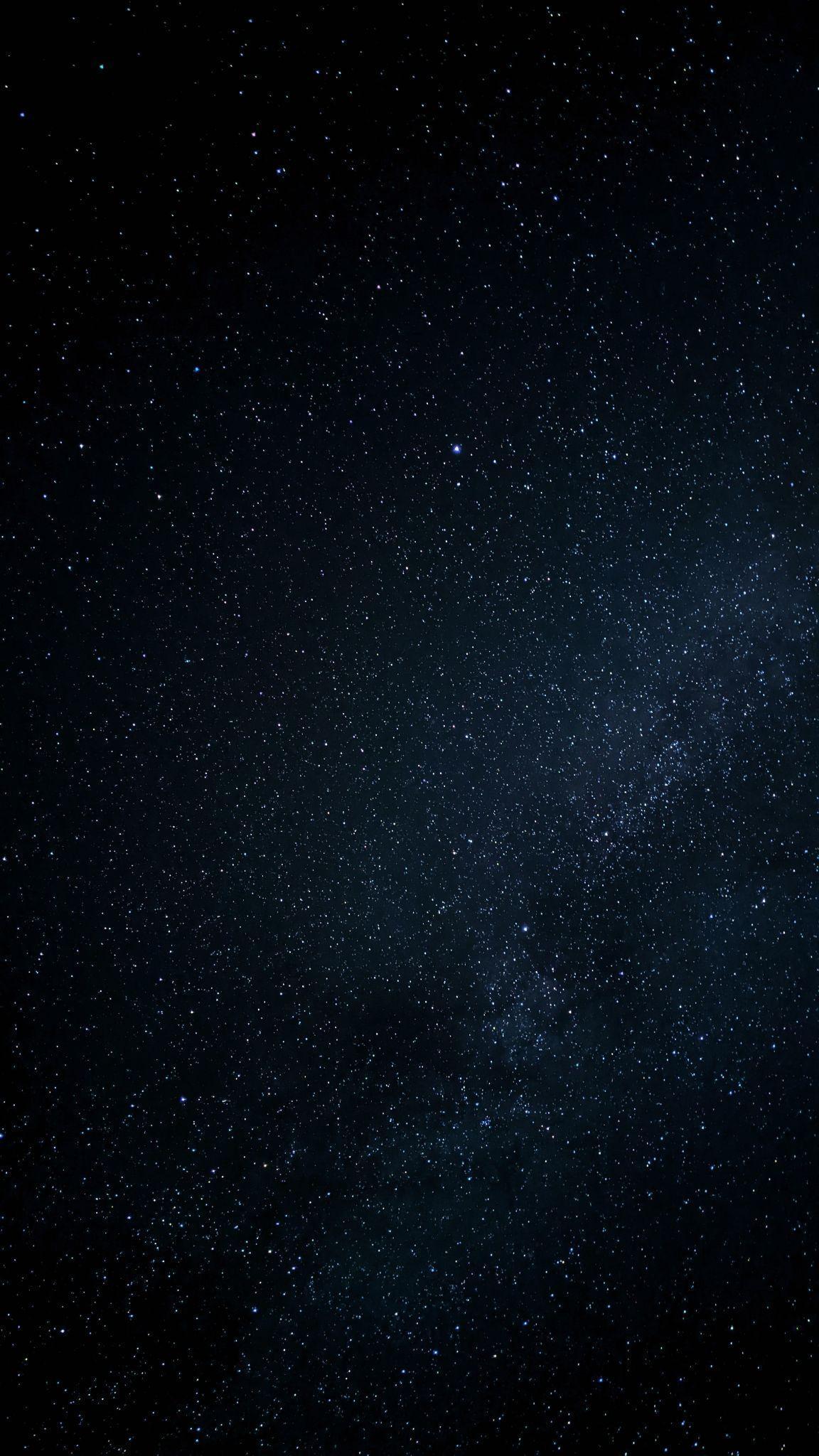
Nebulae: Orbital Space Habitat
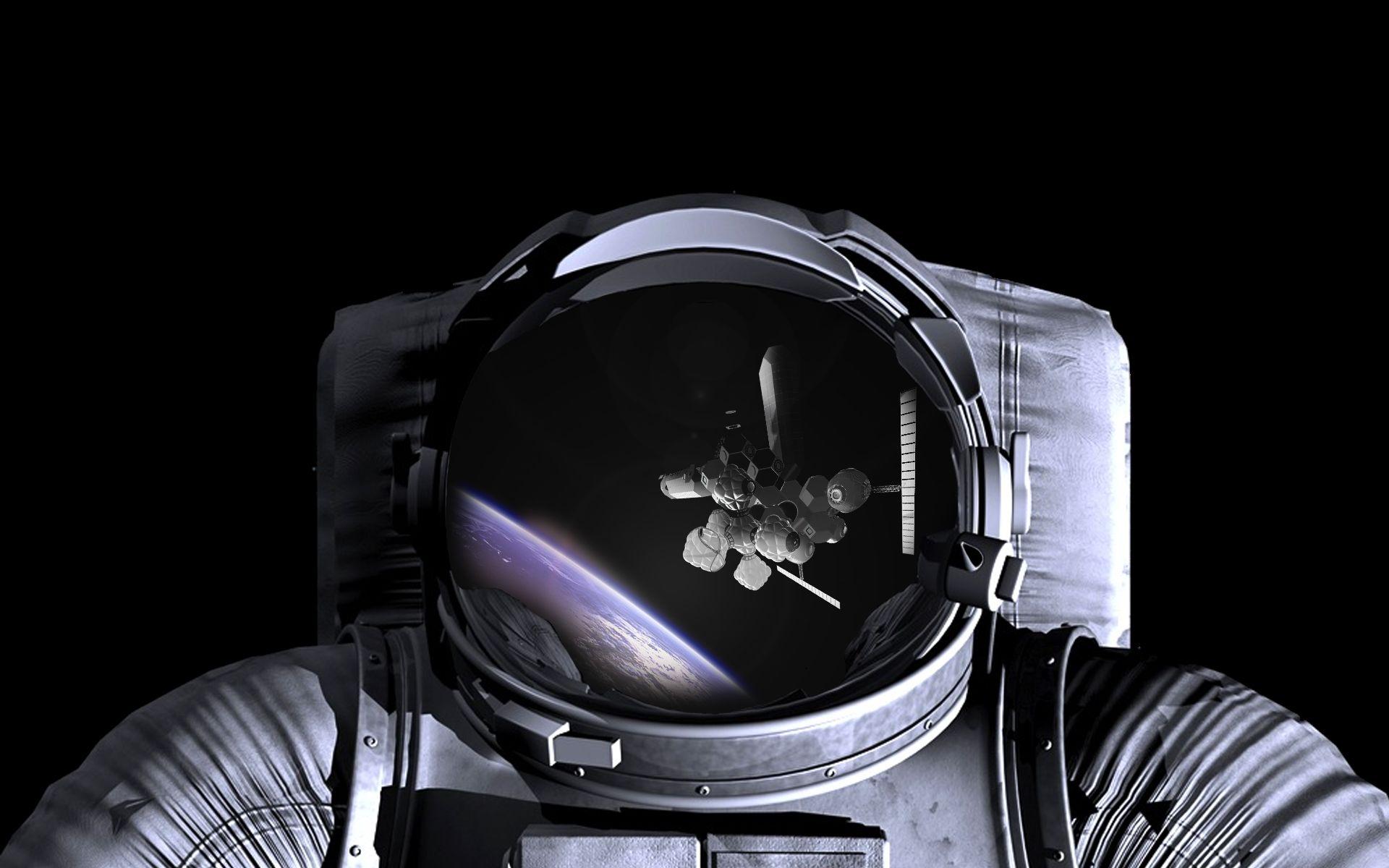
UNIT CONFIGURATION AND ASSEMBLY
The central module connects its neighbours by hatching its connecting faces. The tensegrity structure presents tension points within the structure to perfectly shape the origami unfolding.
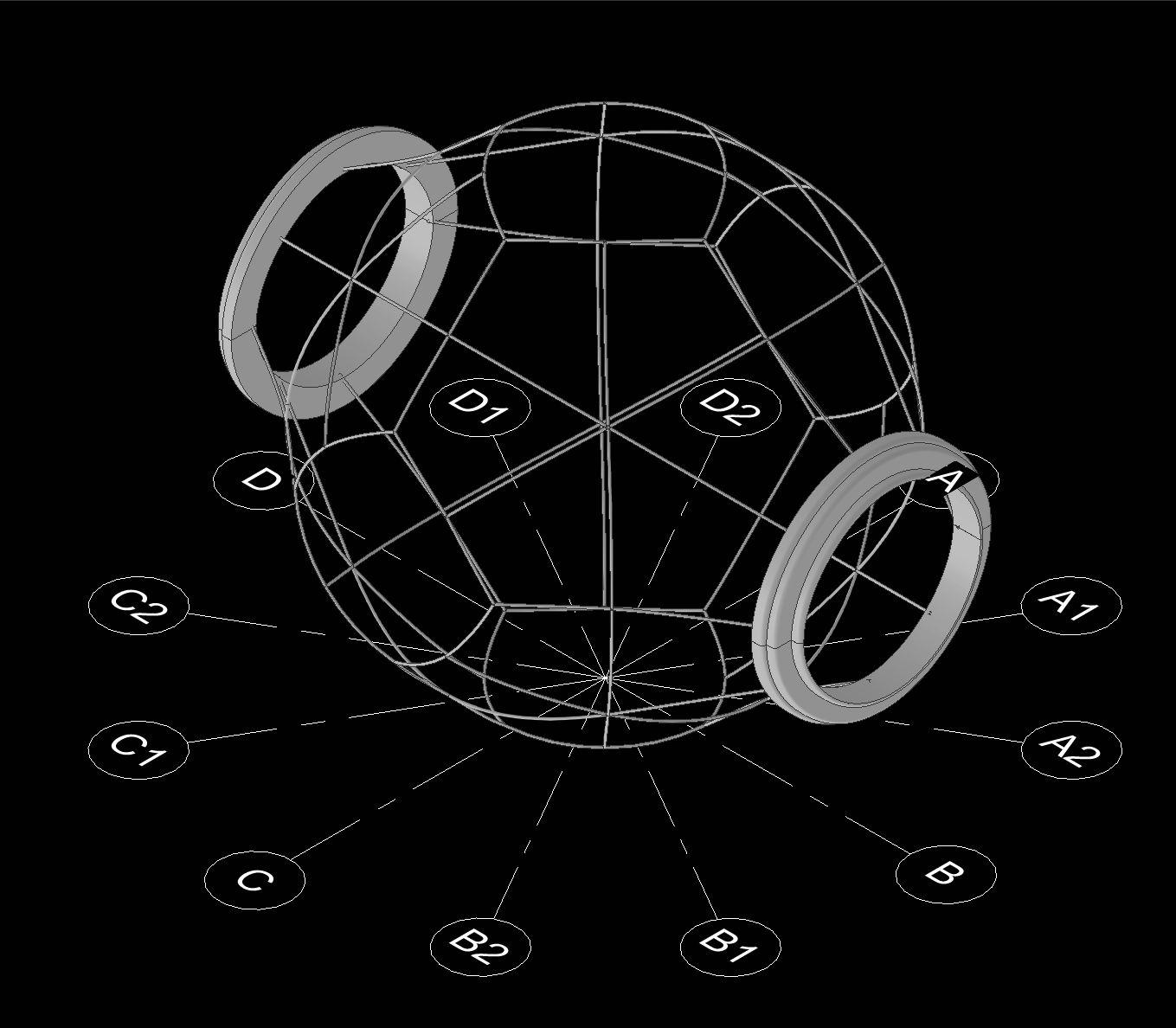
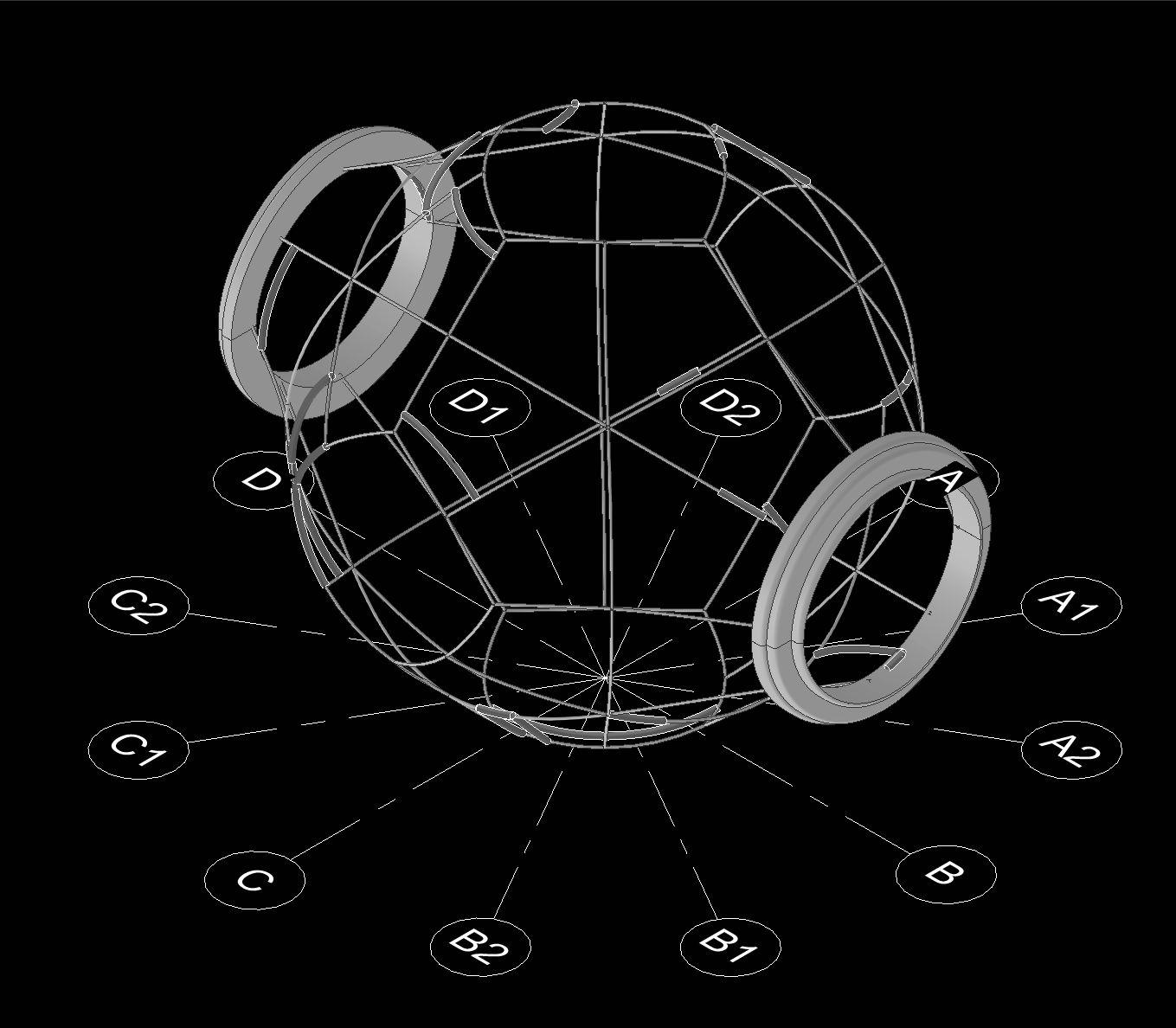
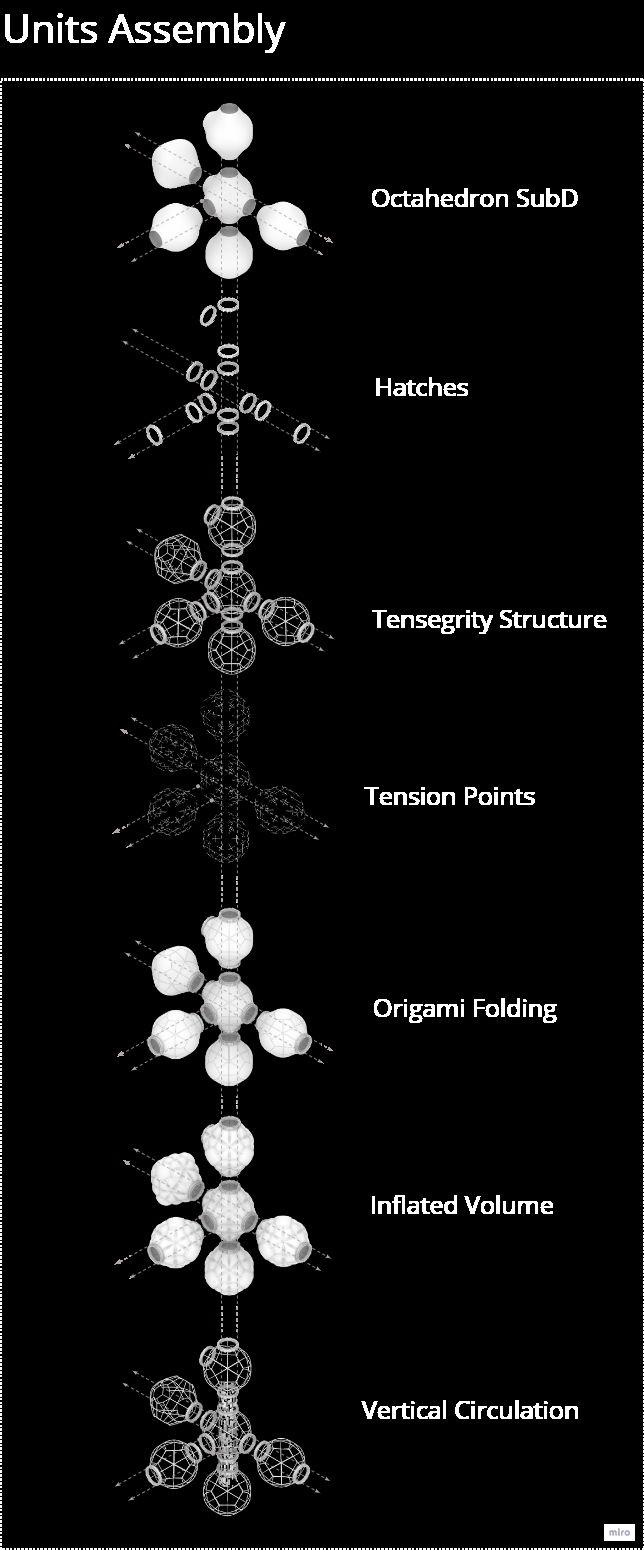
NEBULAE – RECREATION MODULES CONFIGURATION
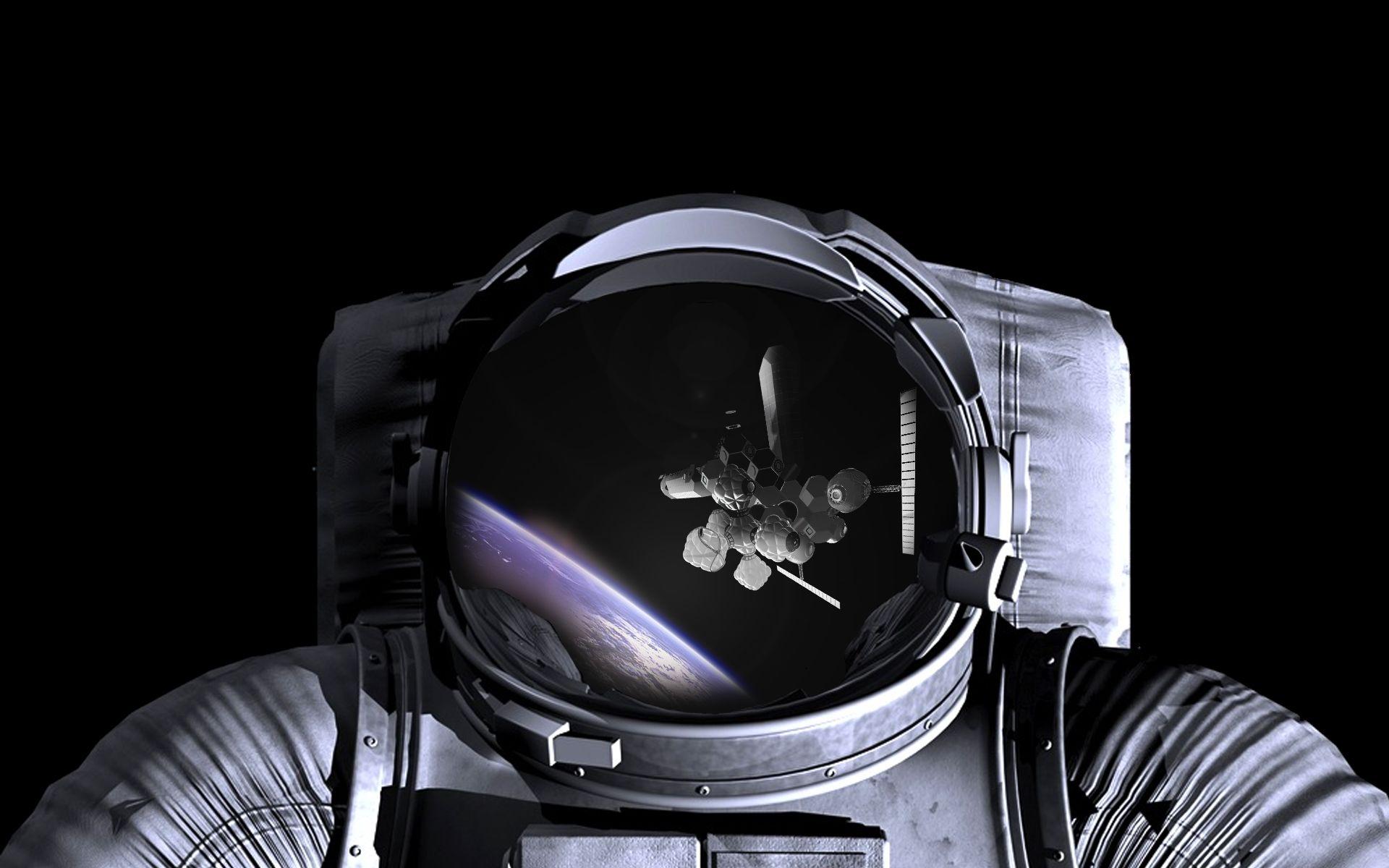
The modules are made up of two structures, one internal and the other external, that support and shape the two inflatable faces of the modules. The internal chamber that is generated is a radiation isolate as it is the receptive chamber for humidity and fluids for recycling.
Interior - Inflatable
Exterior - Inflatable
NEBULAE
DAY AND NIGHT MODULES
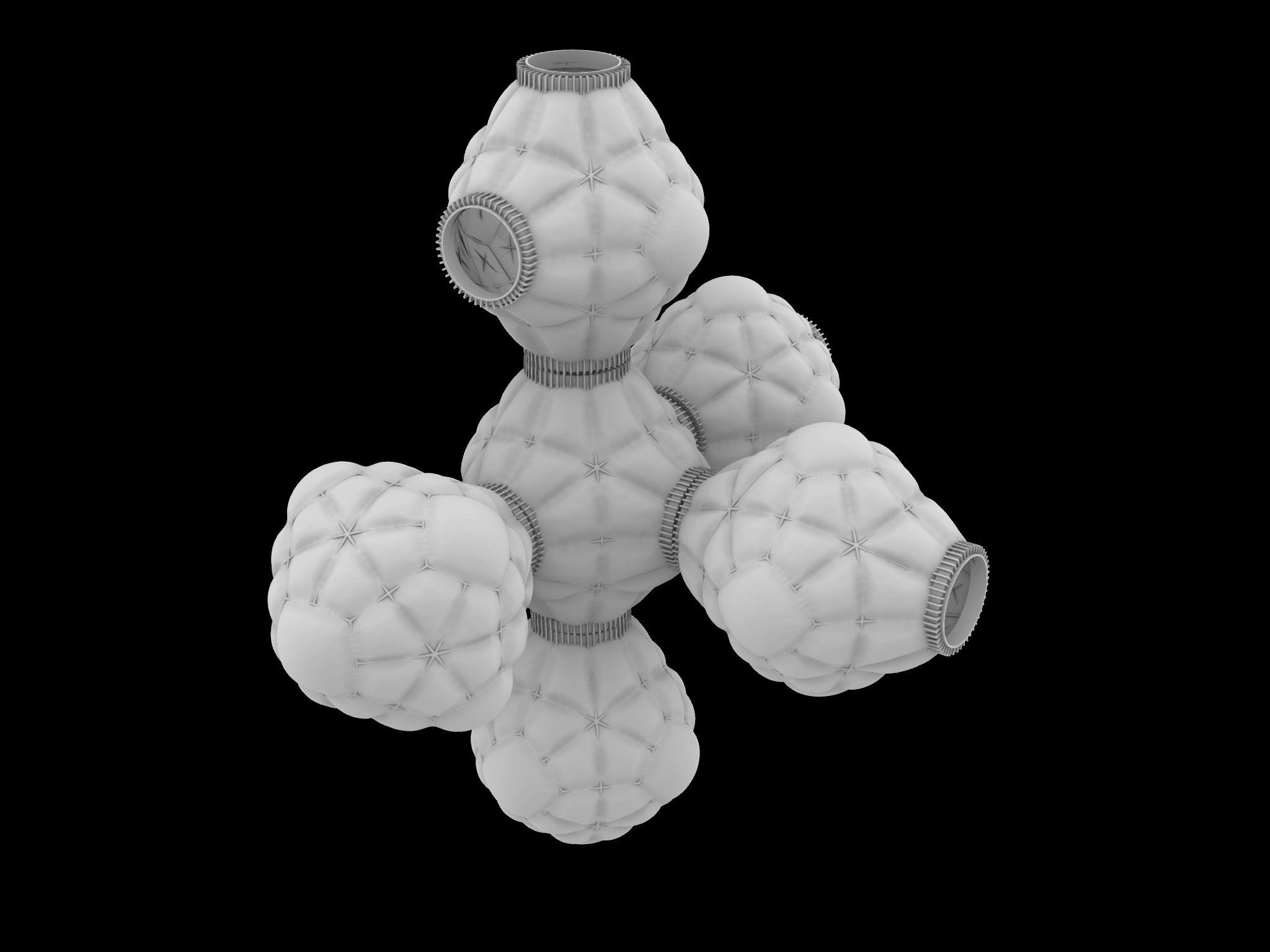
To determine the activities of each module we are based on present and absent activities. That is, the present activities are related to the necessary ones established by NASA, while the absent ones are those related to recreation. Recreational activities are divided into 4 modules that present: sport + physical activity, fun activities, sociability, and mental health. To increase the quality of mental health for astronauts and visitors, each activity is divided into day and night activities. Where the user chooses how to feel. As is known, every 45 minutes approx. dawn and dusk inside the station. This is why each module presents a different opening to the activity, creating a catalogue of modules by activity. The socialization module or CASINO is a night module, mental health – MEDITATION, READING + AR and a hybrid -Isolation module, GAMING + SPORTS and EXERCISE are daytime modules. Finally, the middle module is assigned as a connector.
Recreation Cluster
Space Orbit Habitat New ISS
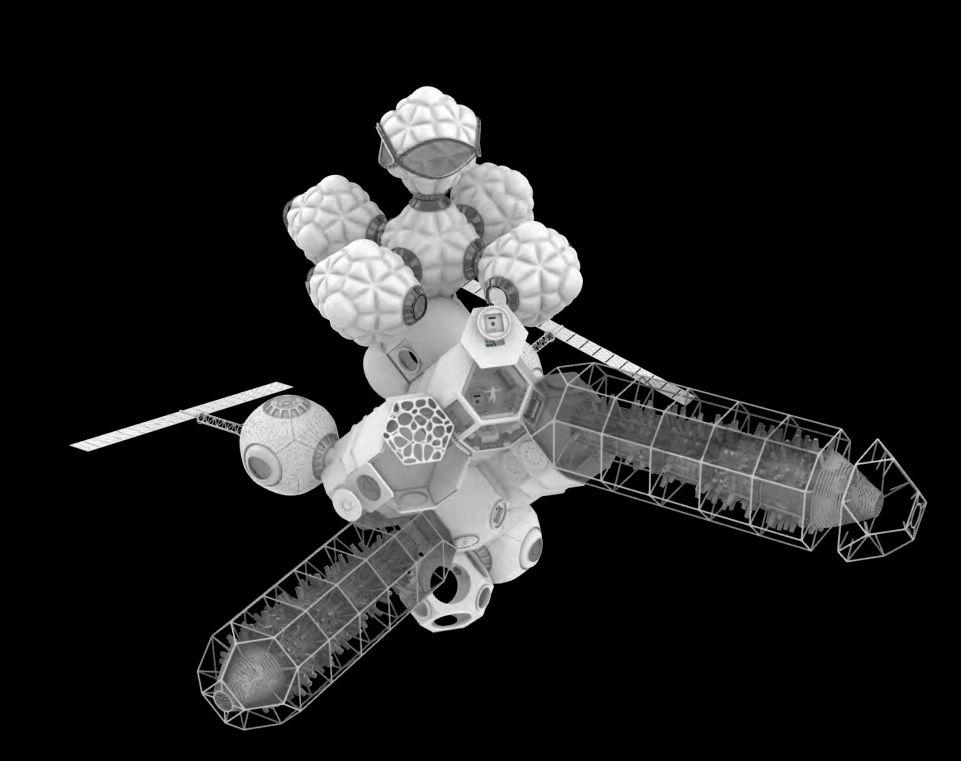
Yoga Module Gaming Module MODULES AGGREGATION
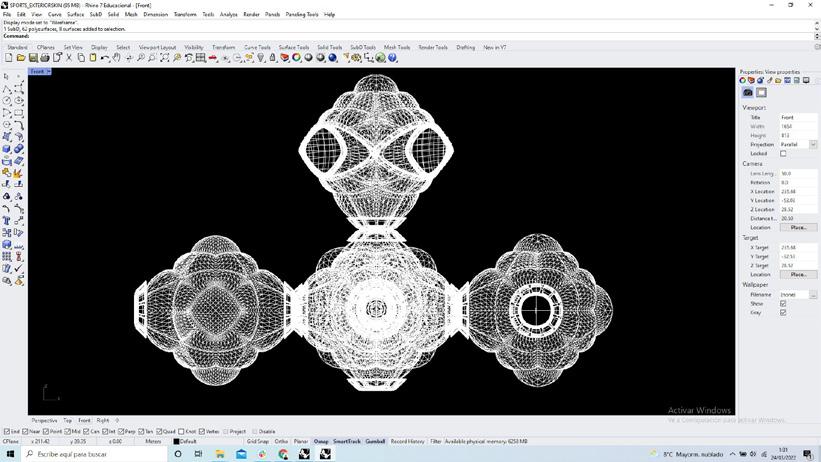
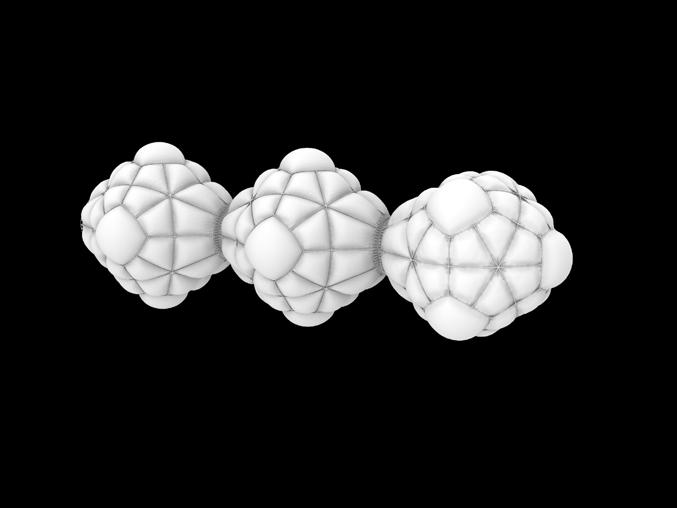
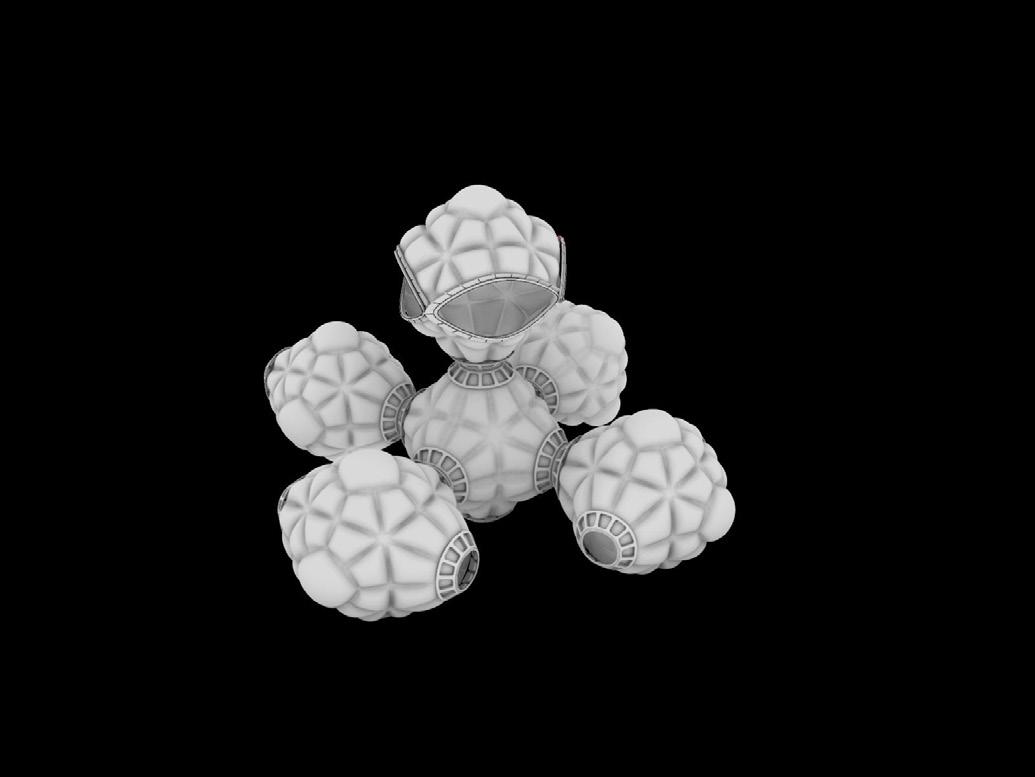
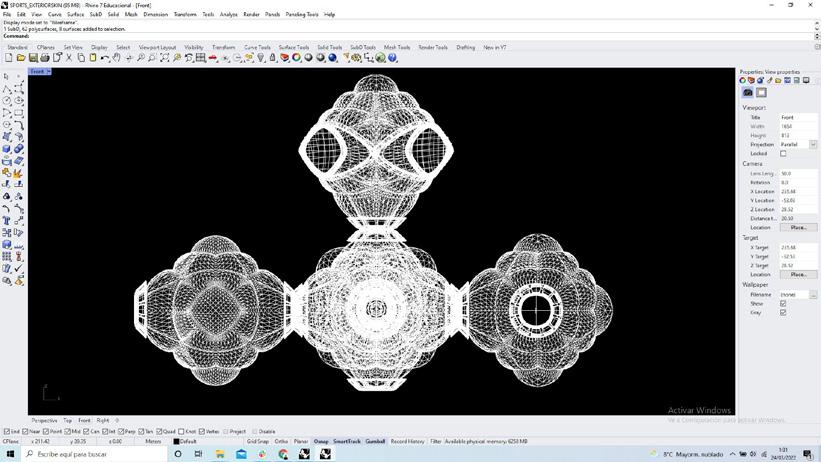
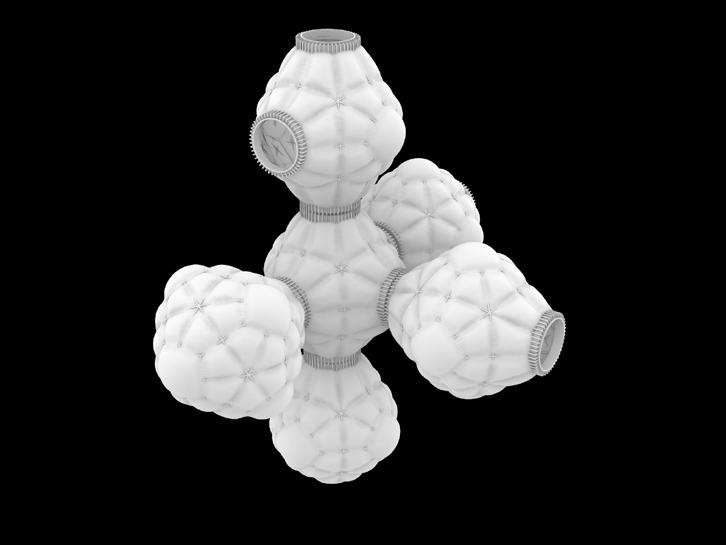
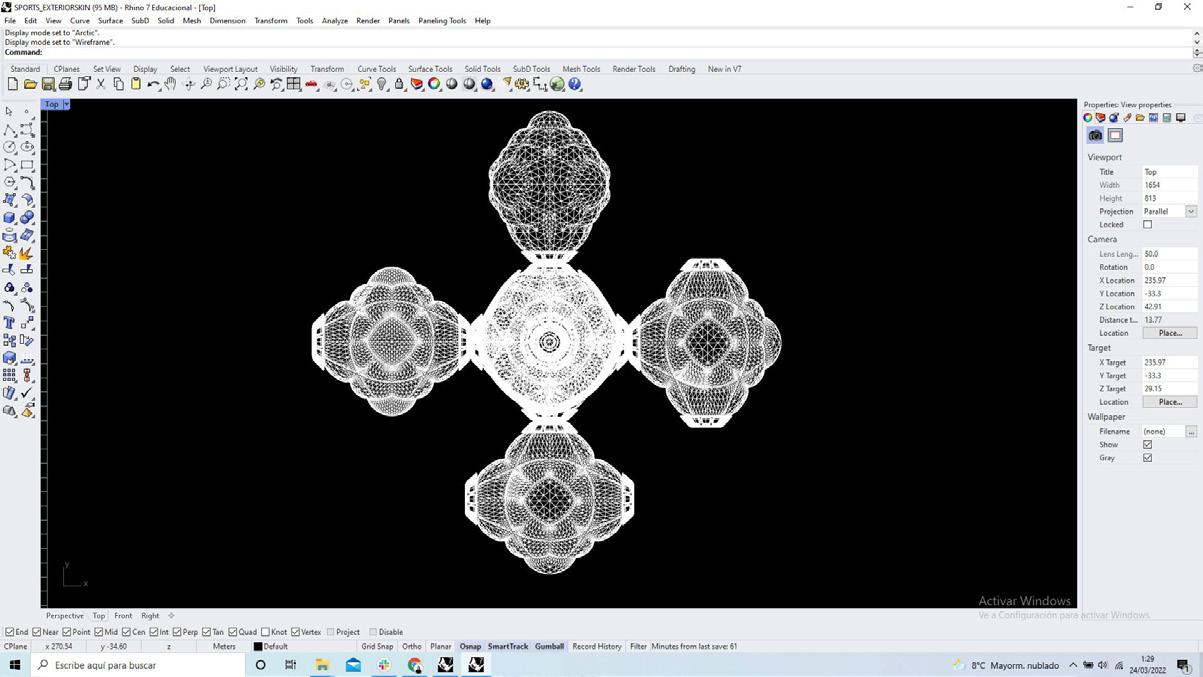
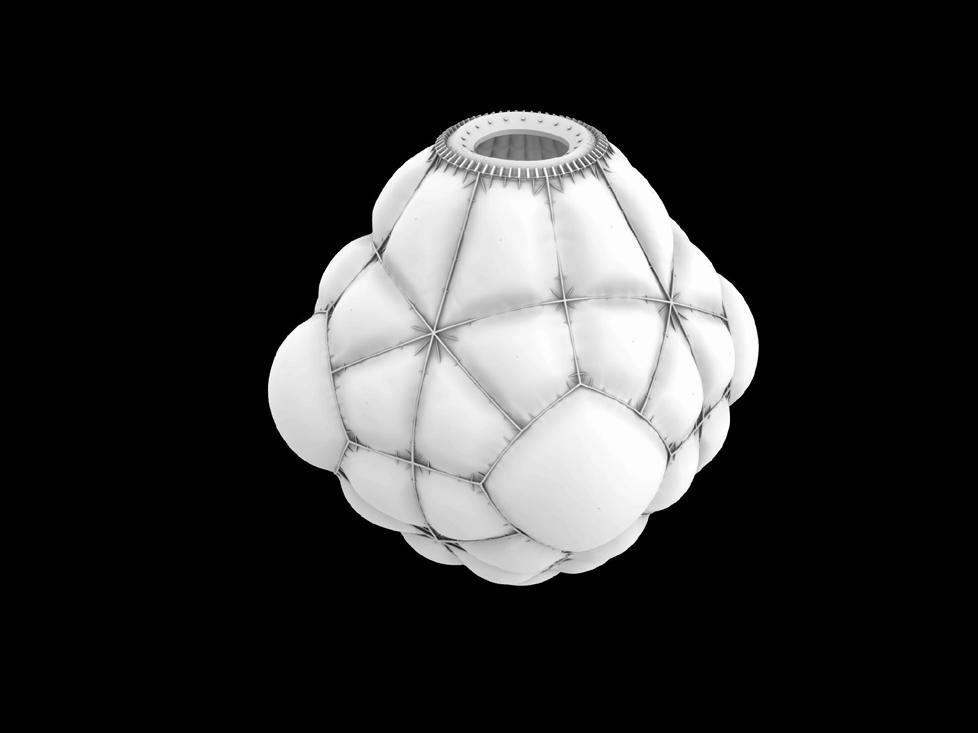
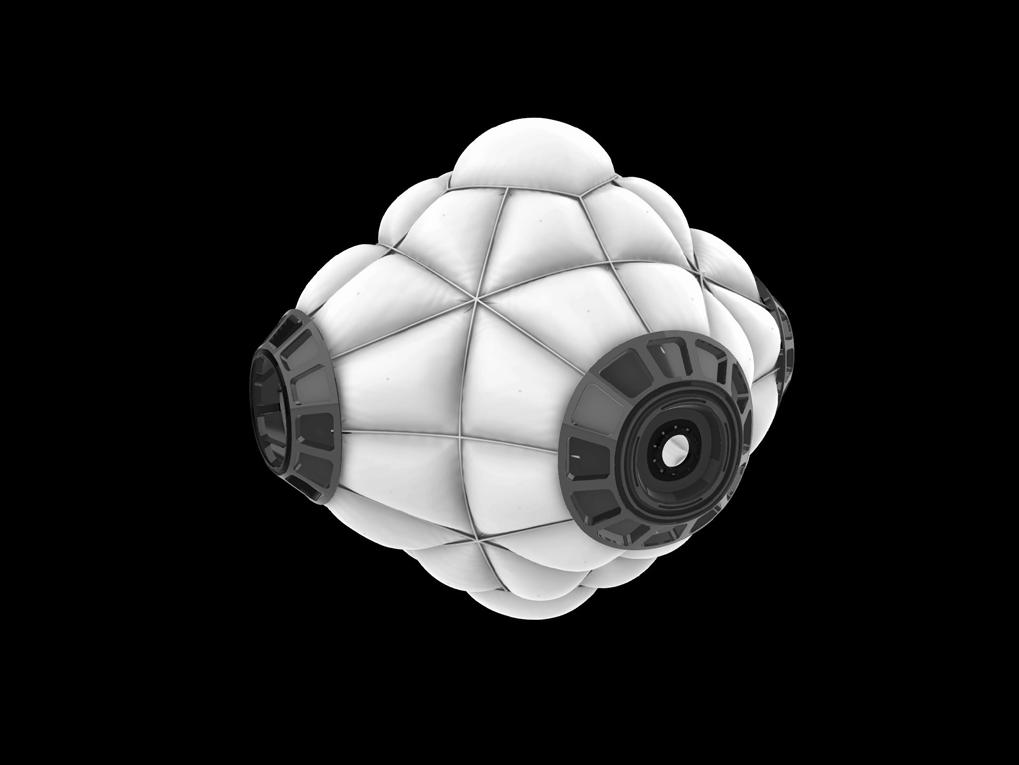
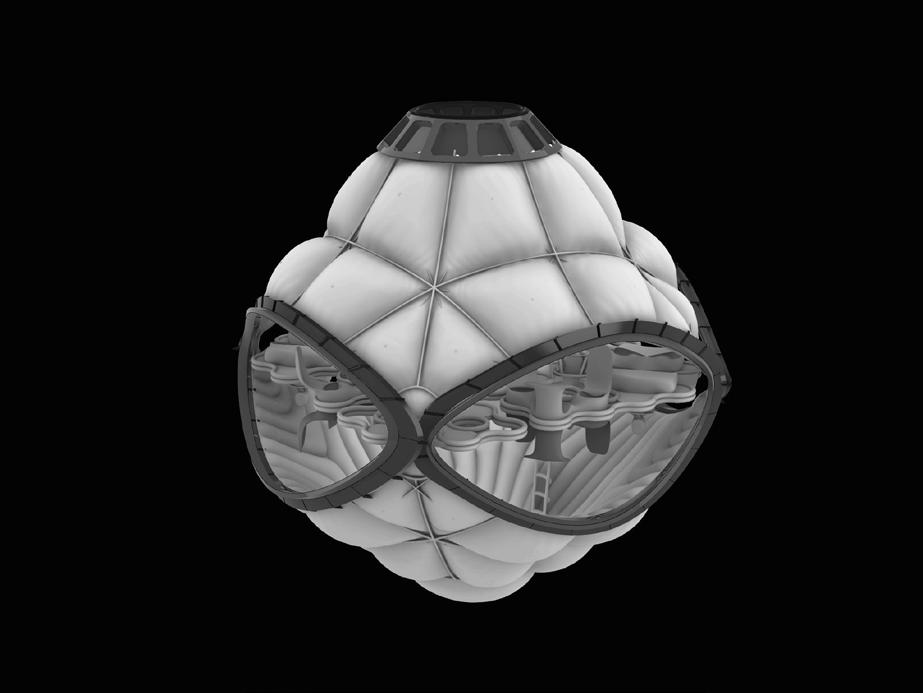
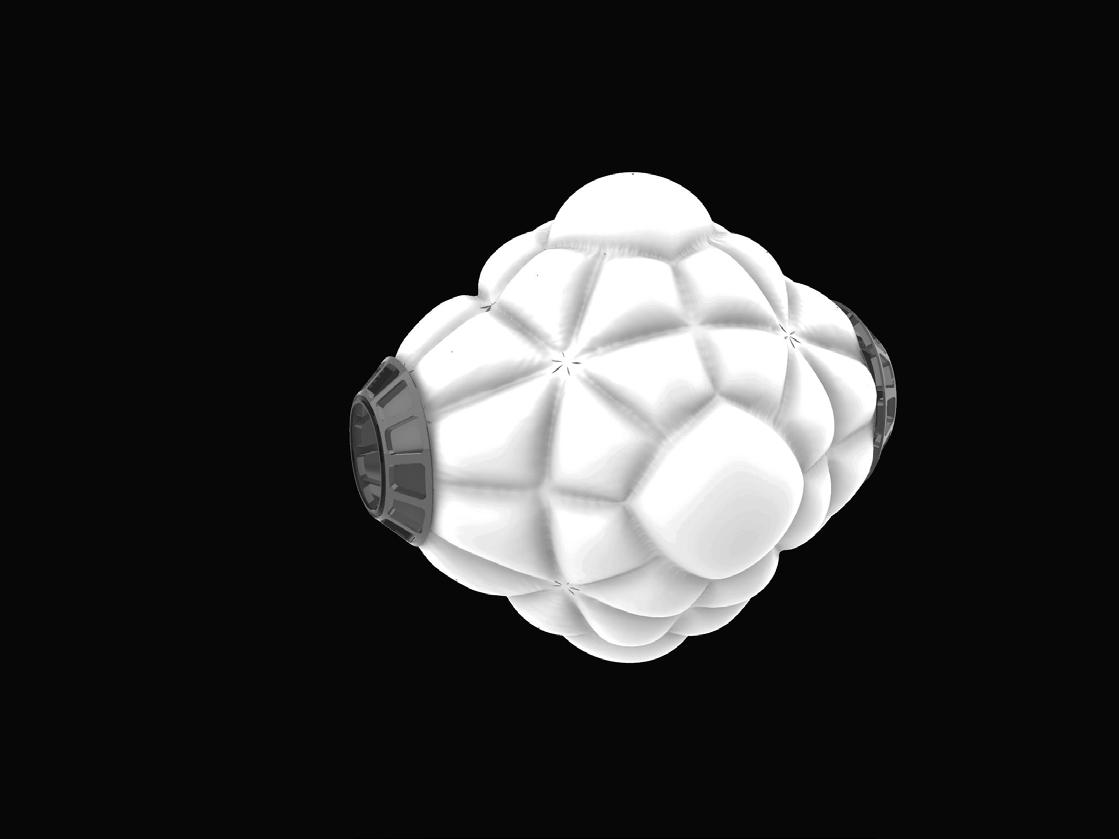
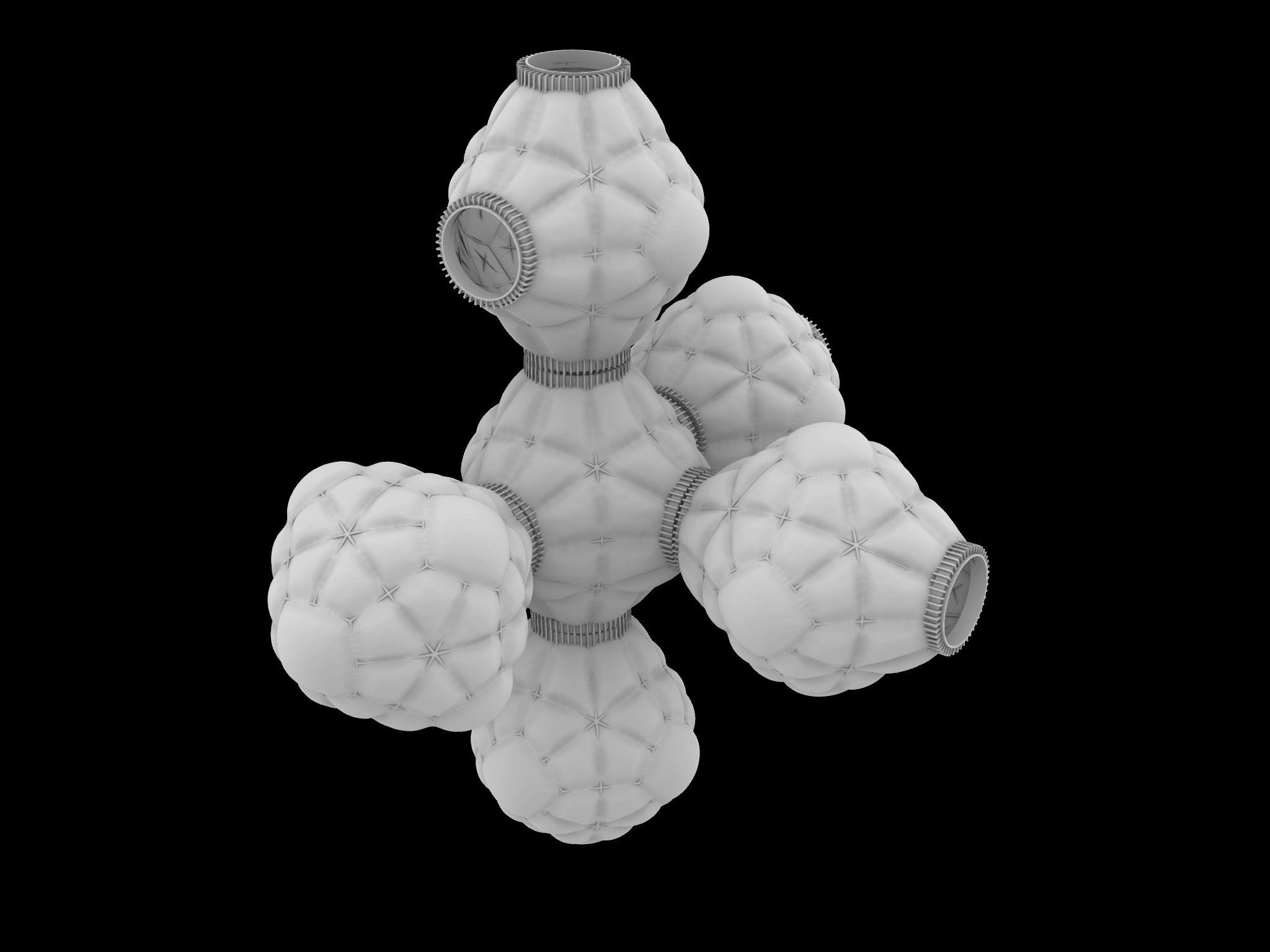
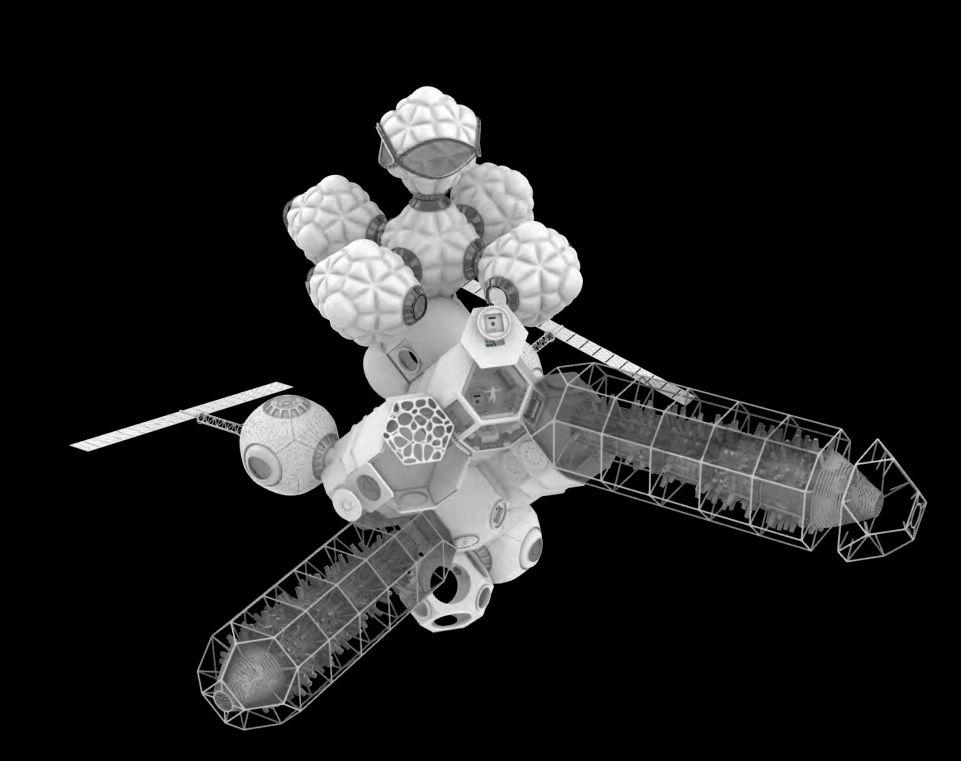
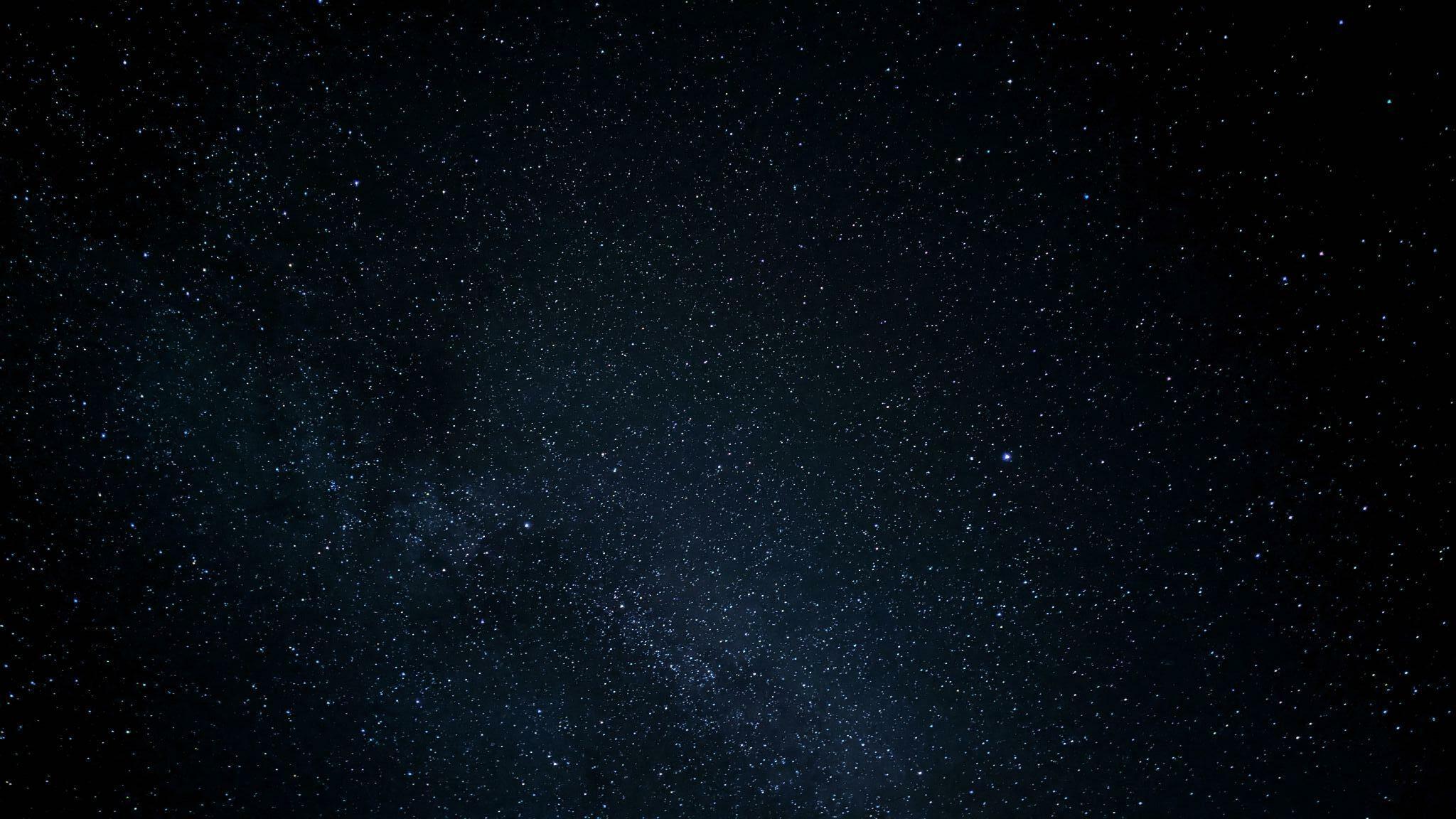
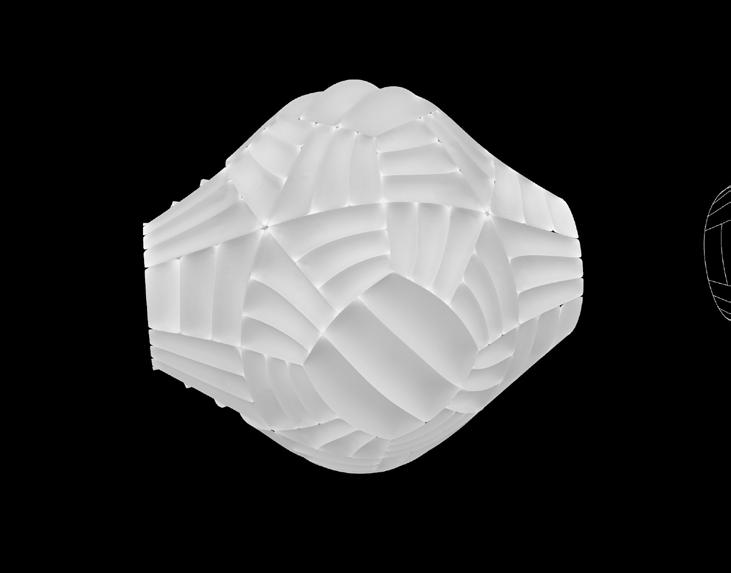
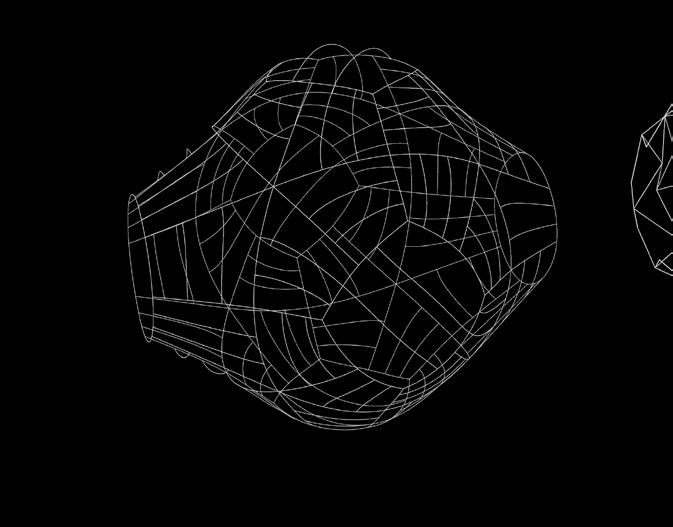
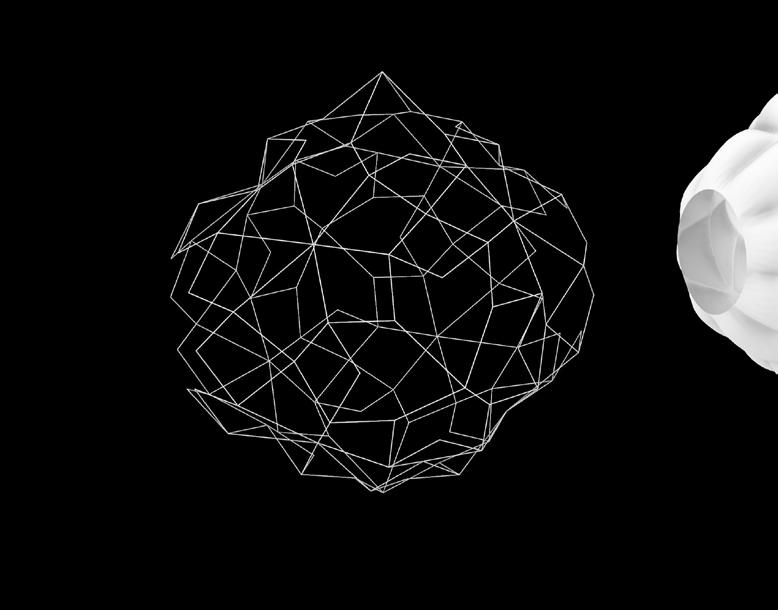
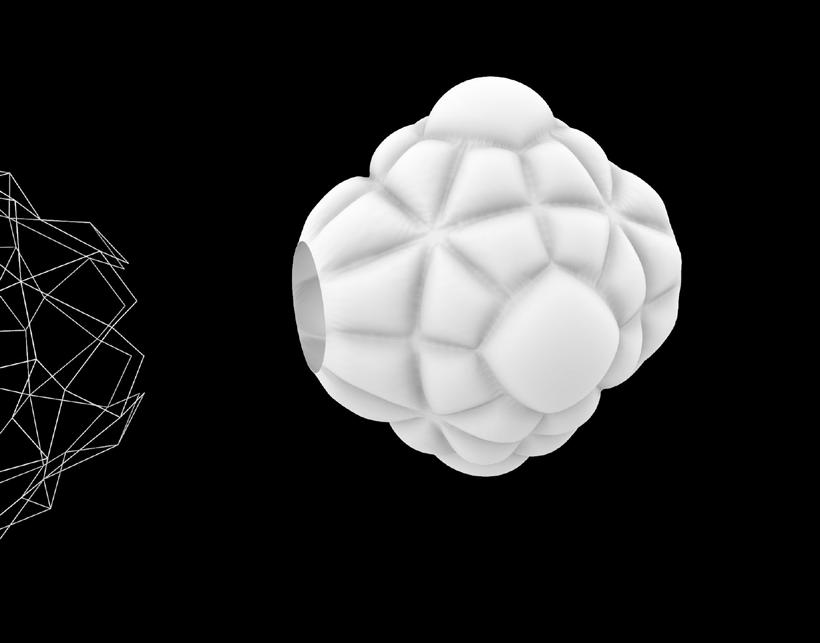
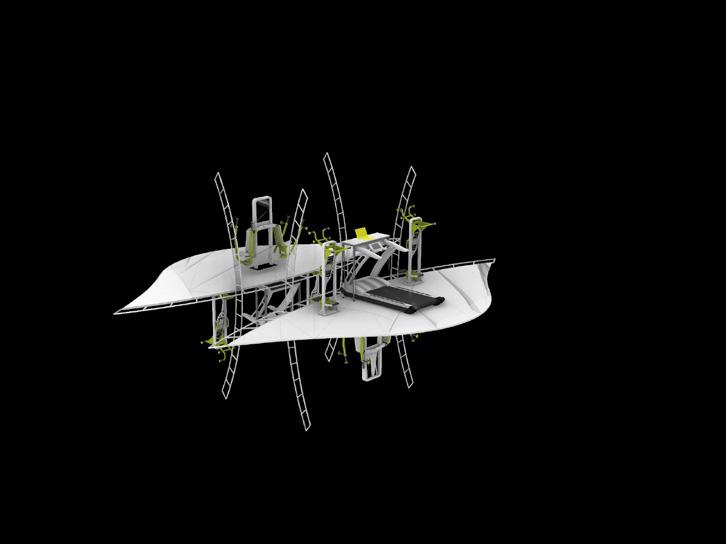
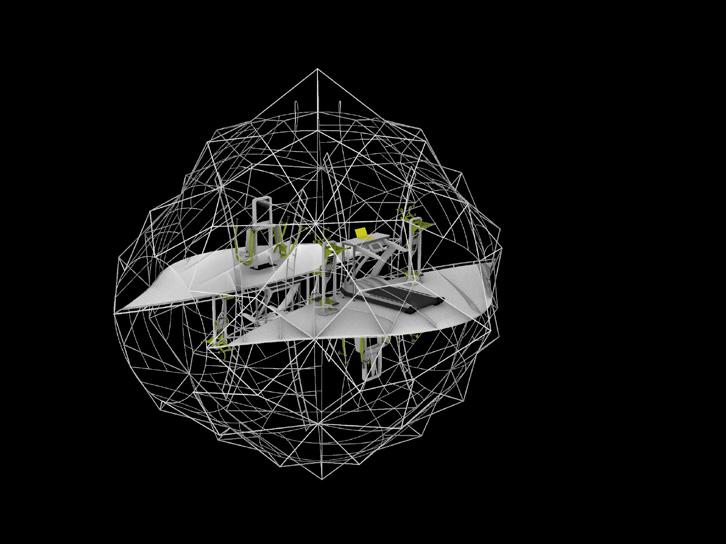
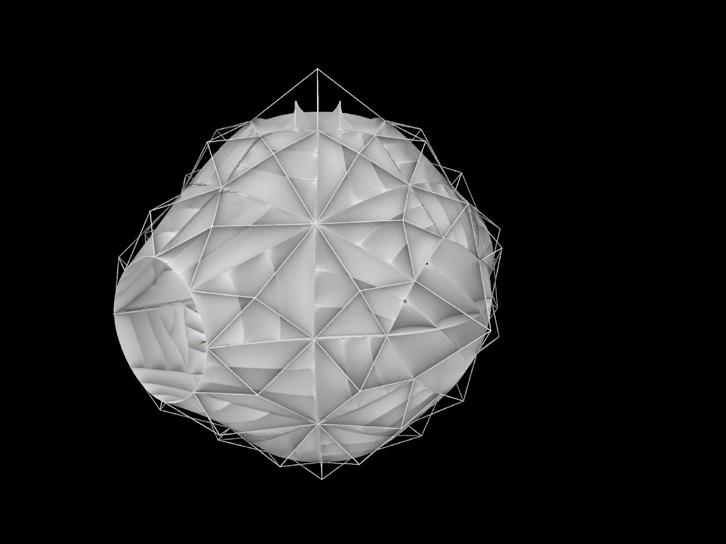
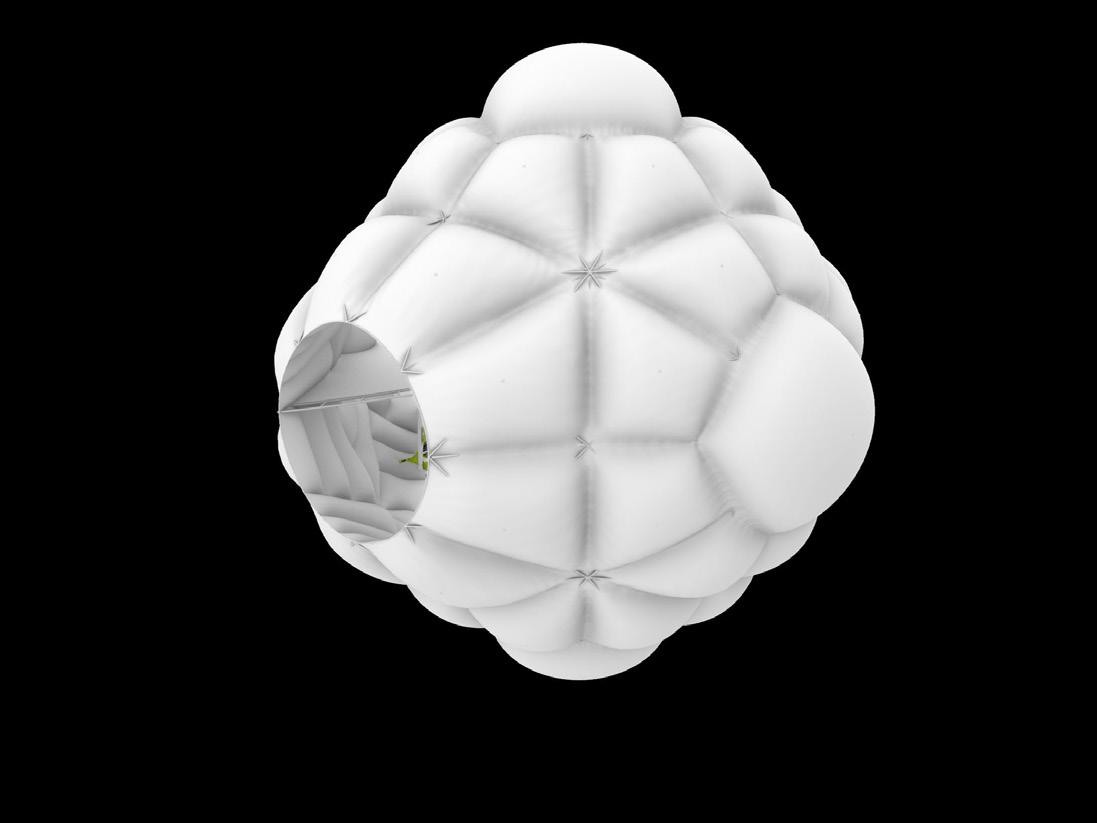
INFLATABLE SYSTEM
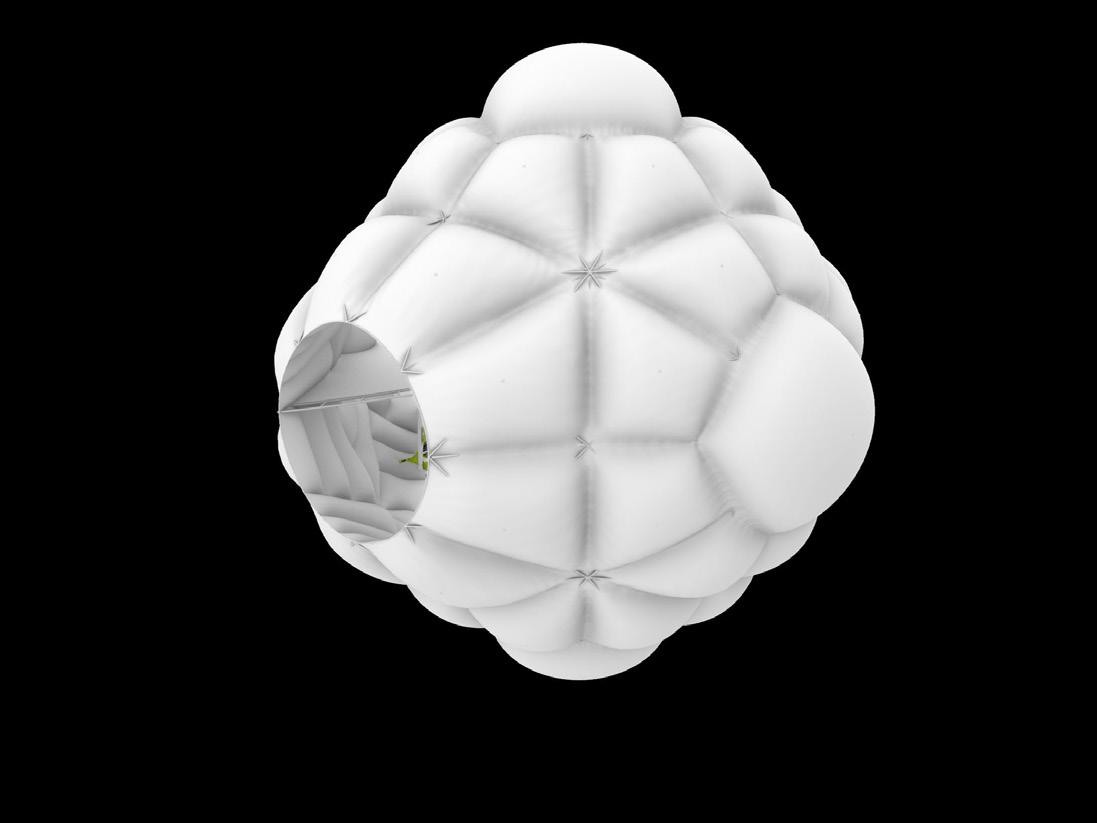
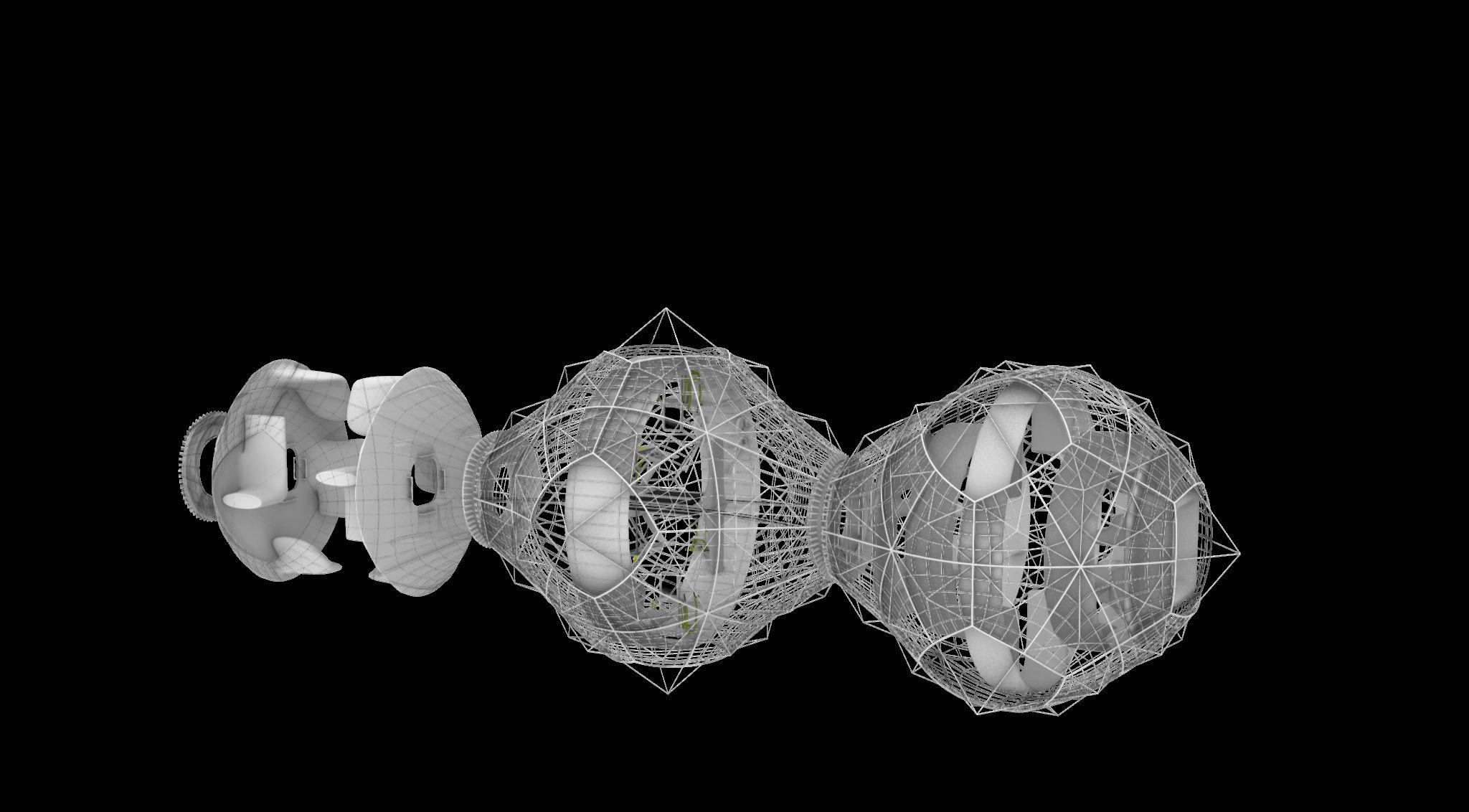
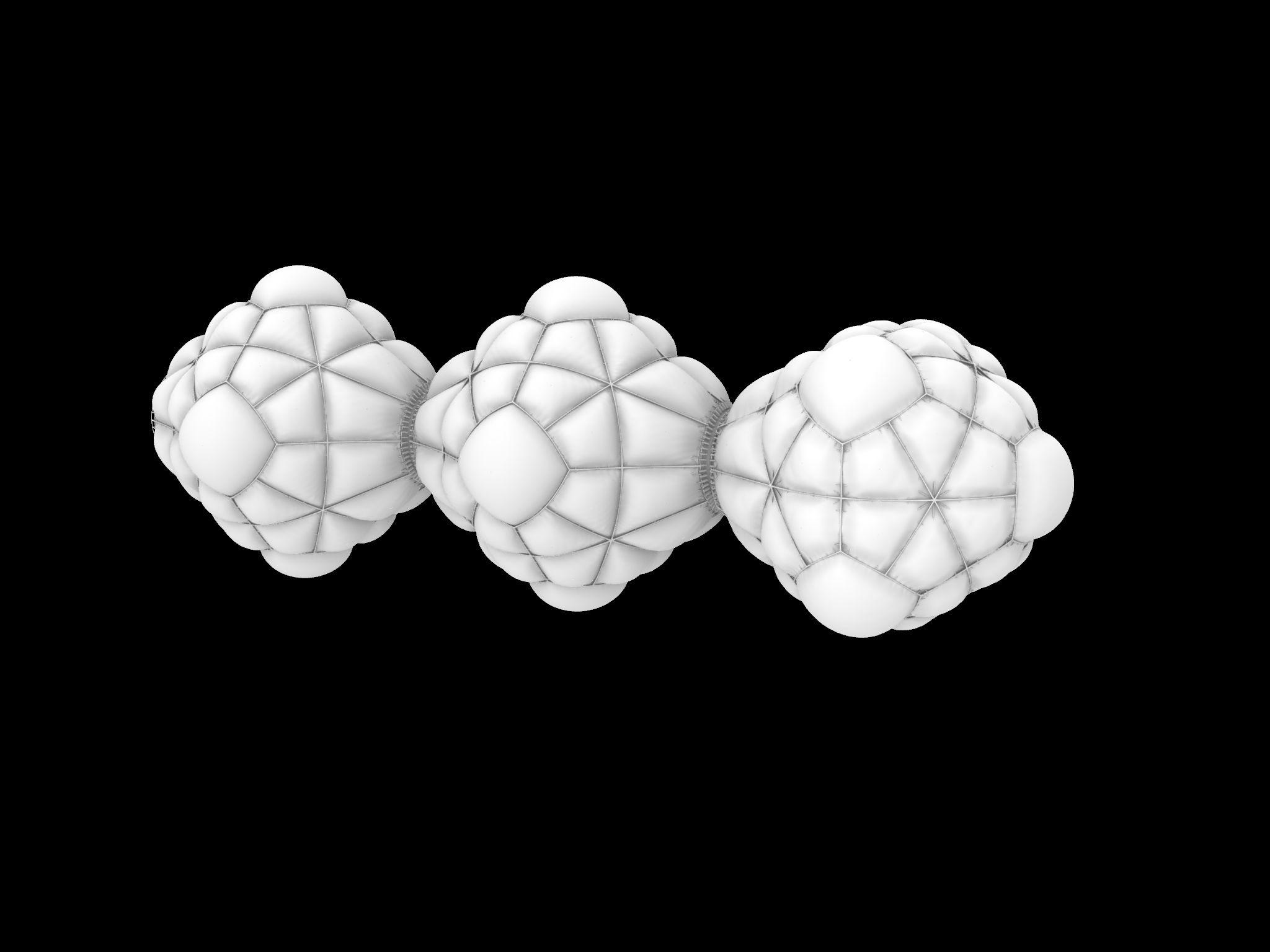
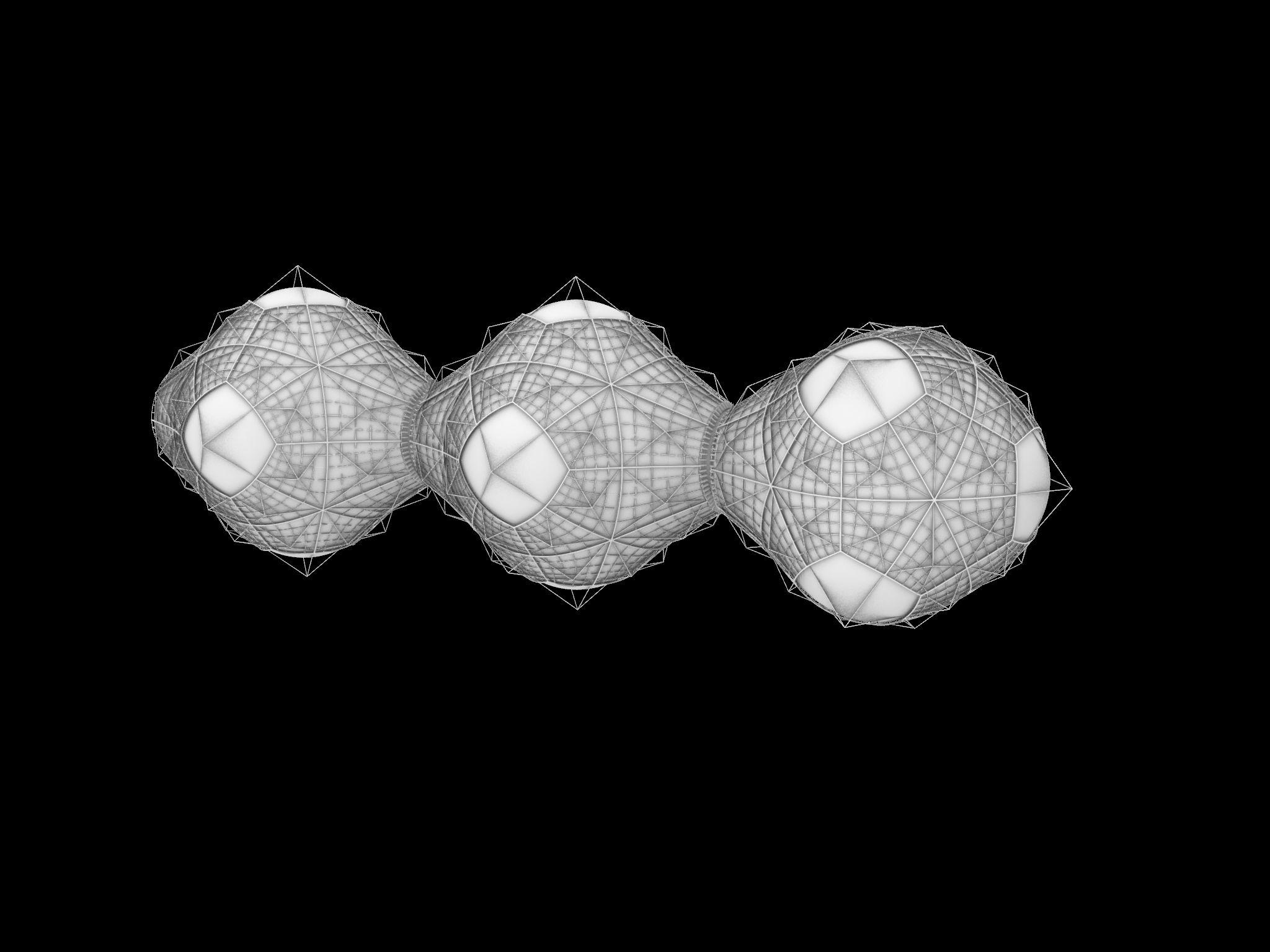
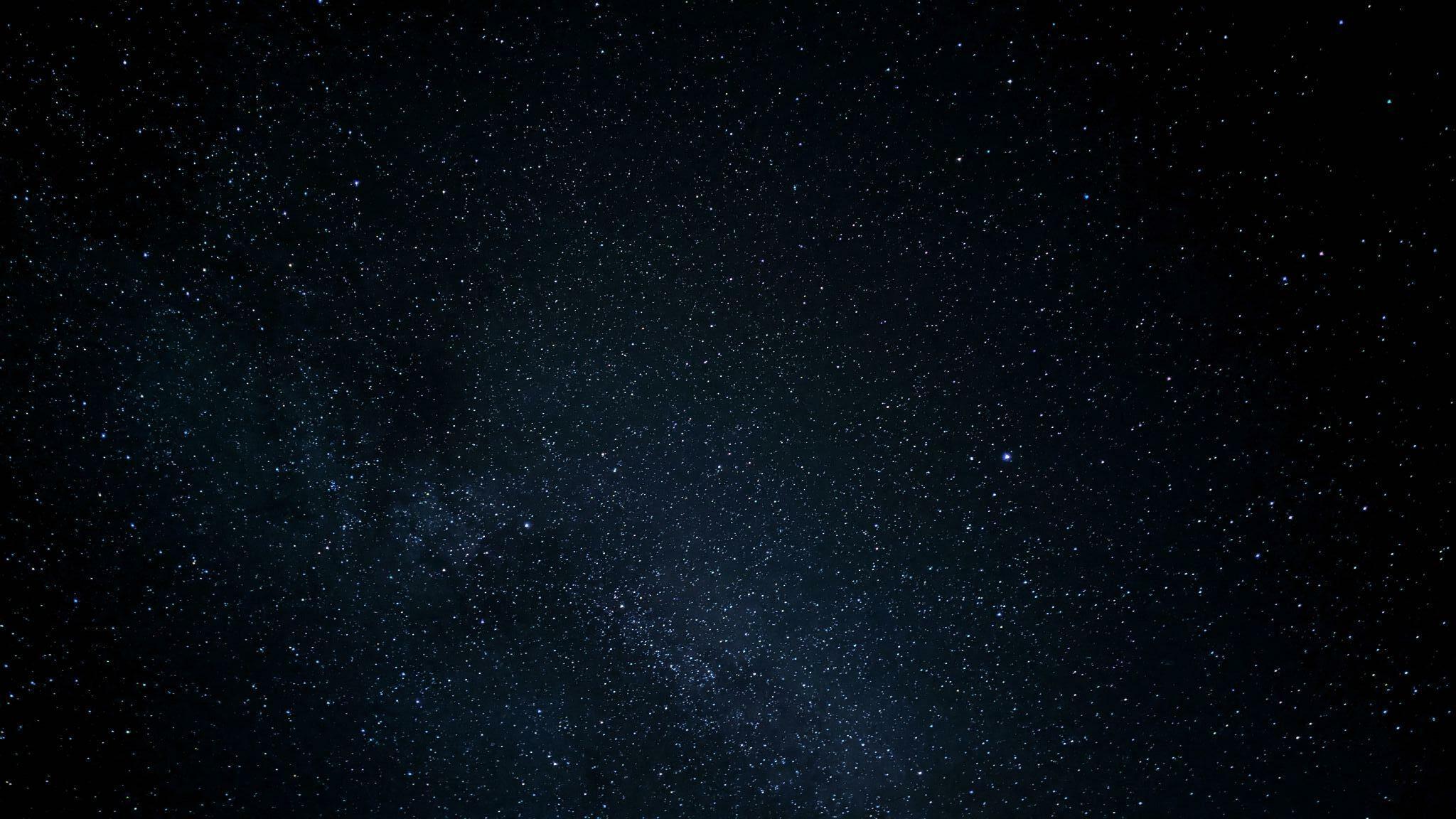
NEBULAE
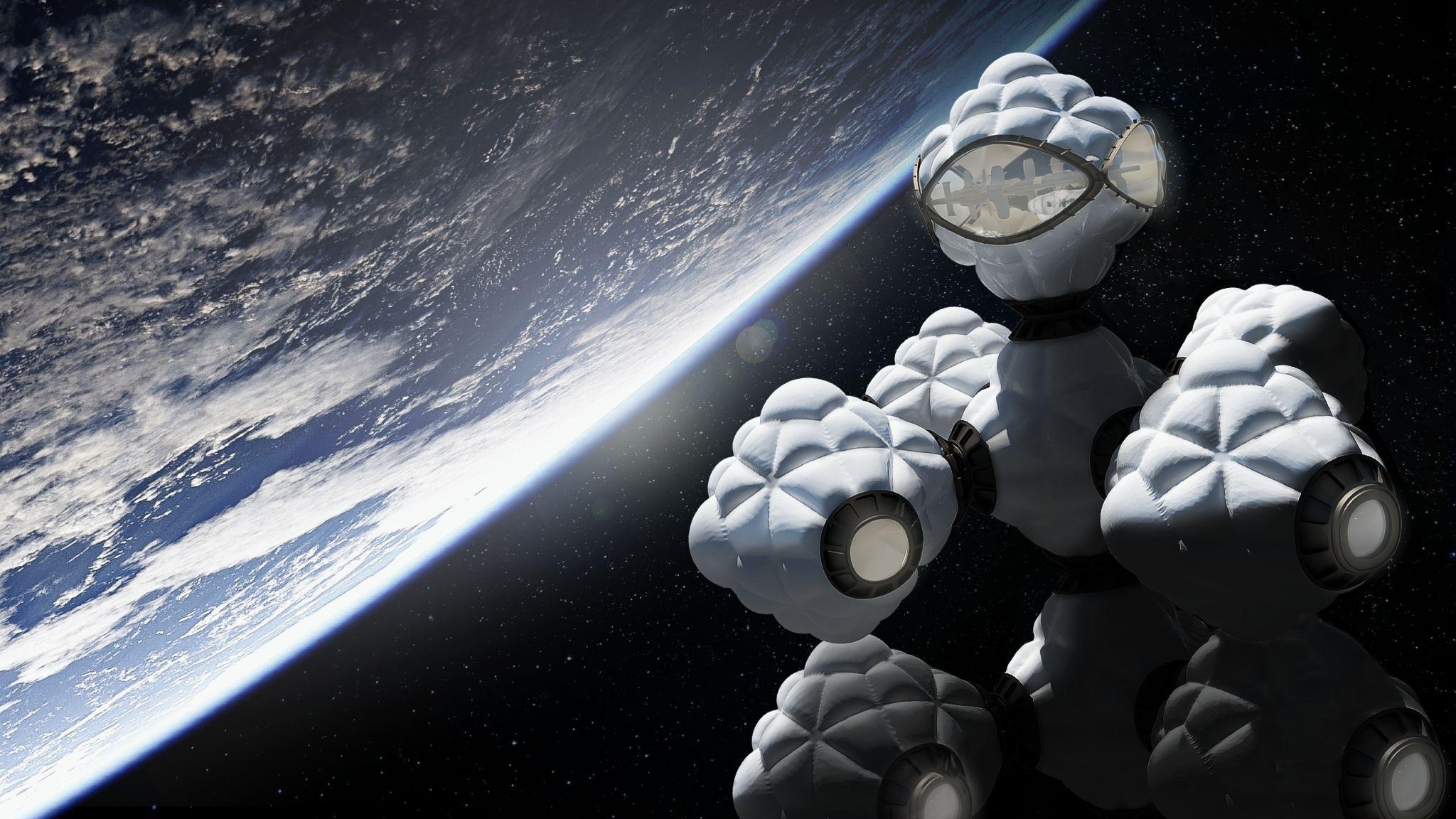
Recreational Typologies

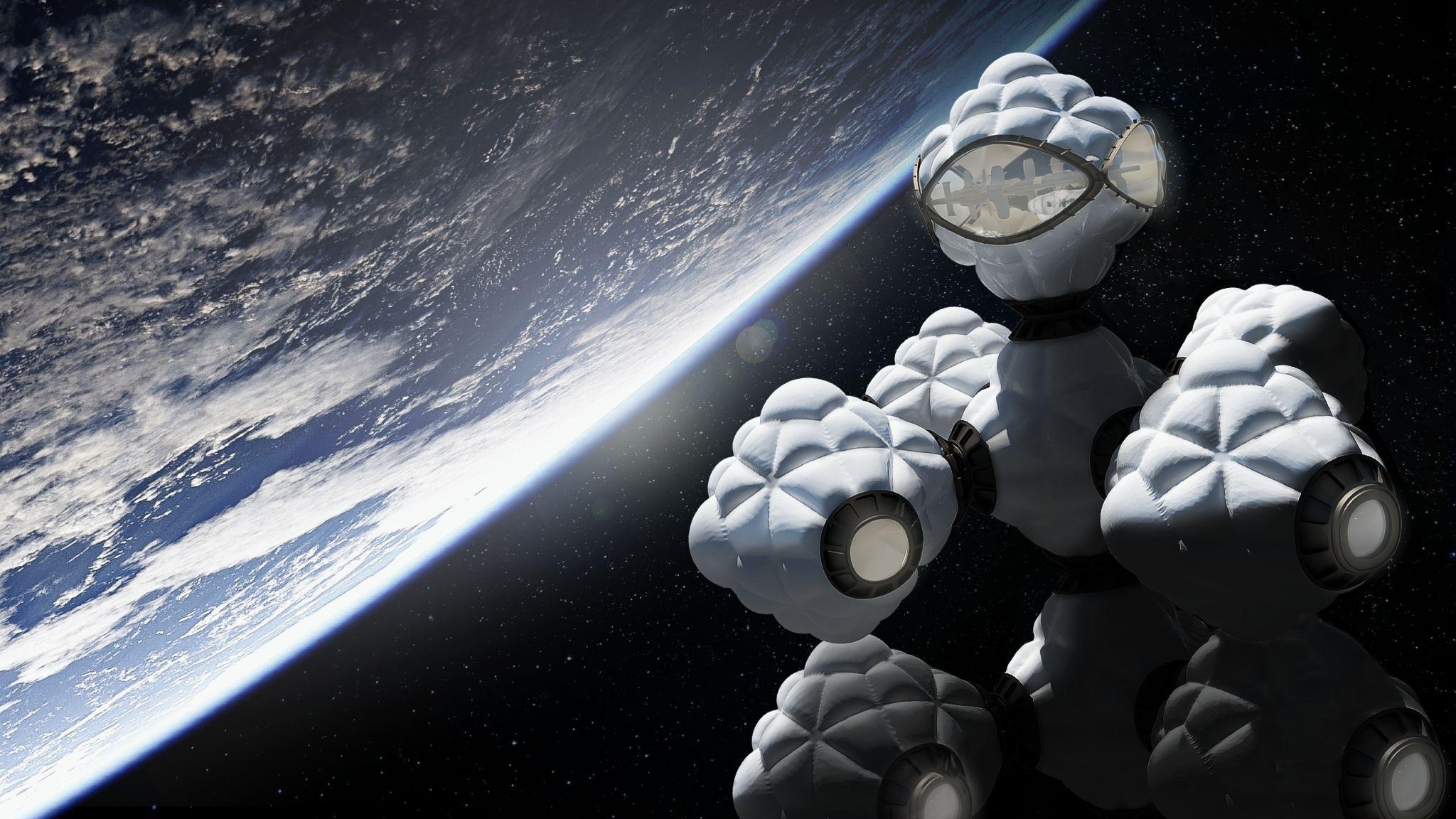
NEBULAE
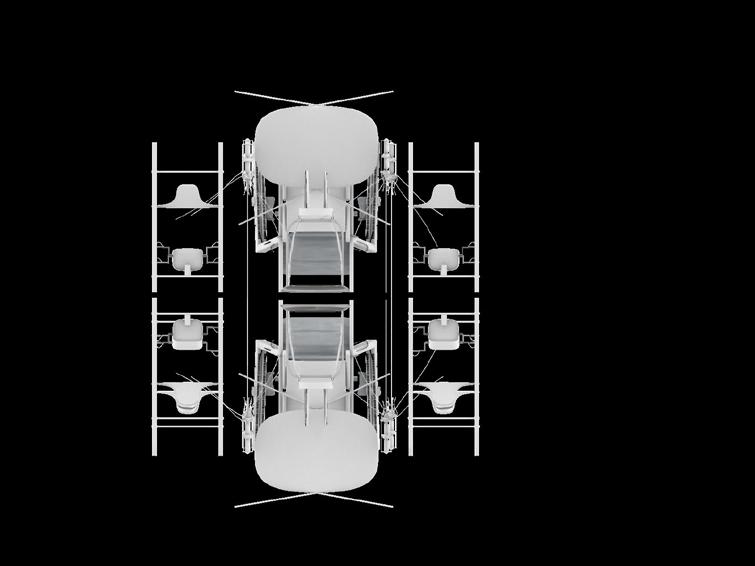
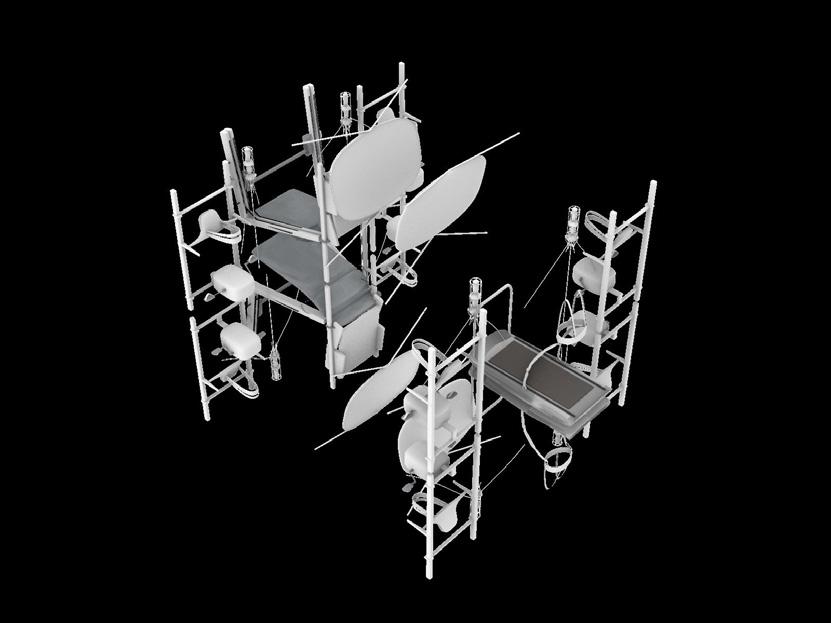
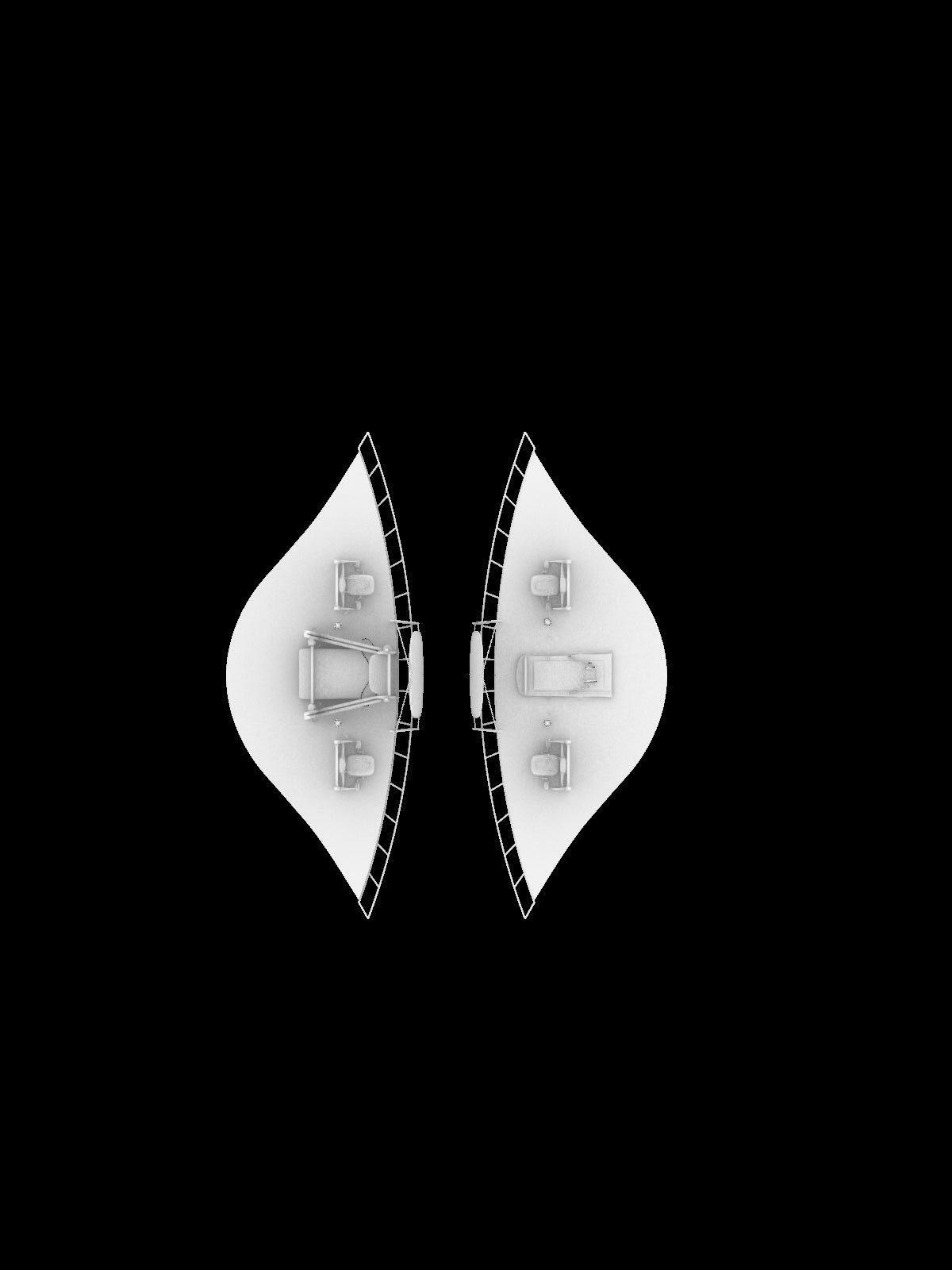
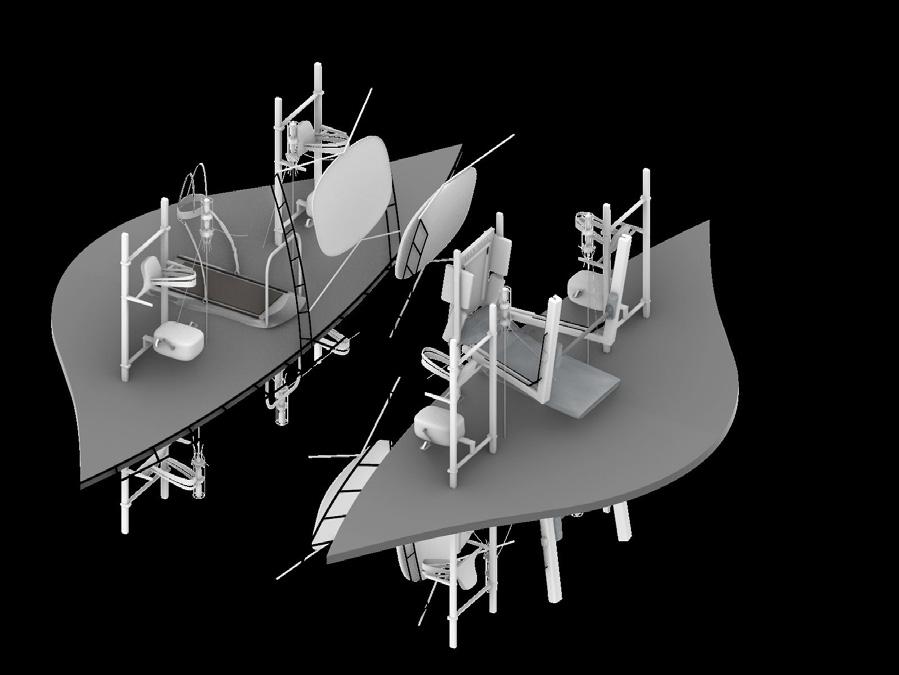
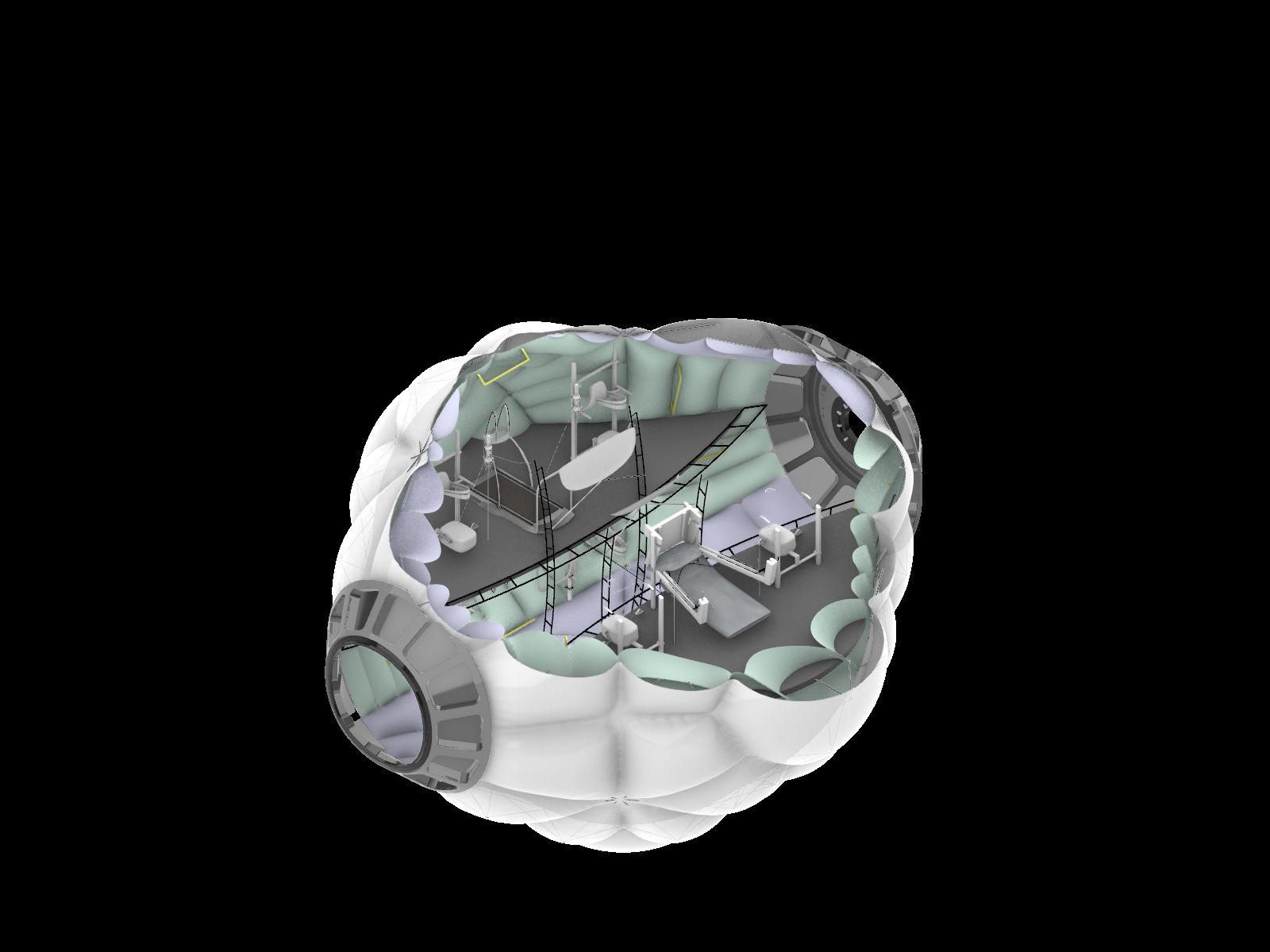
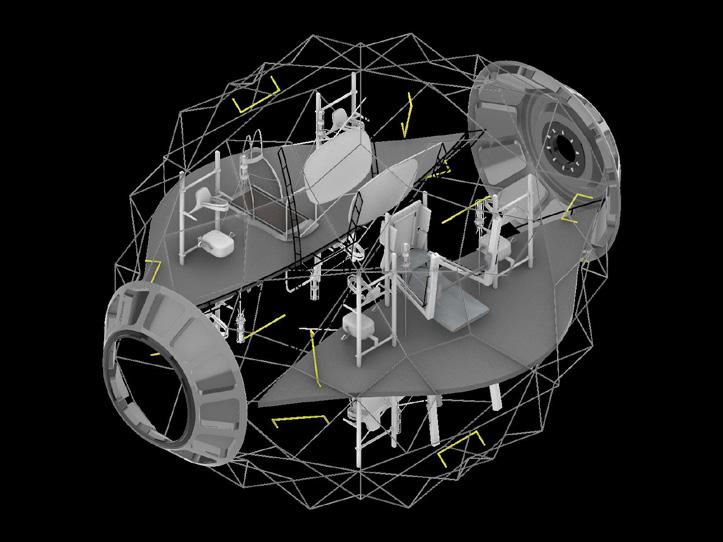
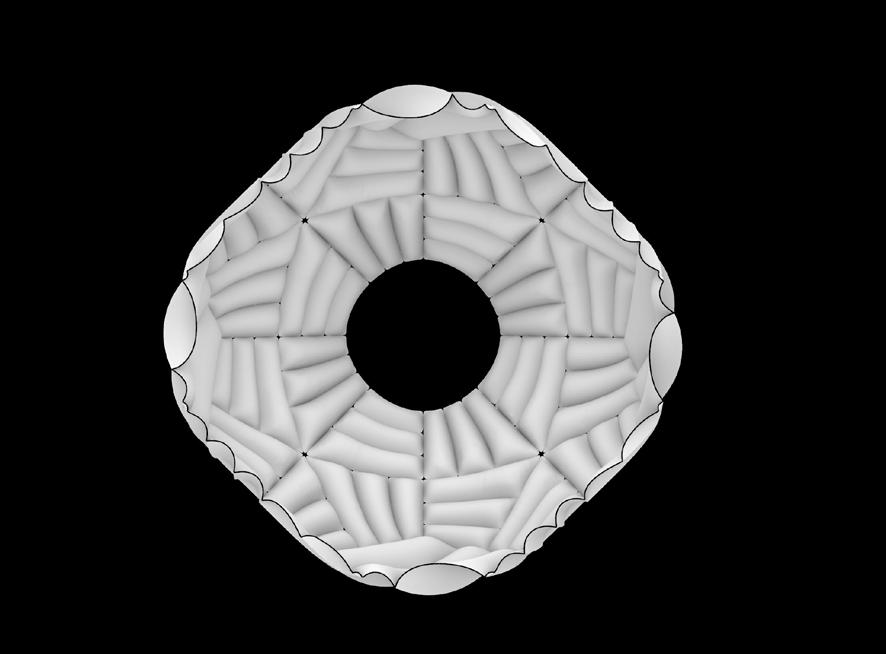
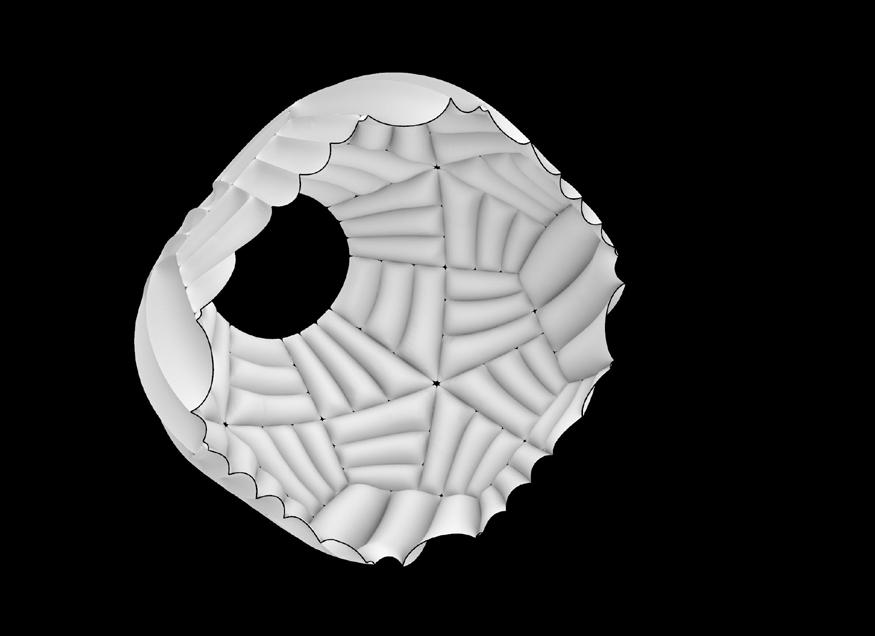
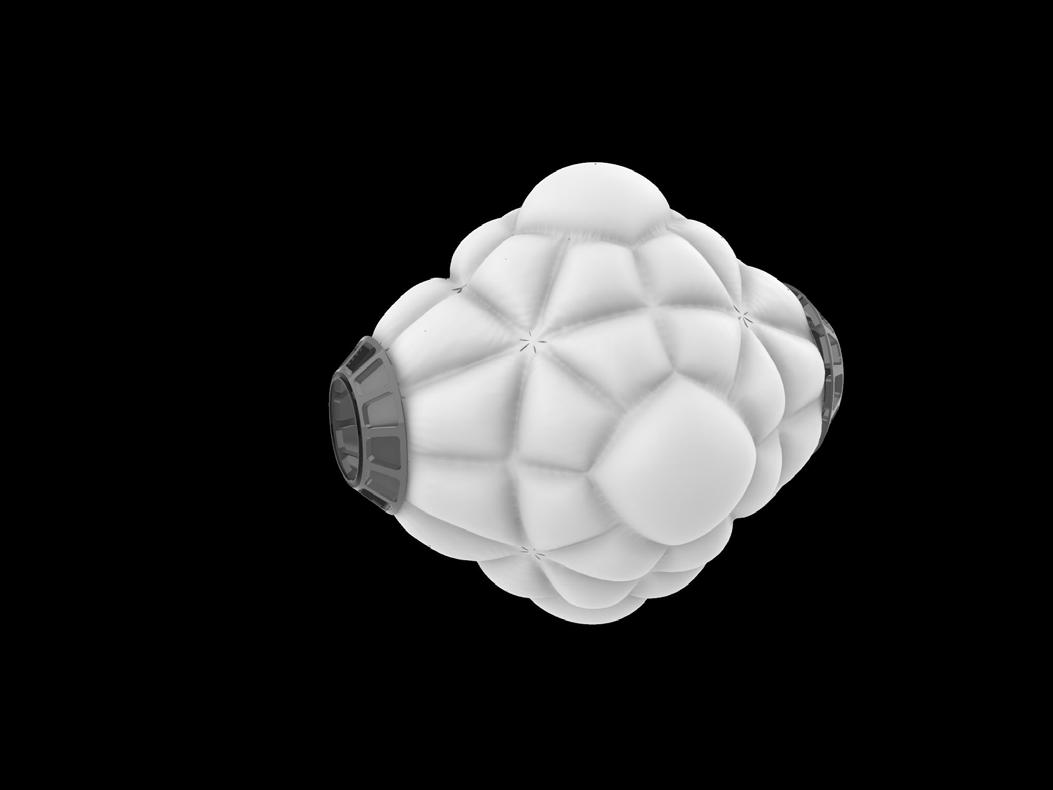
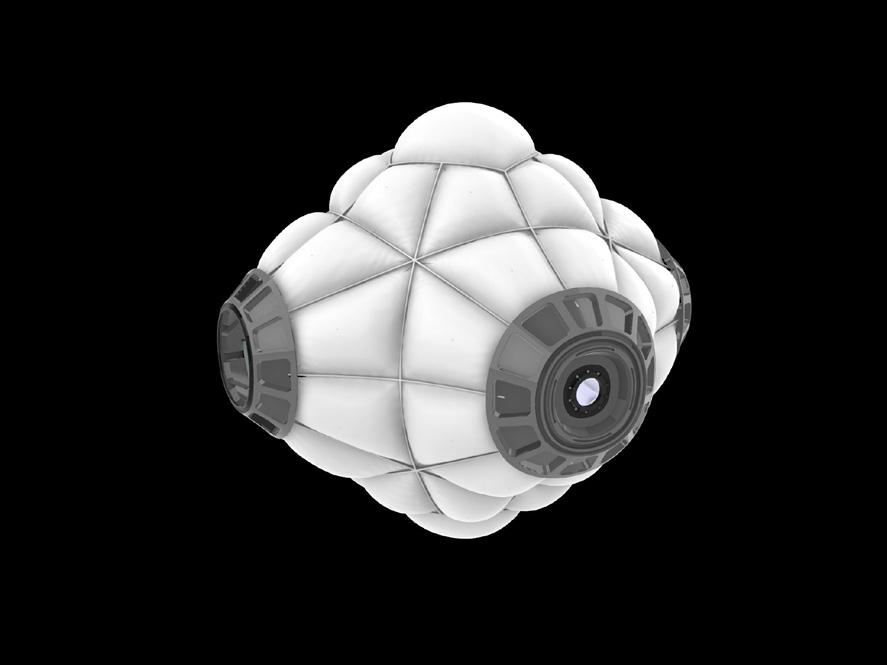
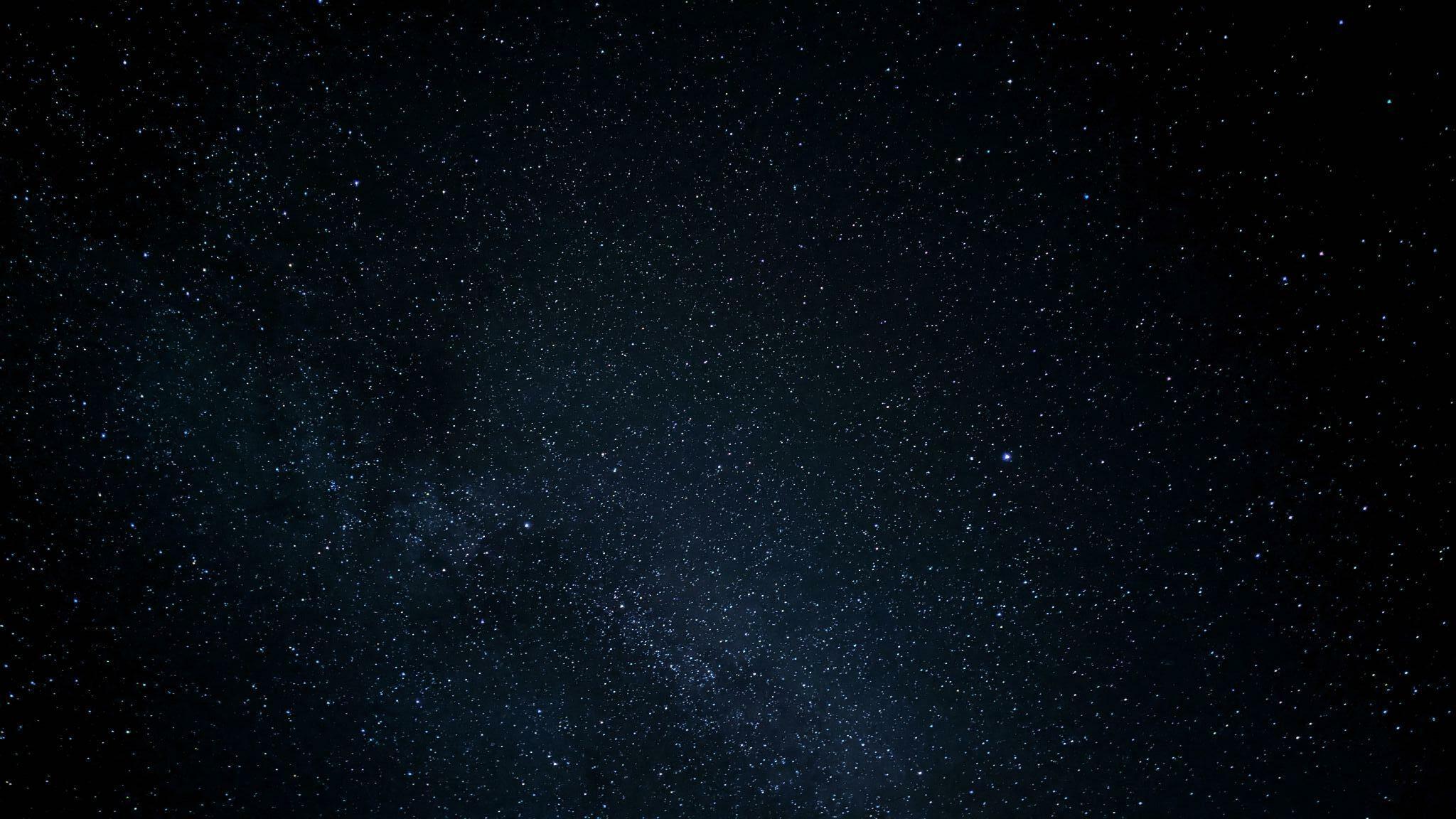
Sports Module
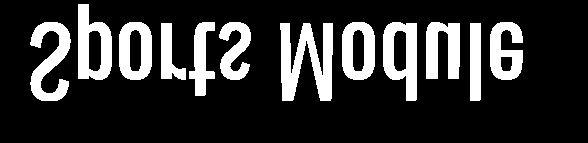
The Exercise Module has the necessary equipment to maintain the shape of the Astronauts. The interior pattern mimics a dynamic and fluid environment. The interior configuration is mirrored, using both sides of the module. The colour of the patterns divides the ground and sky perspective by introducing colours to the patterns.
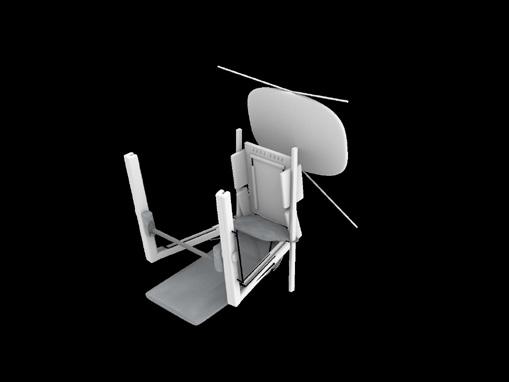
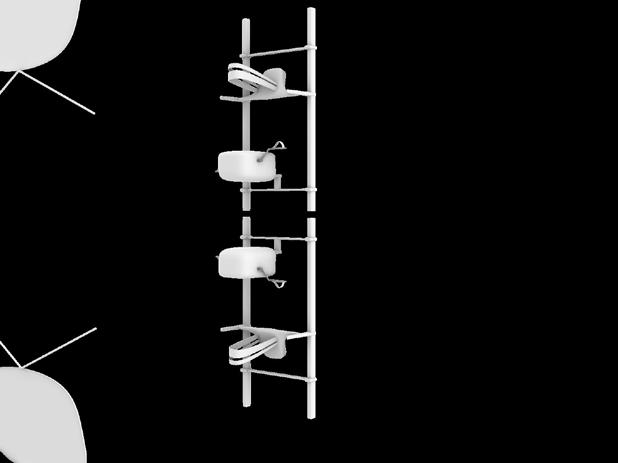
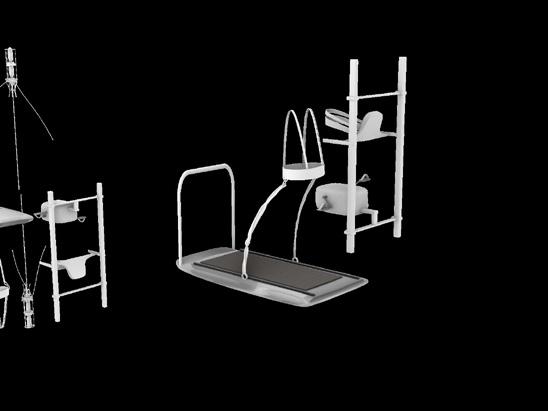
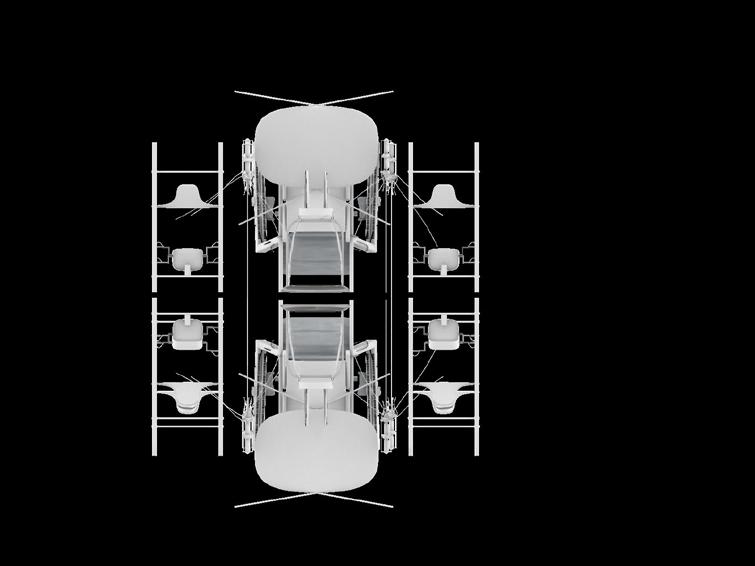
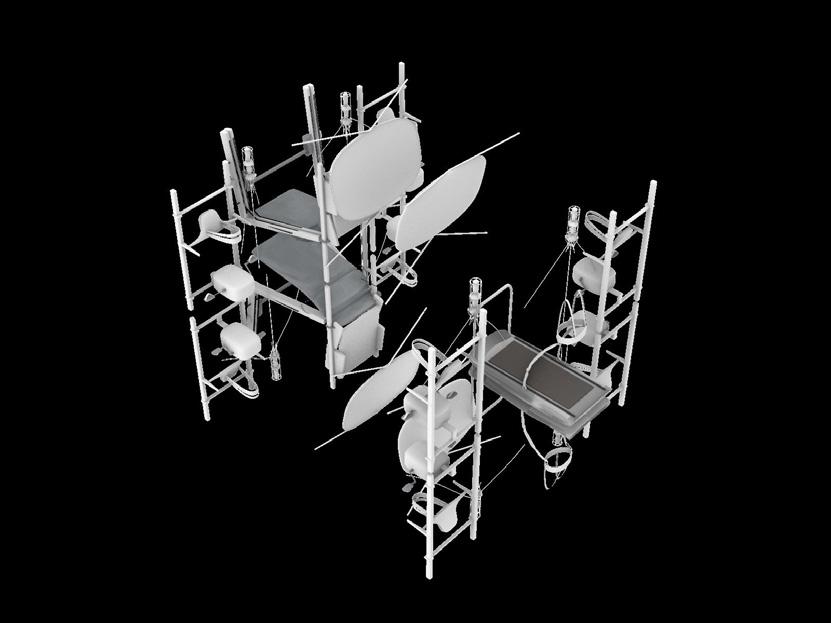
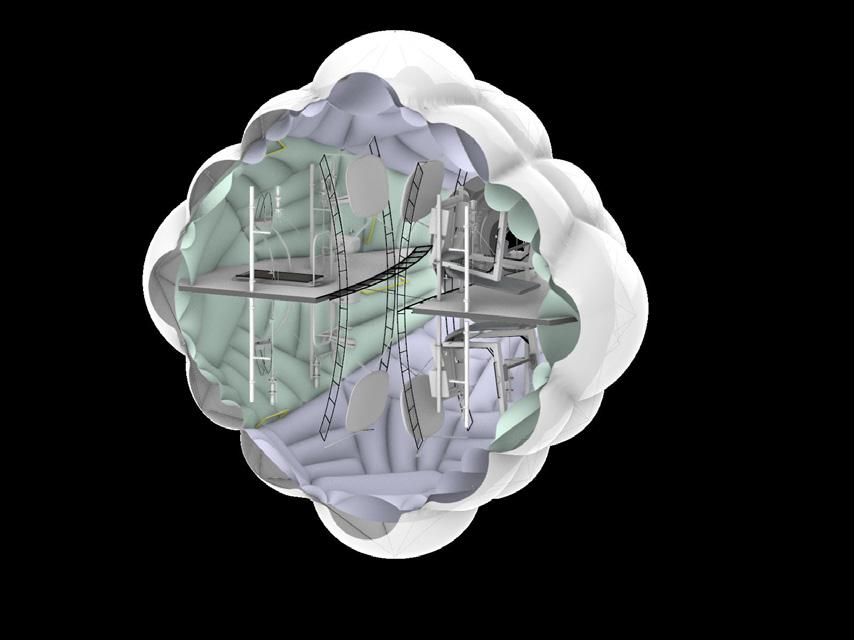
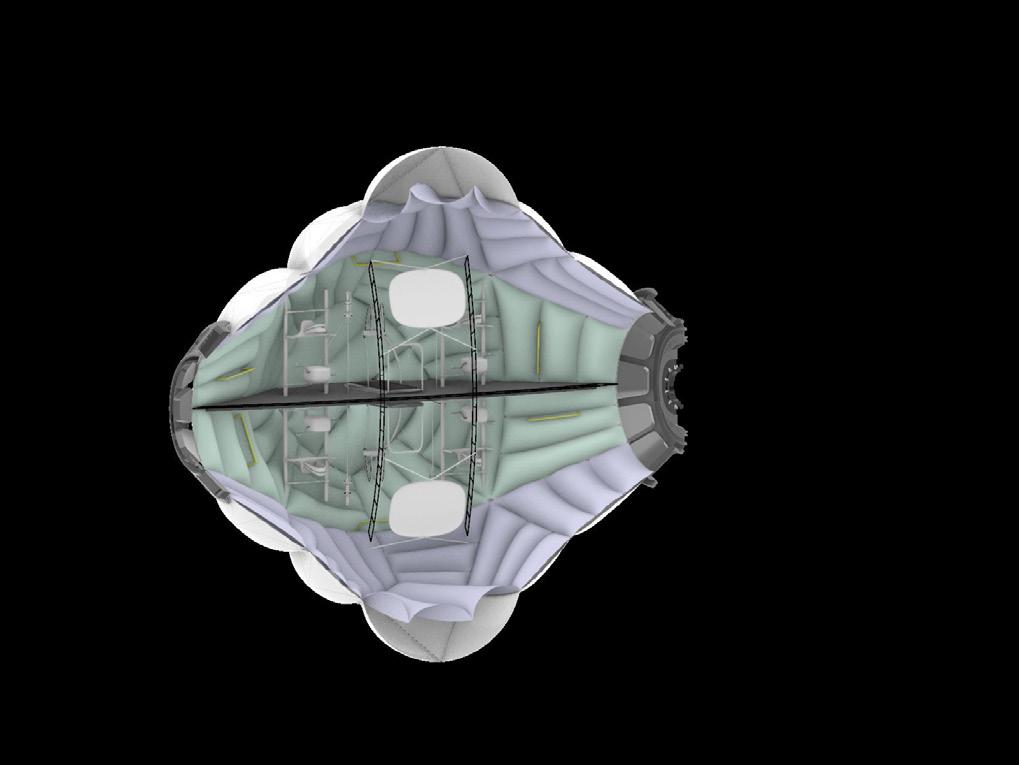
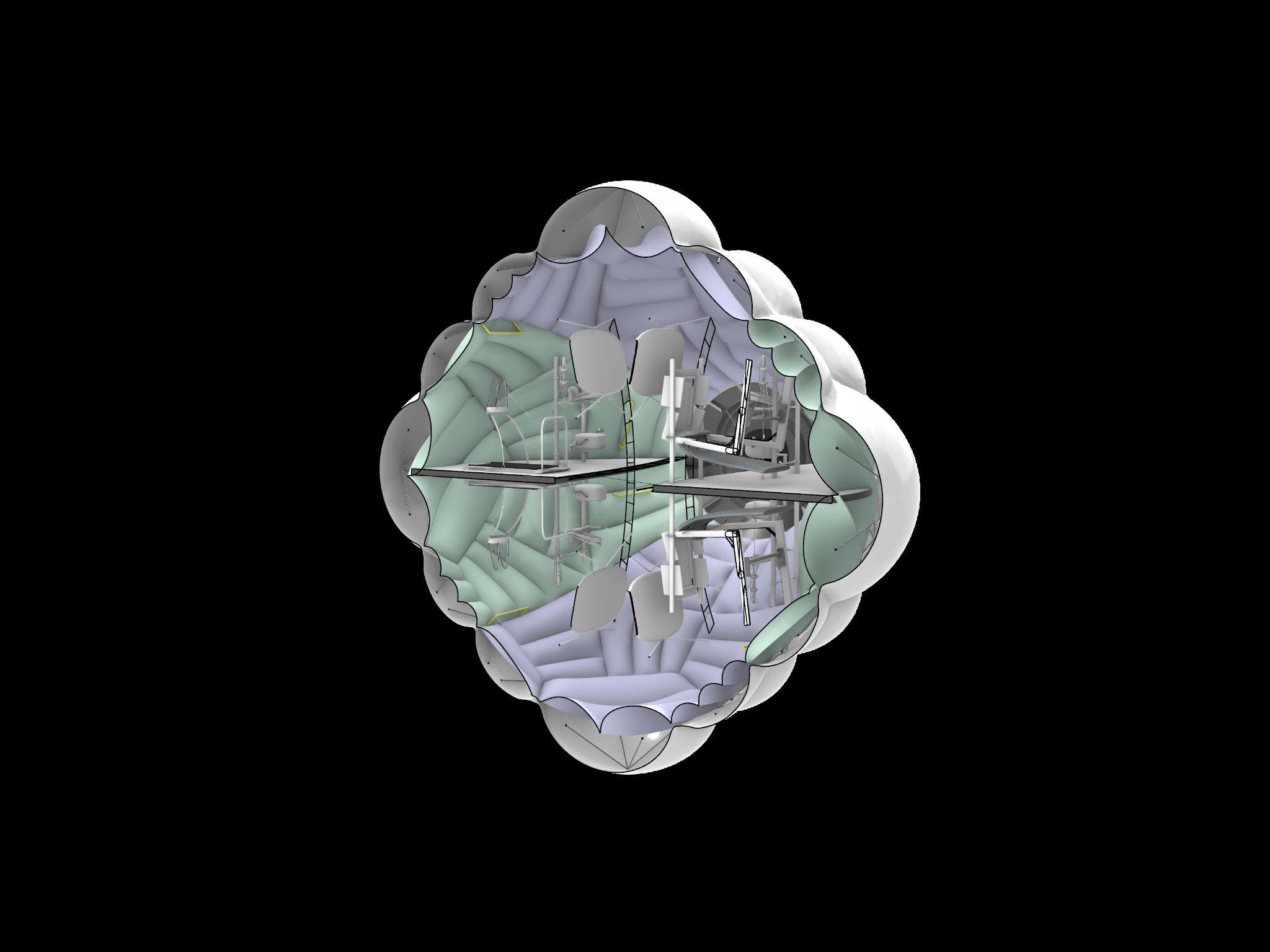
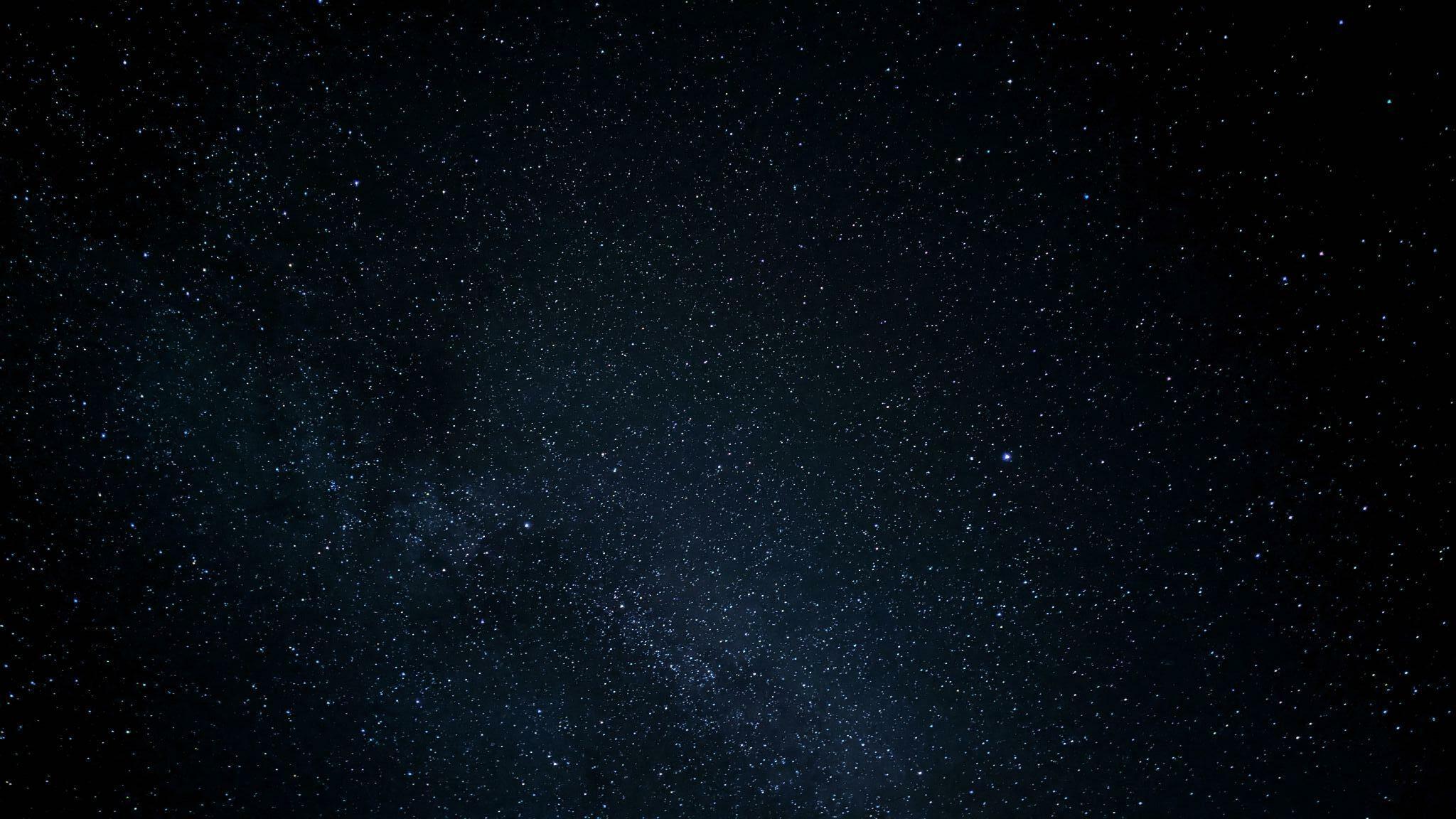
NEBULAE
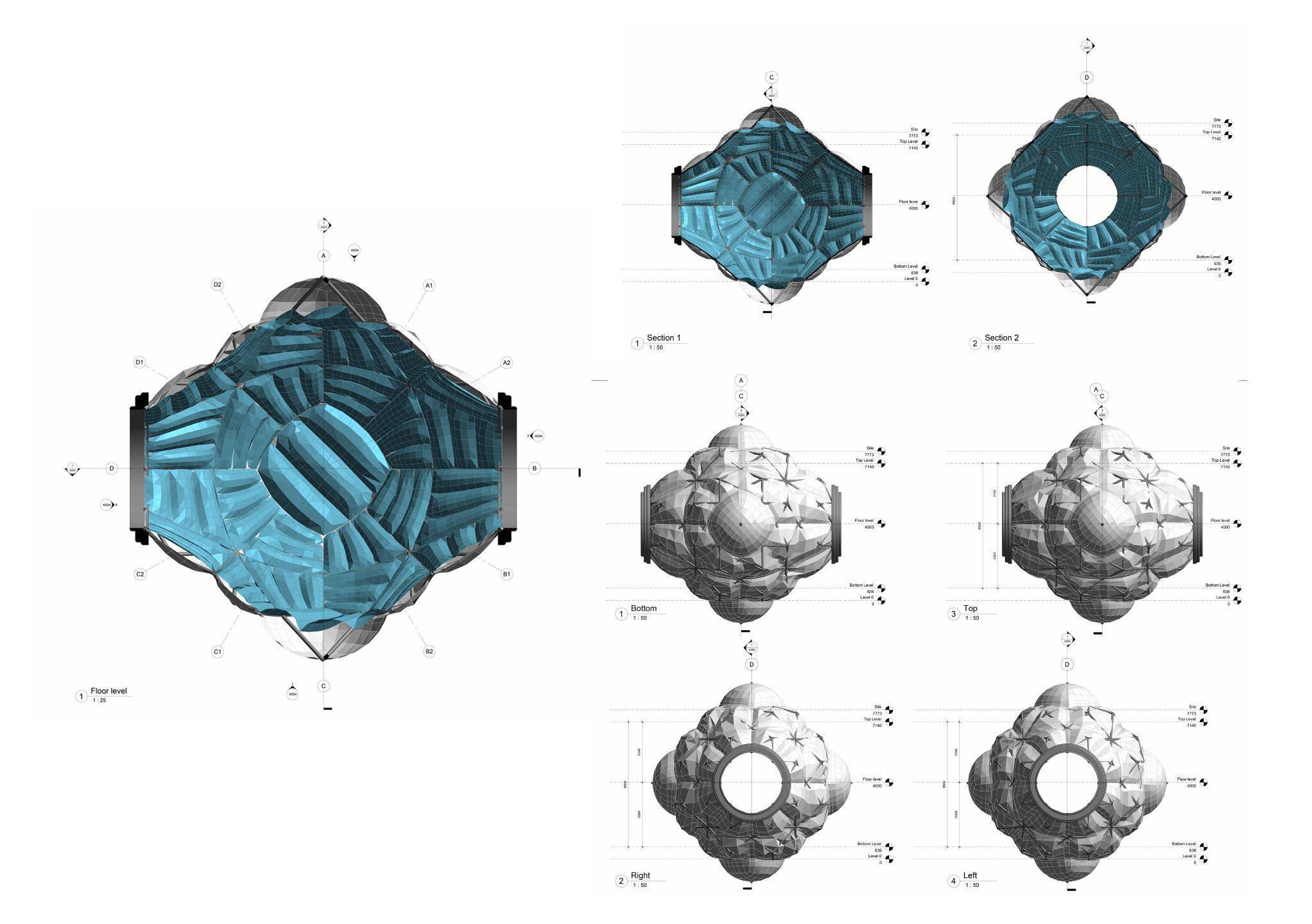
Sports Module Documentations
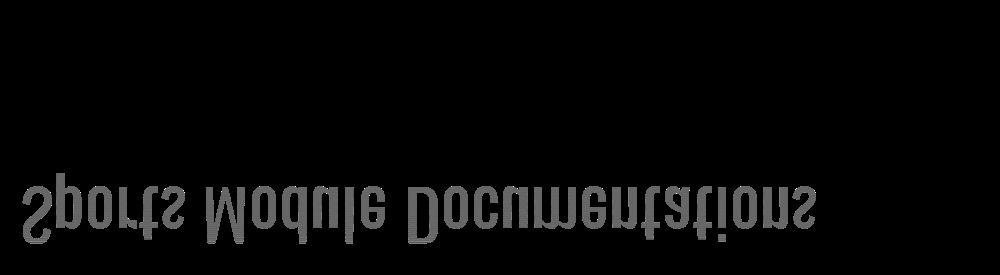
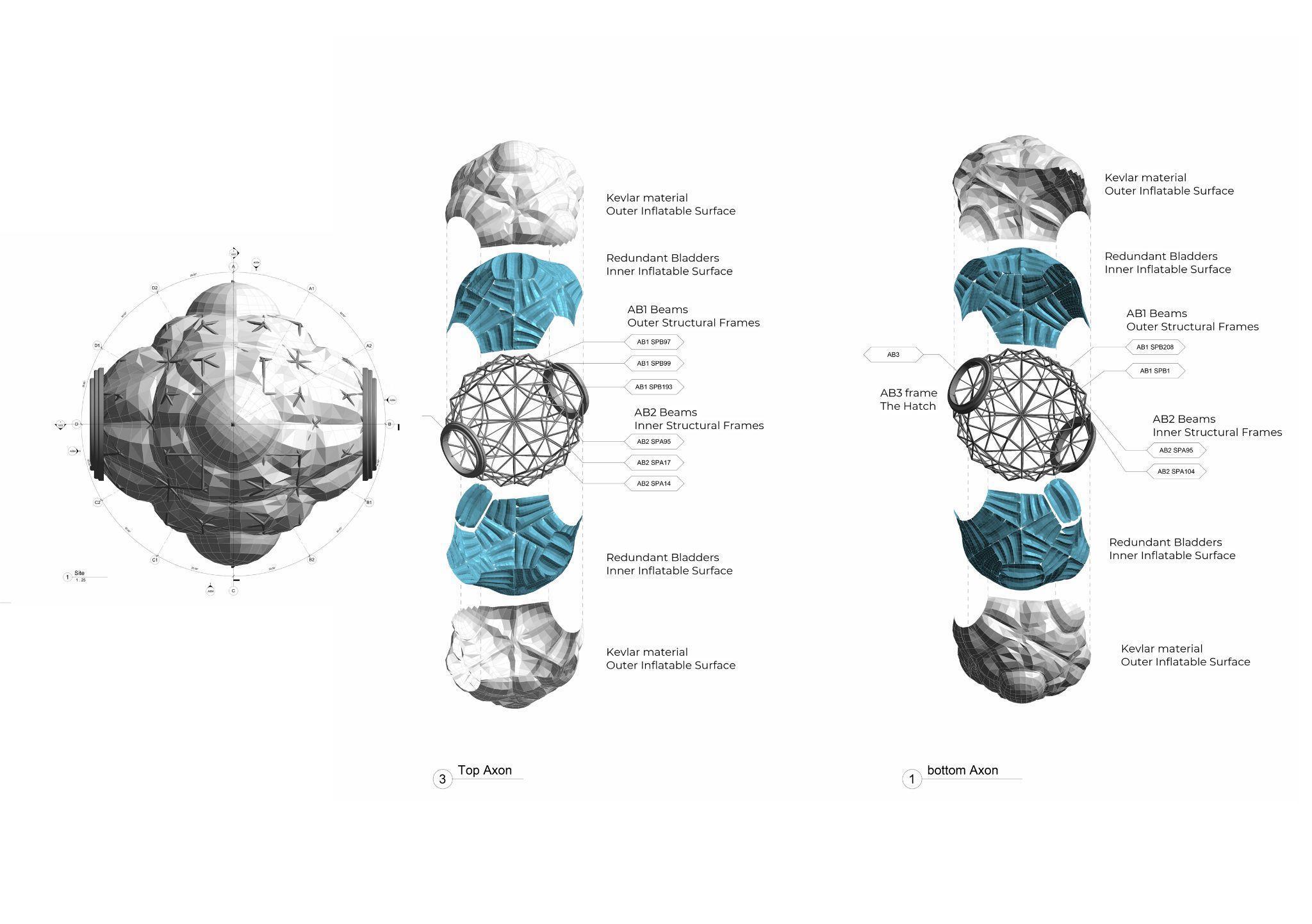
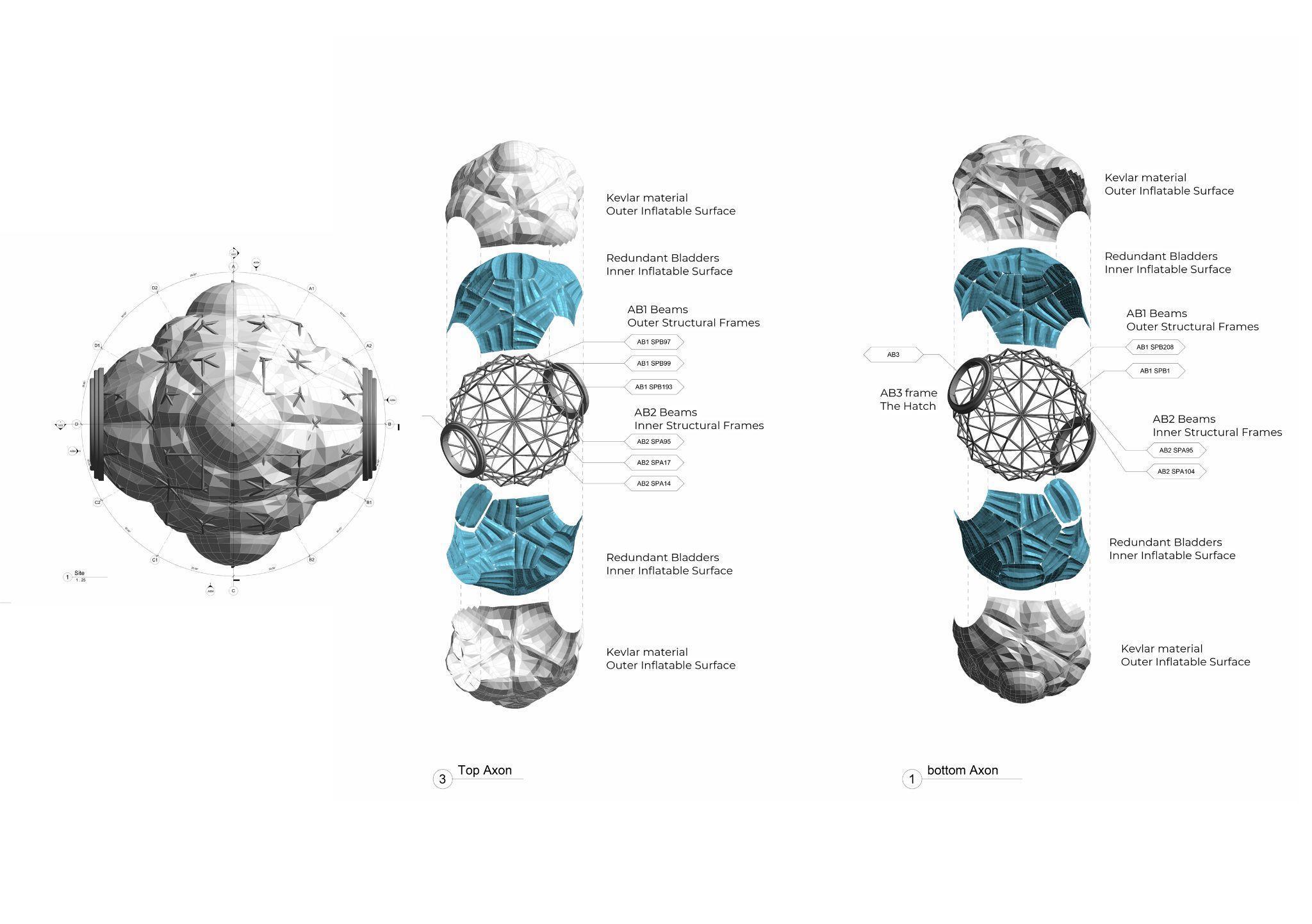
Using Revit 3D, and Rhino.inside
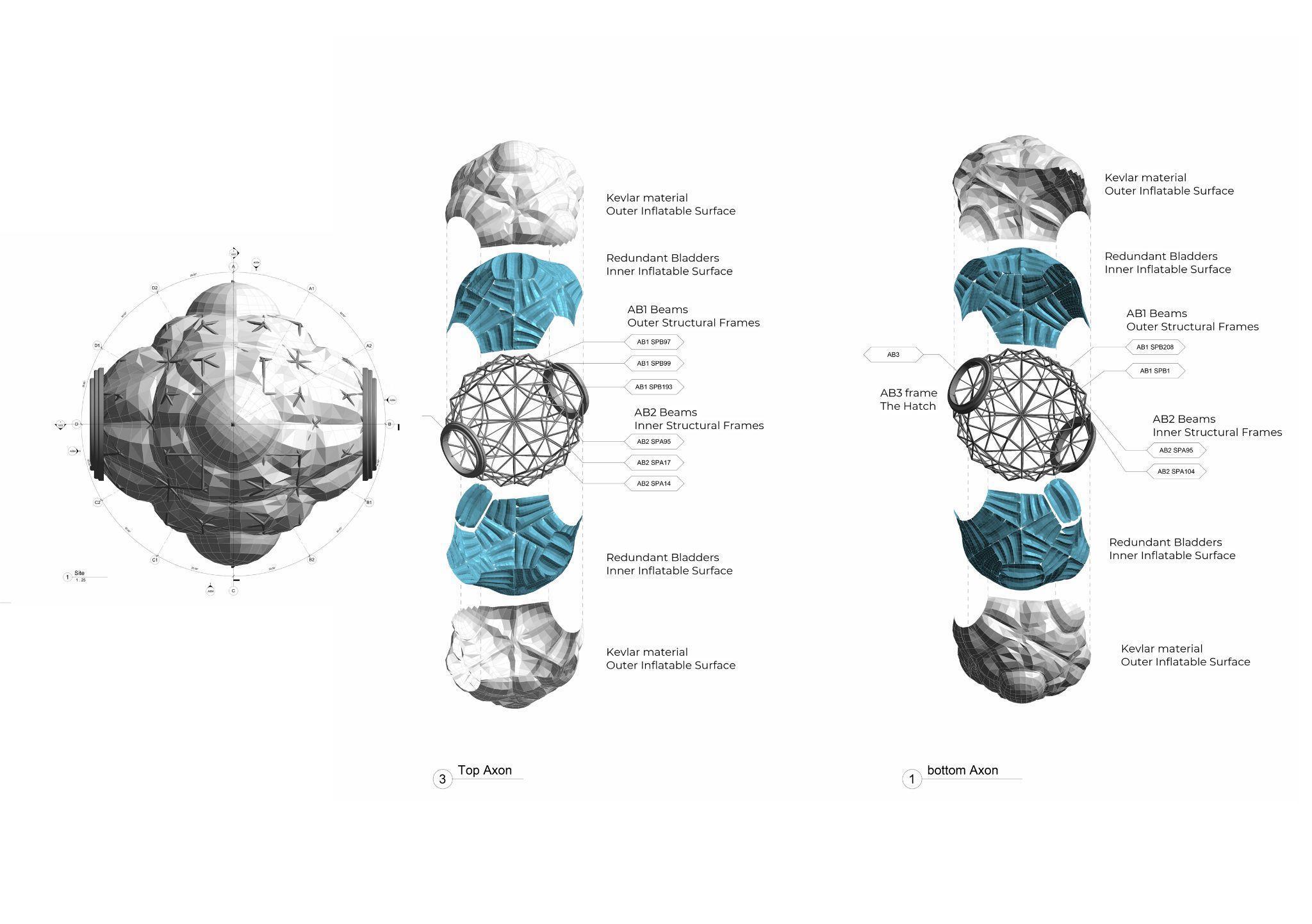
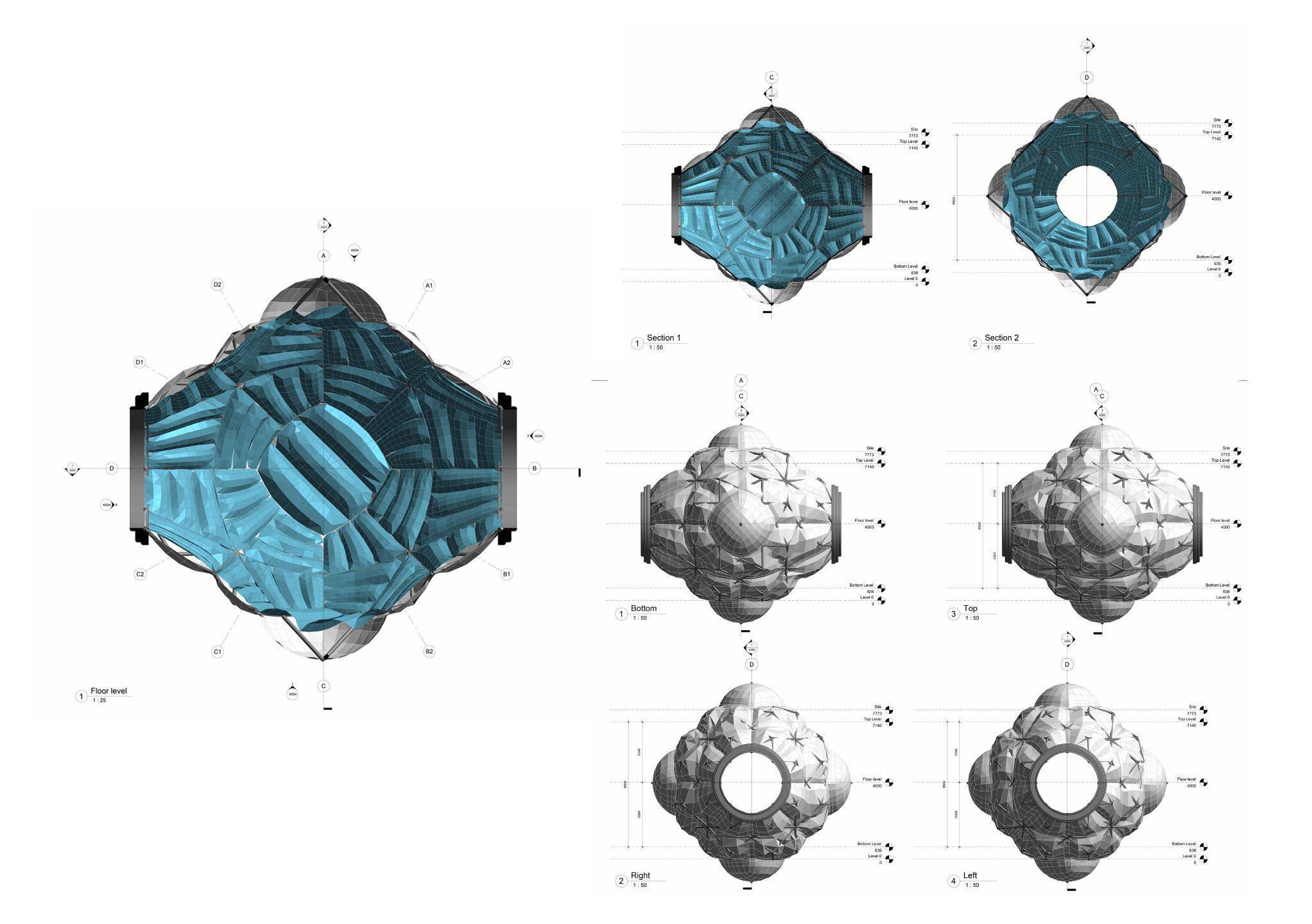
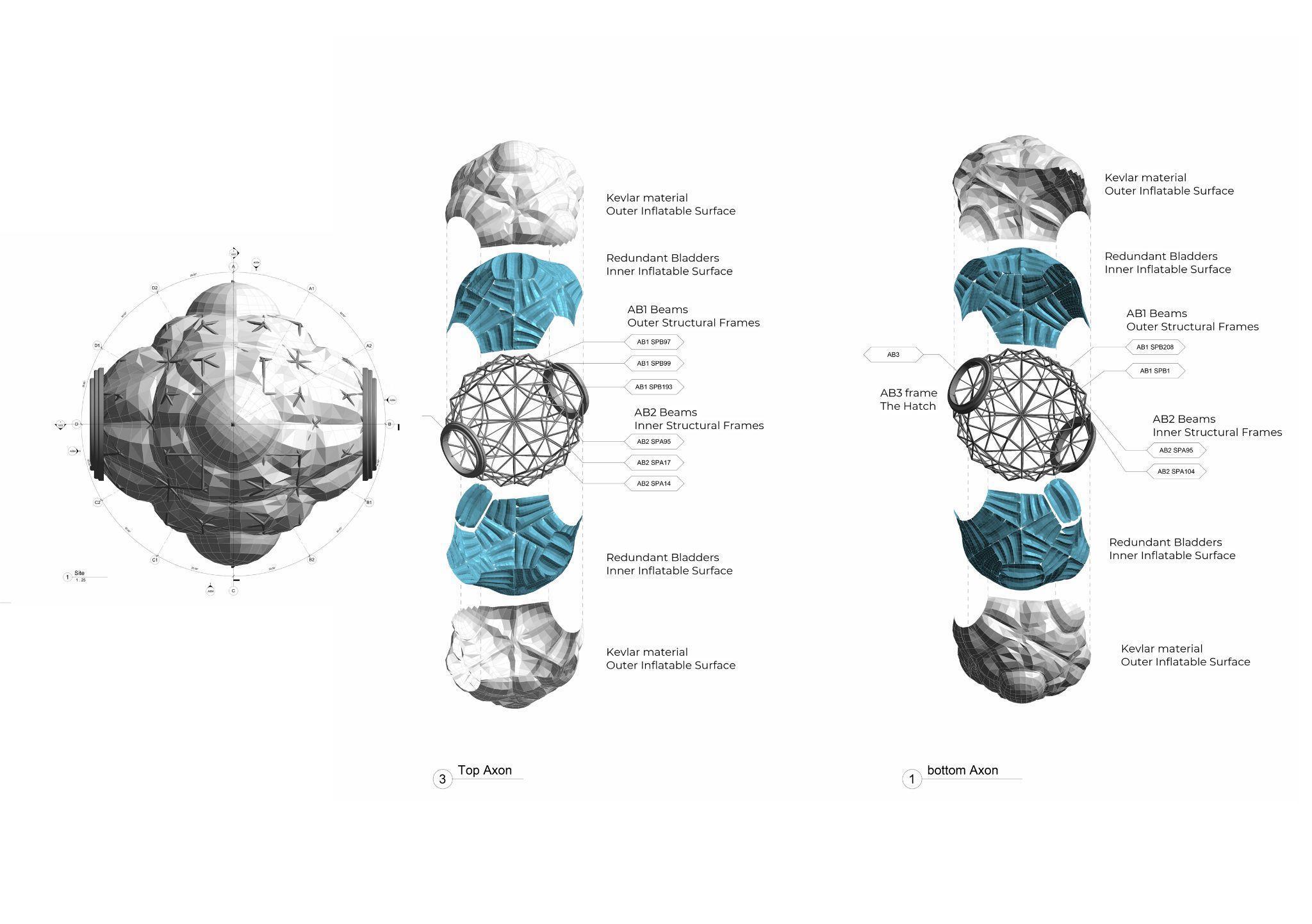
NEBULAE
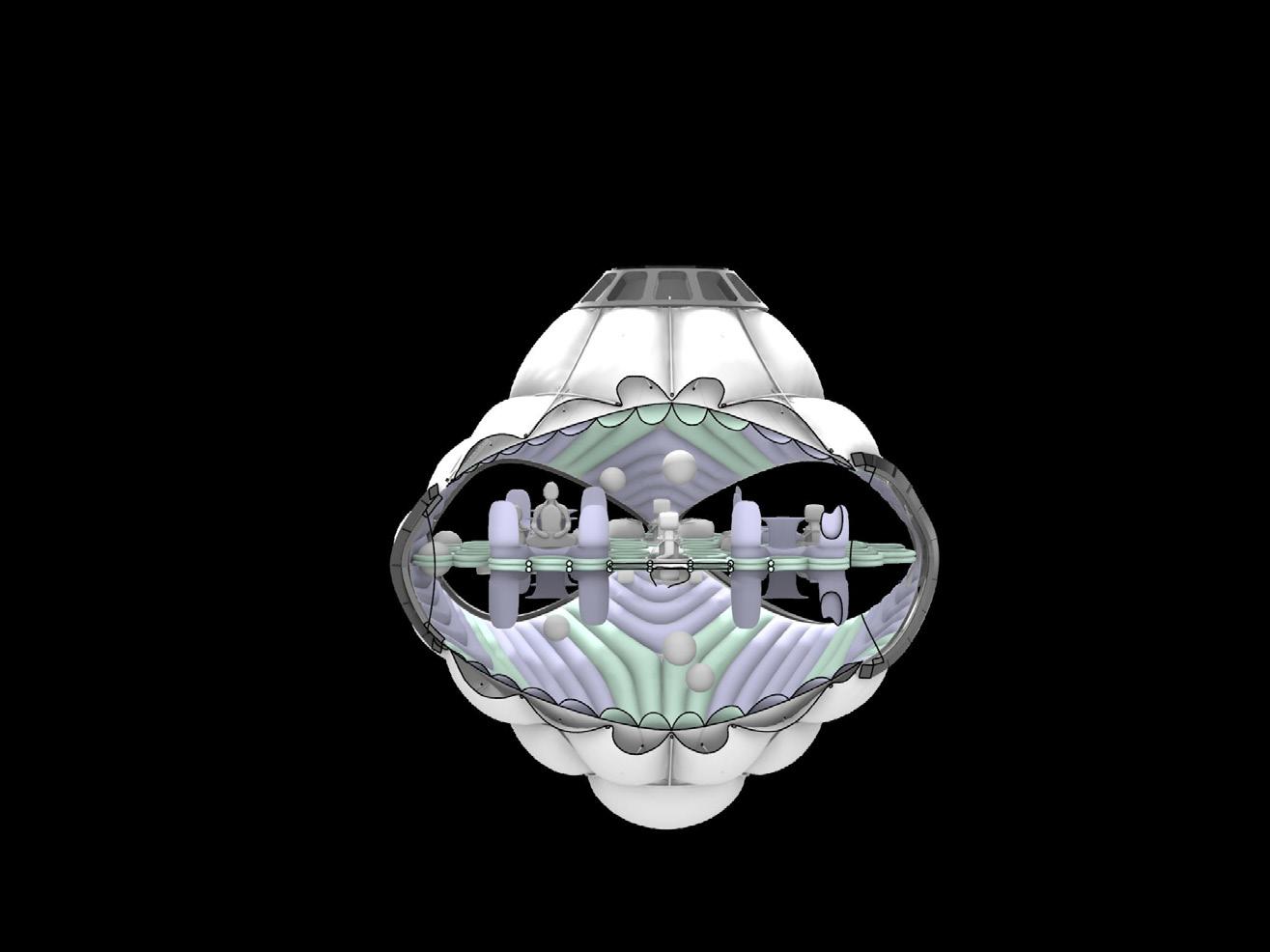
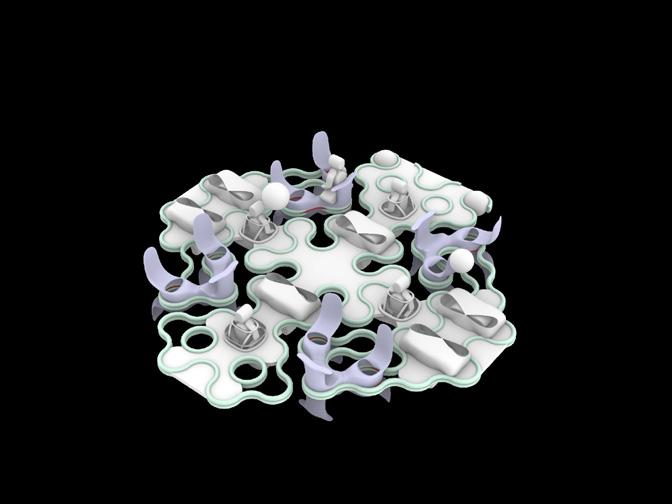
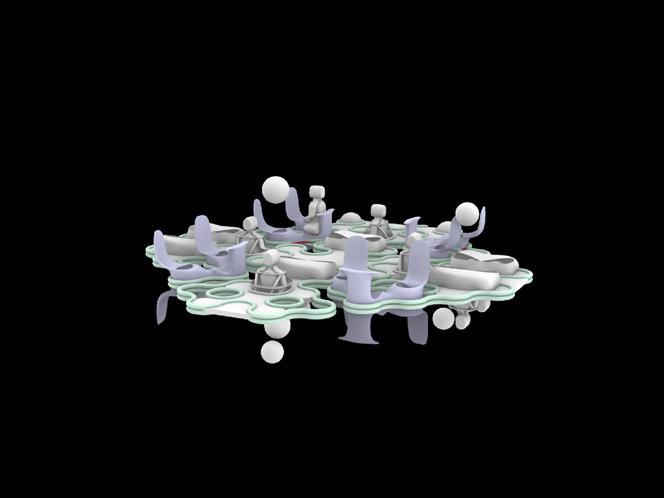
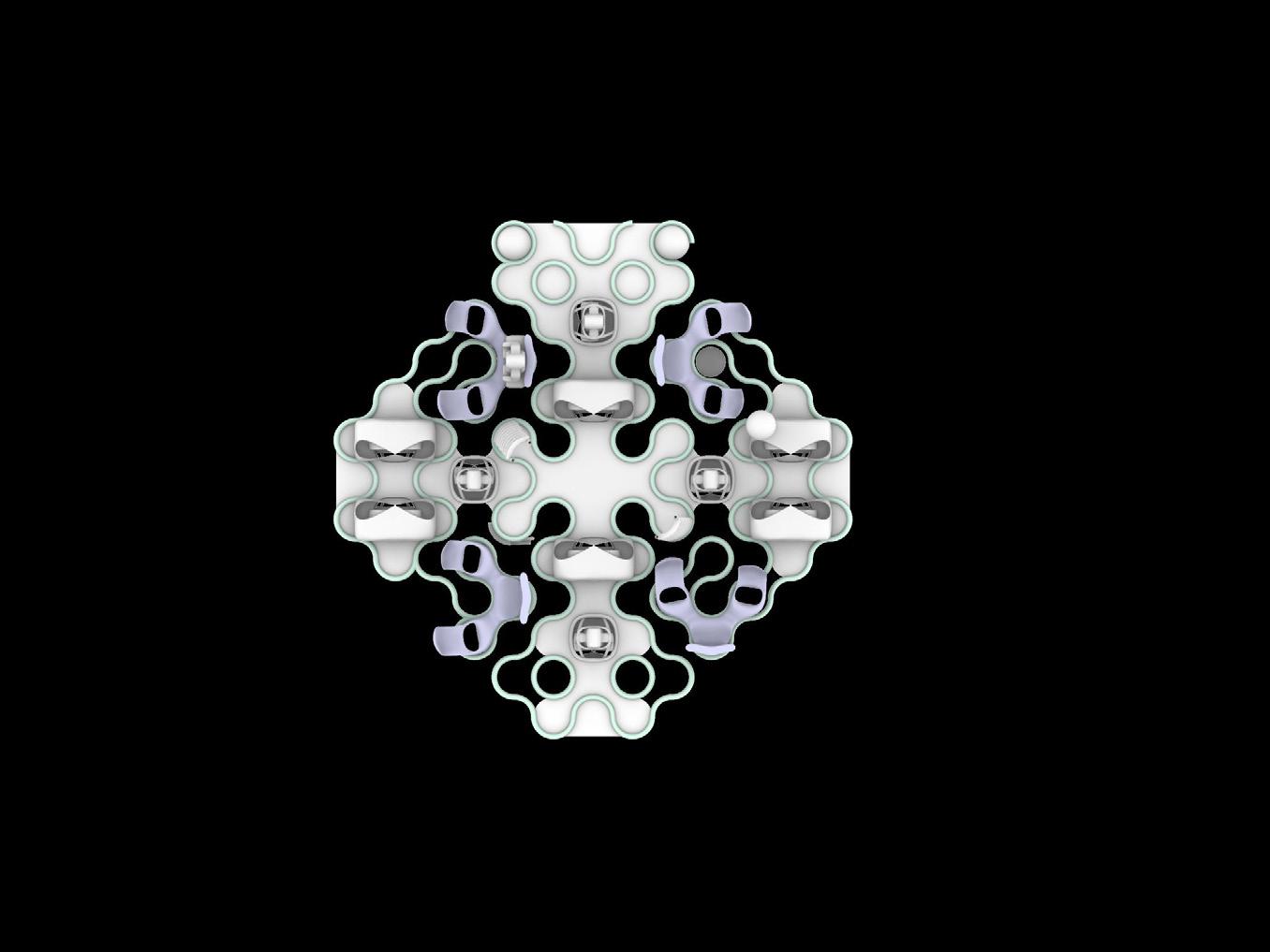
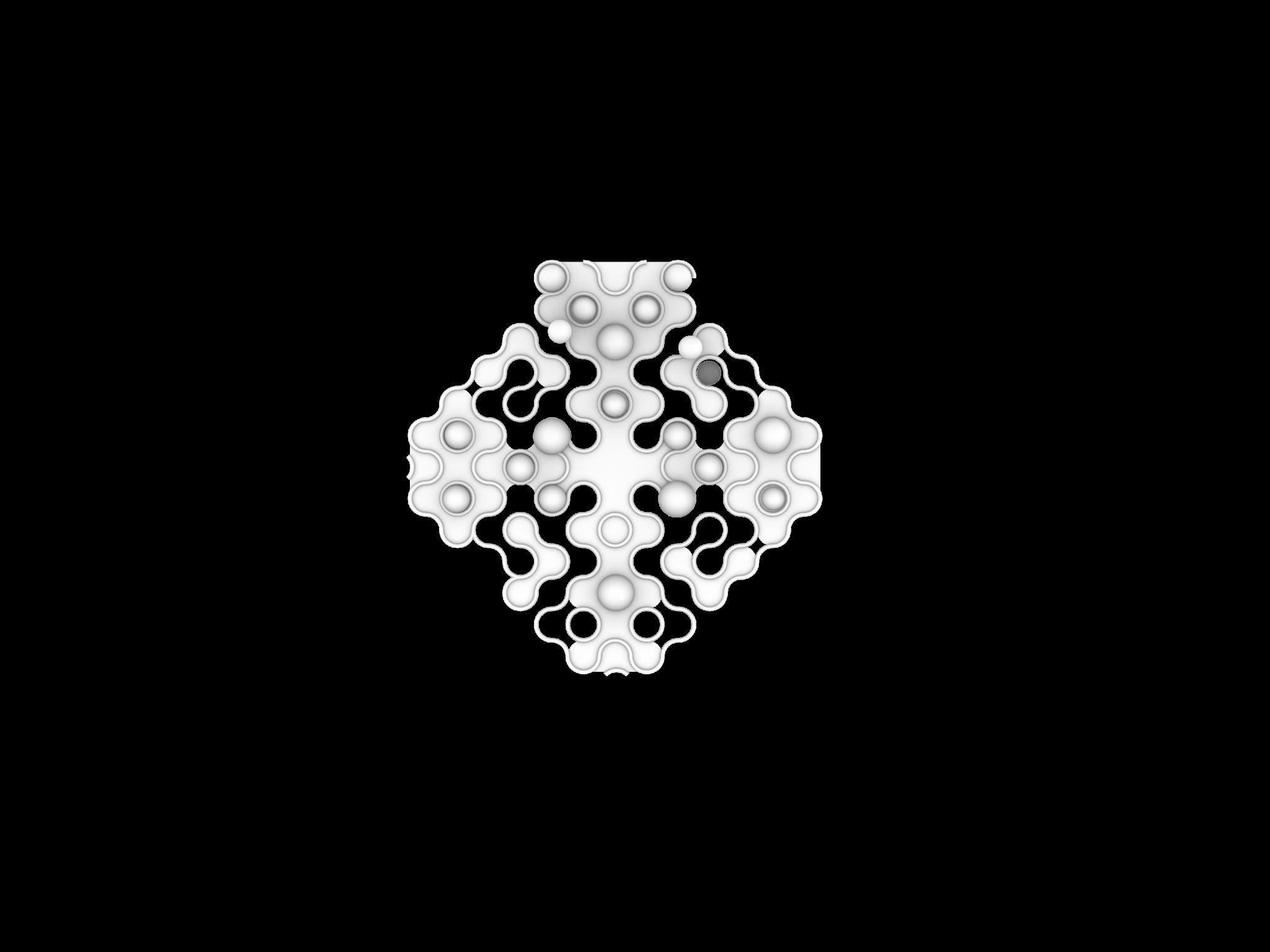
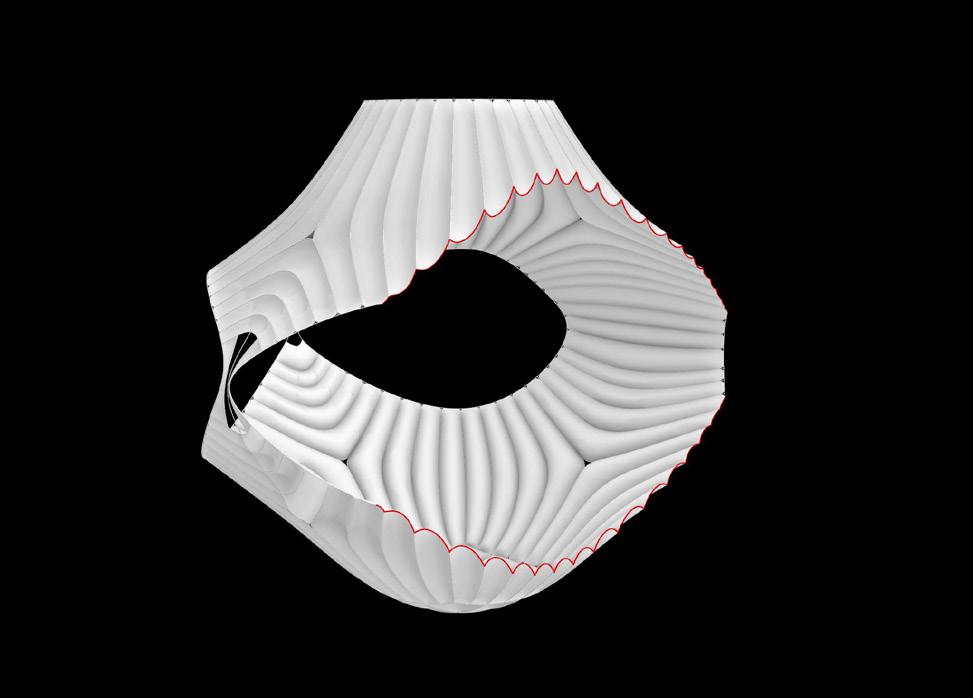
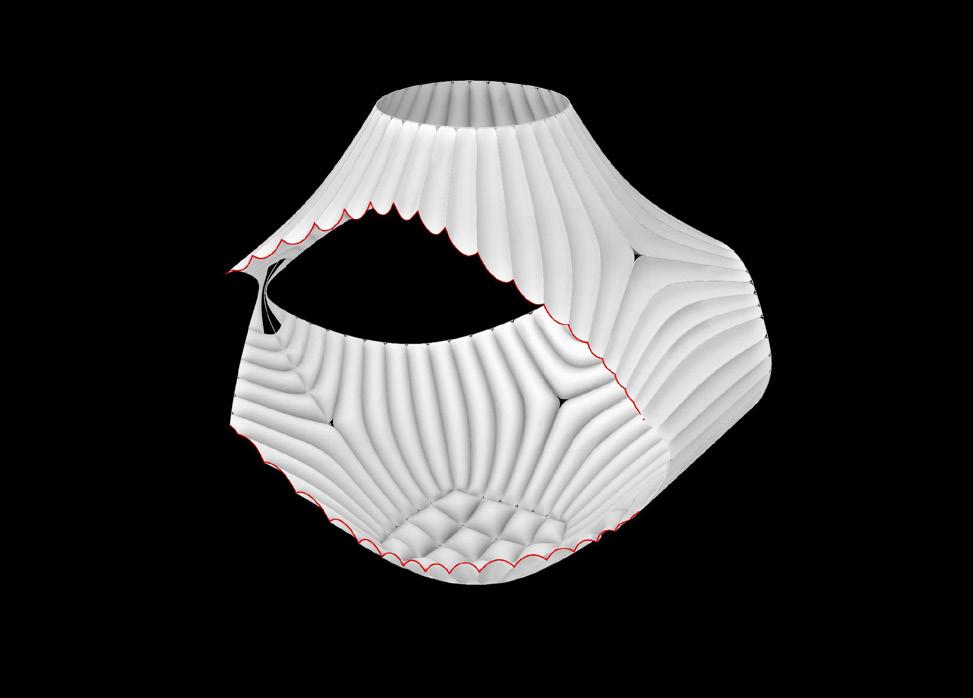
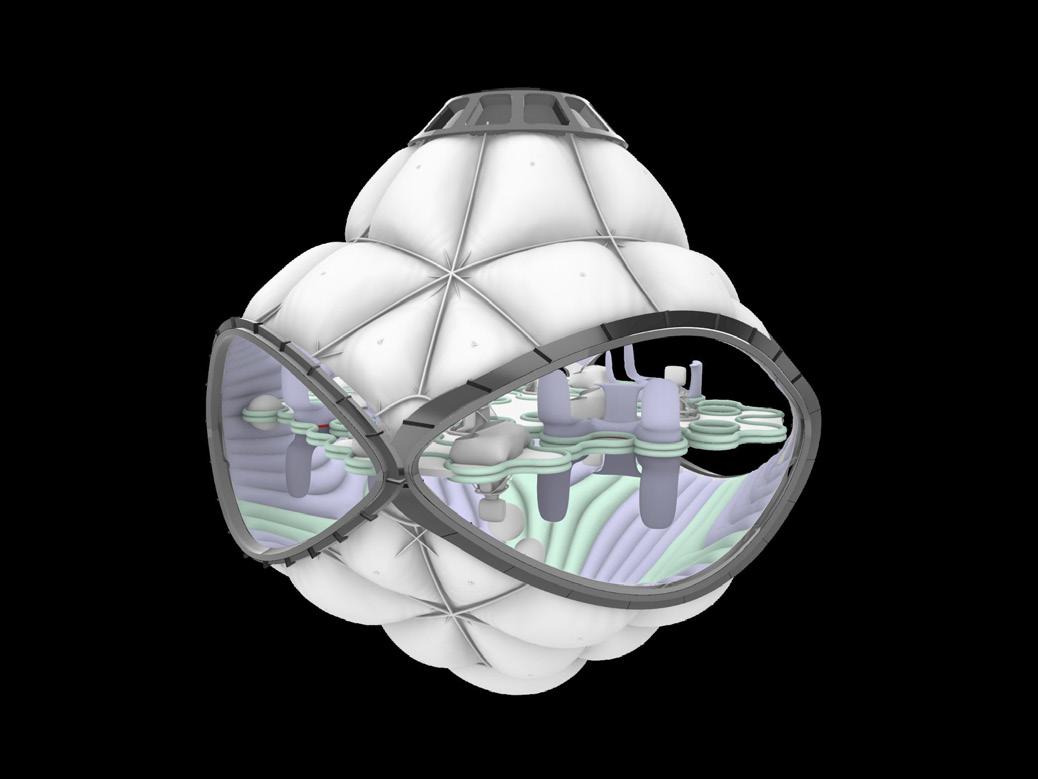
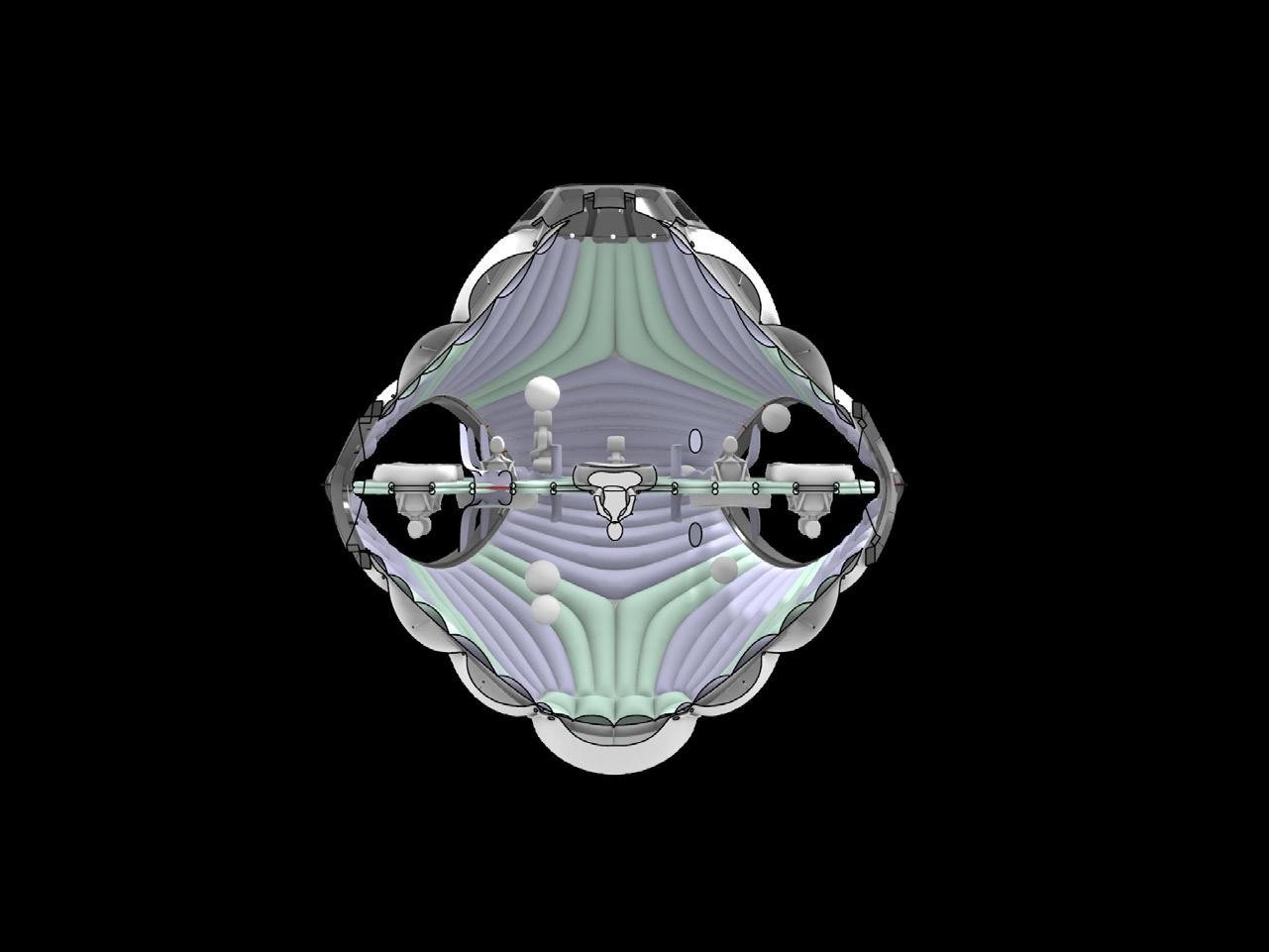
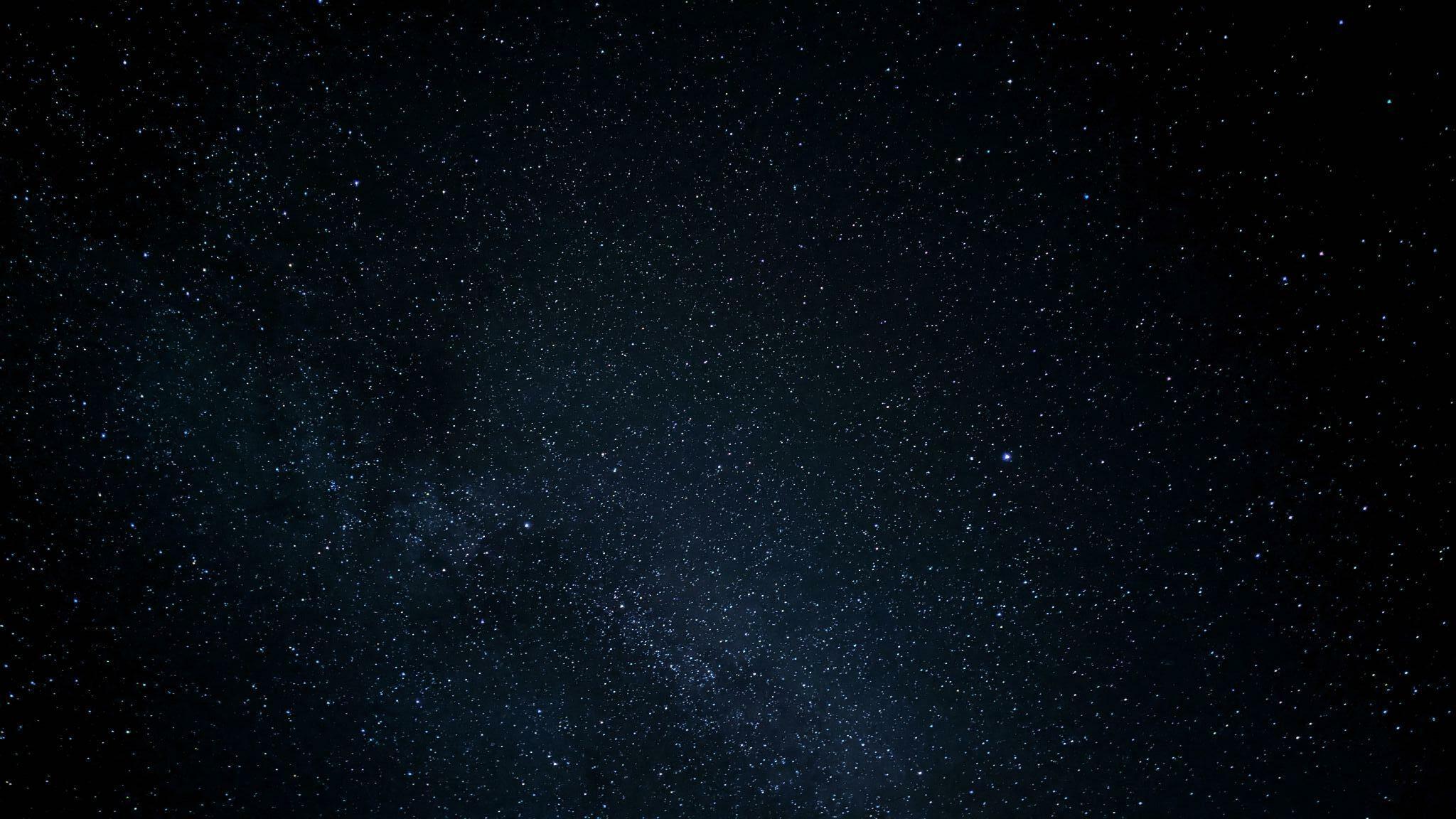
Meditation Module
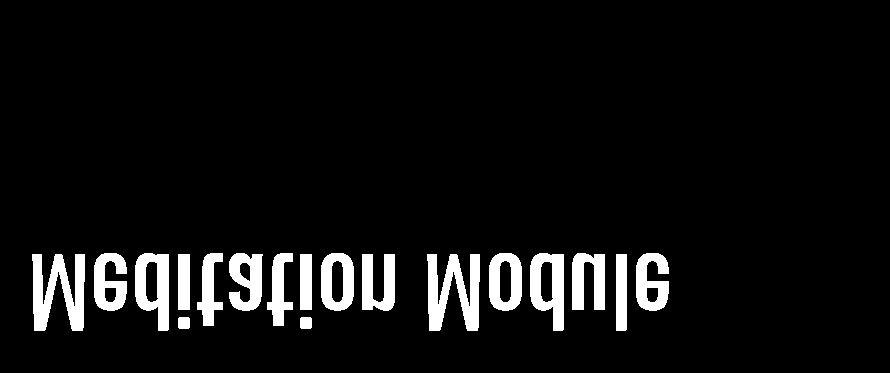
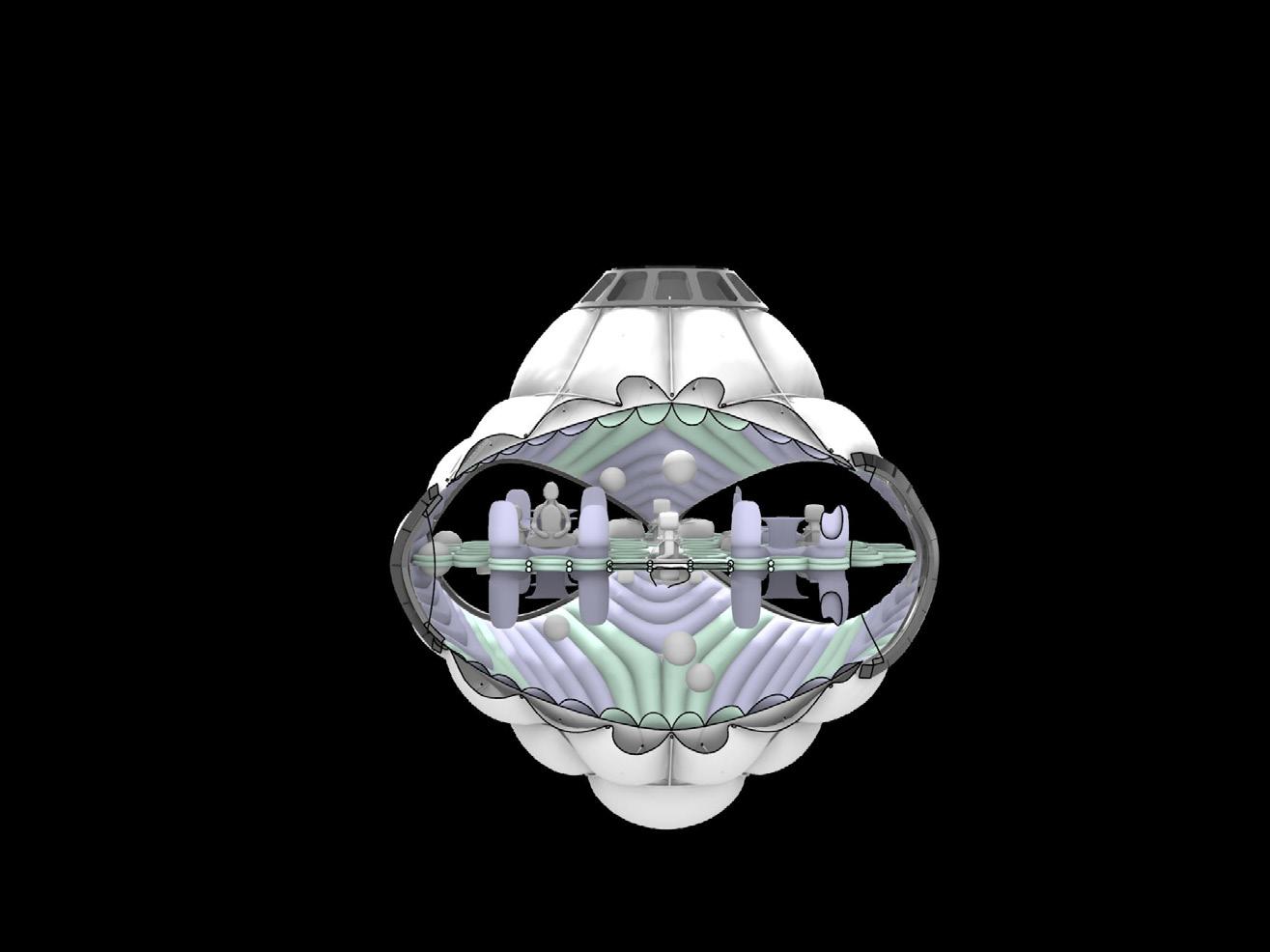
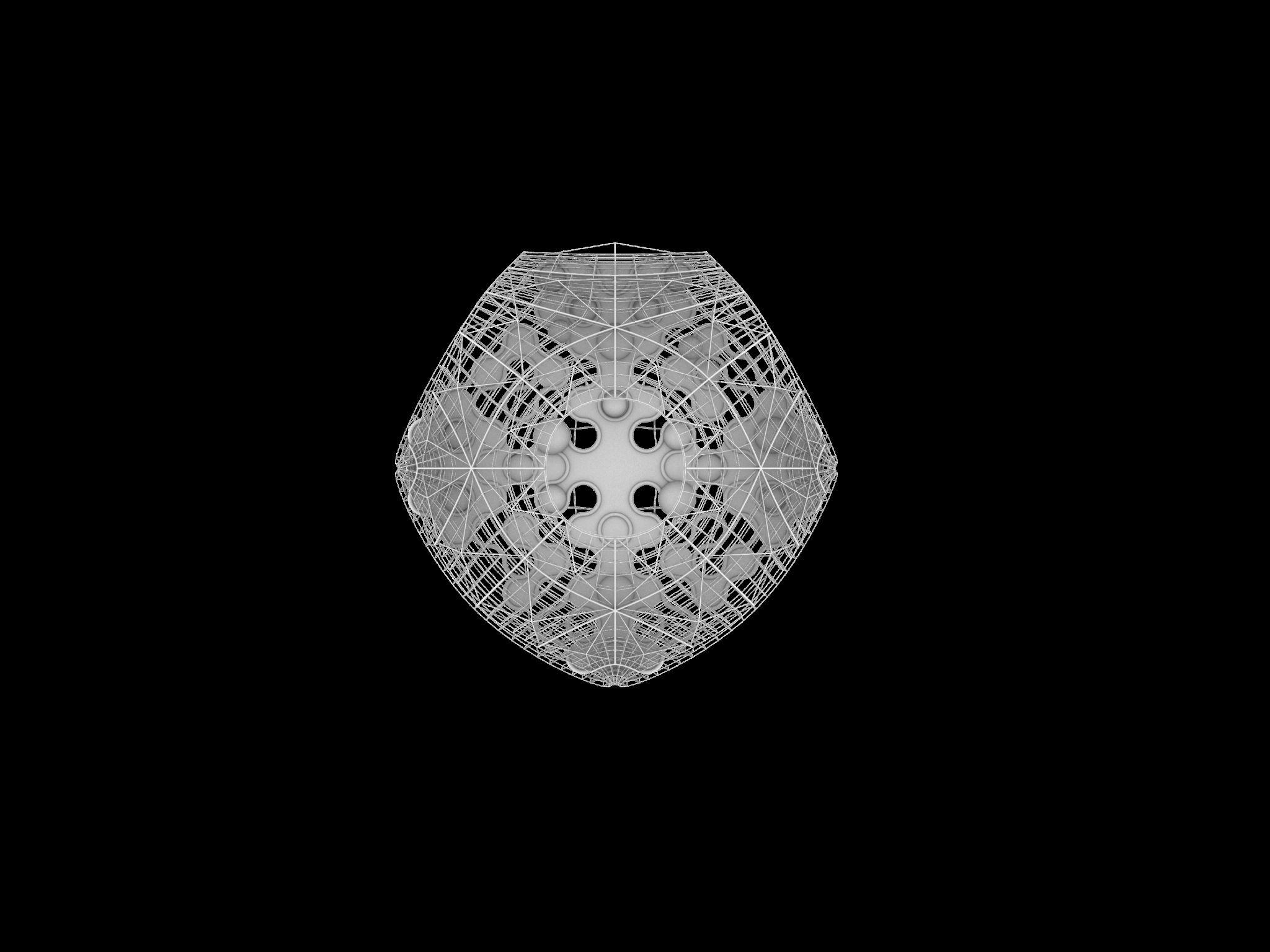
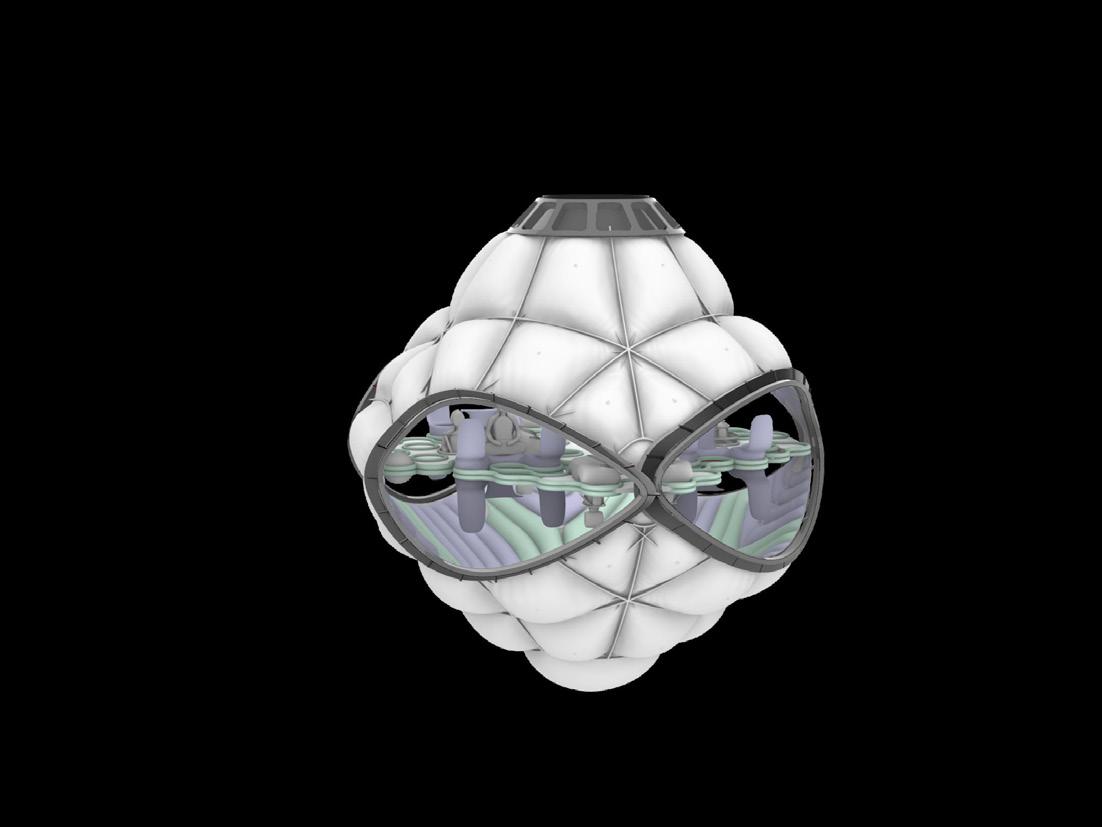
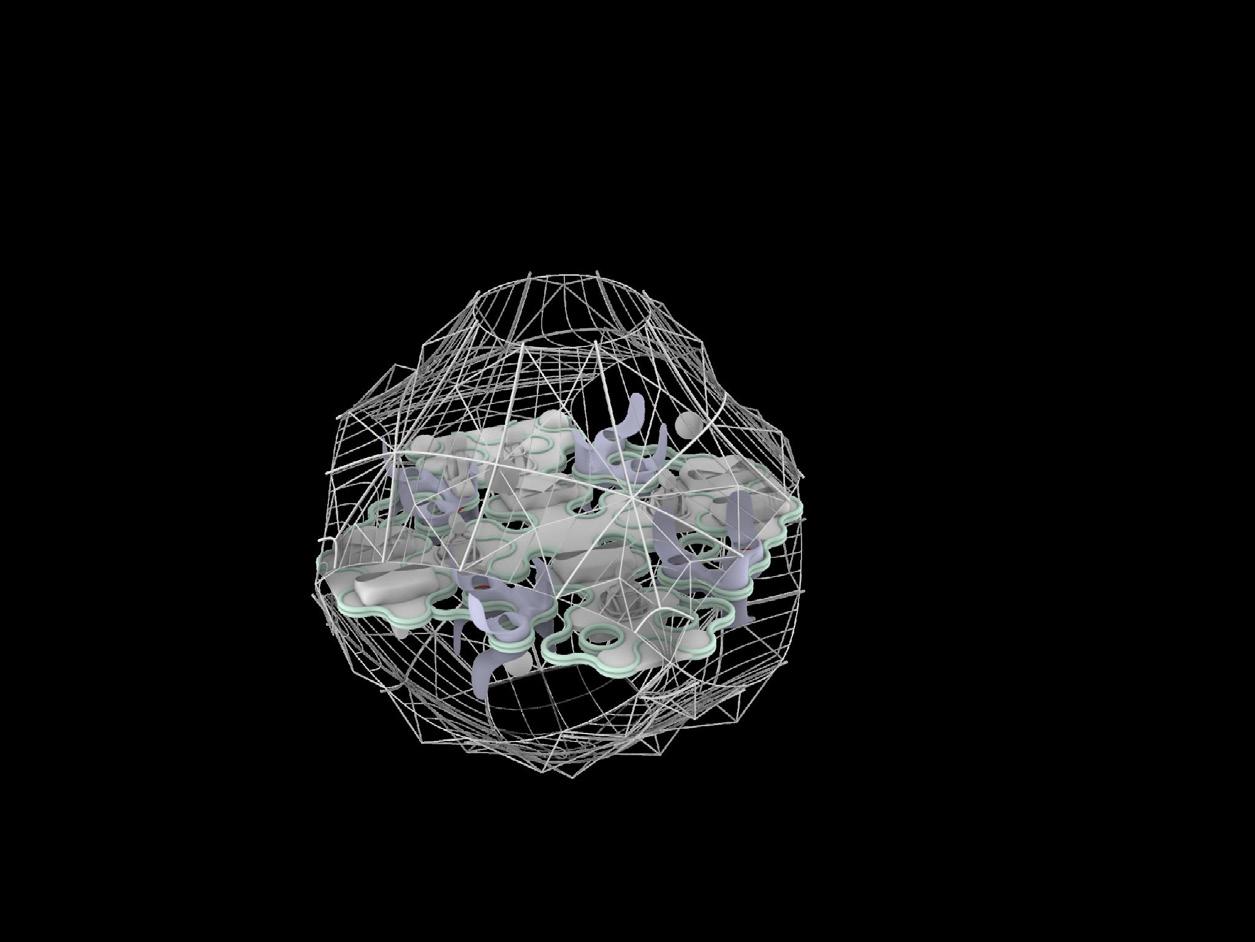
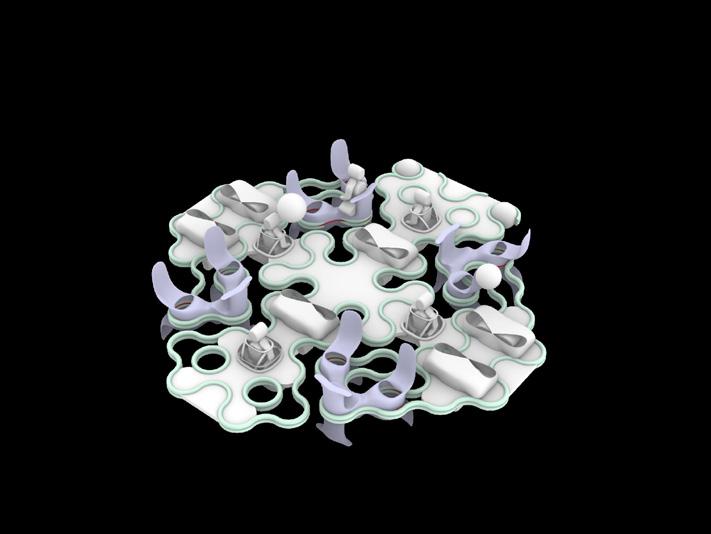
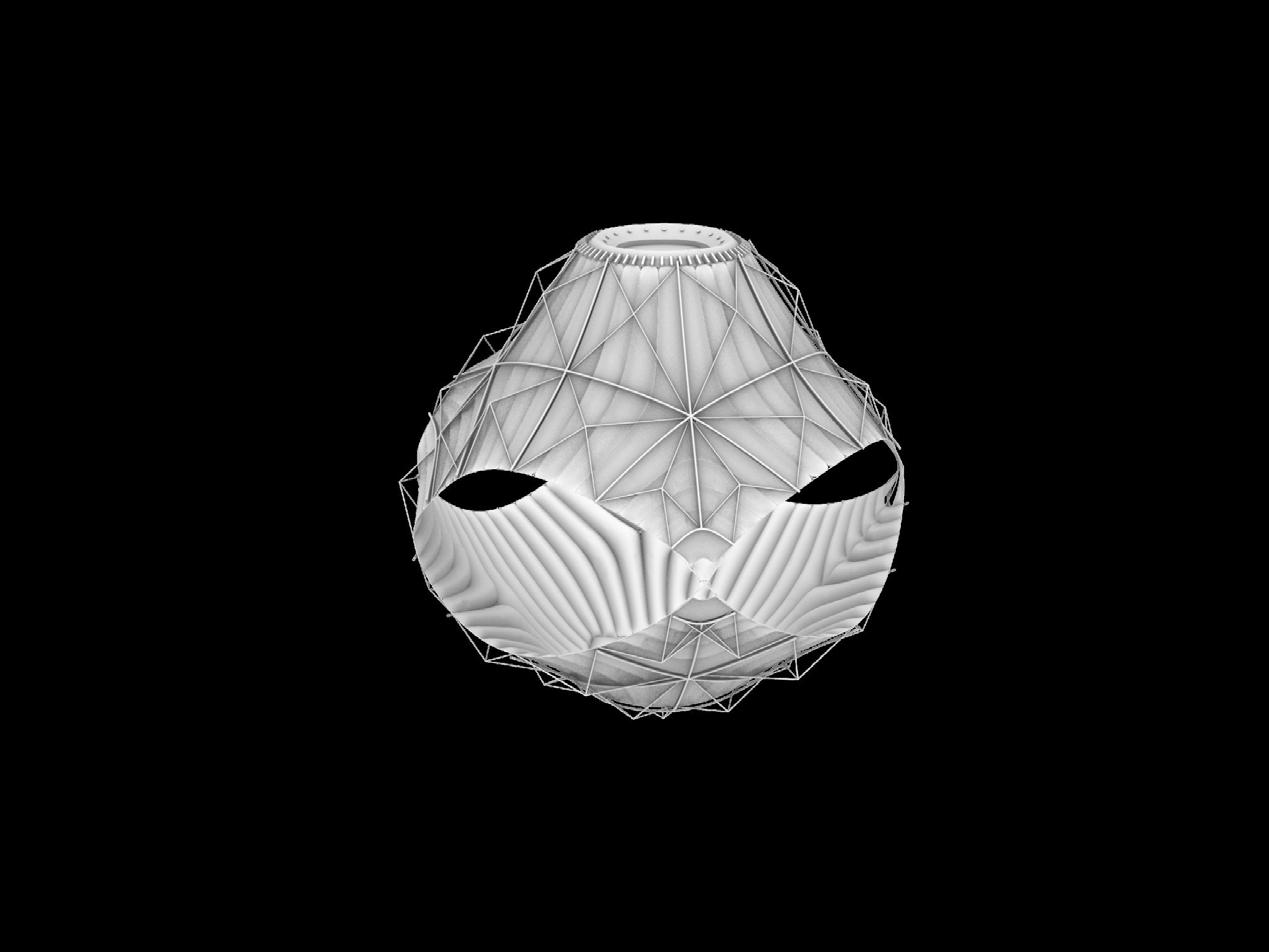
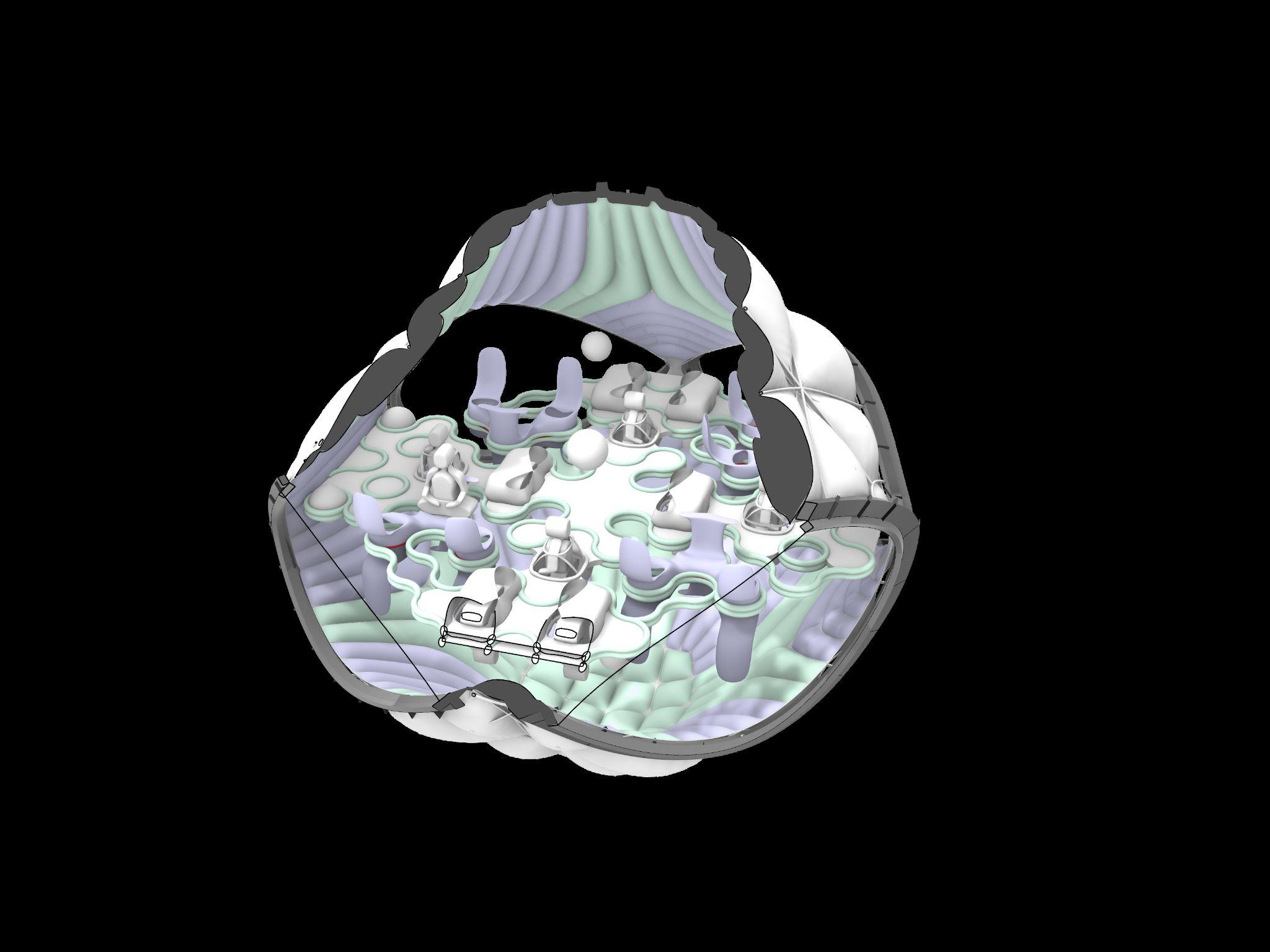
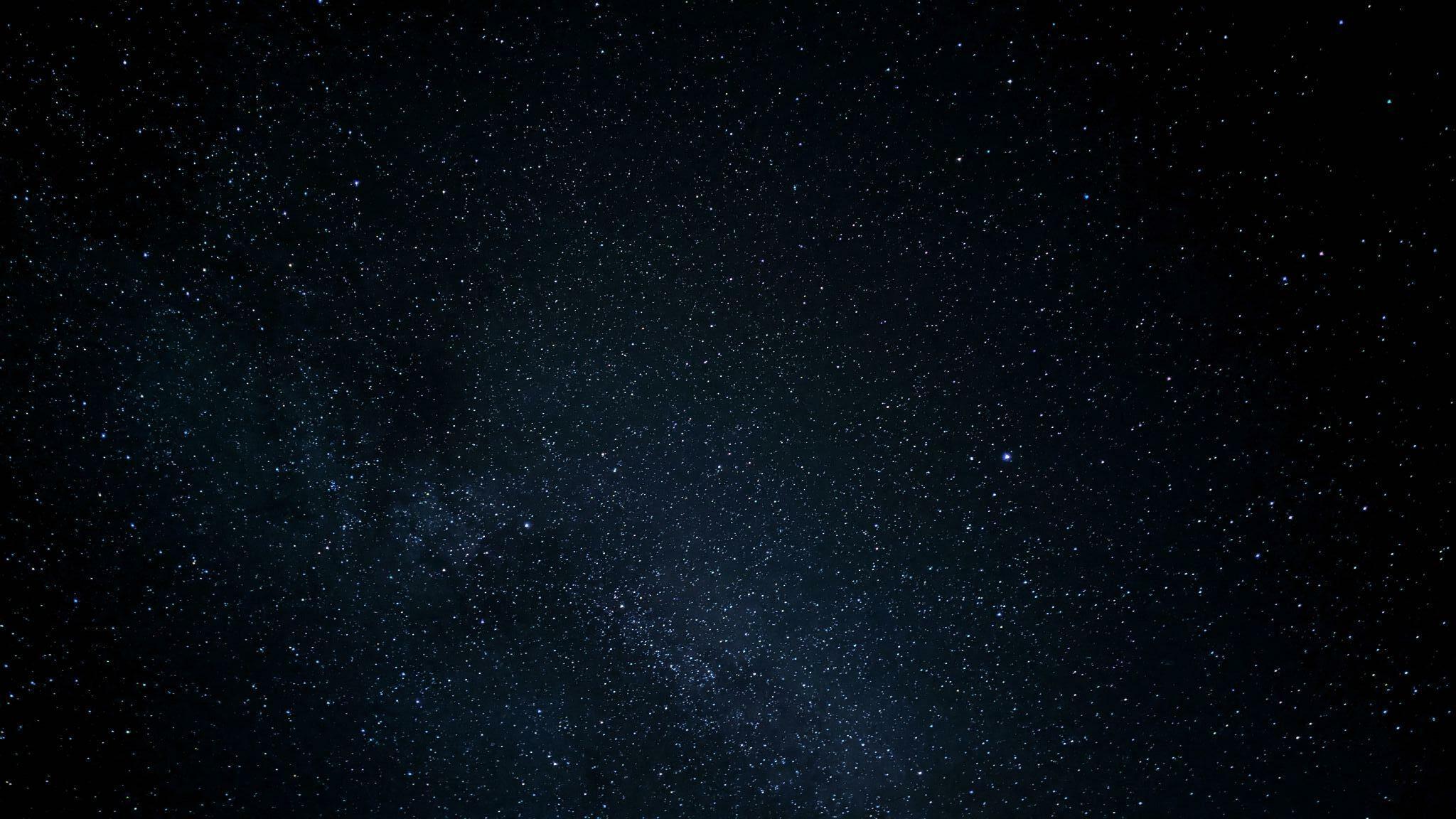
NEBULAE
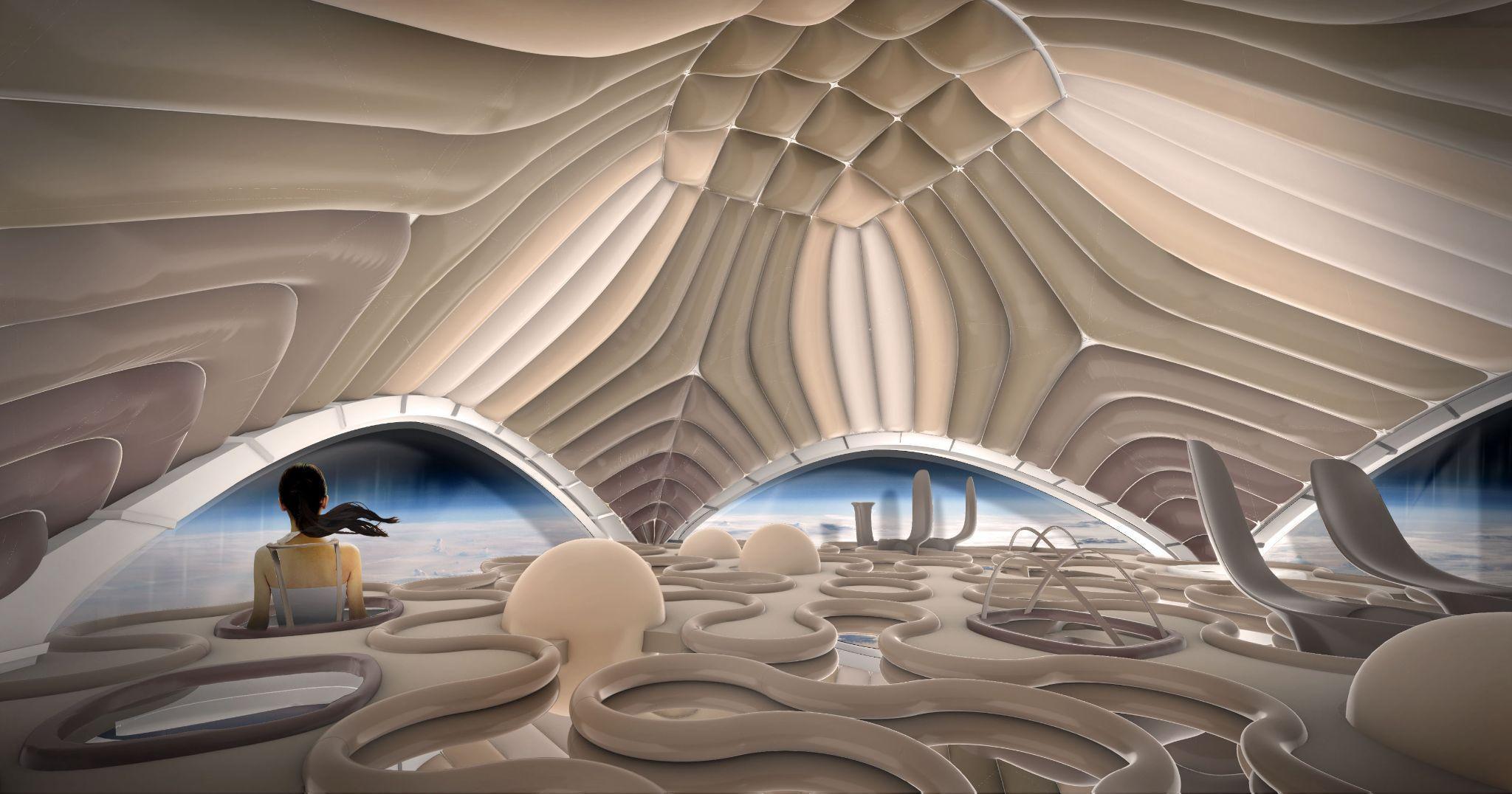
Meditation in Space
The Meditation Module presents a calm structure with soft meditation and yoga spaces suitable for microgravity. The interior pattern mimics a fluid and calm environment. The interior configuration is mirrored, using both sides of the module. The colour of the patterns divides the ground and sky perspective by introducing colours to the patterns, representing a warm environment. It is based on the isolation module with bigger openings for space interaction.
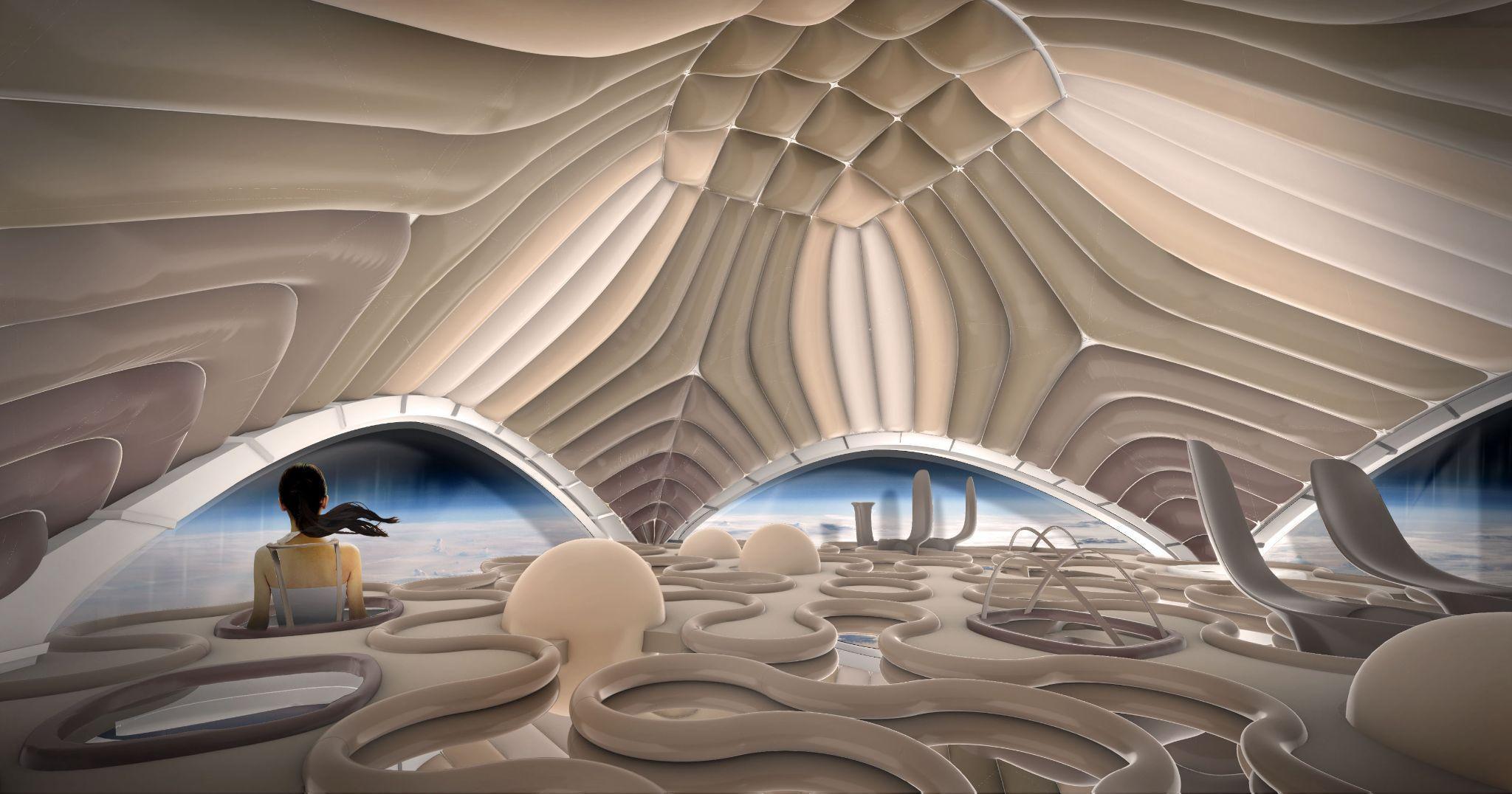
NEBULAE Meditation Module Documentations
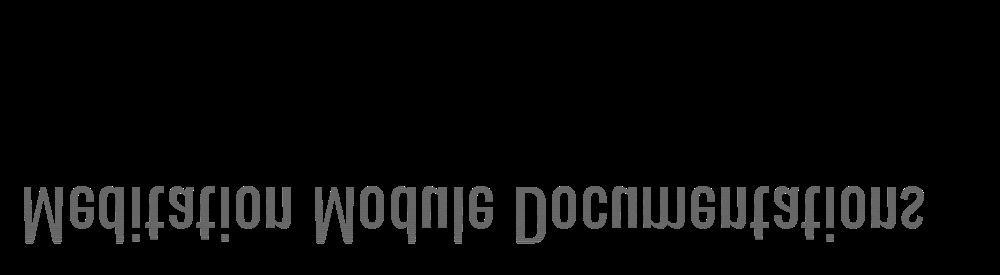
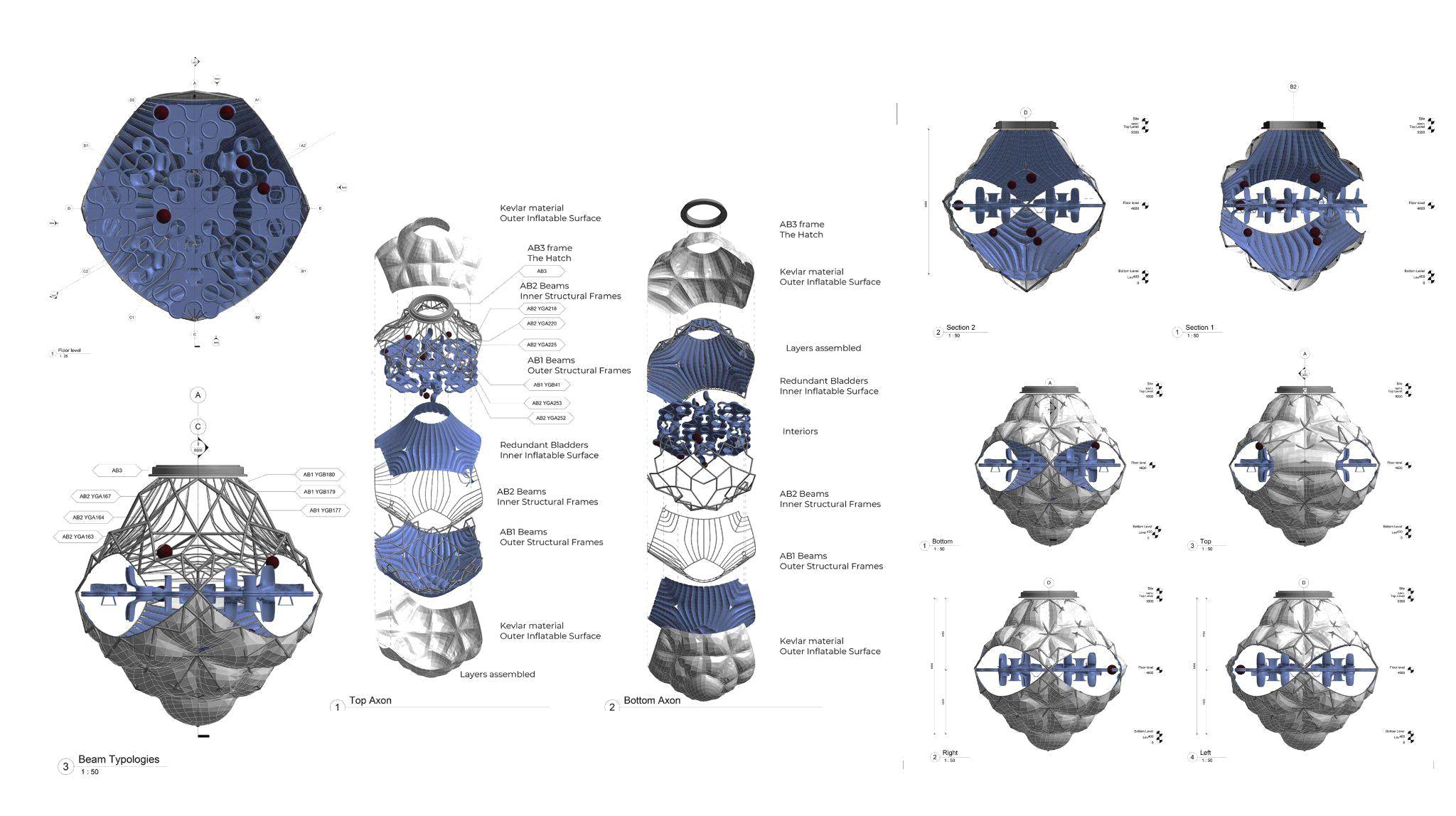
Using Revit 3D, and Rhino.inside
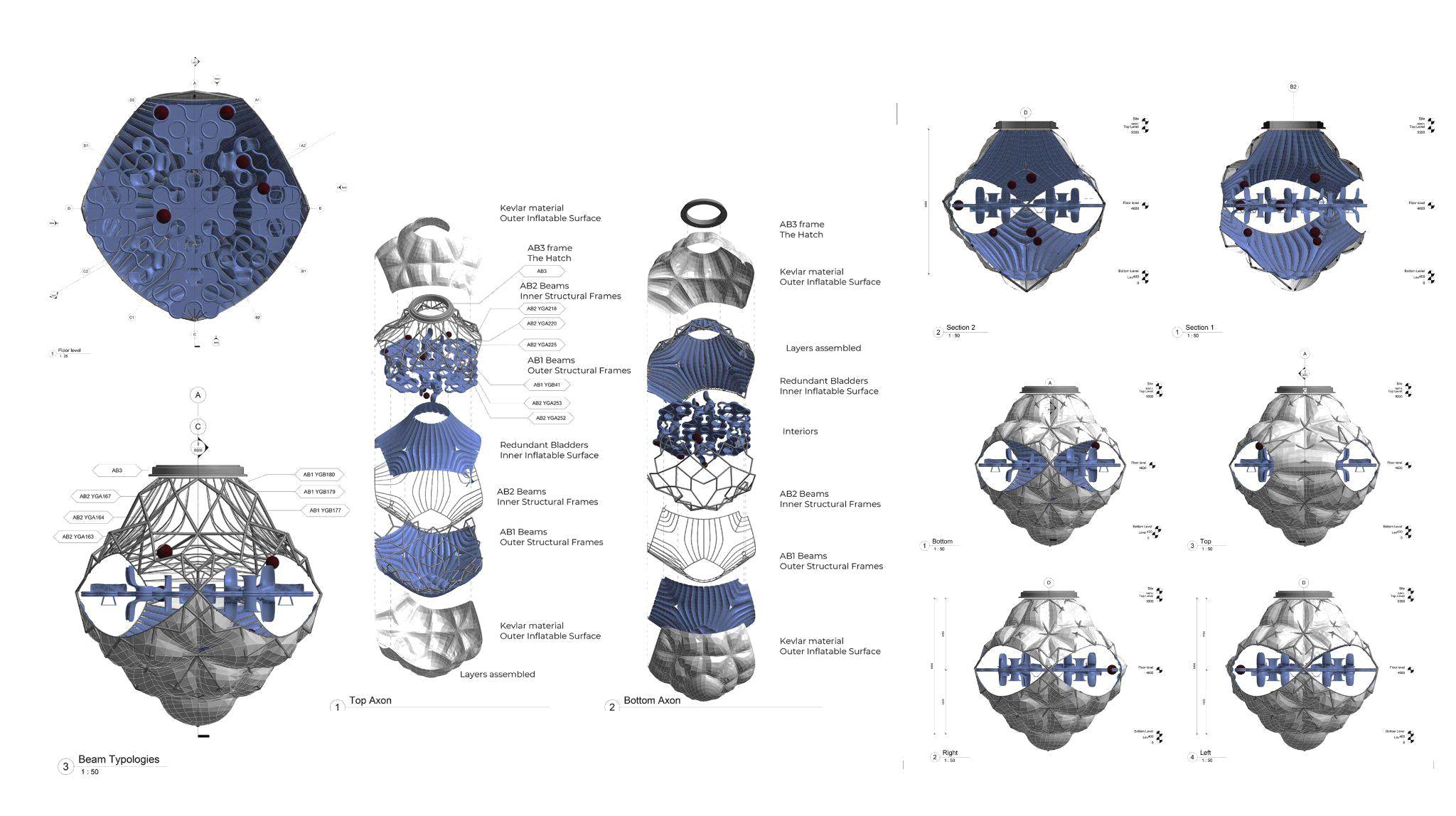
NEBULAE
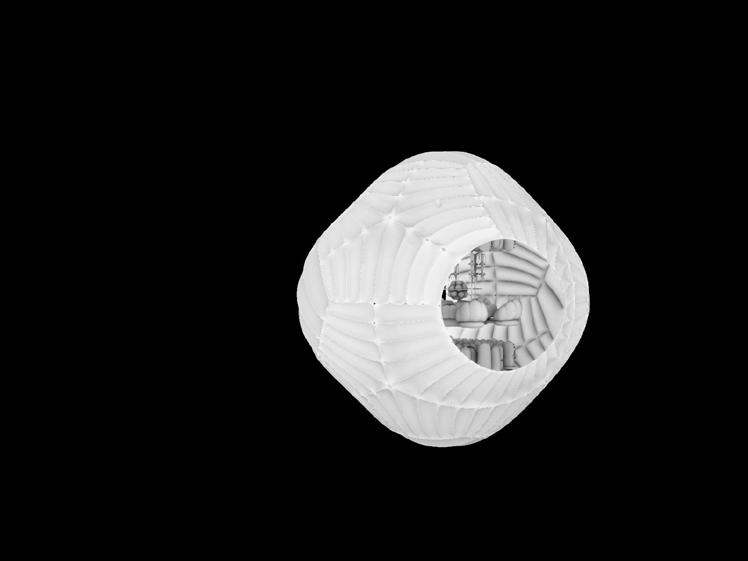
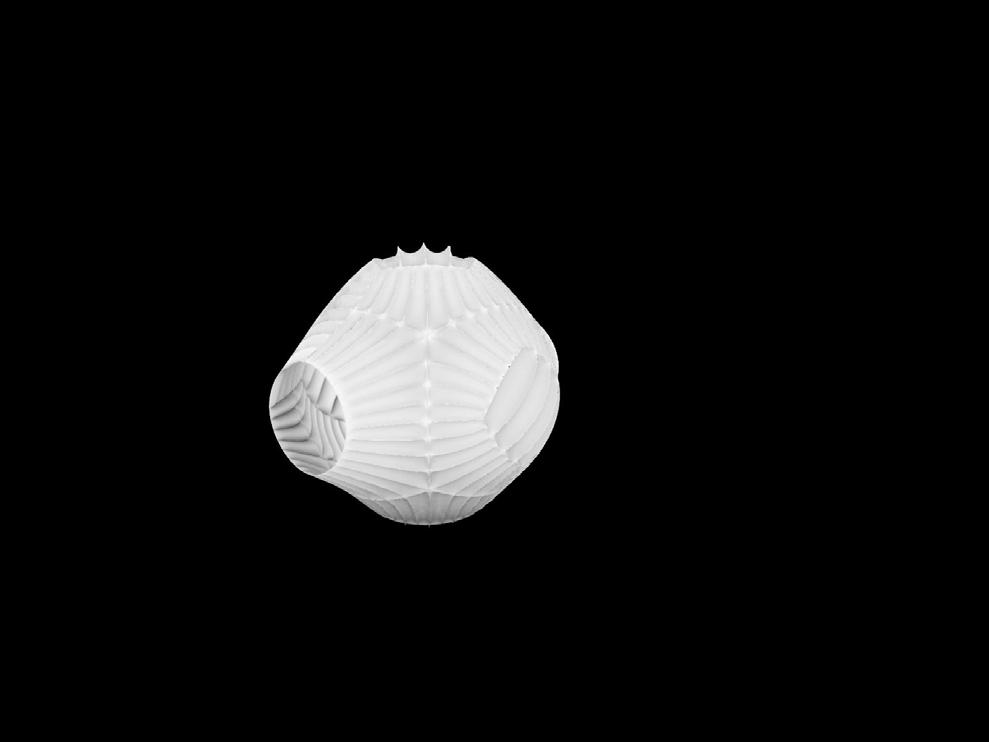

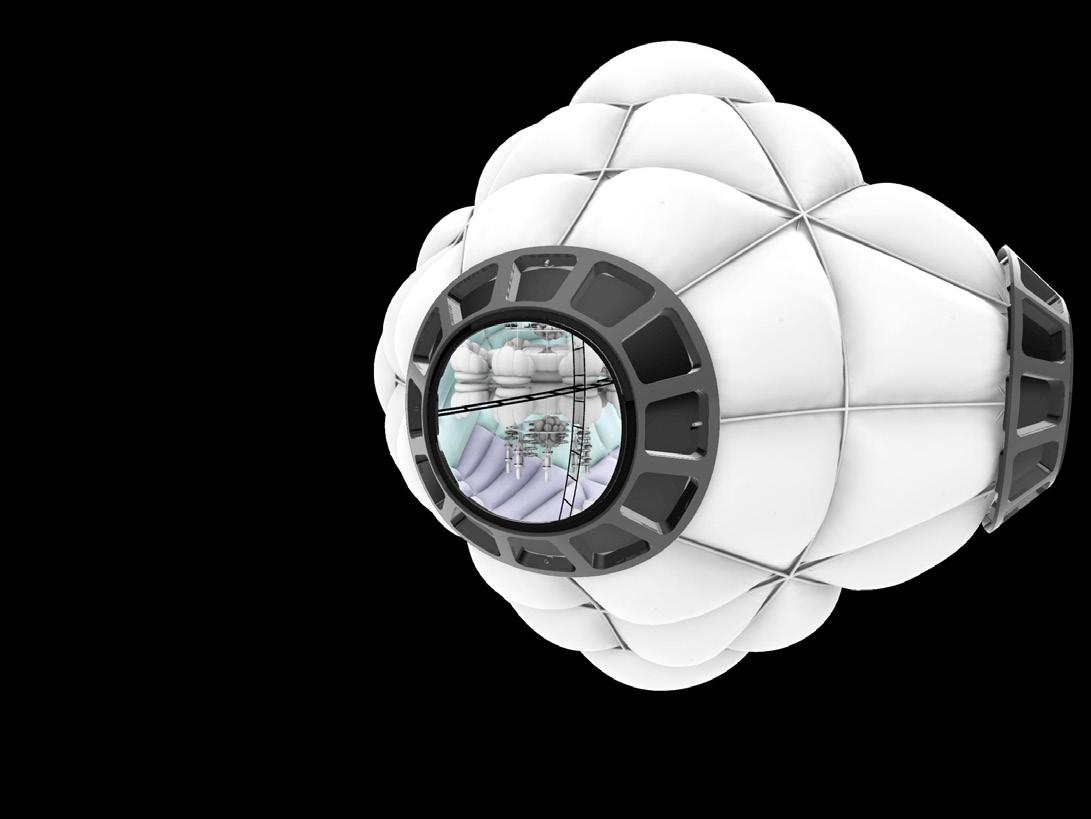
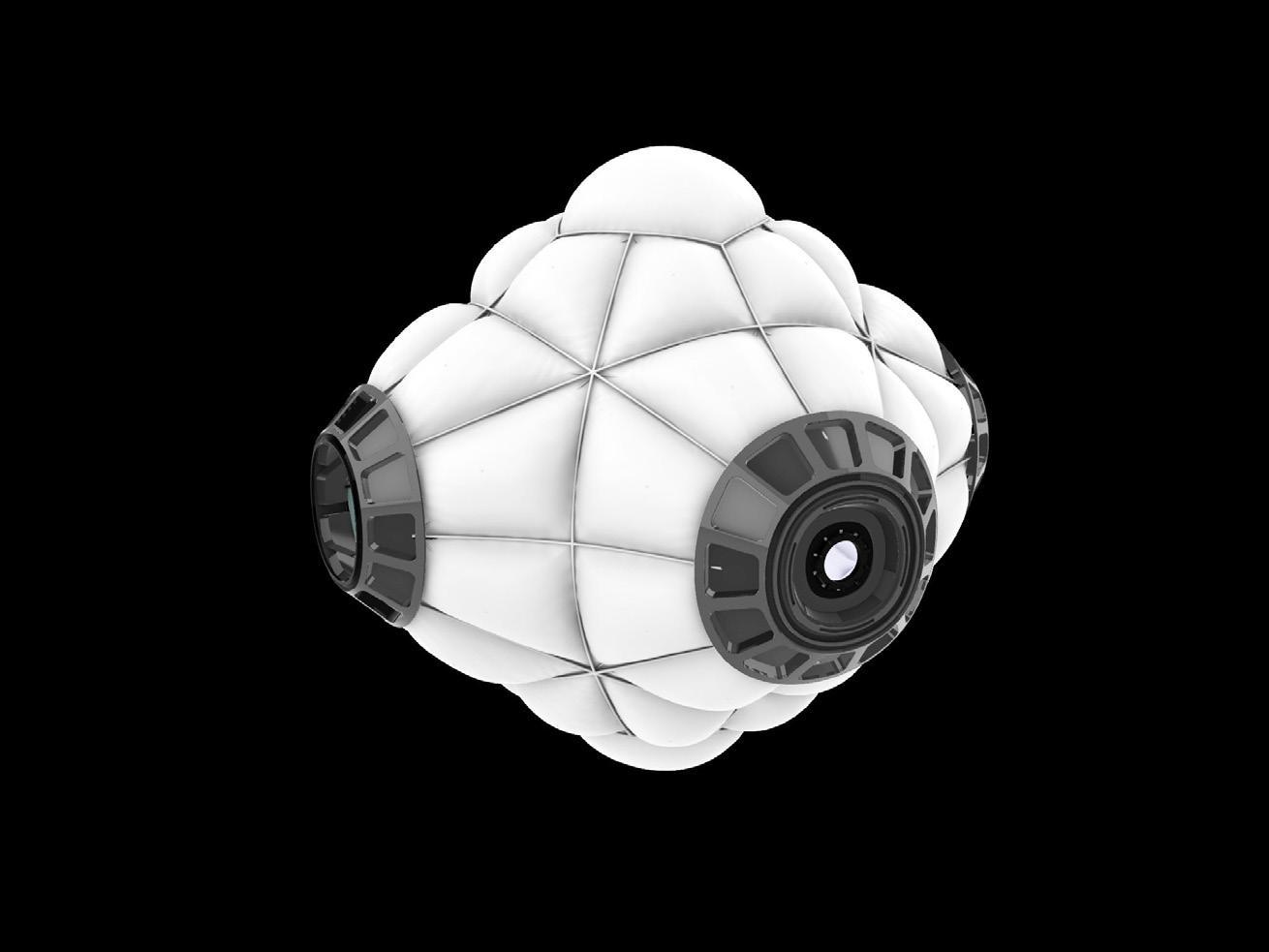
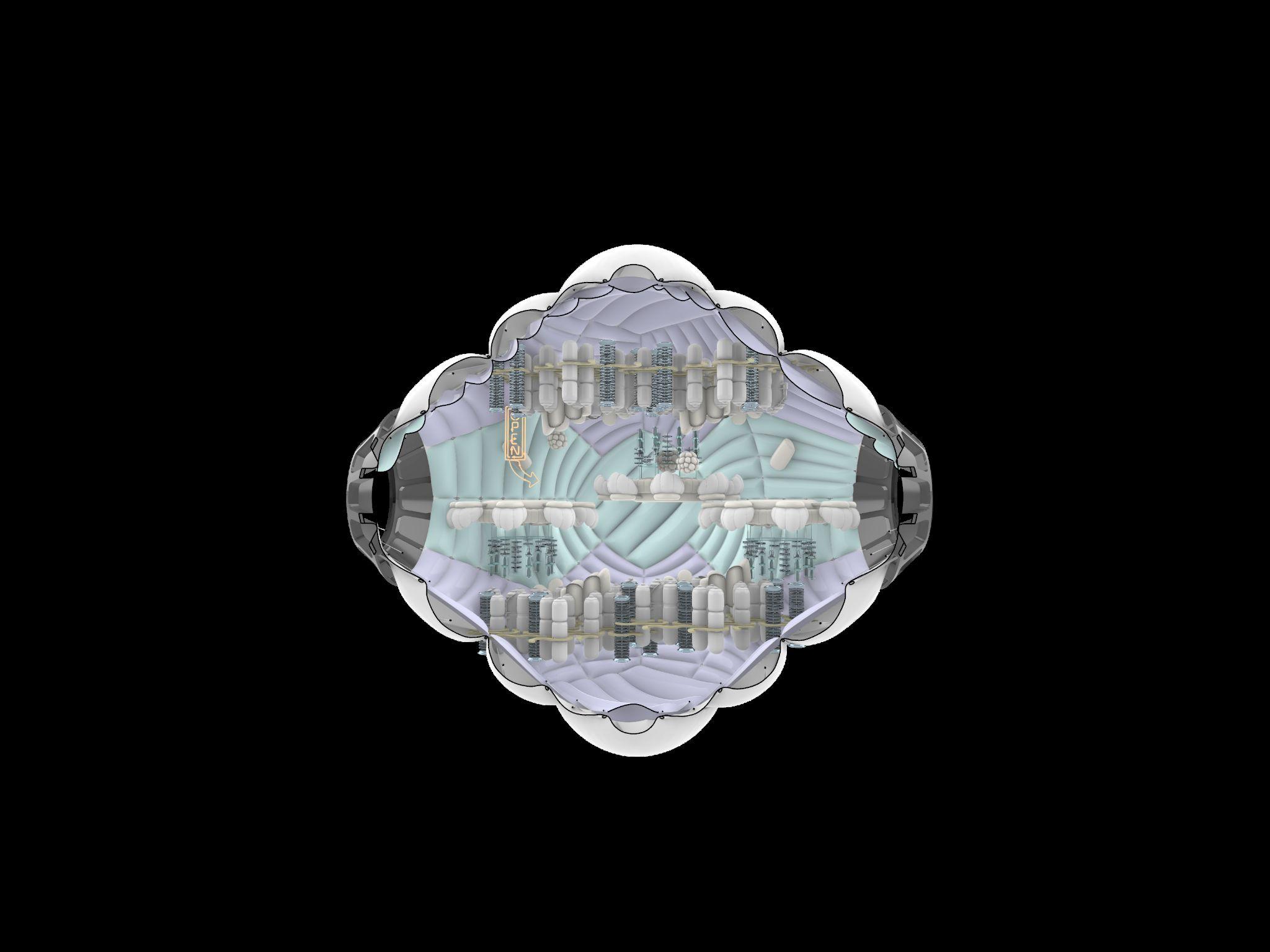
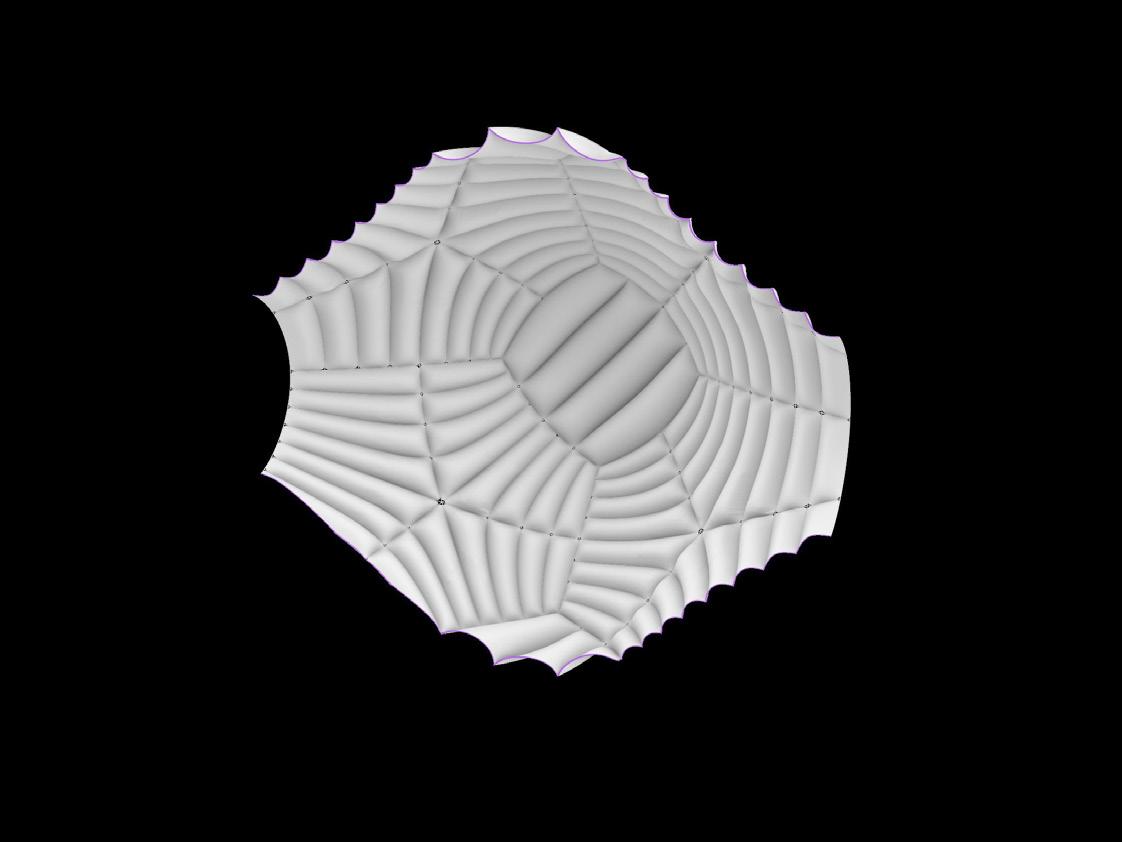

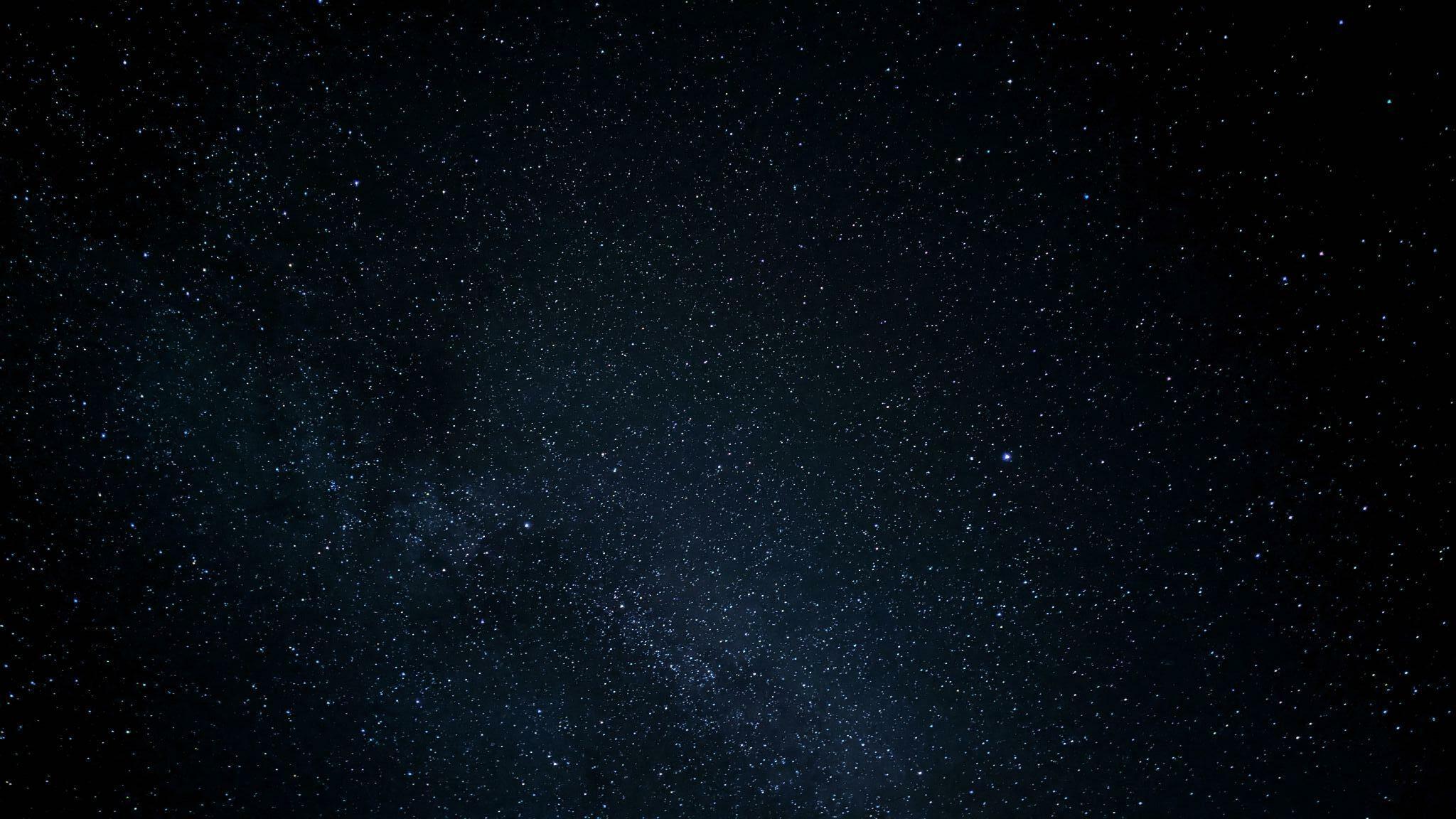
Casino Module
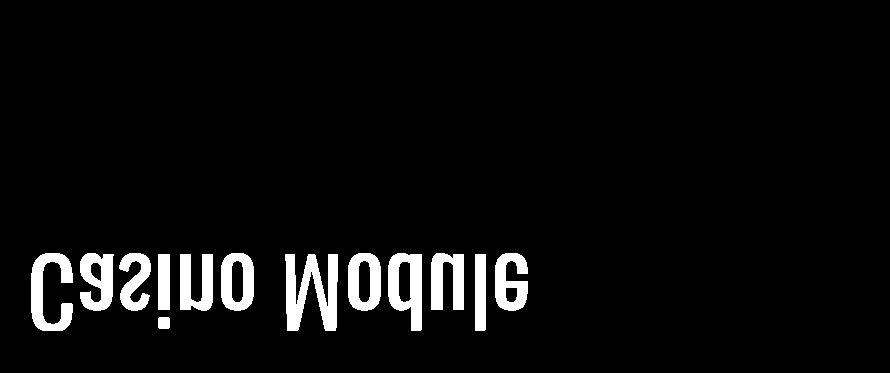
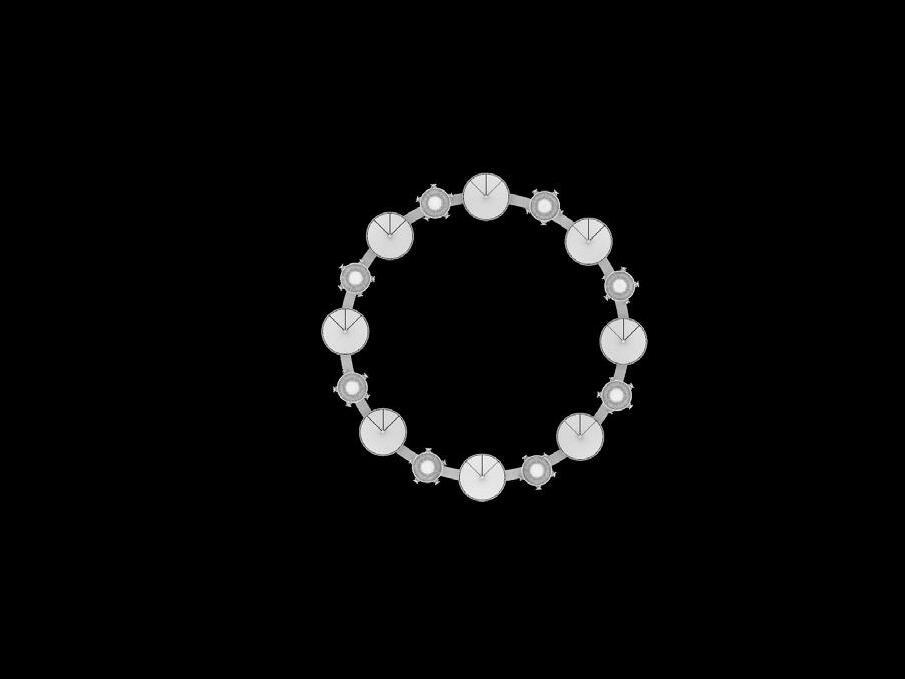
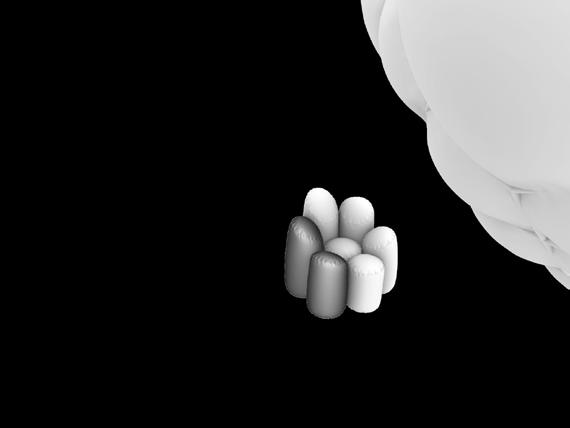
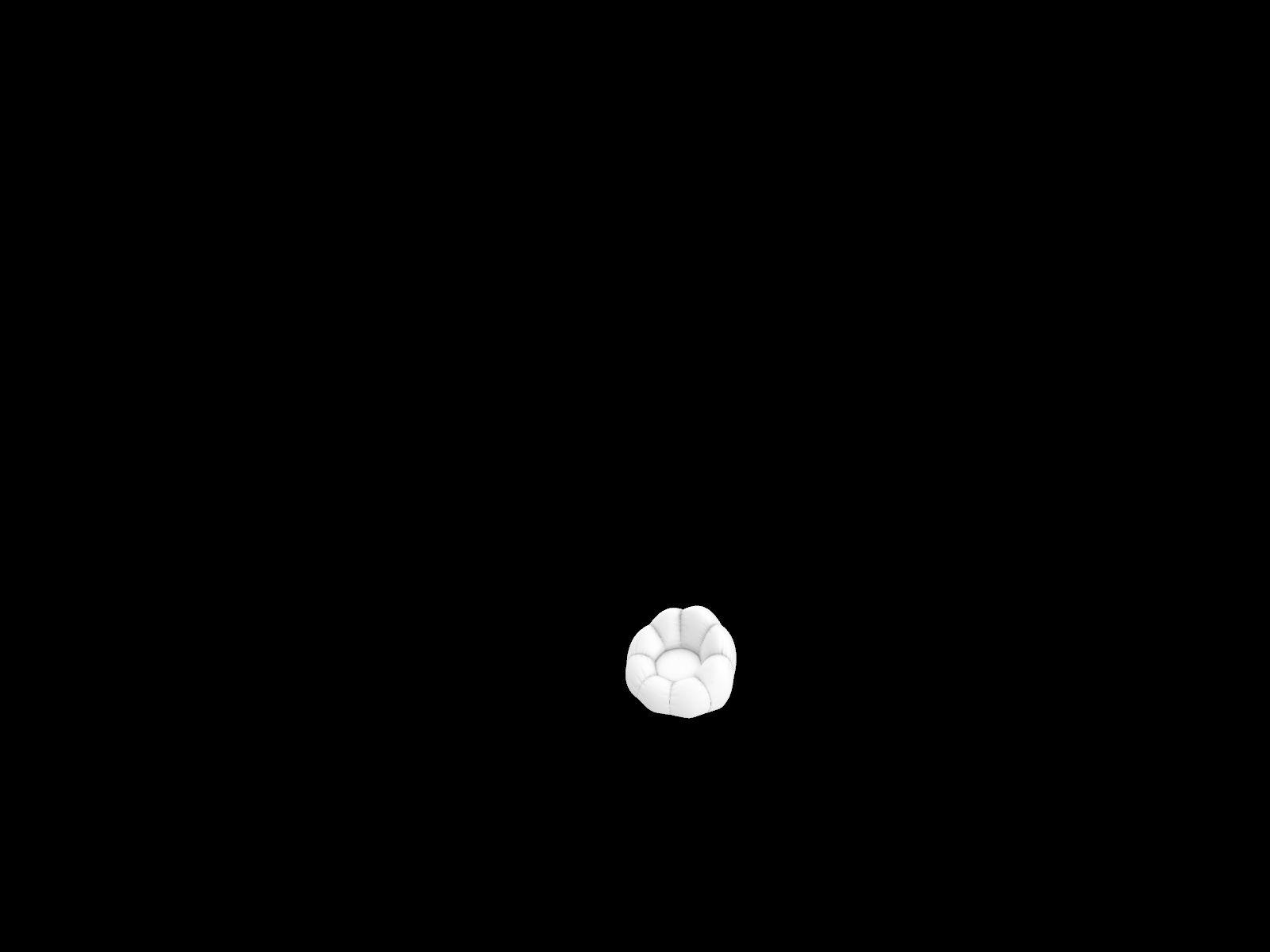
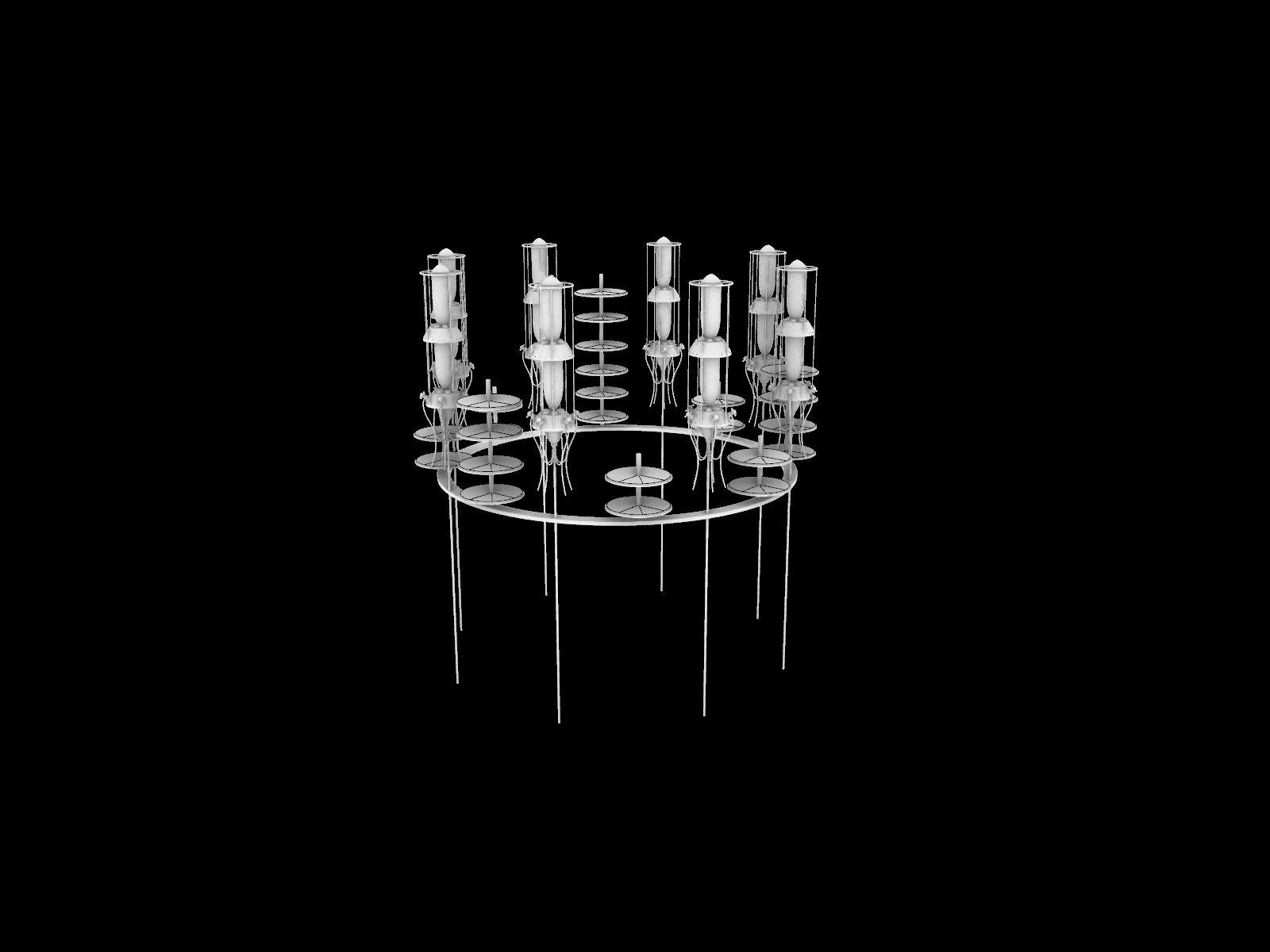
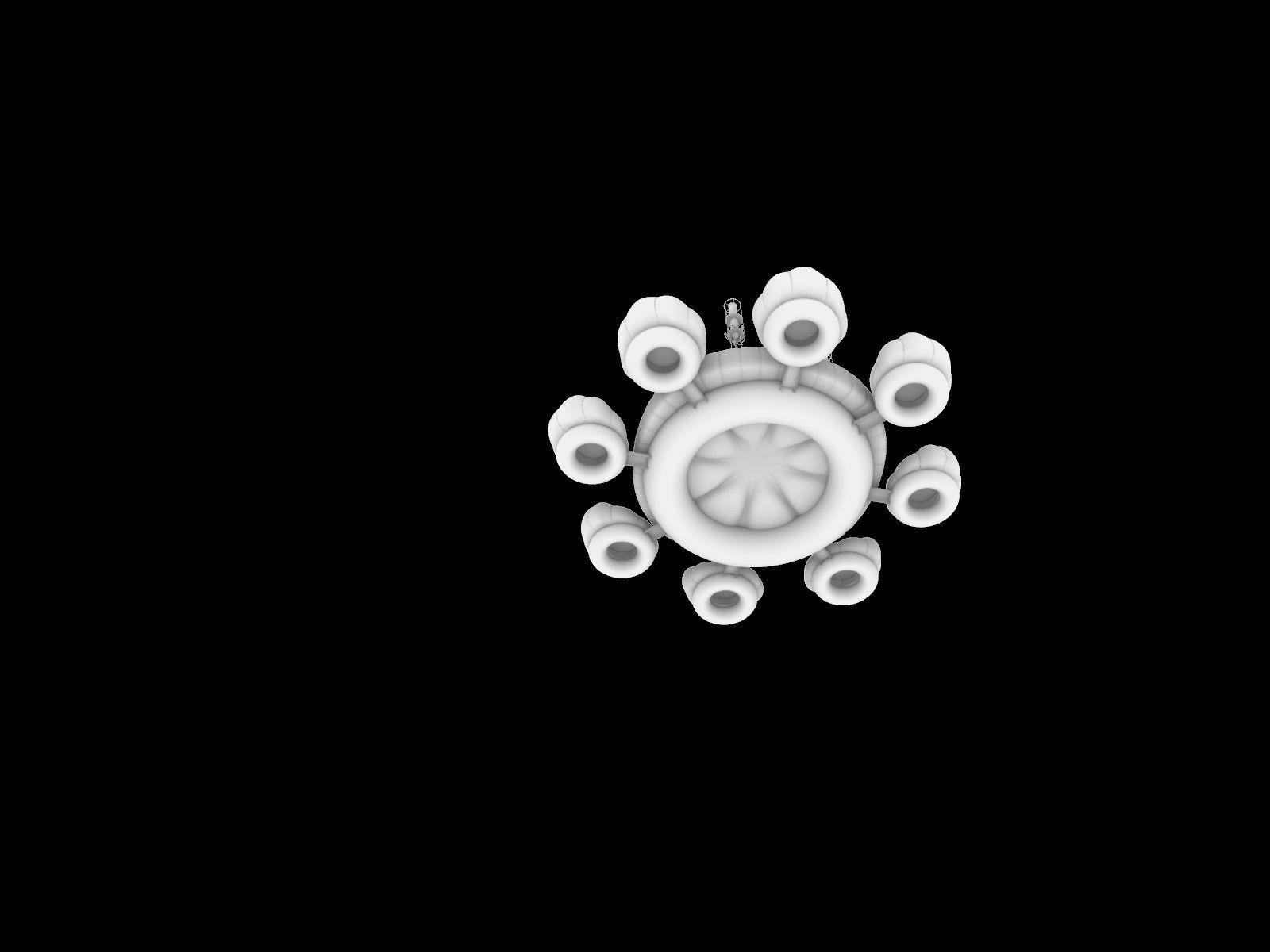
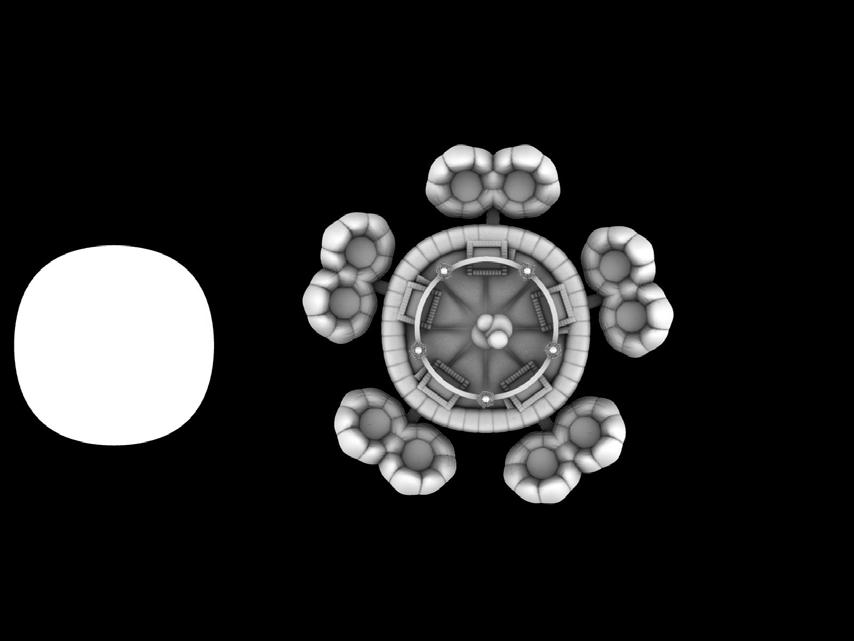
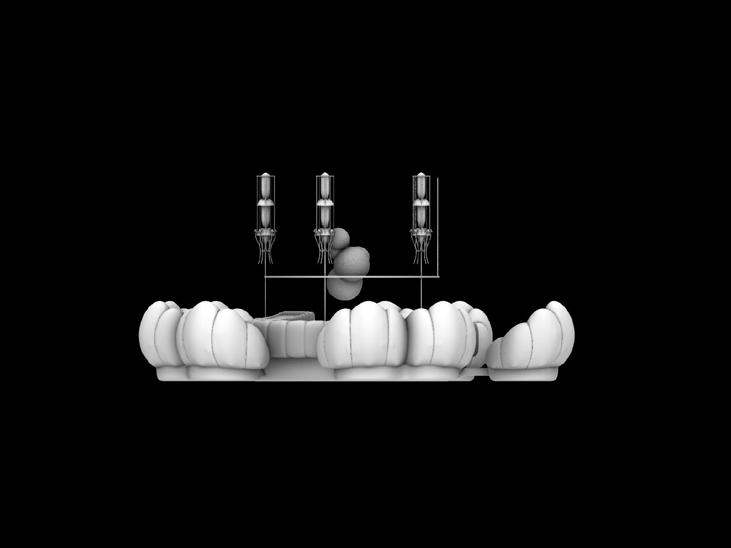
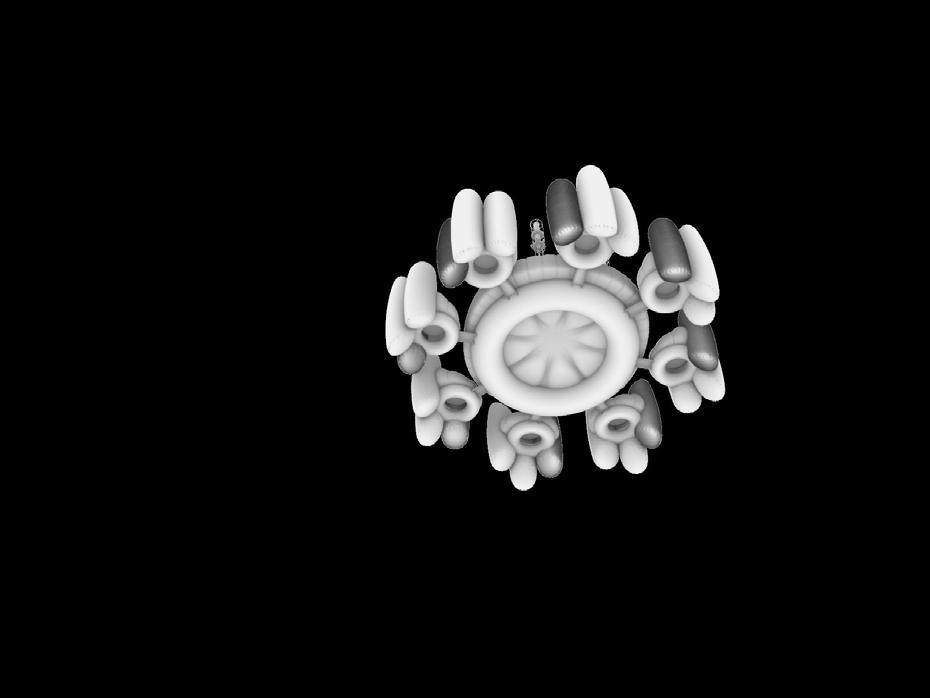
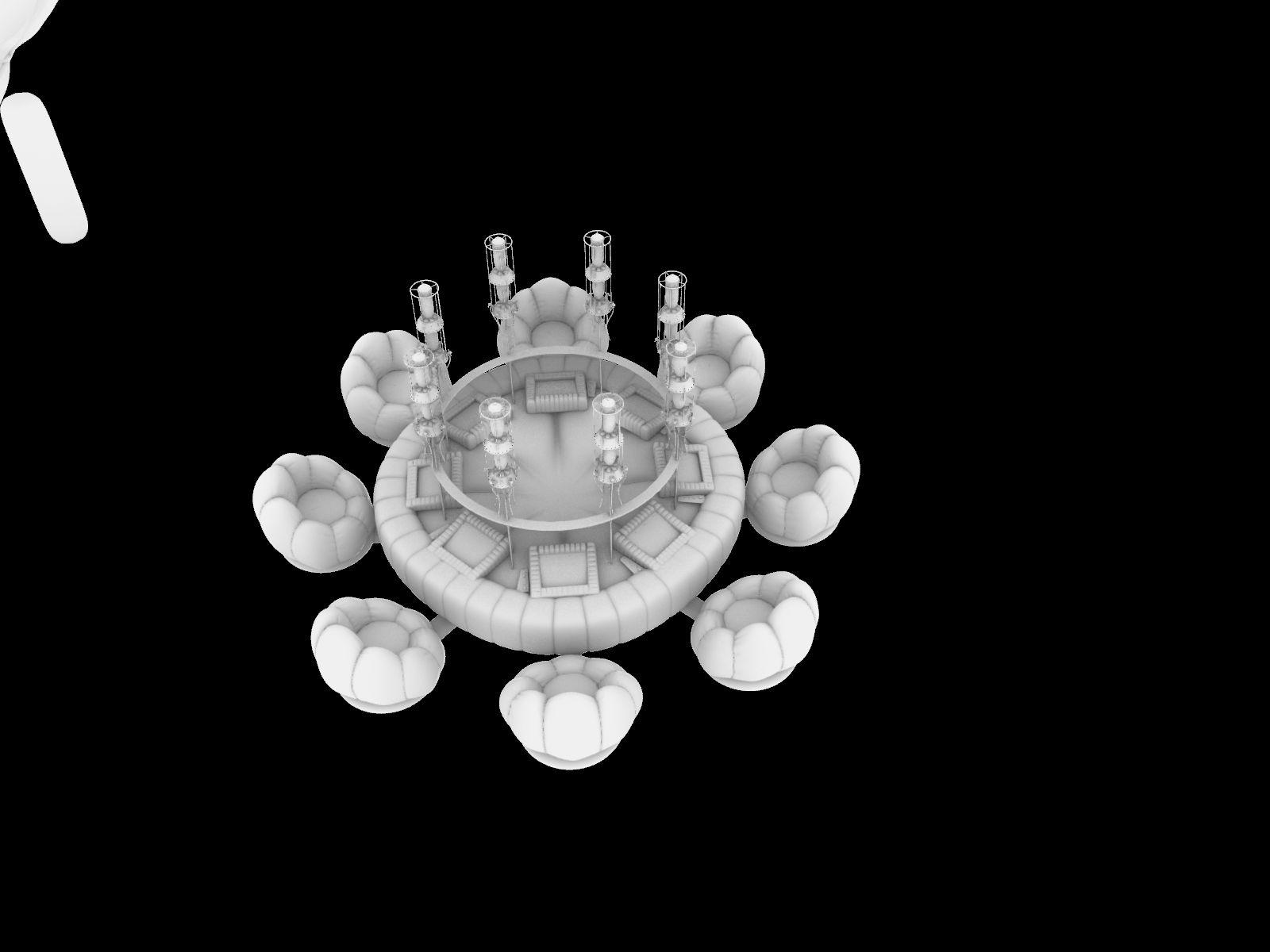
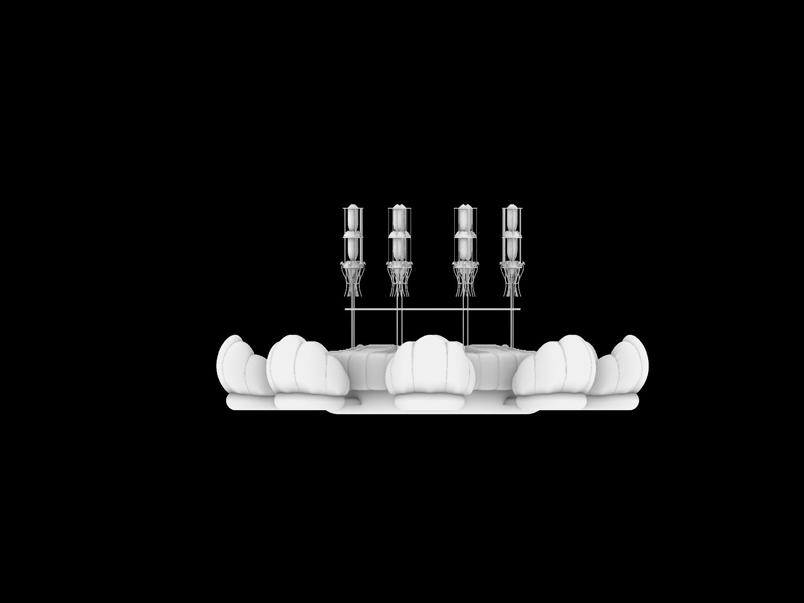

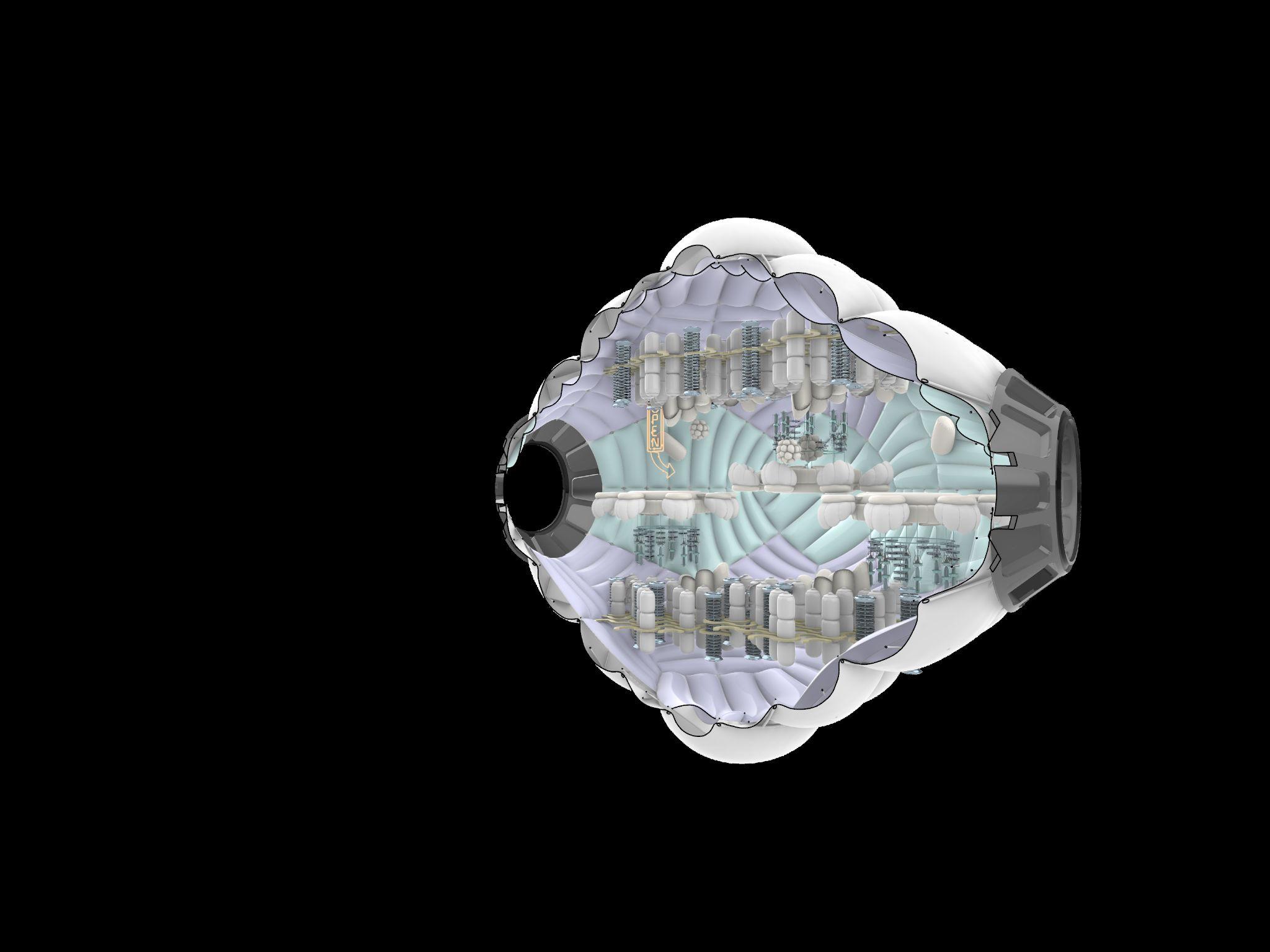
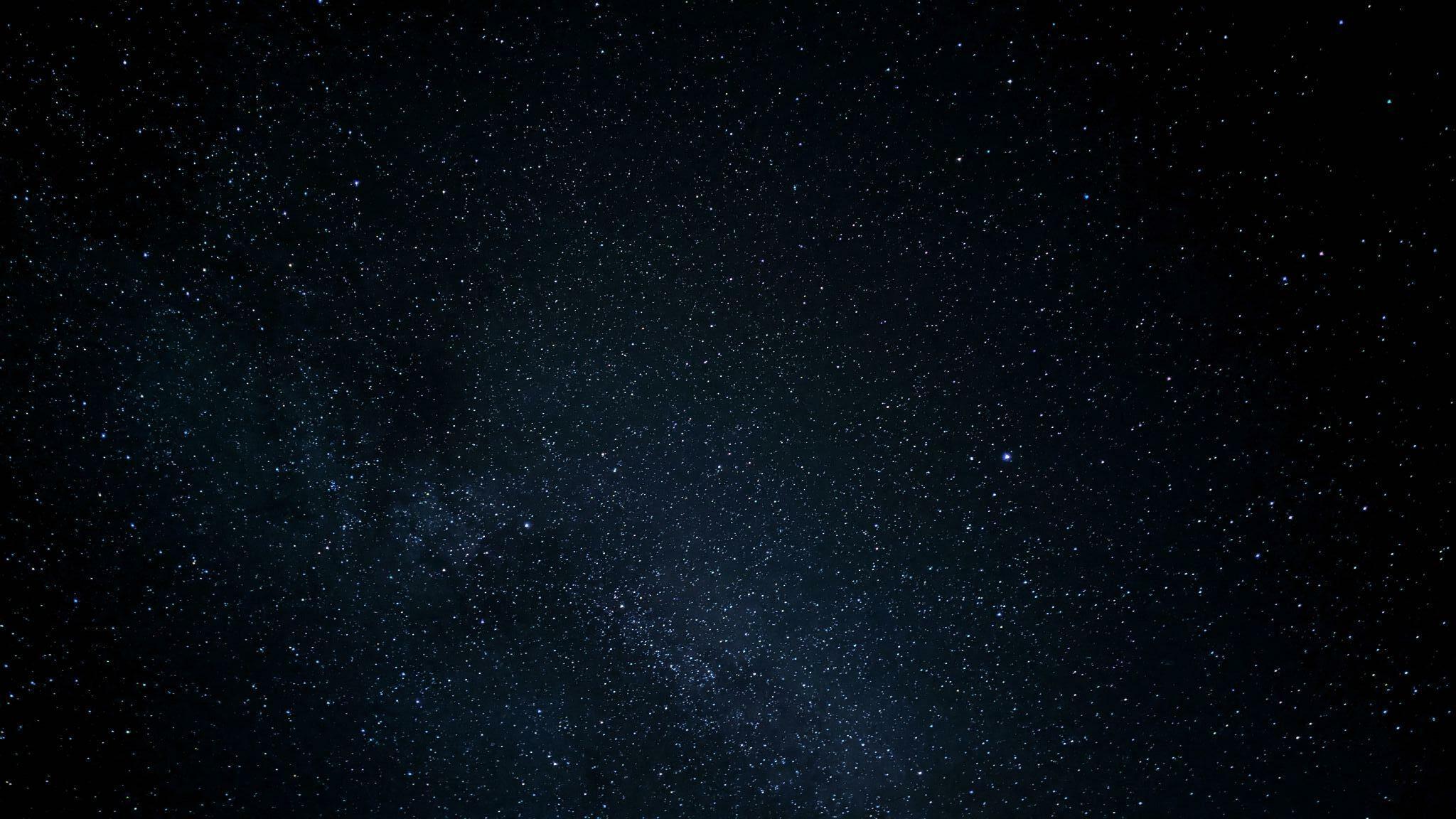
NEBULAE Casino Module
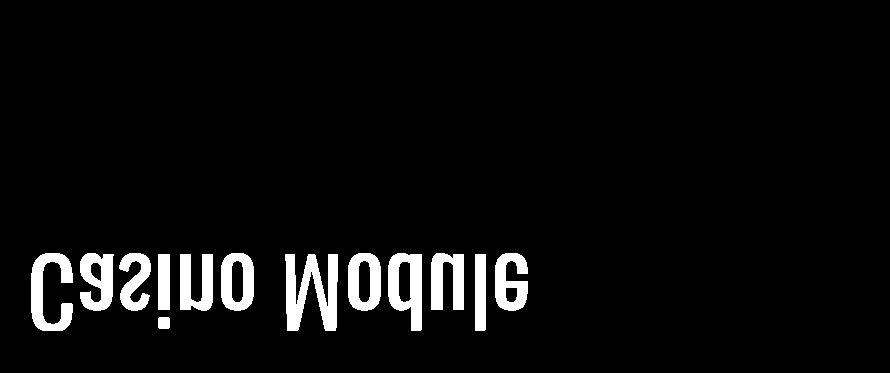
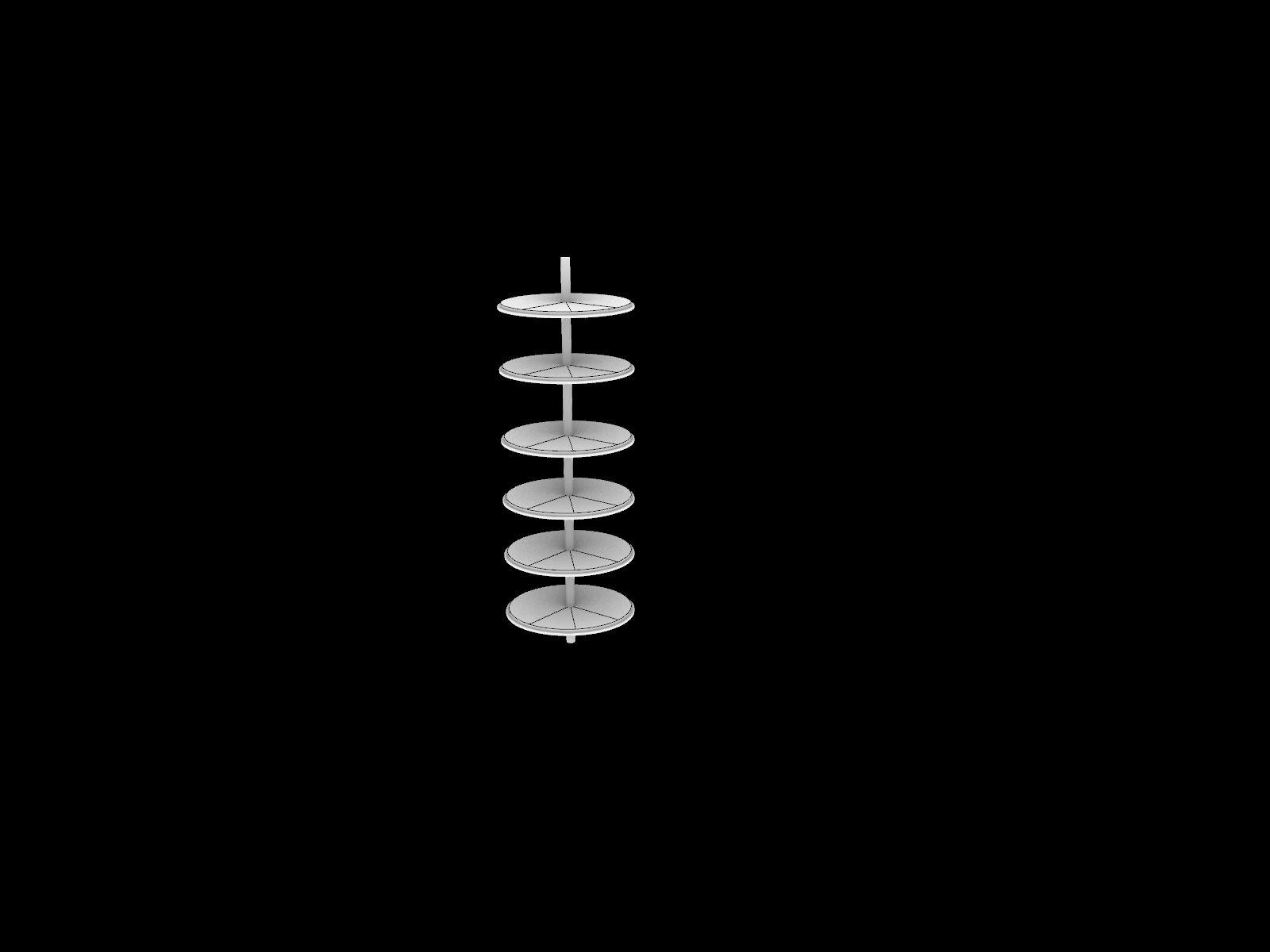
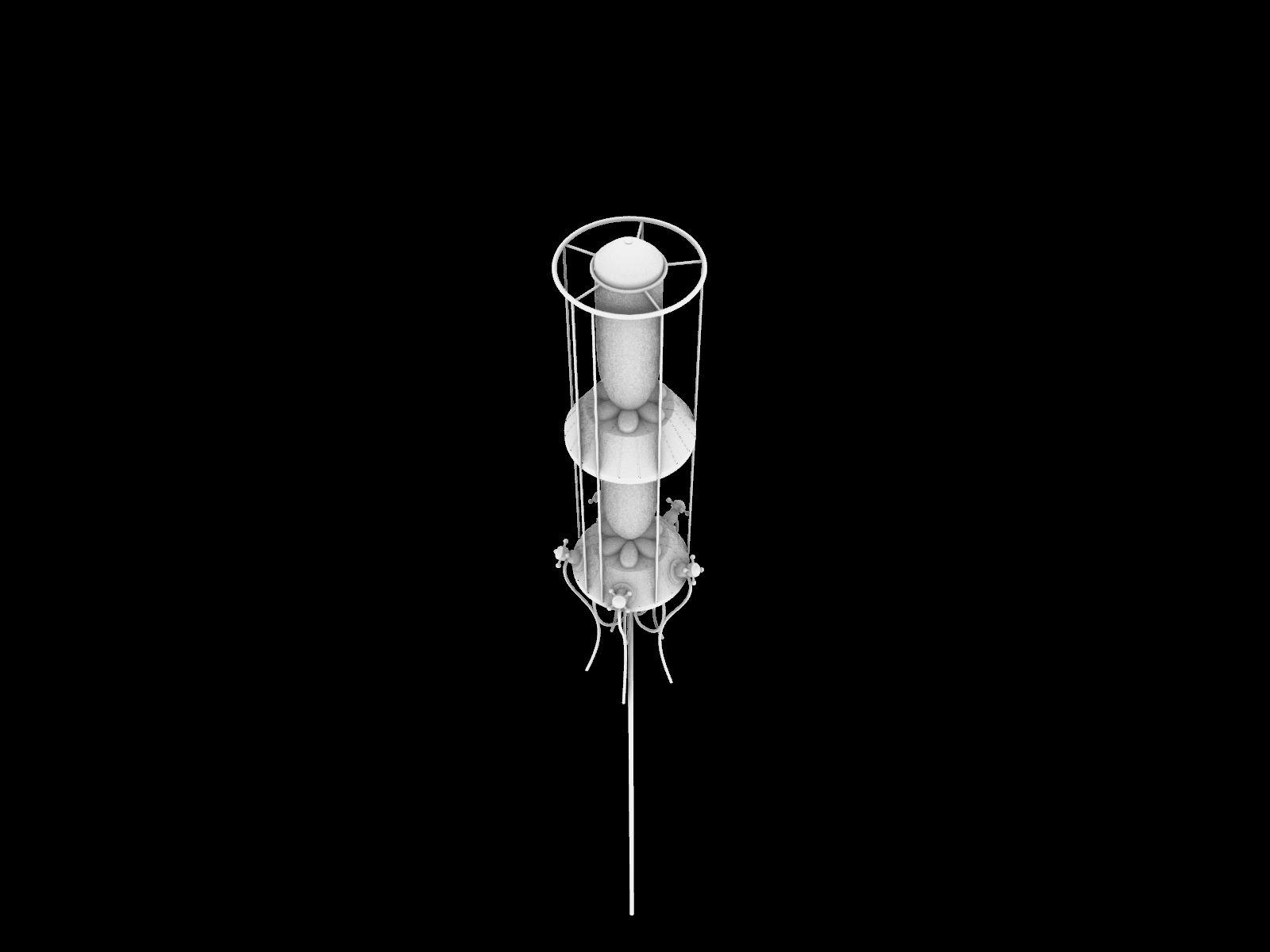
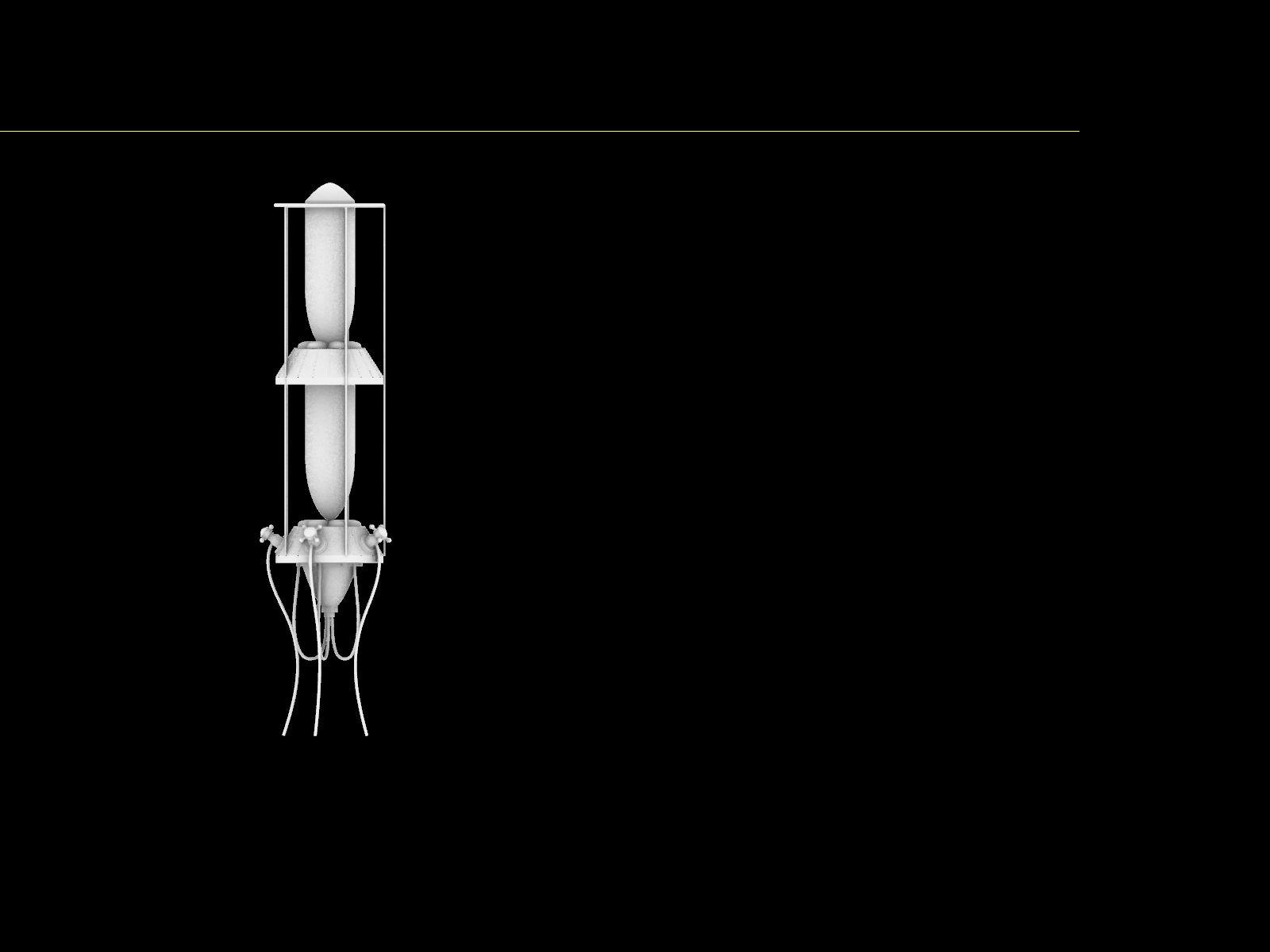
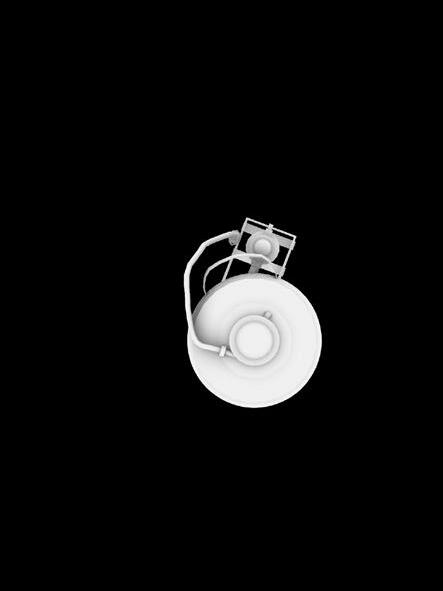
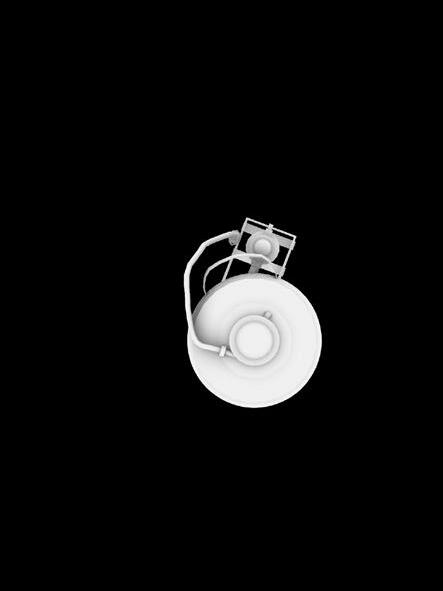
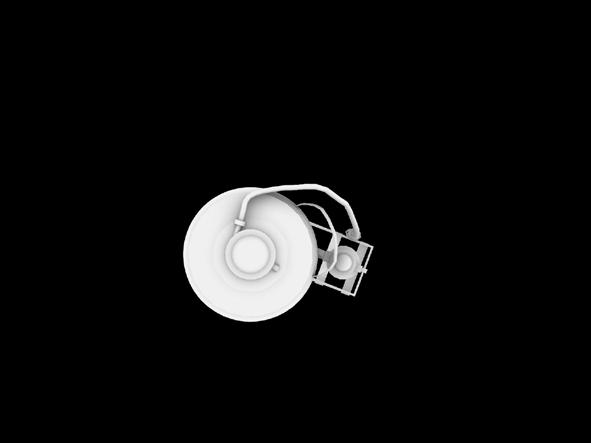
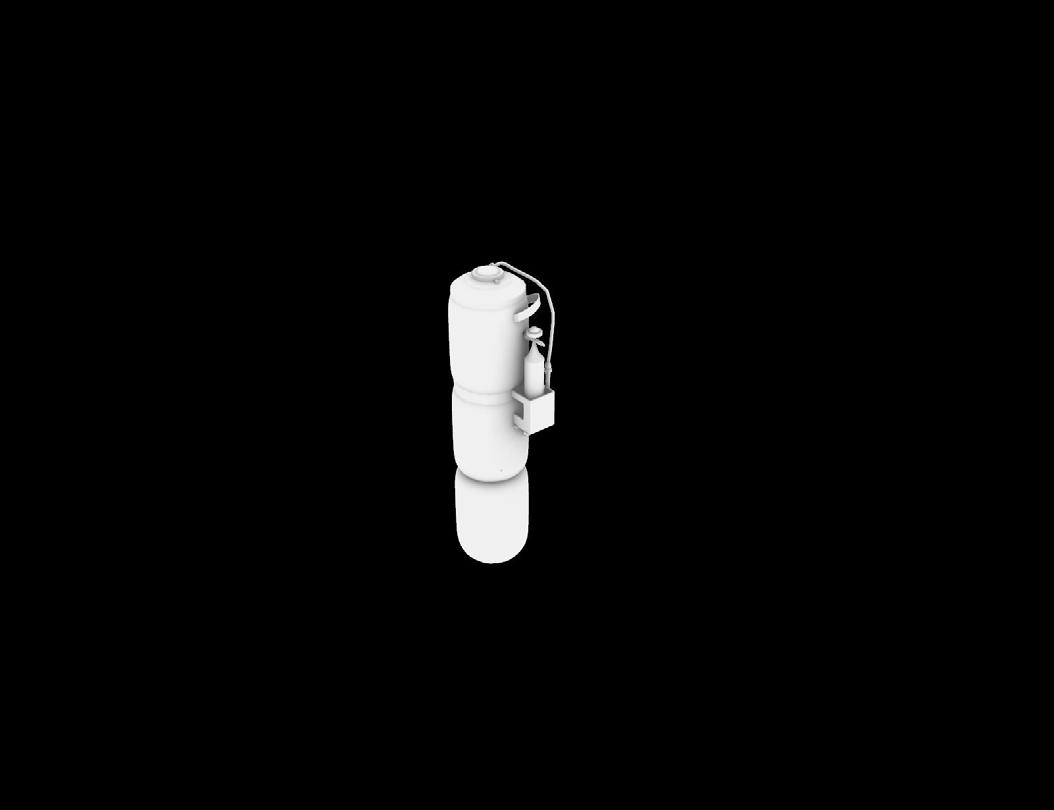
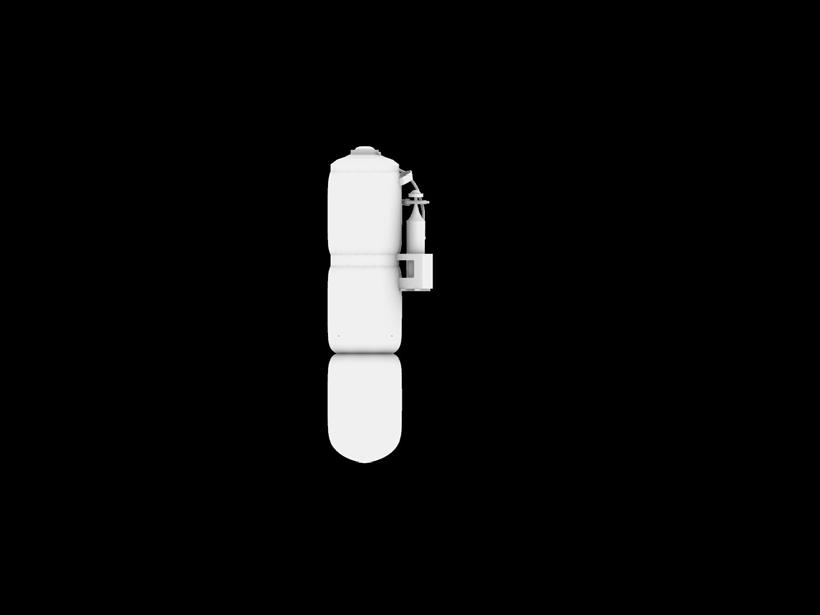
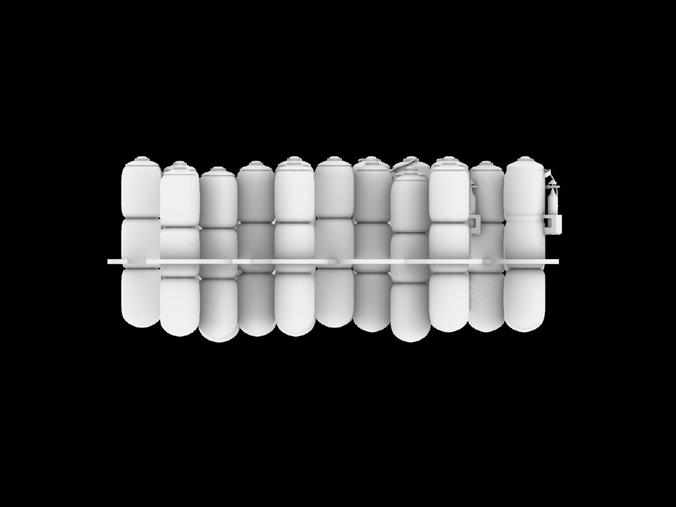
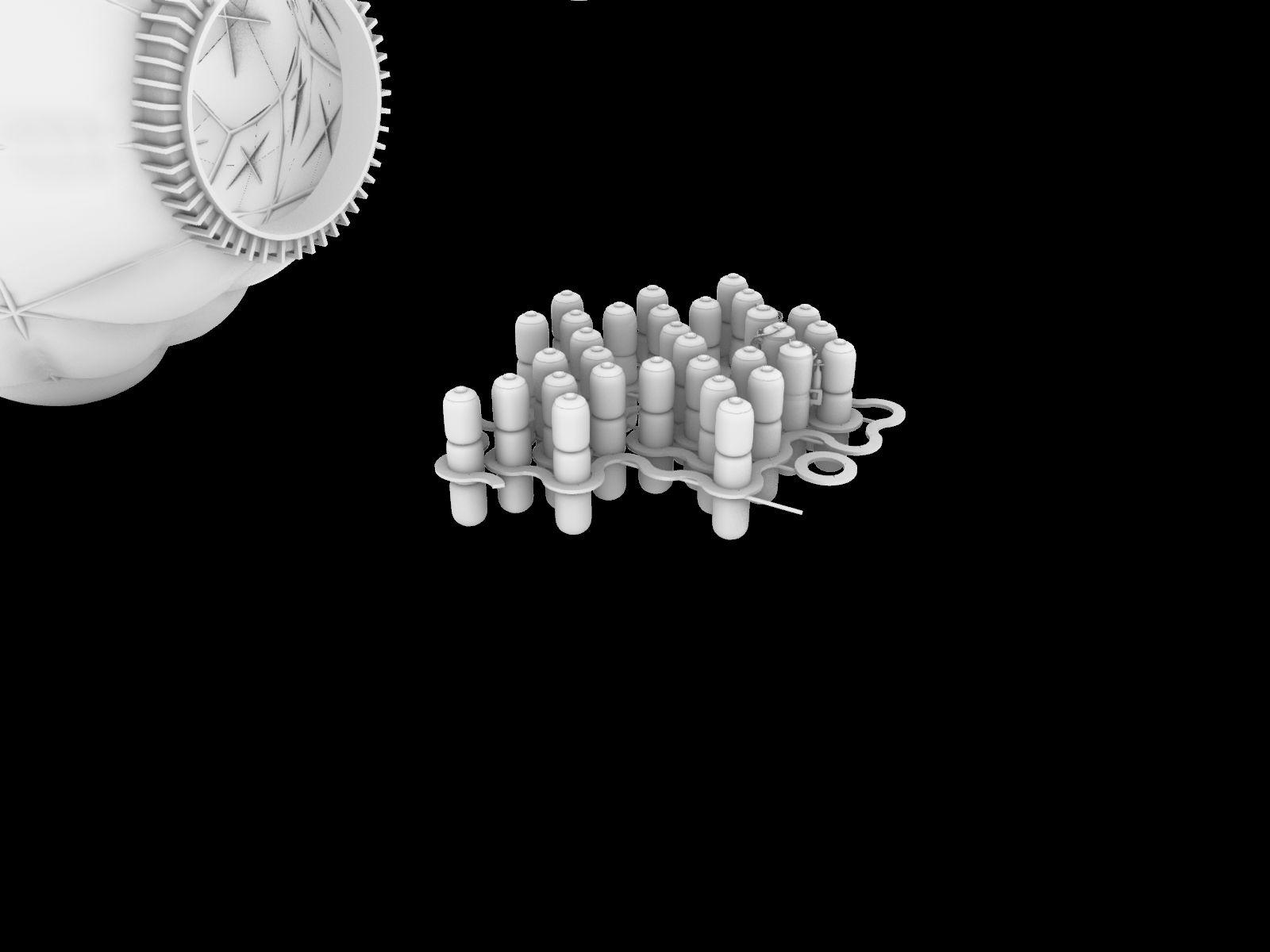
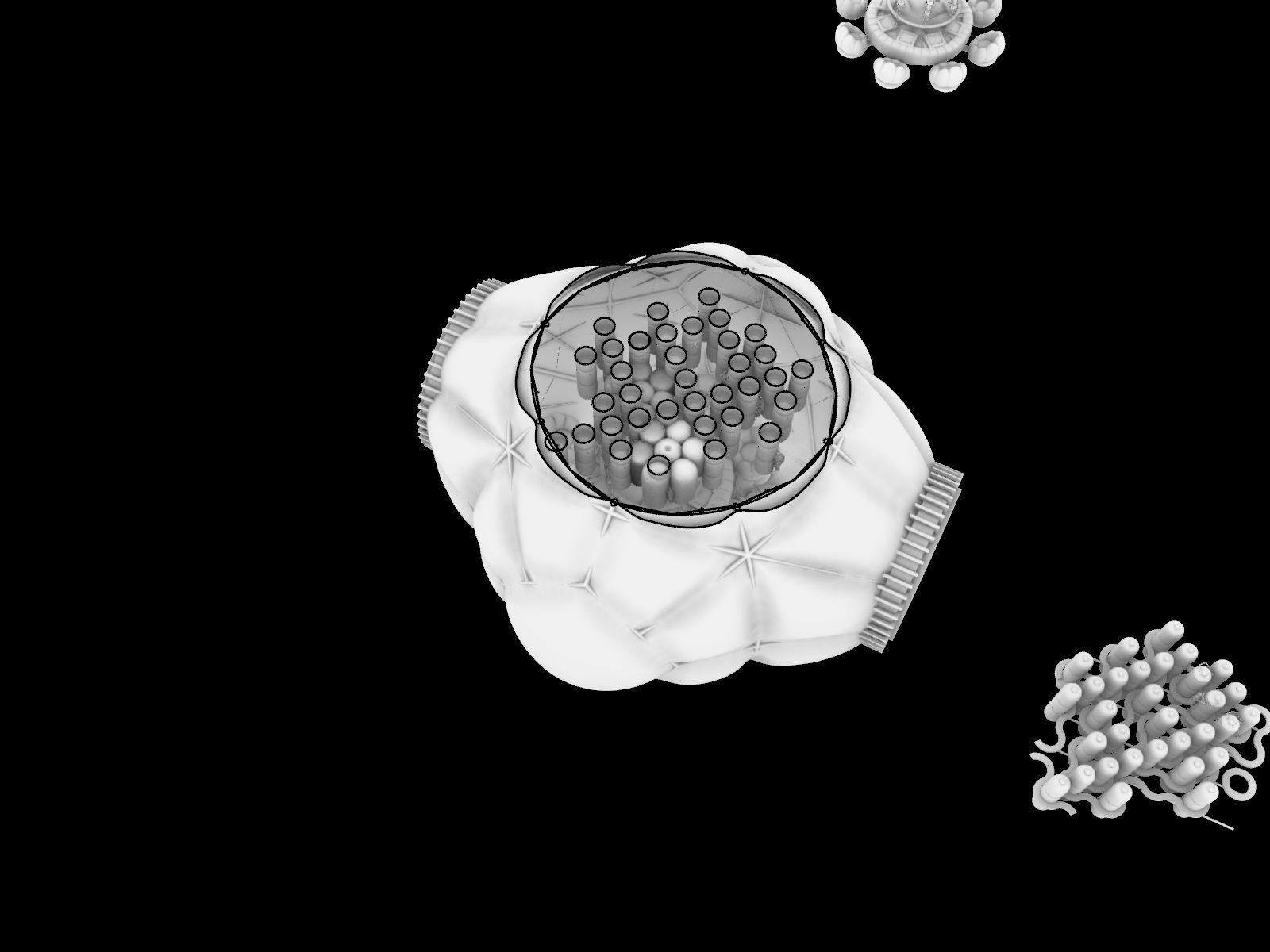
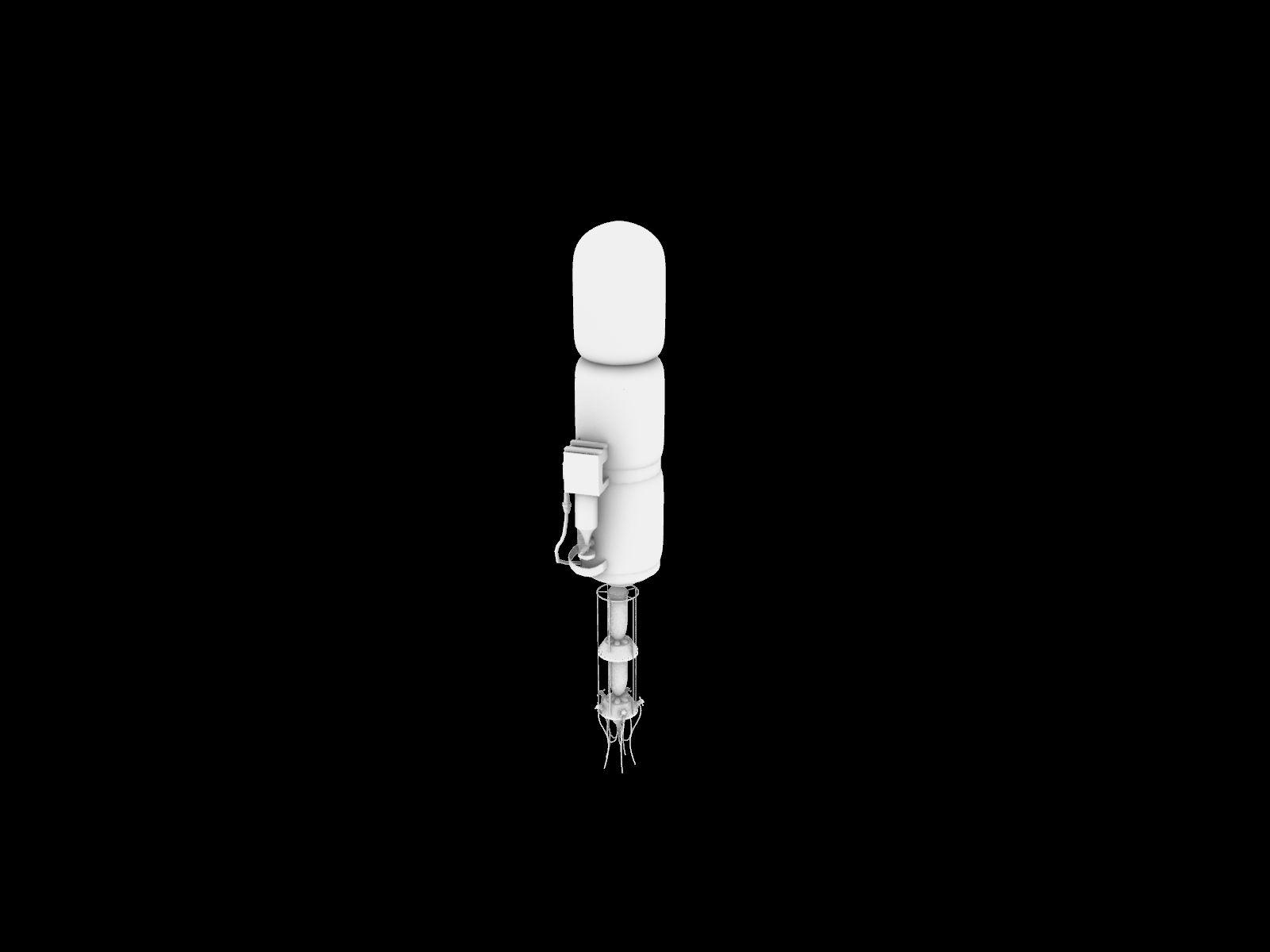
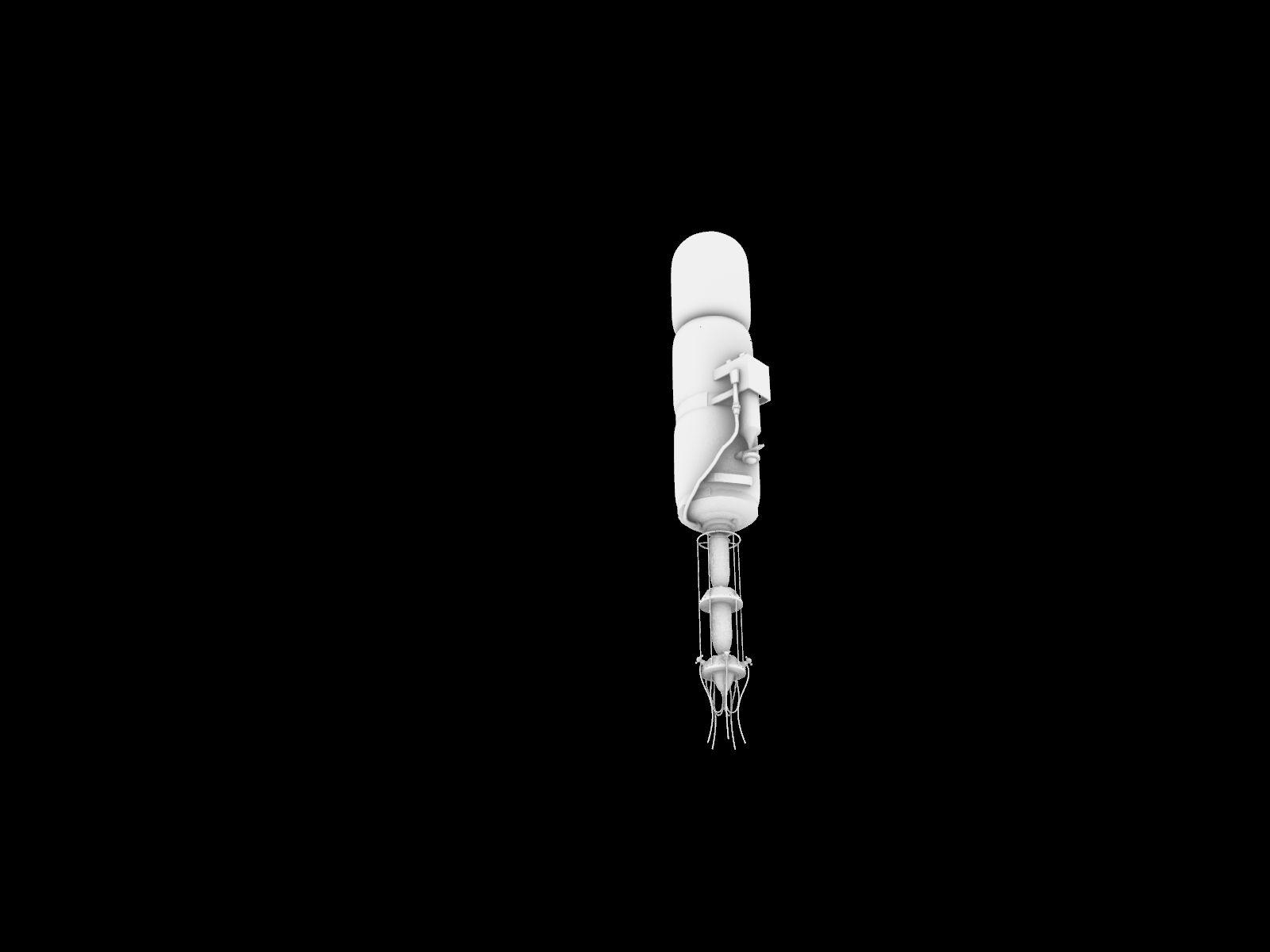
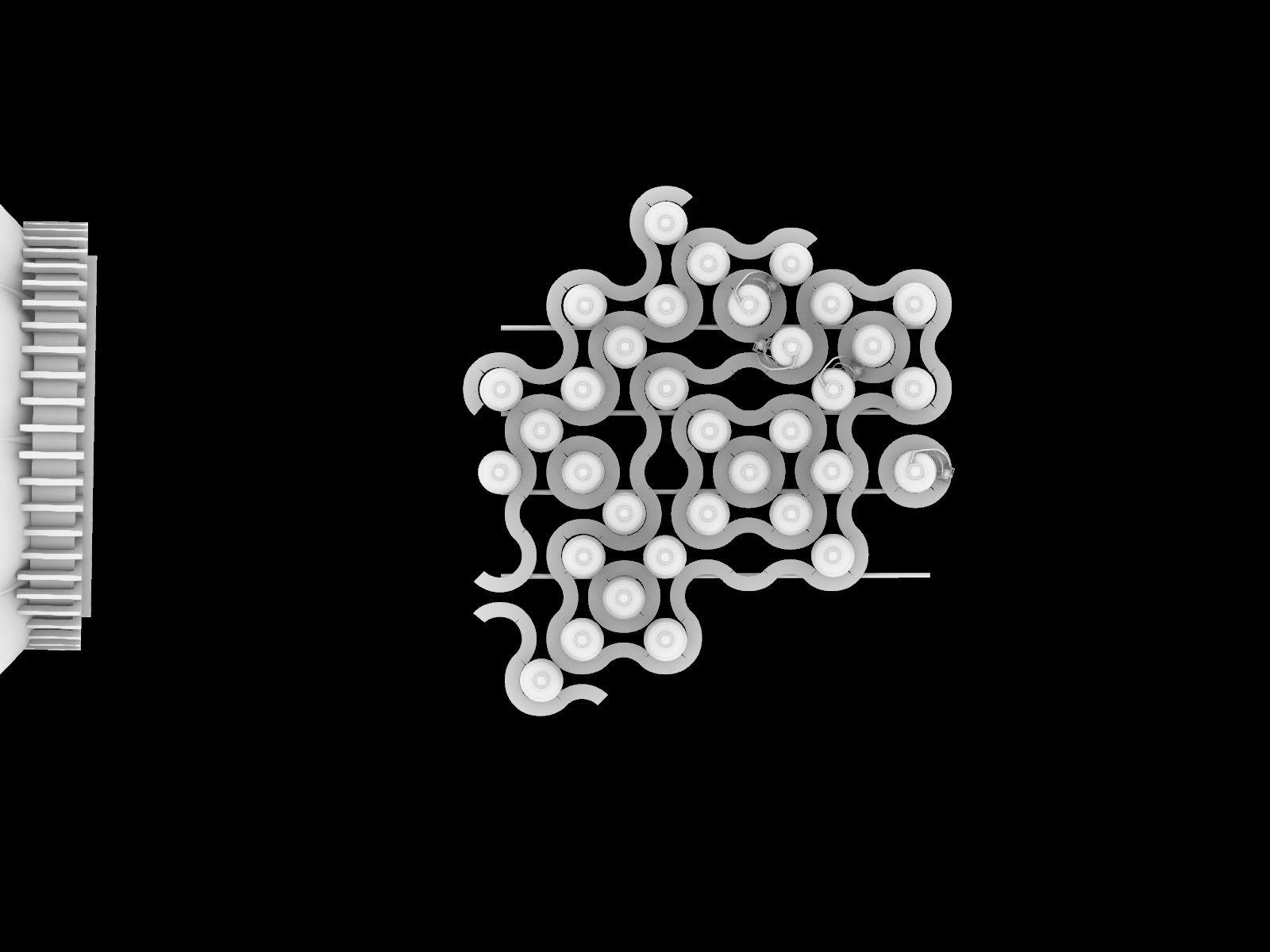
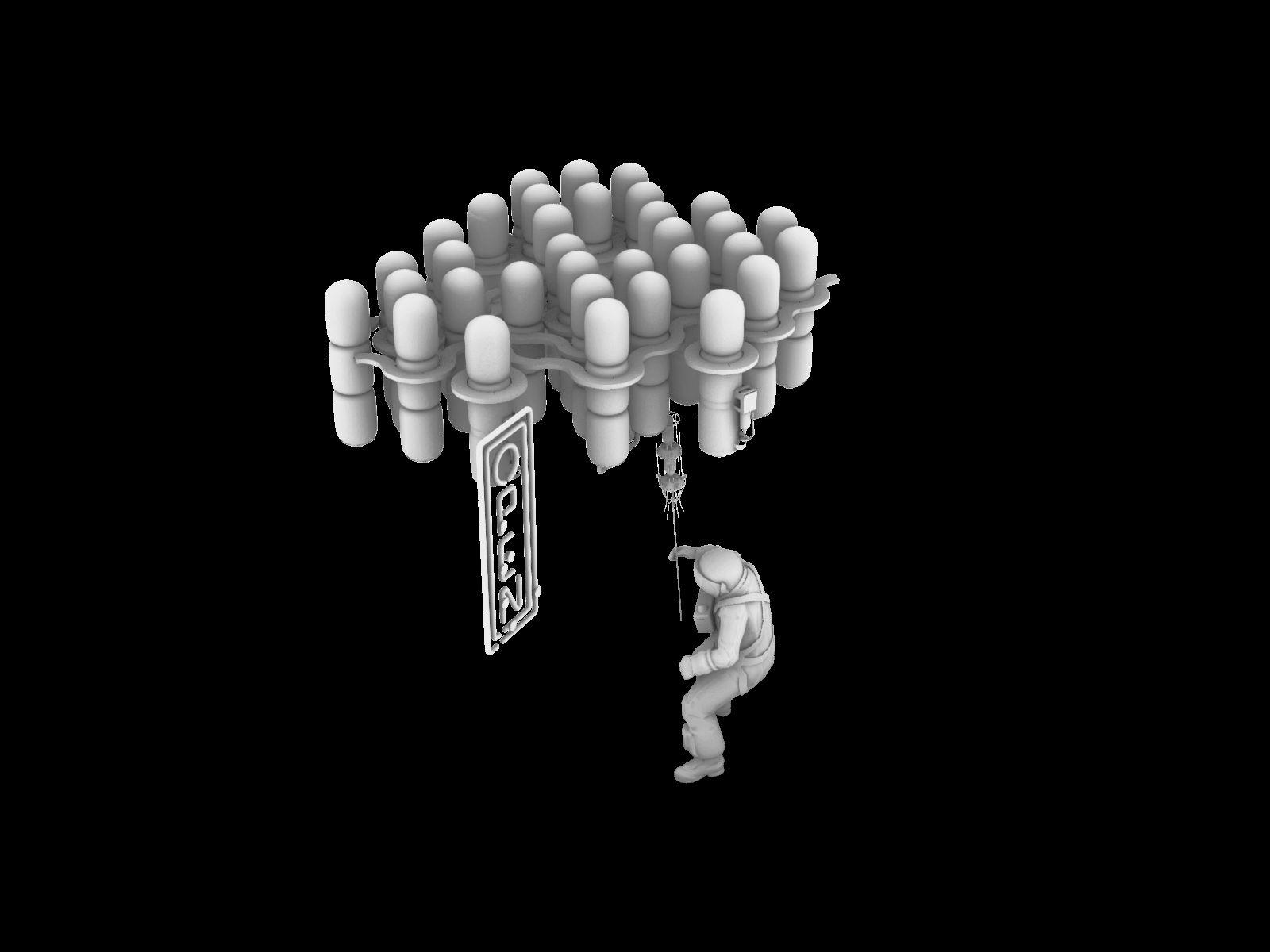
Casino in Space
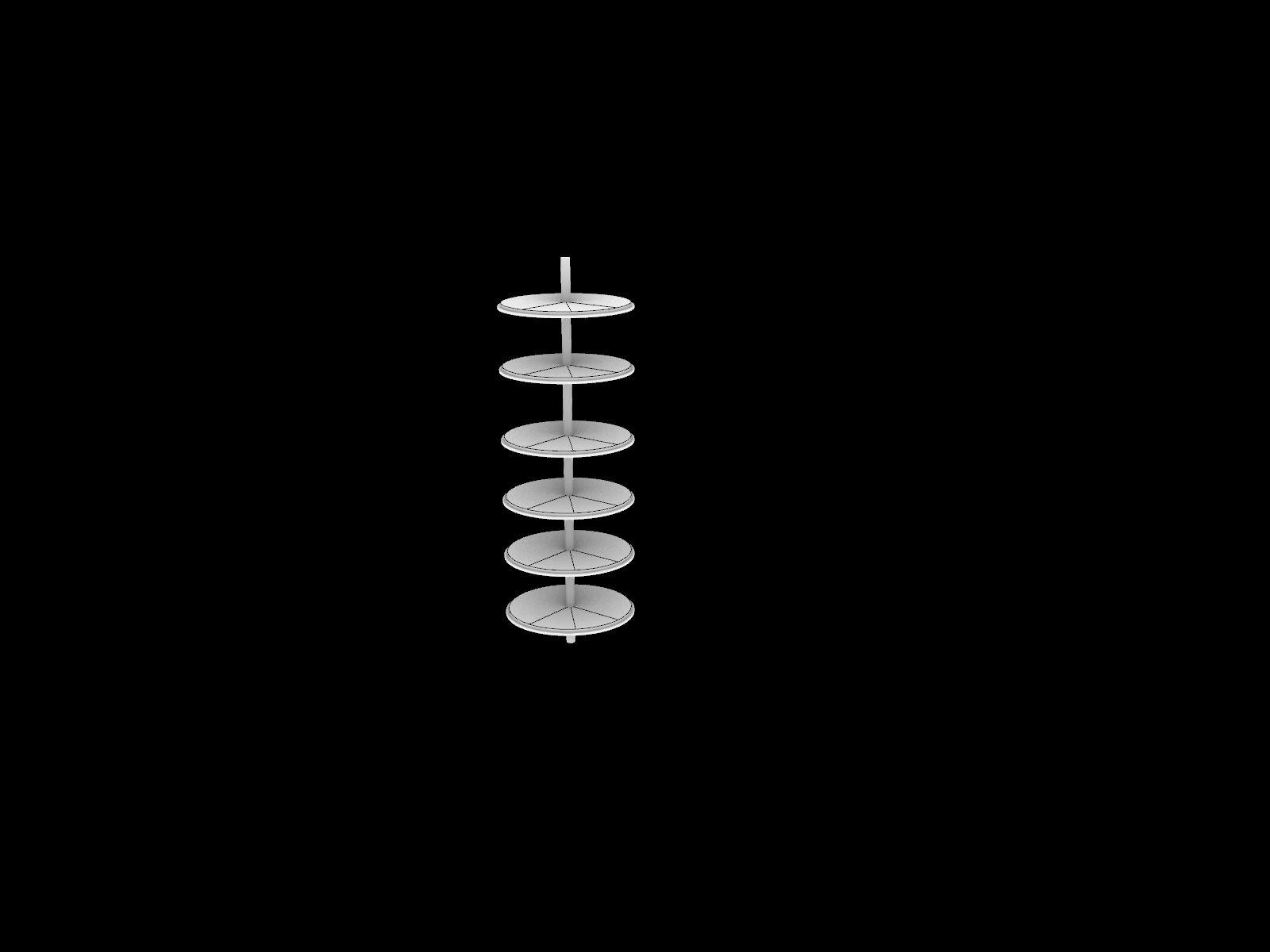
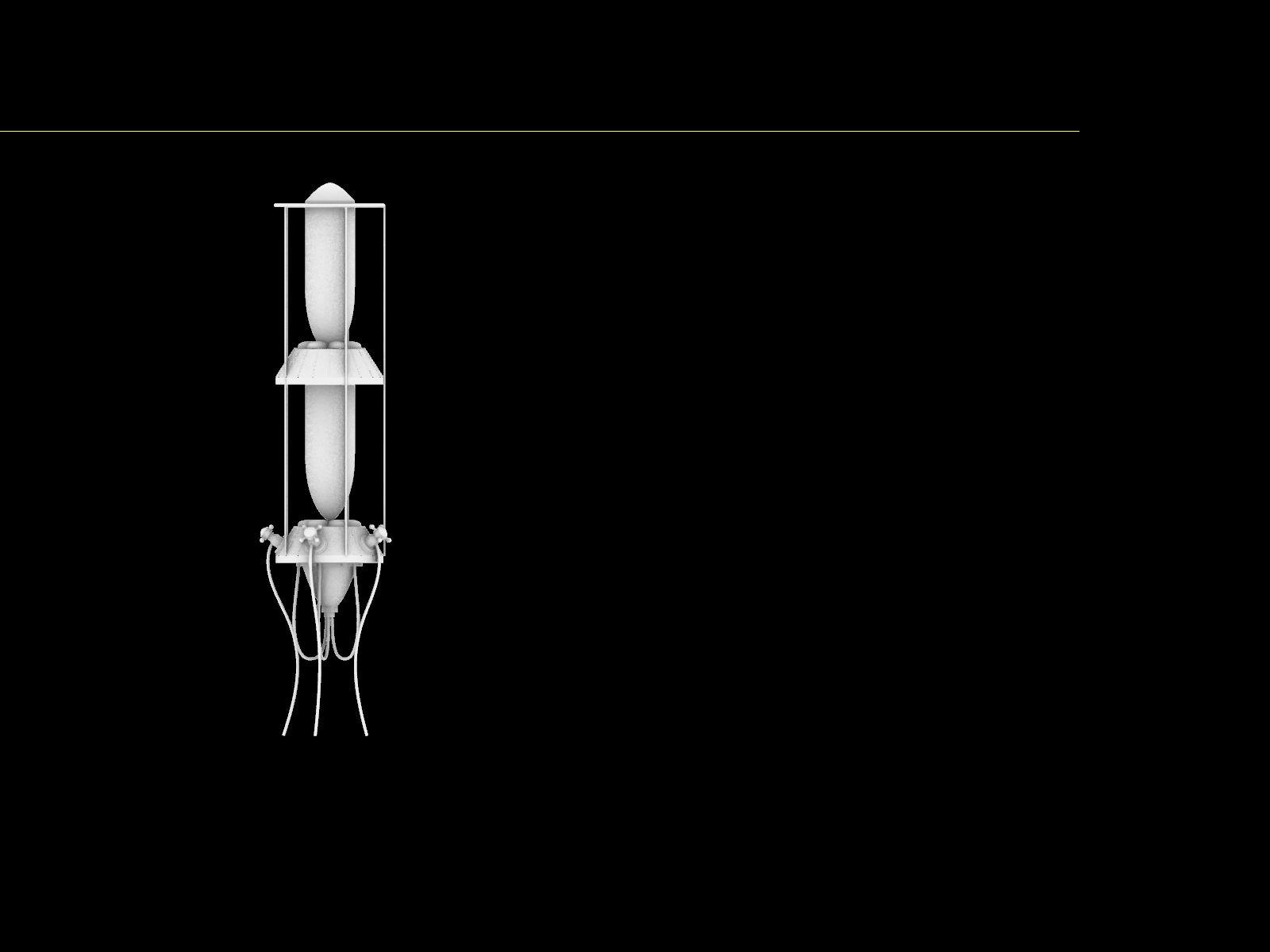
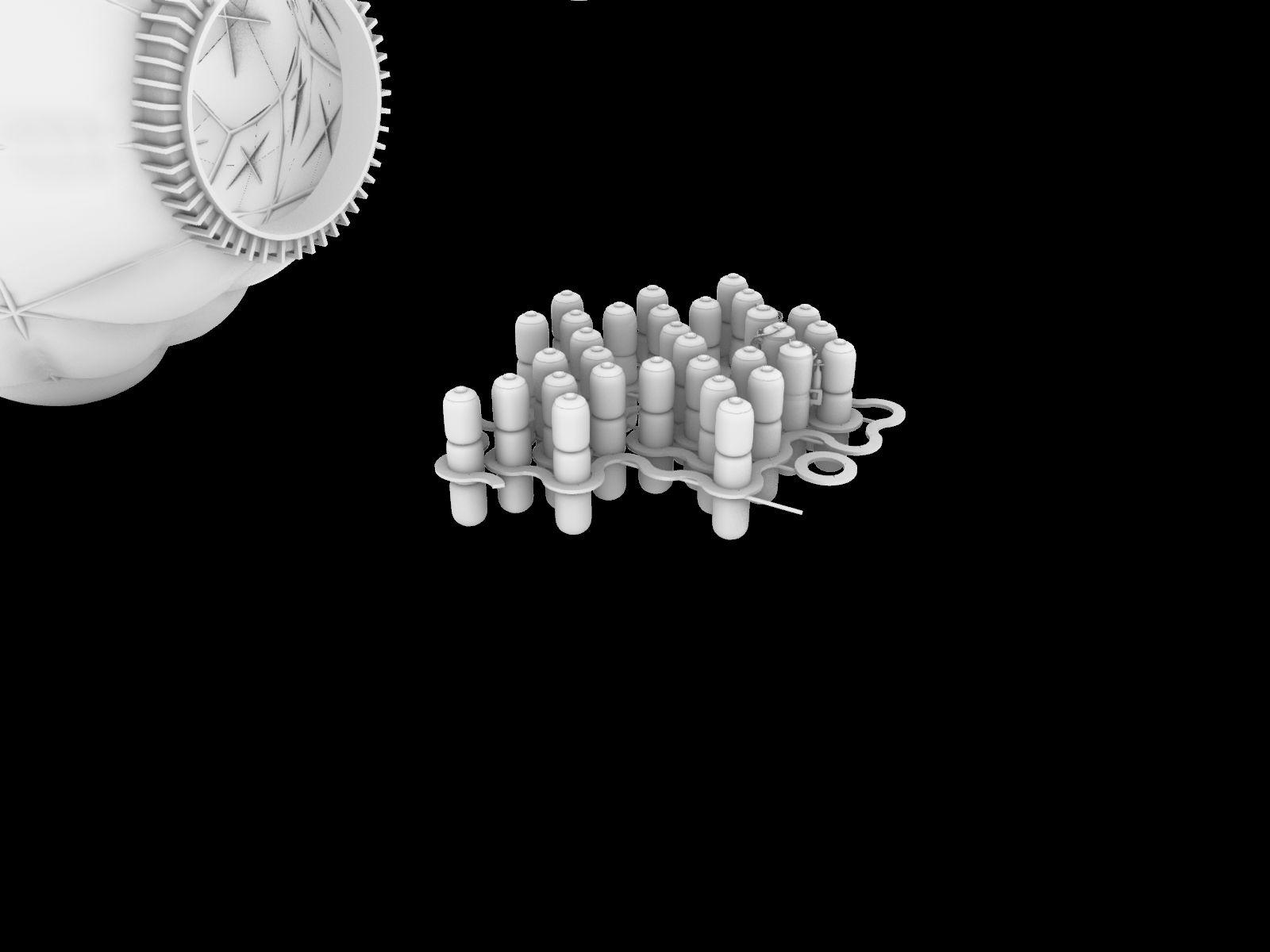
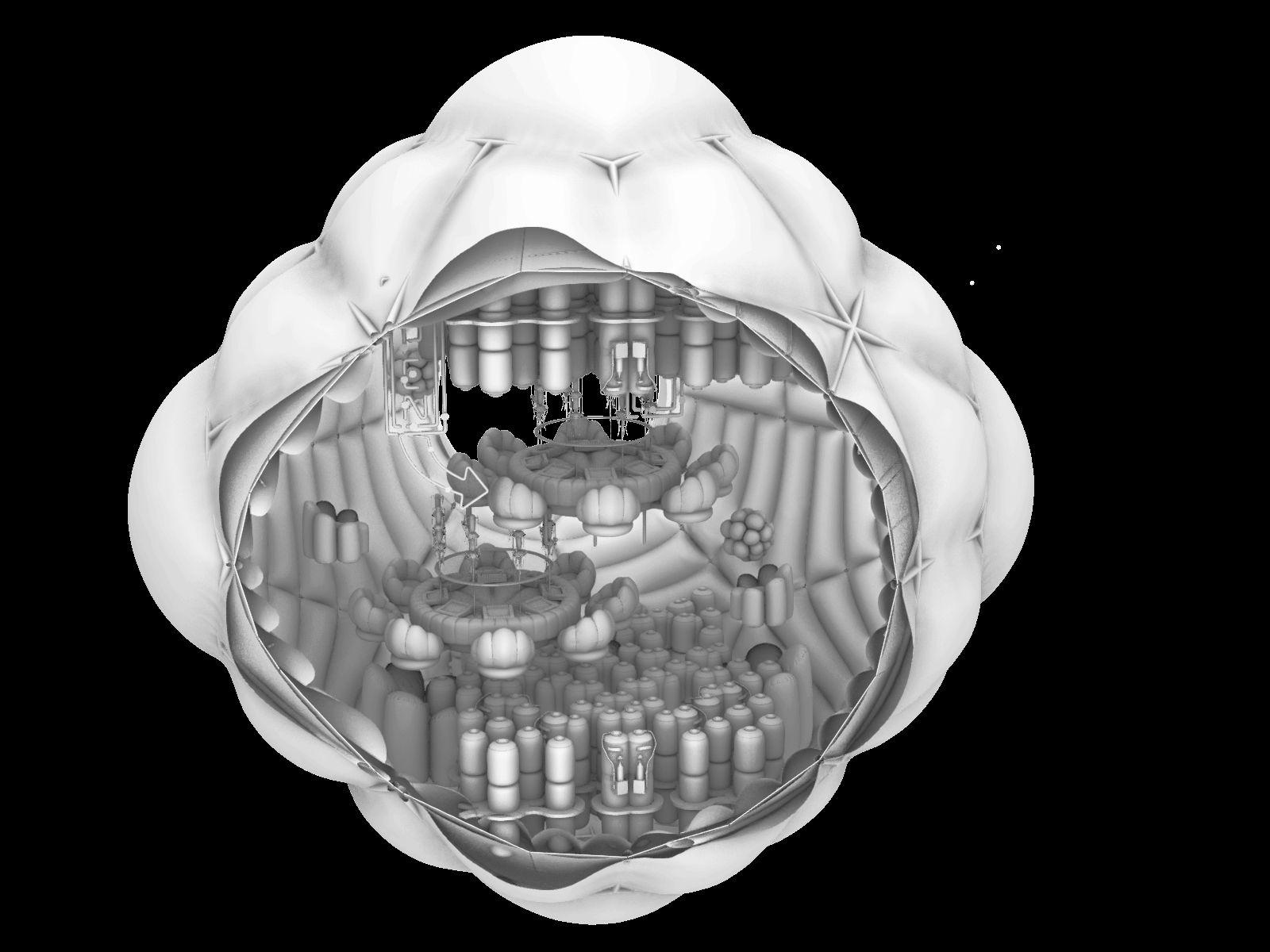
The Casino Module presents a noisy structure with inflatables as seating and table-like furniture. It incorporates space bottles with beverages and food trays designed for space. The interior pattern is fluid, dynamic and colourful. The interior configuration is mirrored, using both sides of the module. The colour of the patterns divides the ground and sky perspective by introducing colours to the patterns, representing a cold but vivid environment. It is based on the night module.
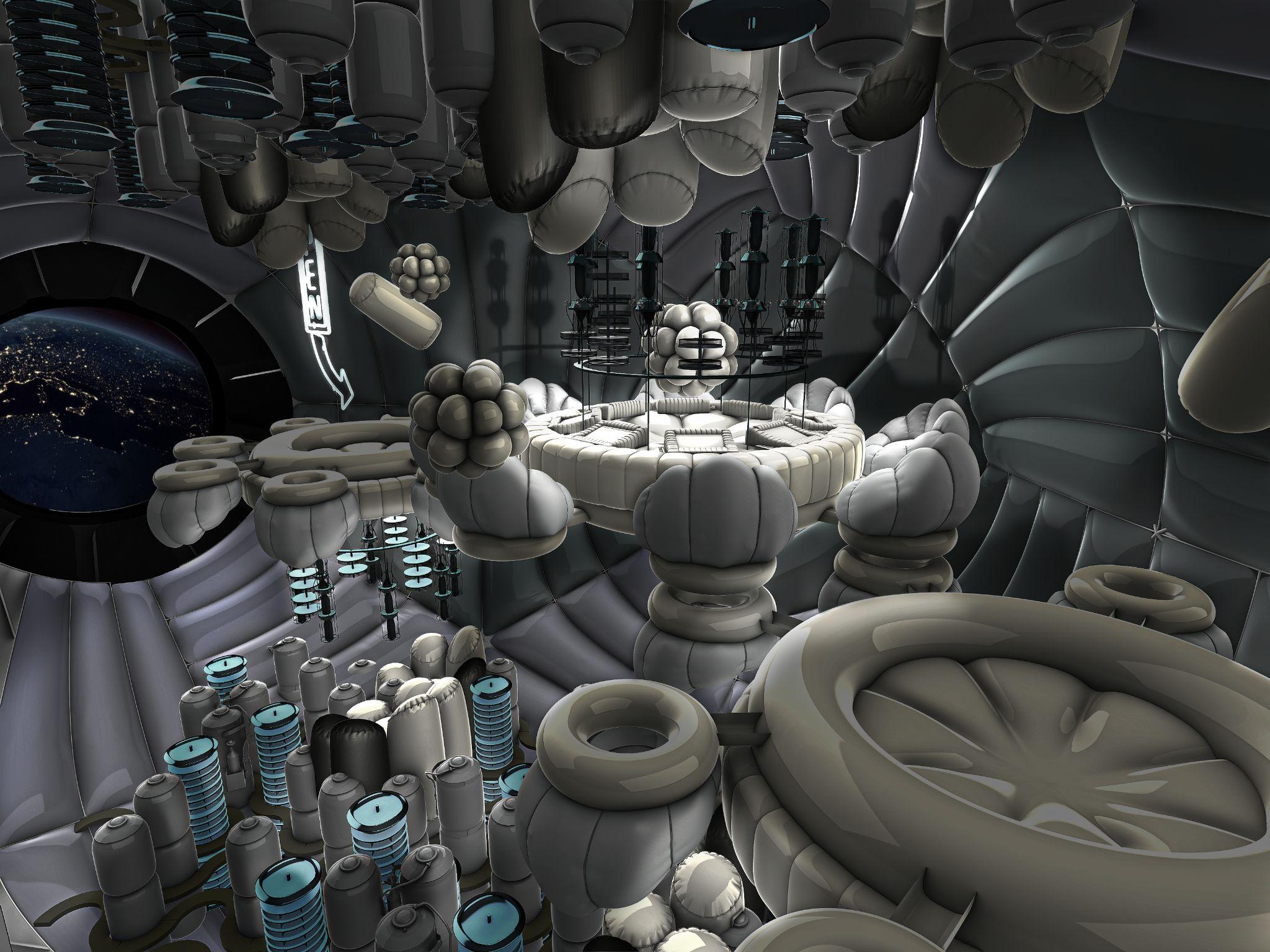
NEBULAE
Casino Module Documentations
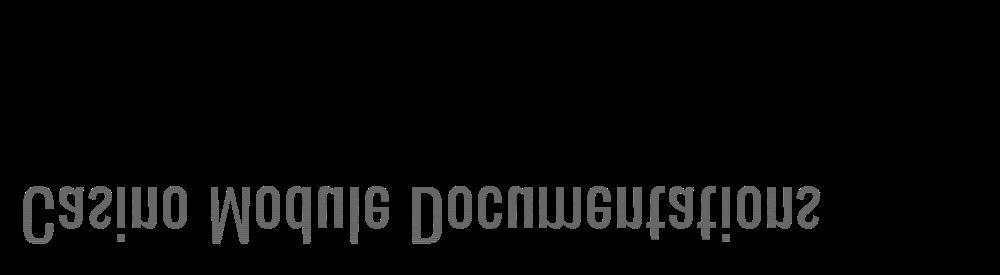
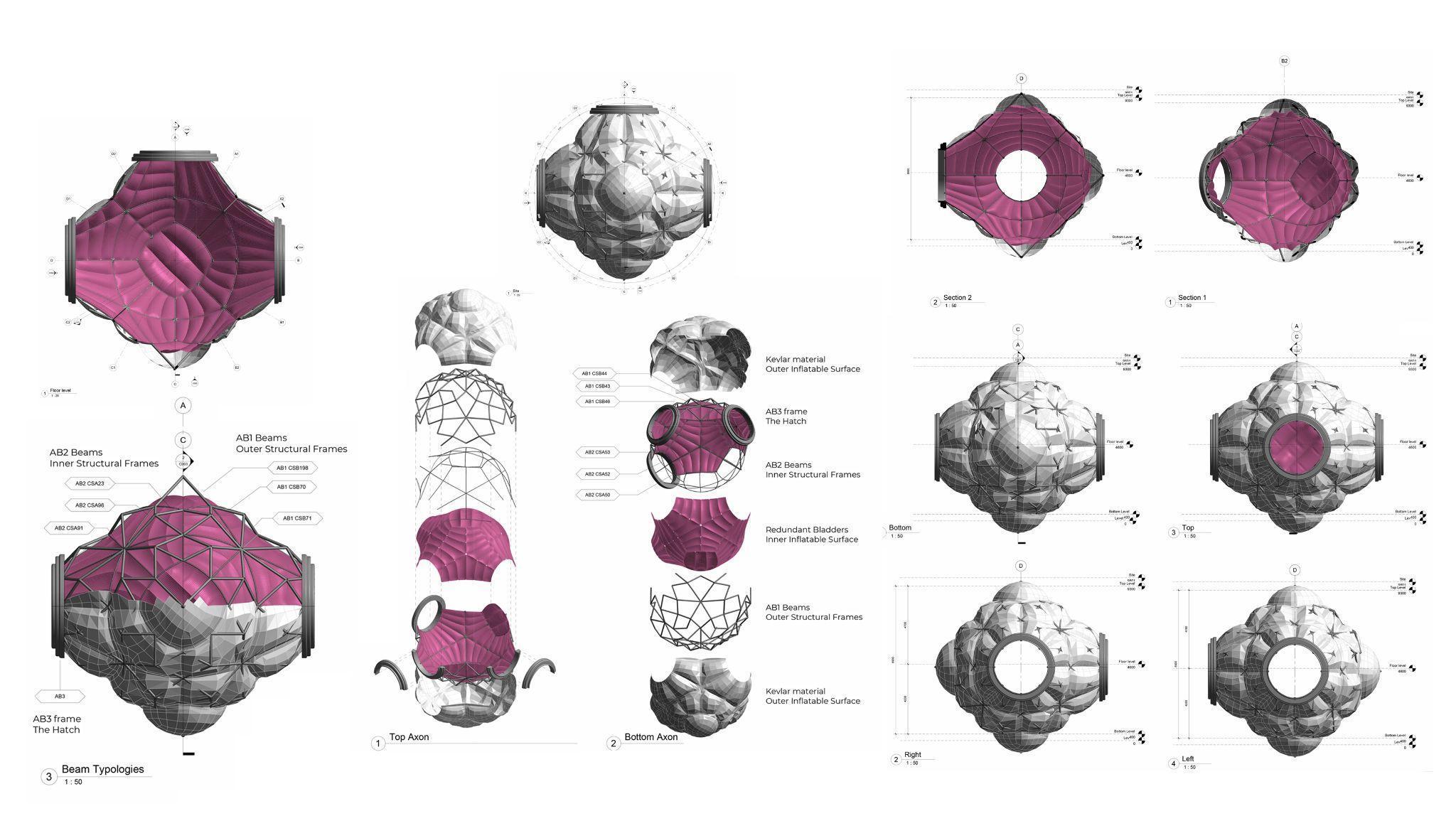
Using Revit 3D, and Rhino.inside
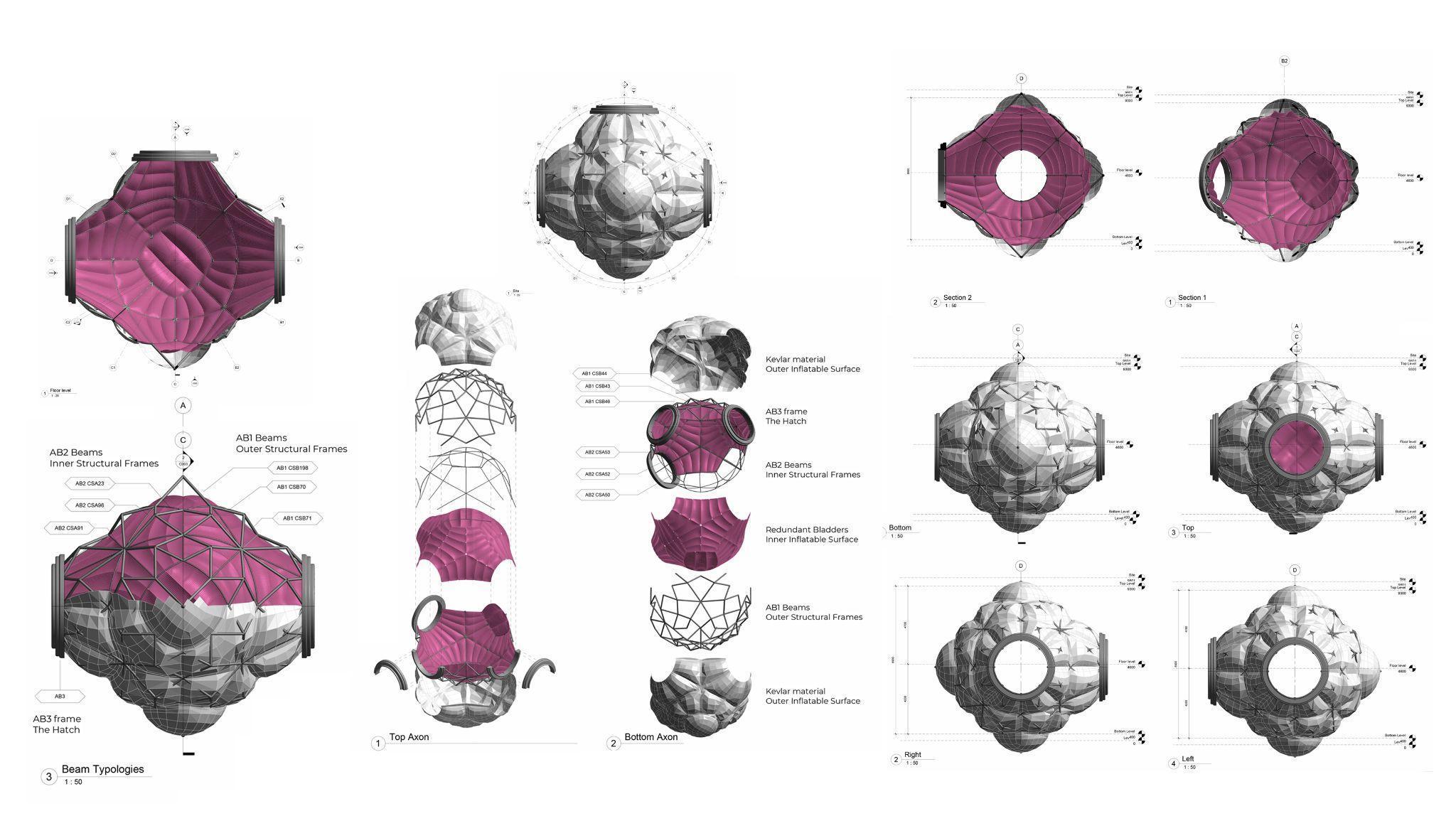
NEBULAE
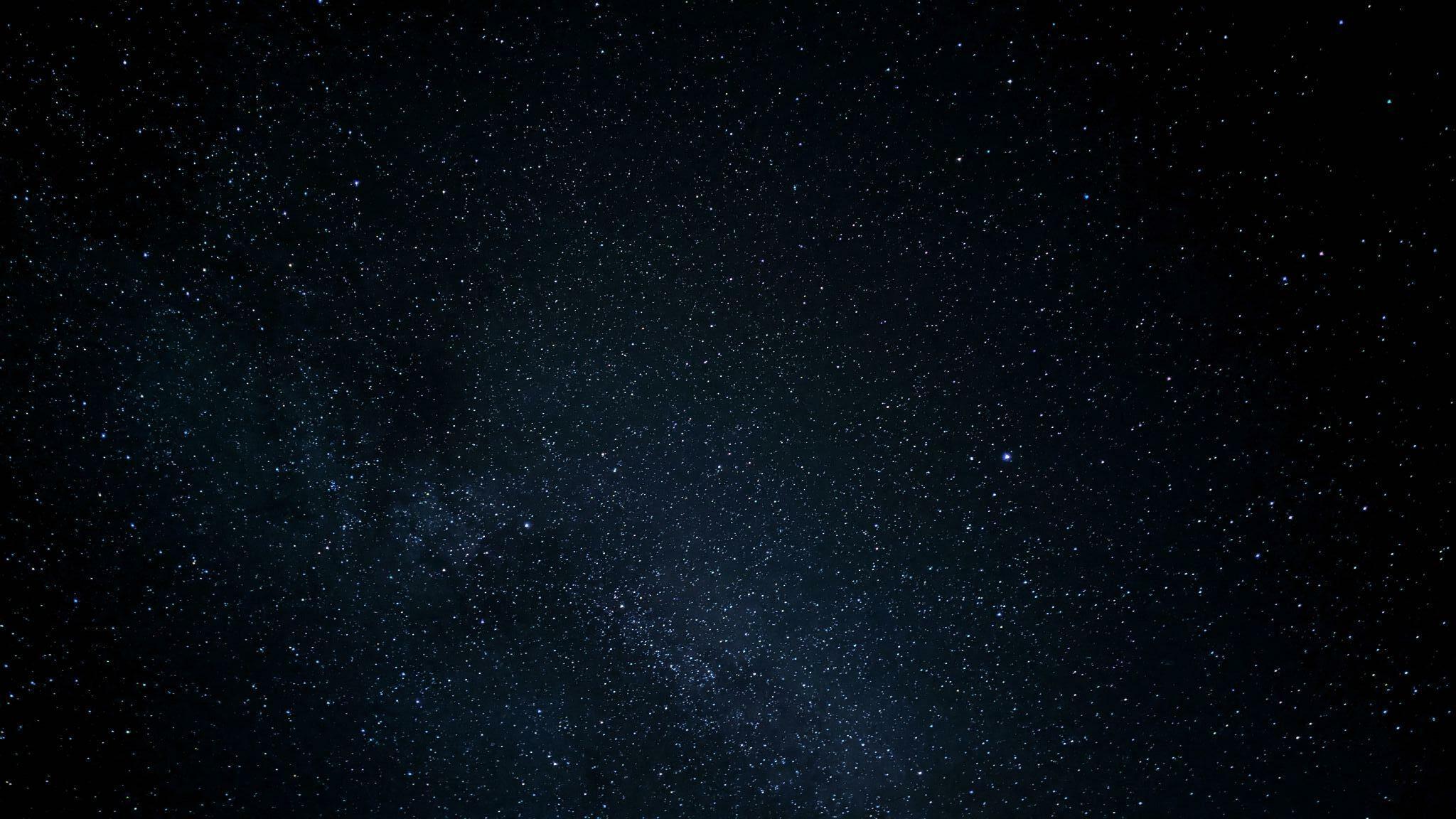
Gaming Module Documentations
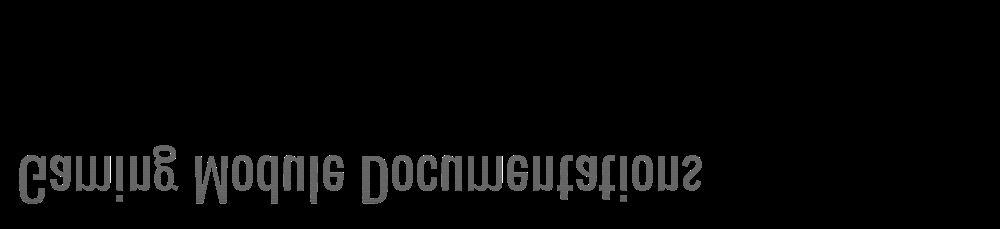
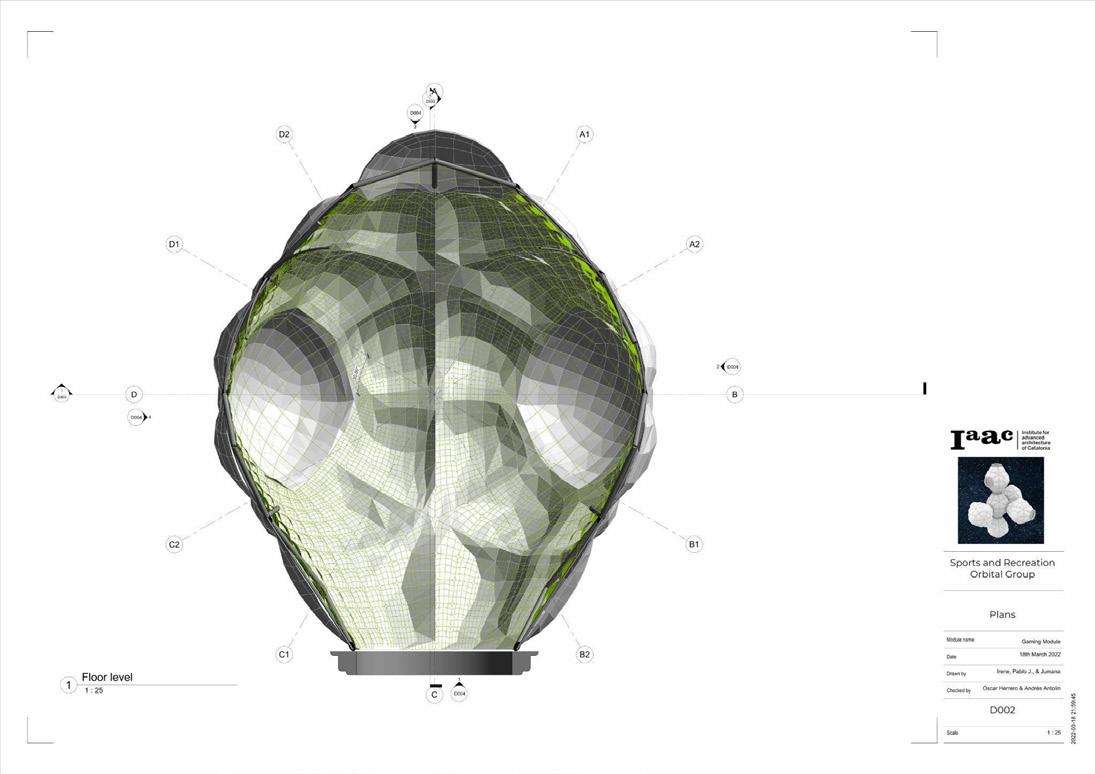
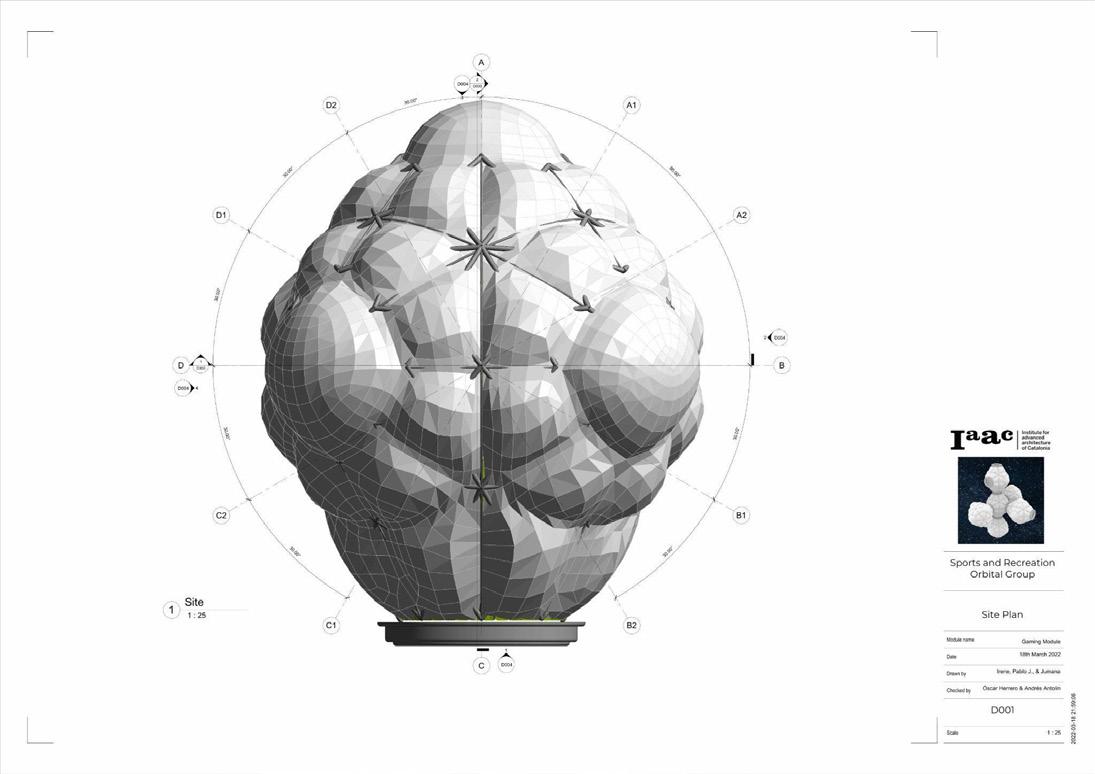

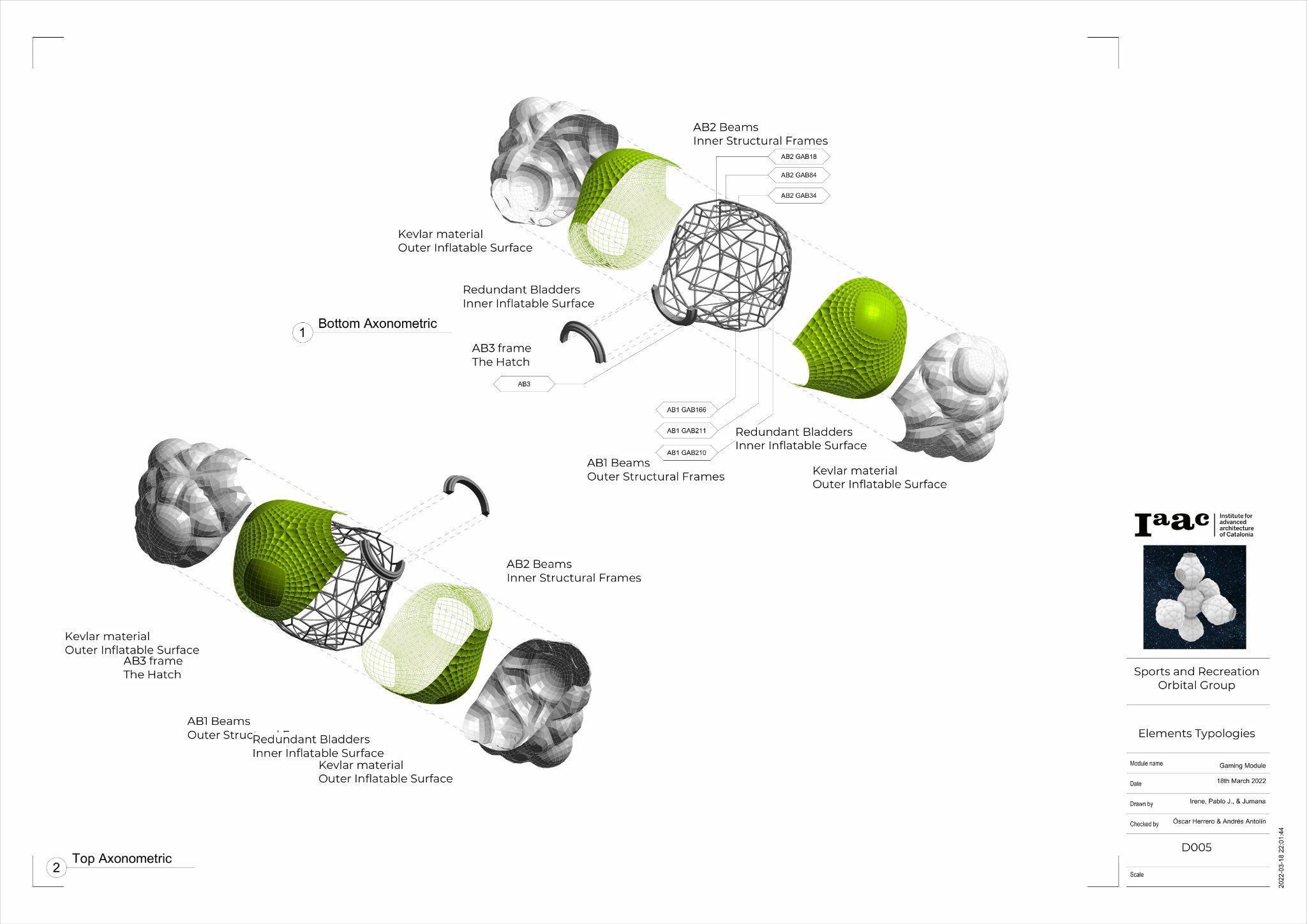
Gaming Module
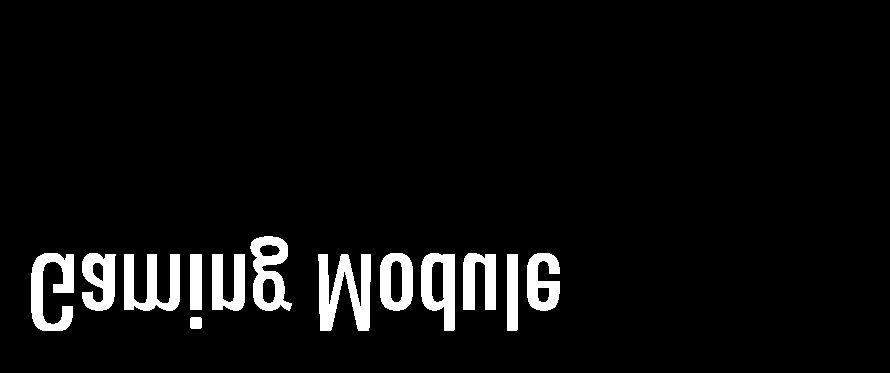
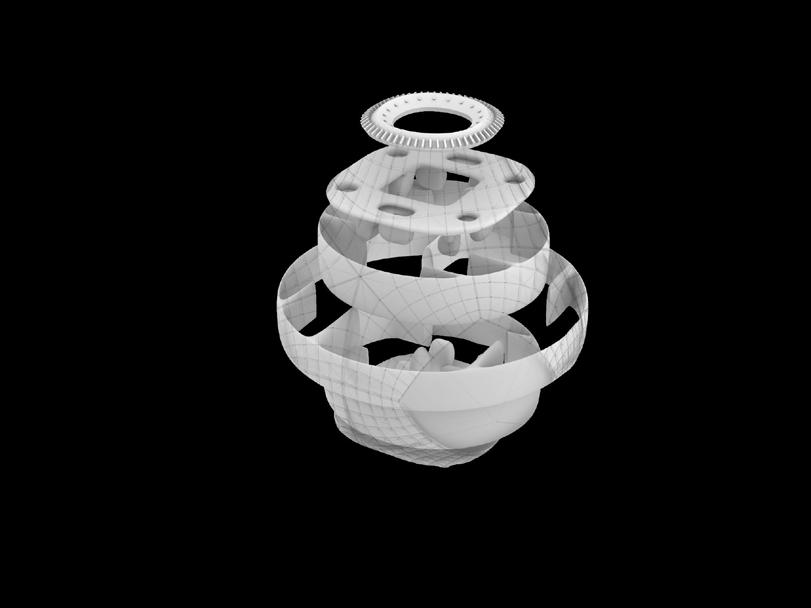
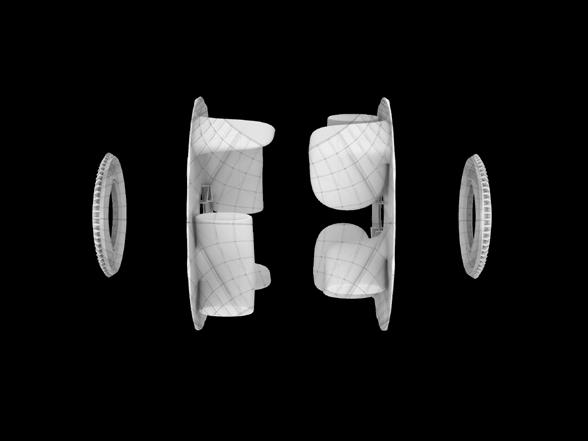
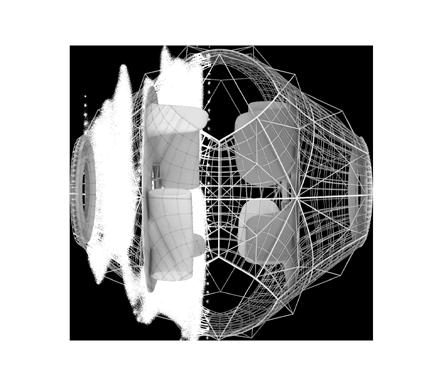
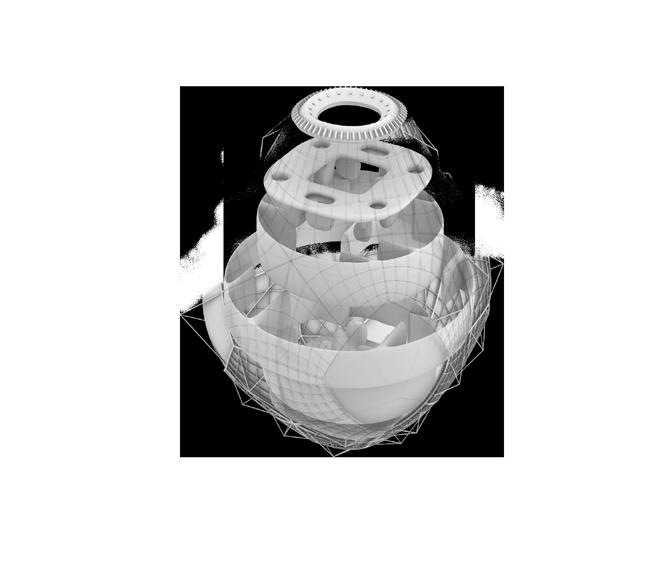
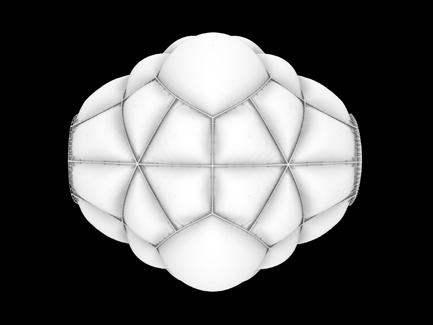
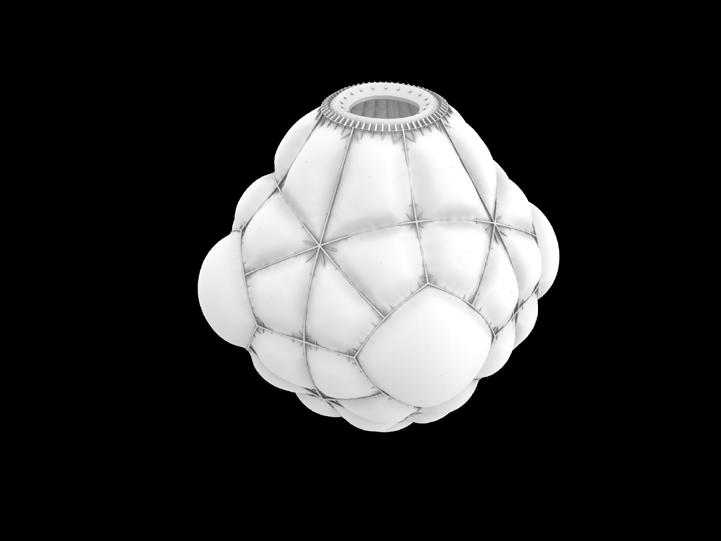
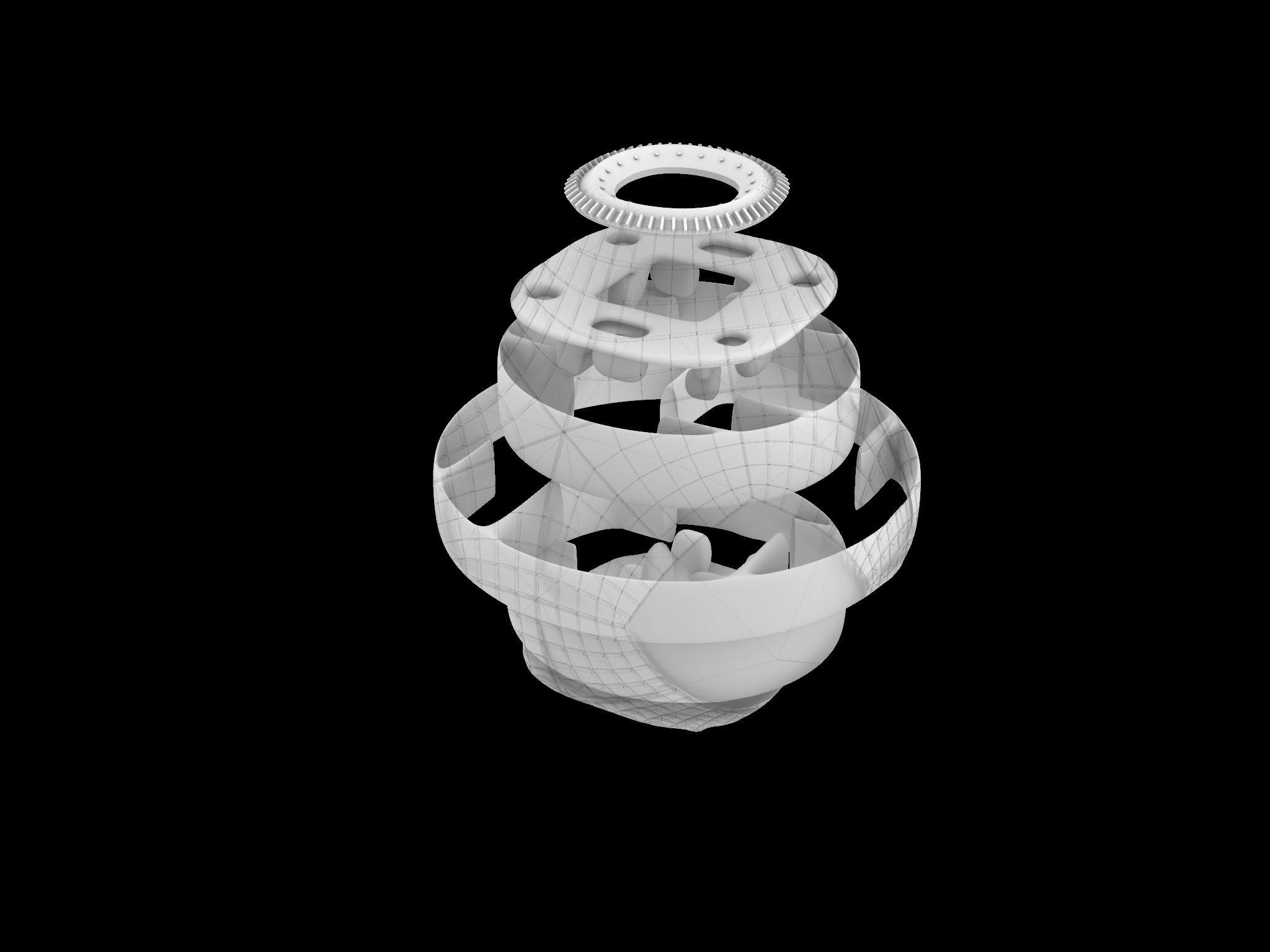
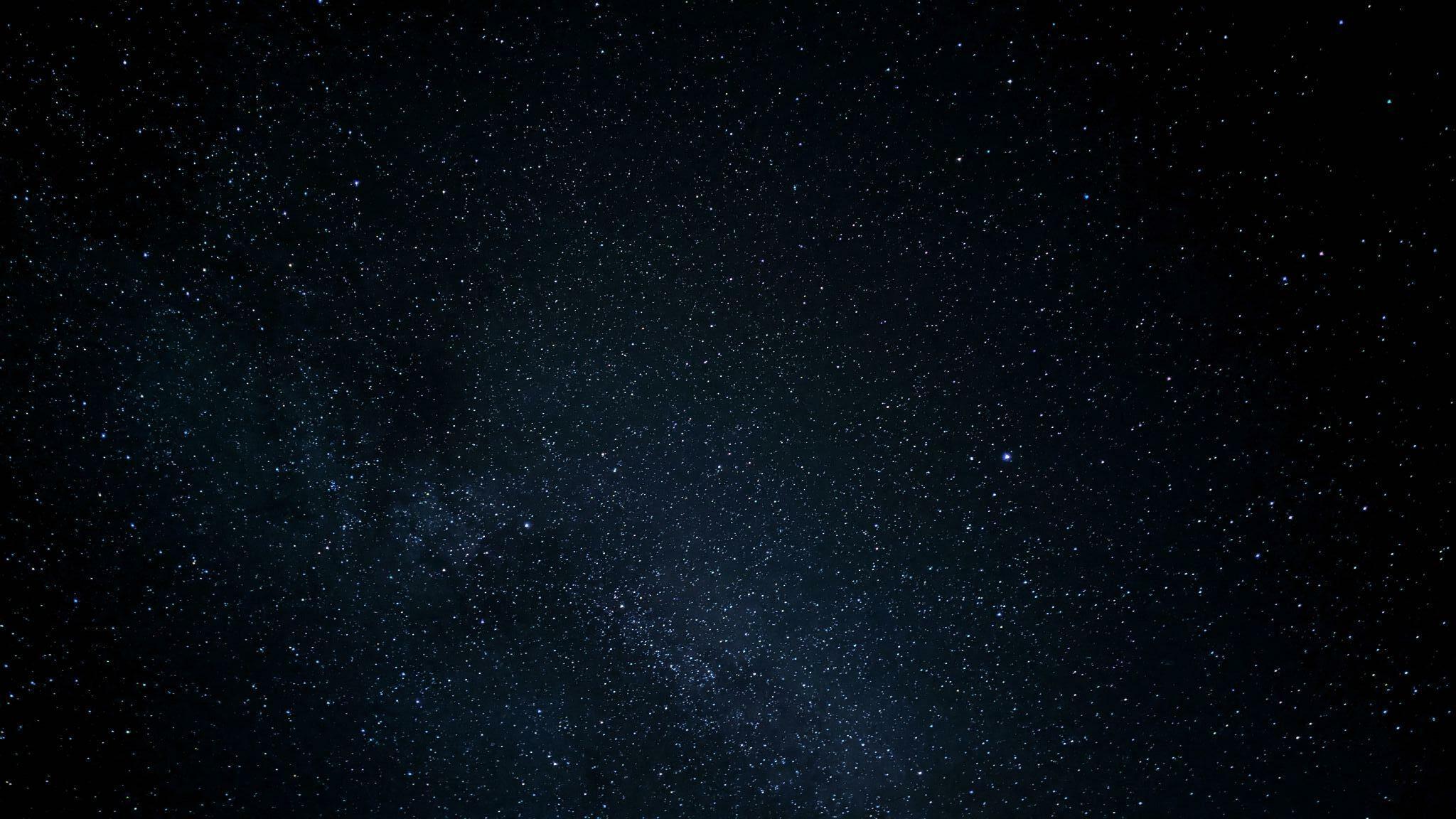
NEBULAE
Nebulae: Orbital Space Habitat
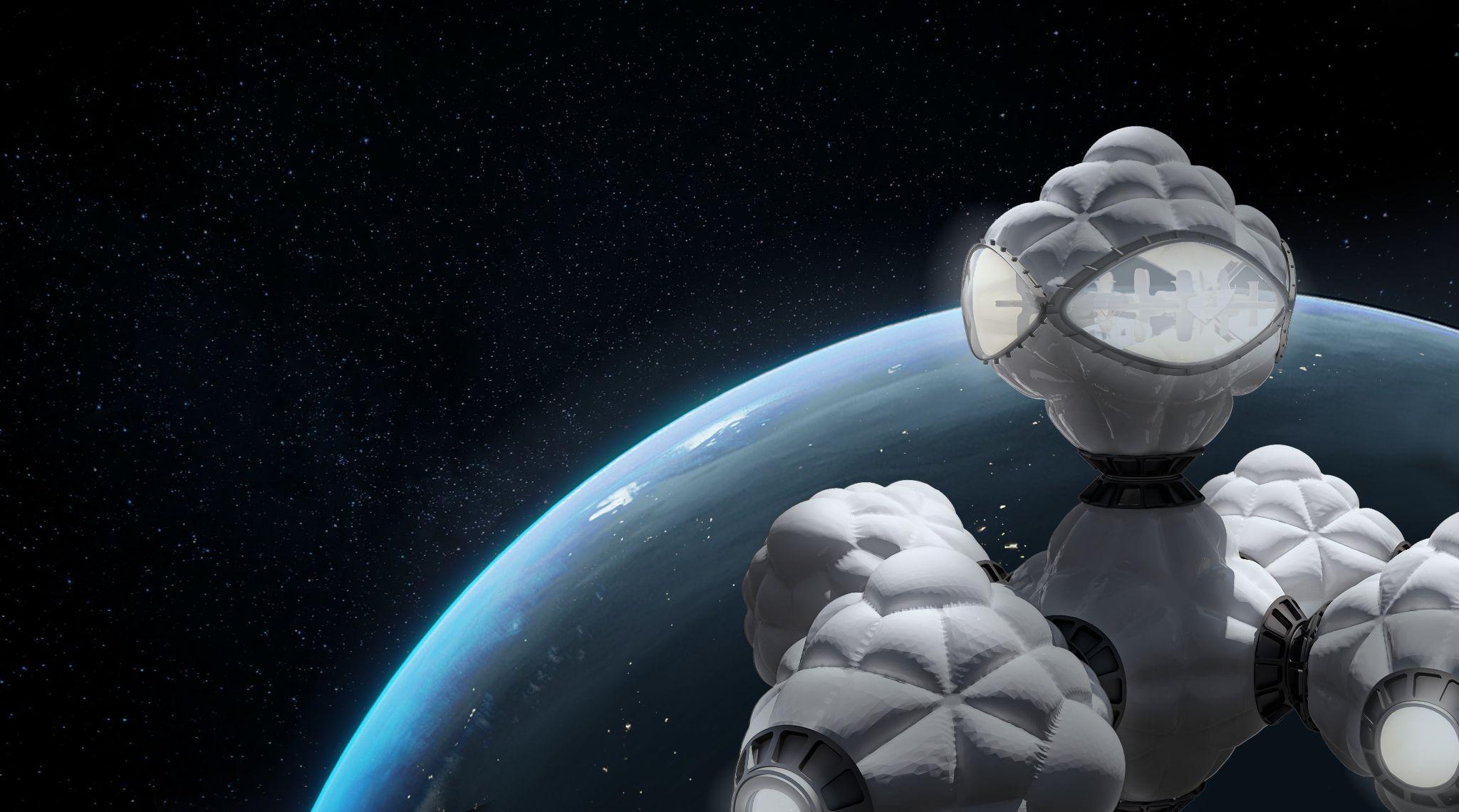
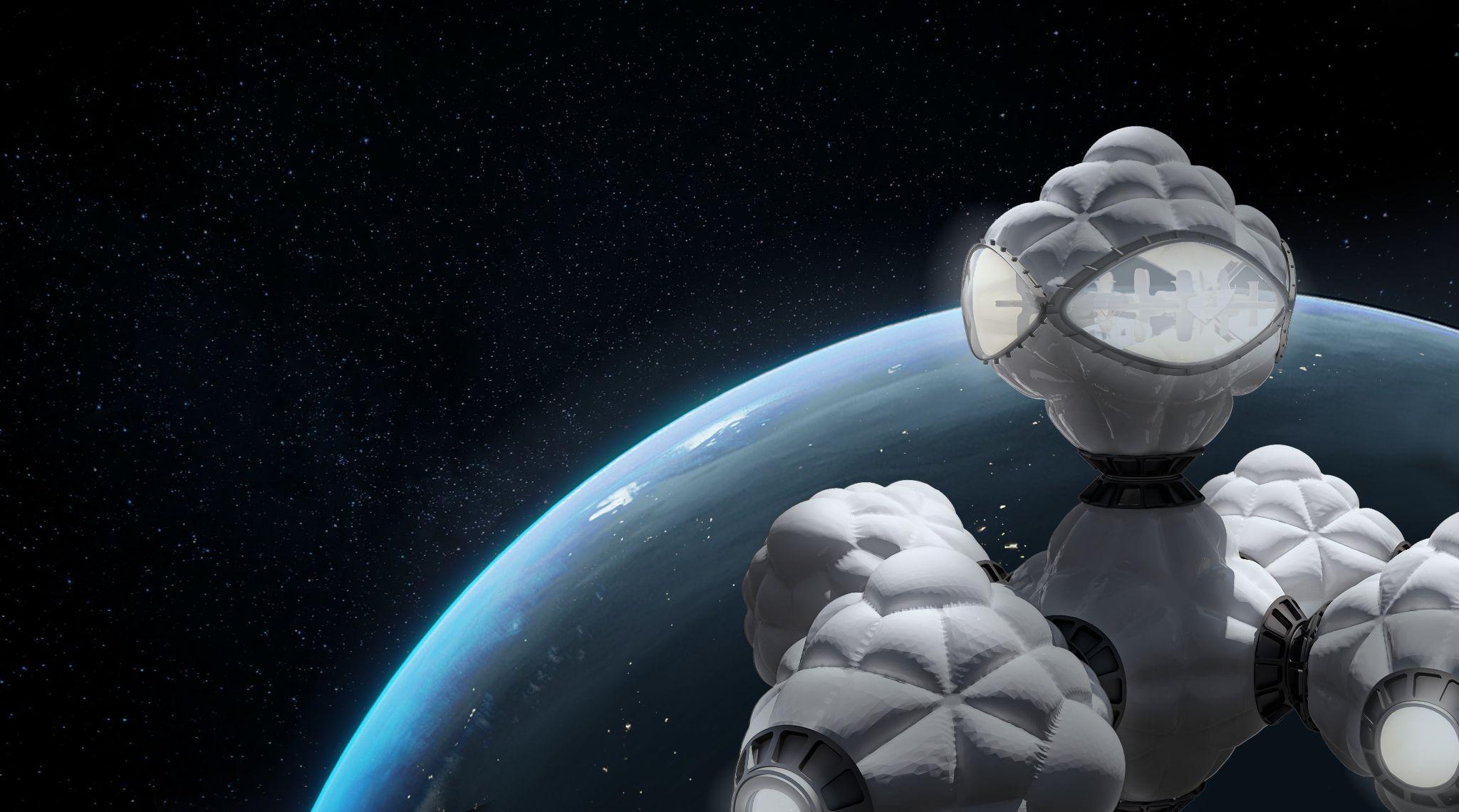
FUZZY
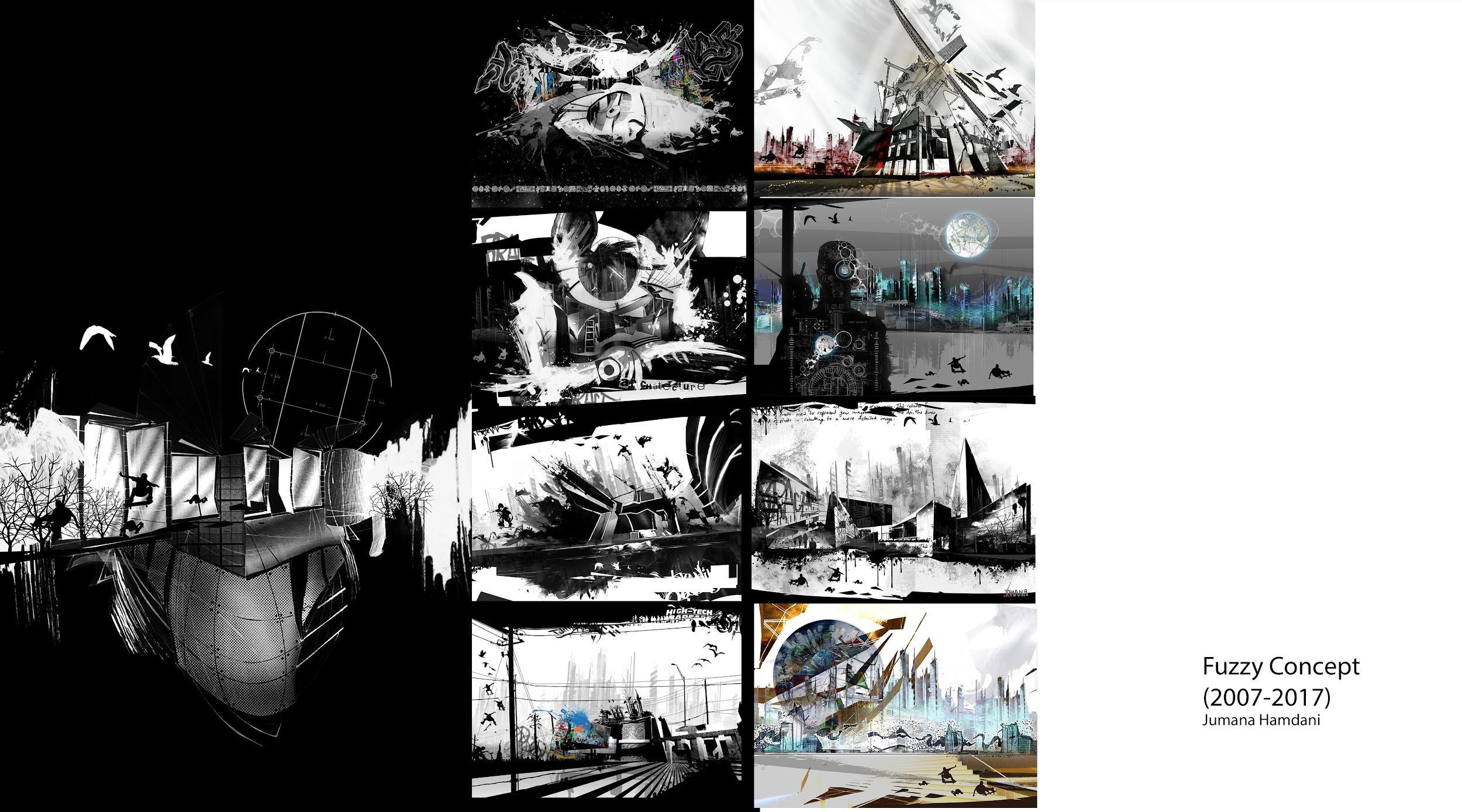
What’s beyond concept…
A fuzzy, lacking a fixed vague, uncertain concept with all the objects, colors, distinguished elements being sometimes meaningless altogether... The point is not to distract the viewers but to let them think out of the limitations of a concept... like kids in early stages they observe things in their primitive senses but through practical experience they learn to identify and build a correct application of an idea, then related it to other concepts. Now that stage before concept called "a fuzzy concept" which could play a good role in the creative process of forming new ones to understand something... and that's what makes a concept genuine in practical stages.
We must emphasize on the need of "research" using graphic, paintings, digital, etc. experiences as a "laboratory” to create exceptional buildings. And here where we find "problems"... innovative dynamic, fluid, alien or even complex structure shouldn't be a barrier. We should keep on researching in a "Designs cannot be built", that problem could solve the link to what's next!
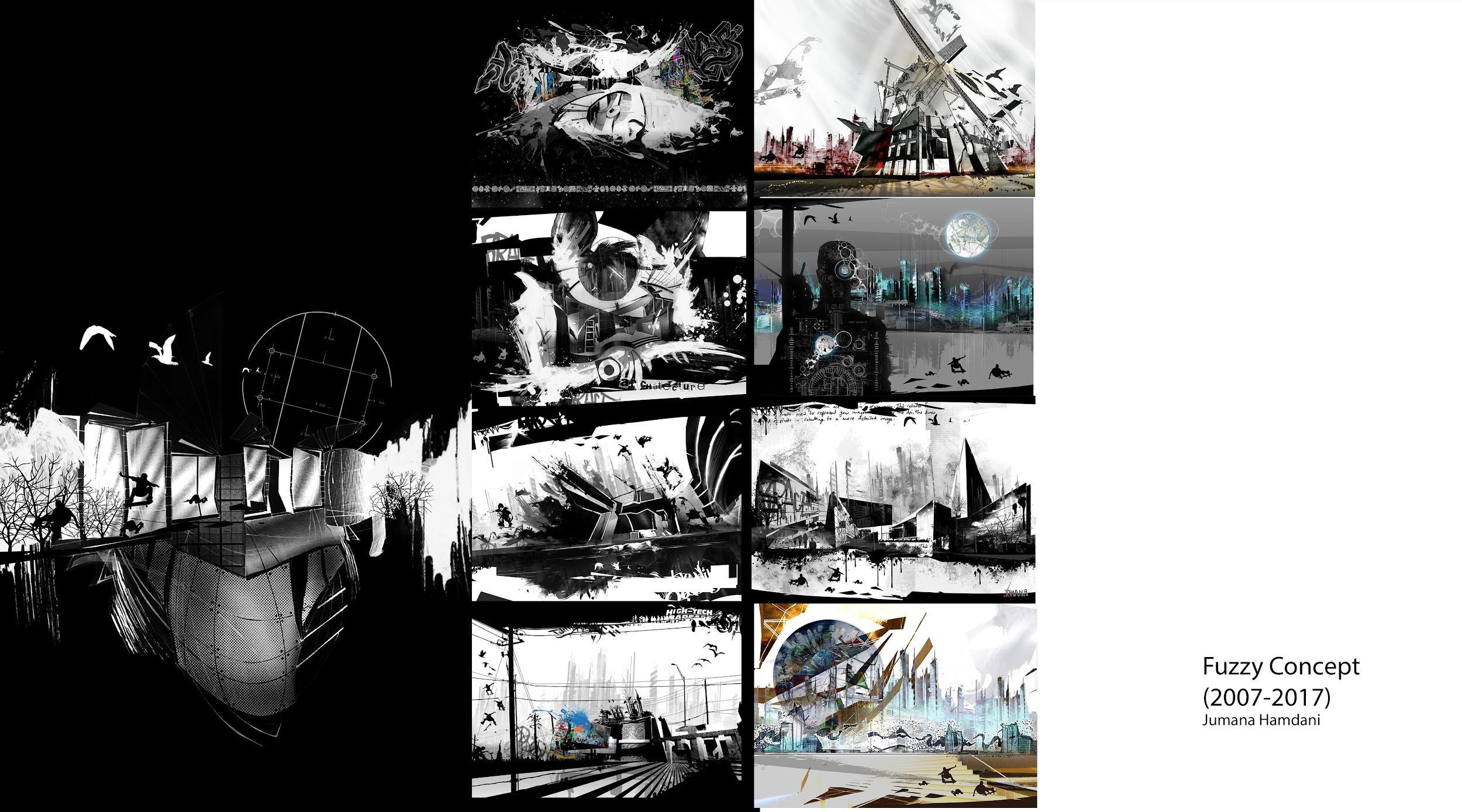
ARCHITECTURAL FANTASIES 22
Date:2007-2017
Project Type: Sketching
Tools: Hand drawings: Watercolors, Ink
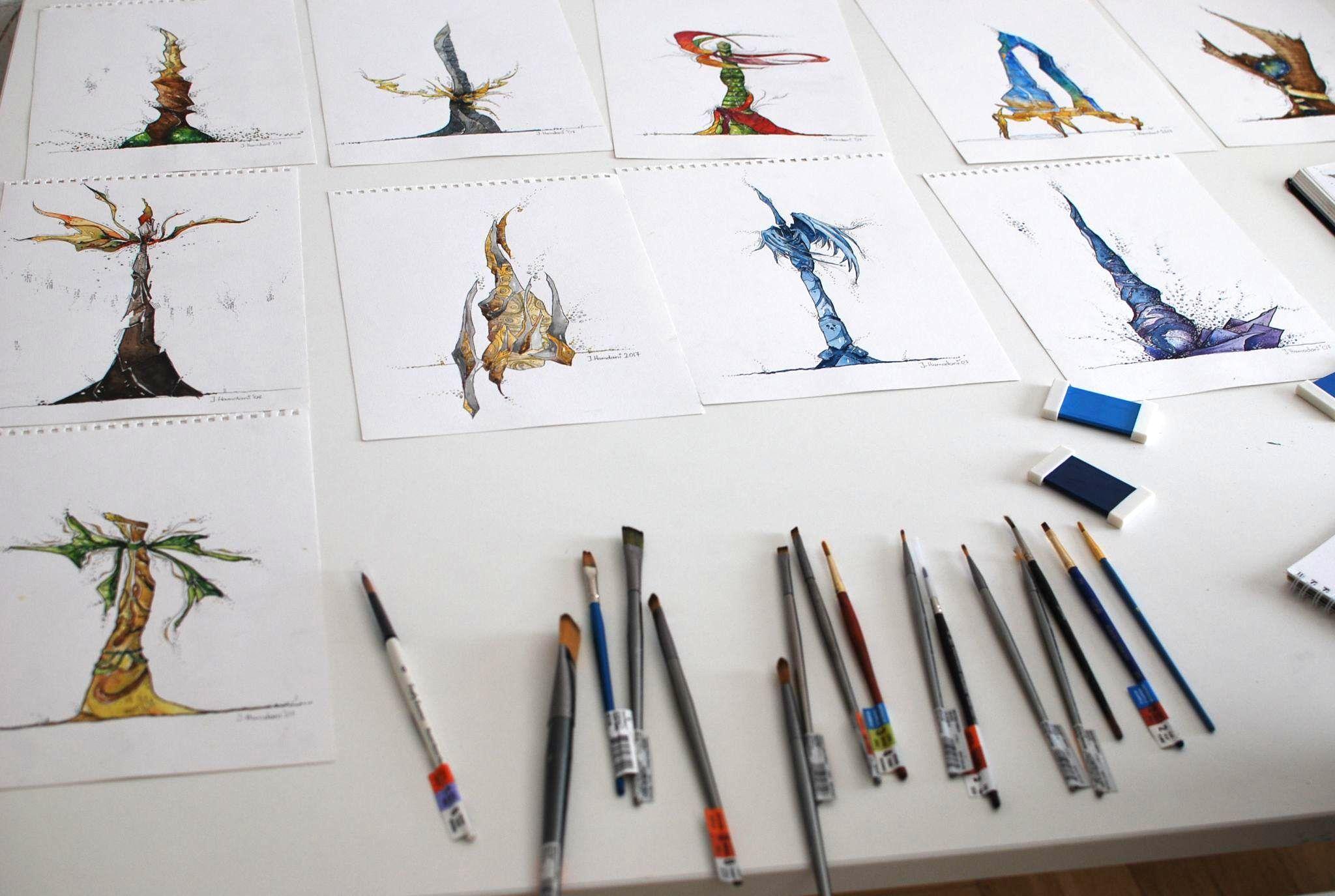
Architectural Fantasies is part of an ongoing project Architectural Series Lab
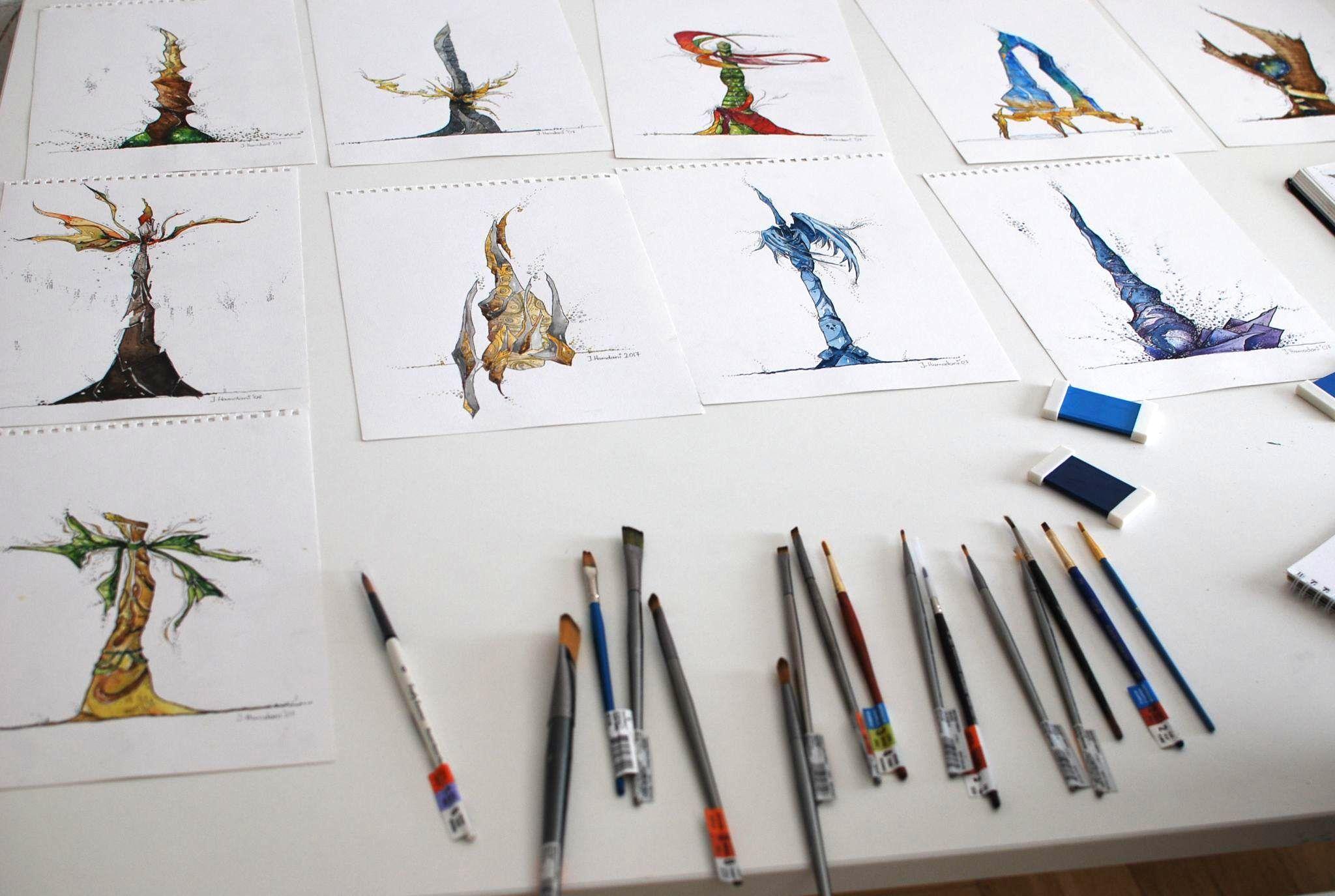
The work is a series of illustrations full of characters, incidents, ideas drawn from war, migration, and diaspora, showing anything seems possible to scramble to repair the damages of the past, to let us imagine to build structures that had been previously inconceivable.
Architectural fantasies also develop the importance of today’s “architect’s research” that stands somewhere between the quest for creative specialness and the need for a network of exchanges with other fields and between the responsibility of the concept and the acceptance of the complexity of our reality (social, economic and anthropological).
The research can play a vital part in the development of current notions about the city as a social organism, the role of technology within it, the importance of beauty and the future survival of higher numbers of people in the context of consuming natural resources.
ARCHITECTURAL FANTASIES
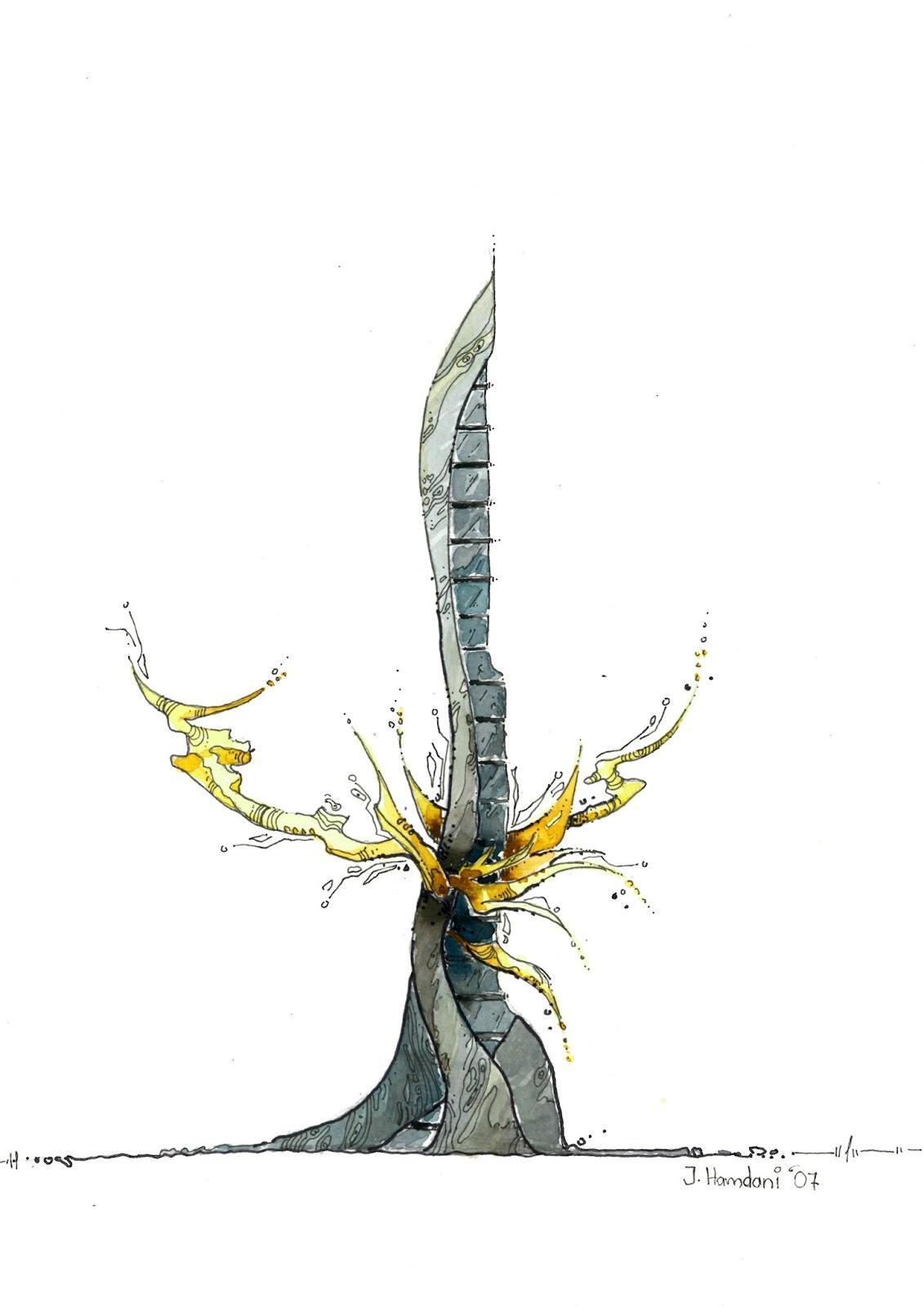
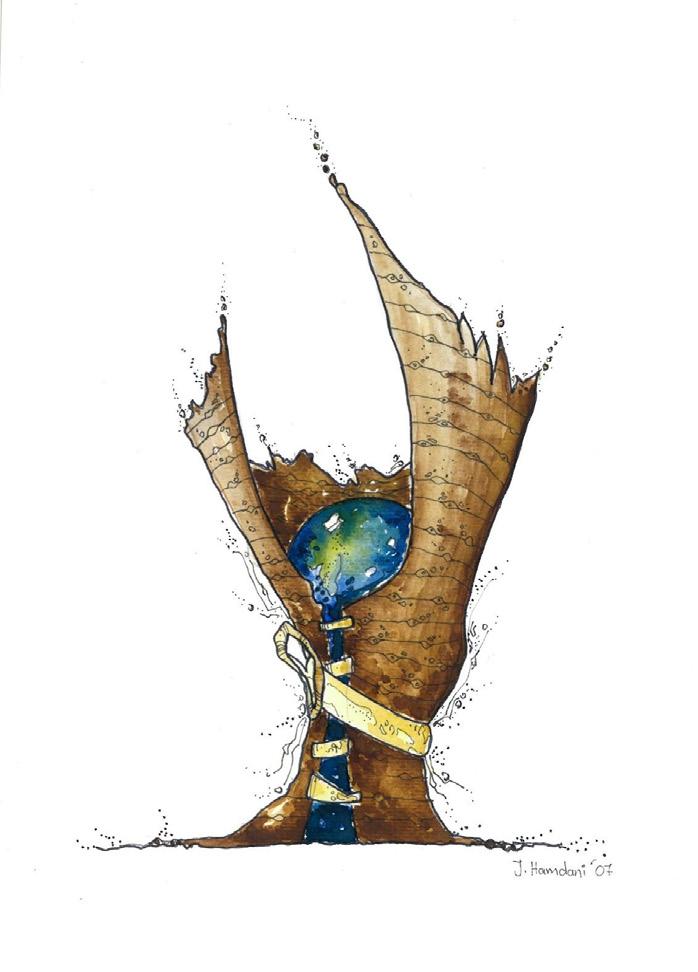
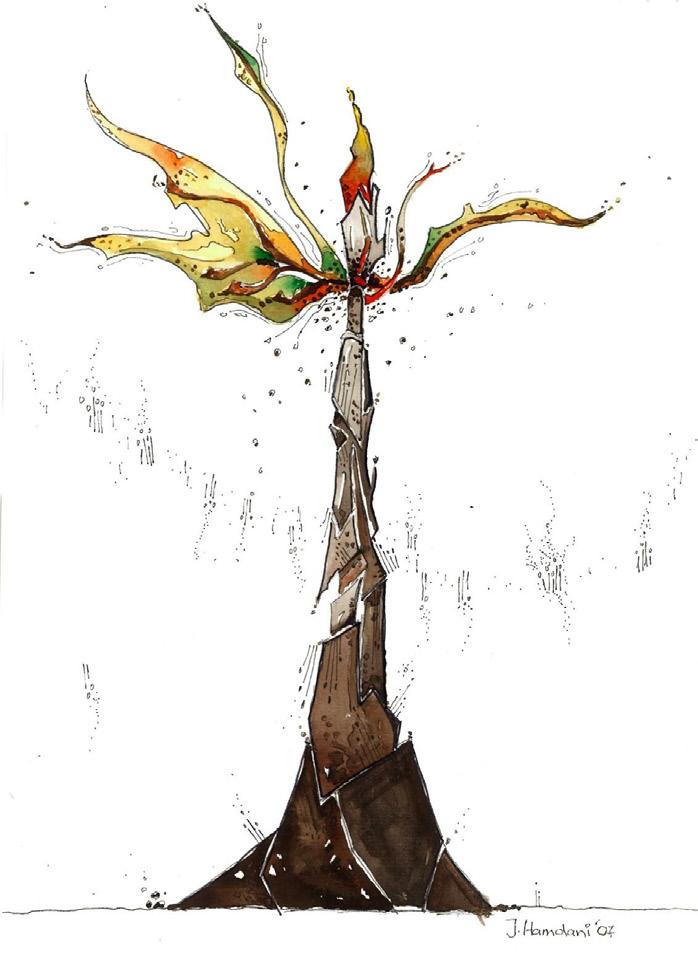
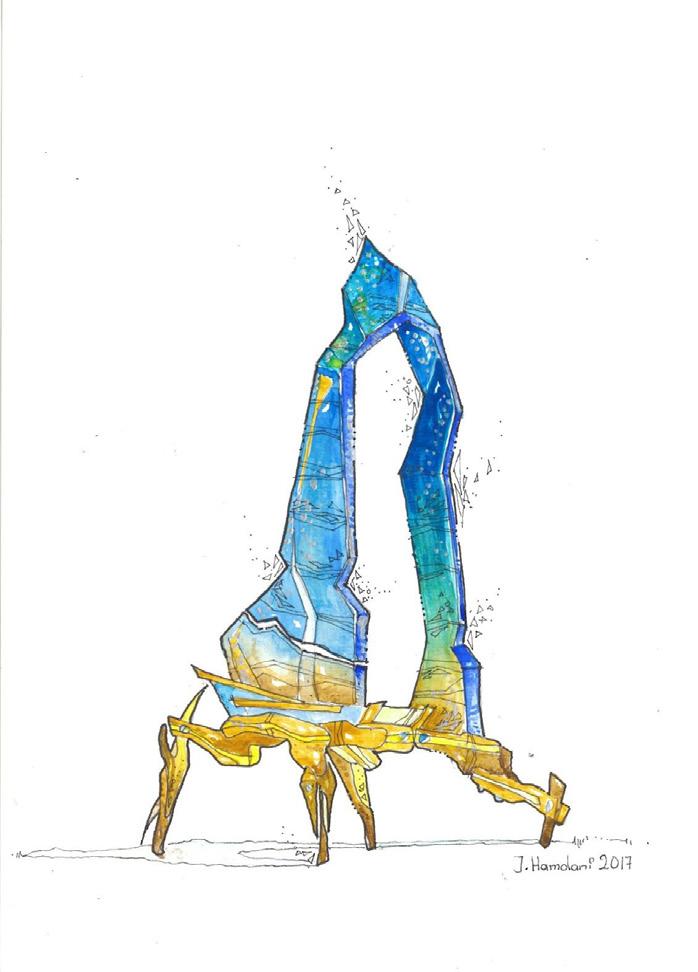
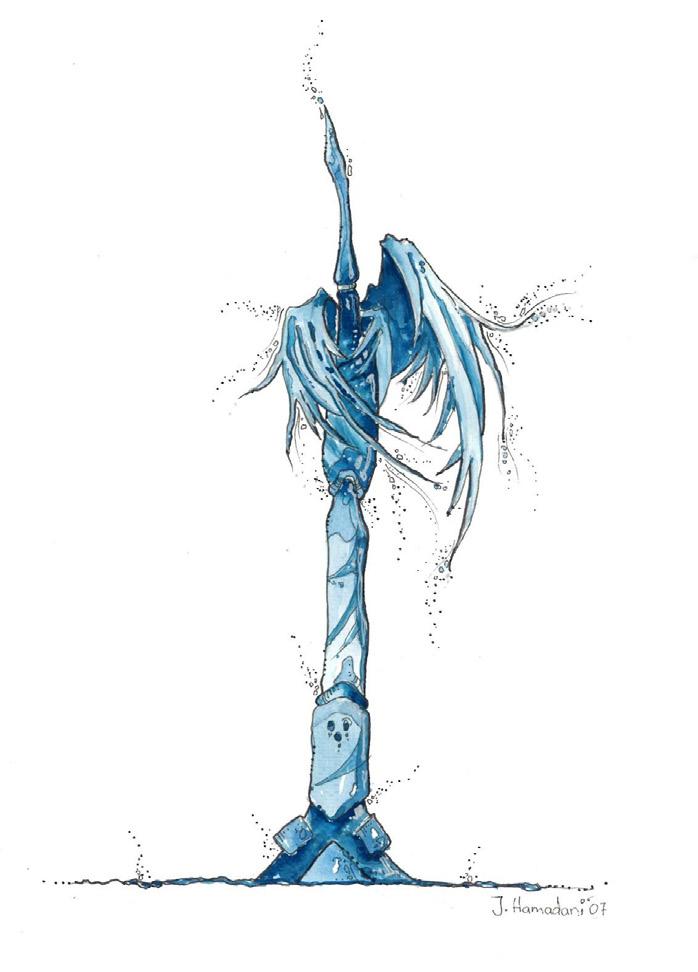
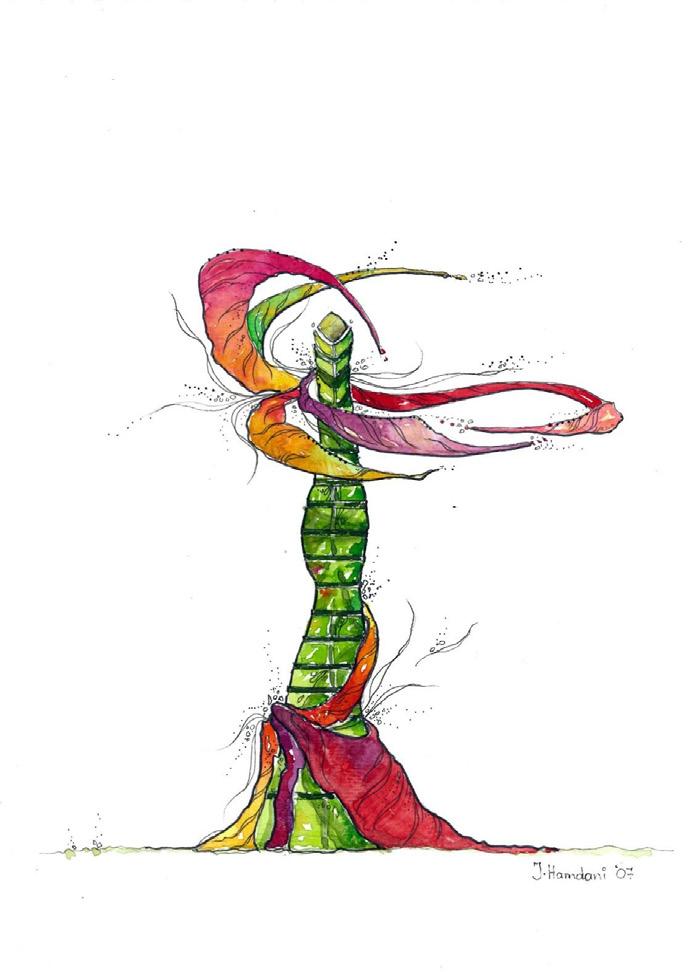
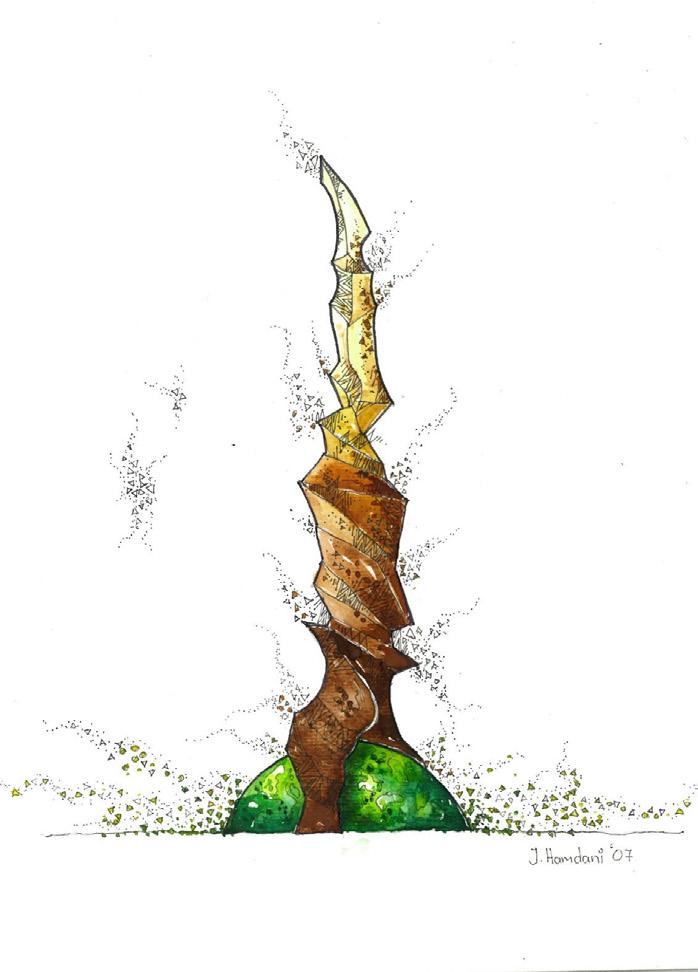
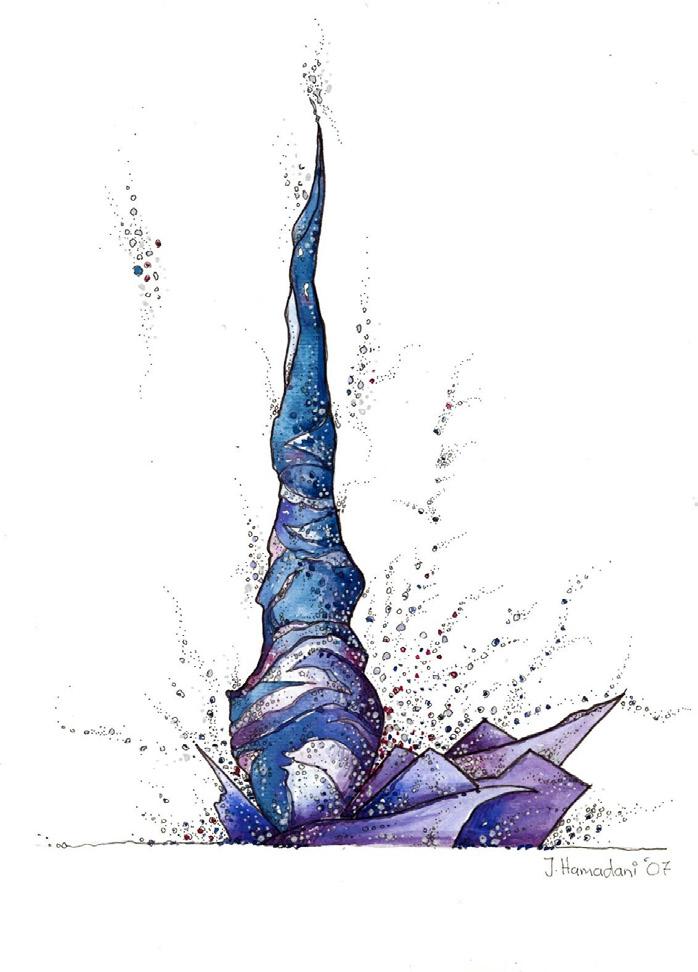
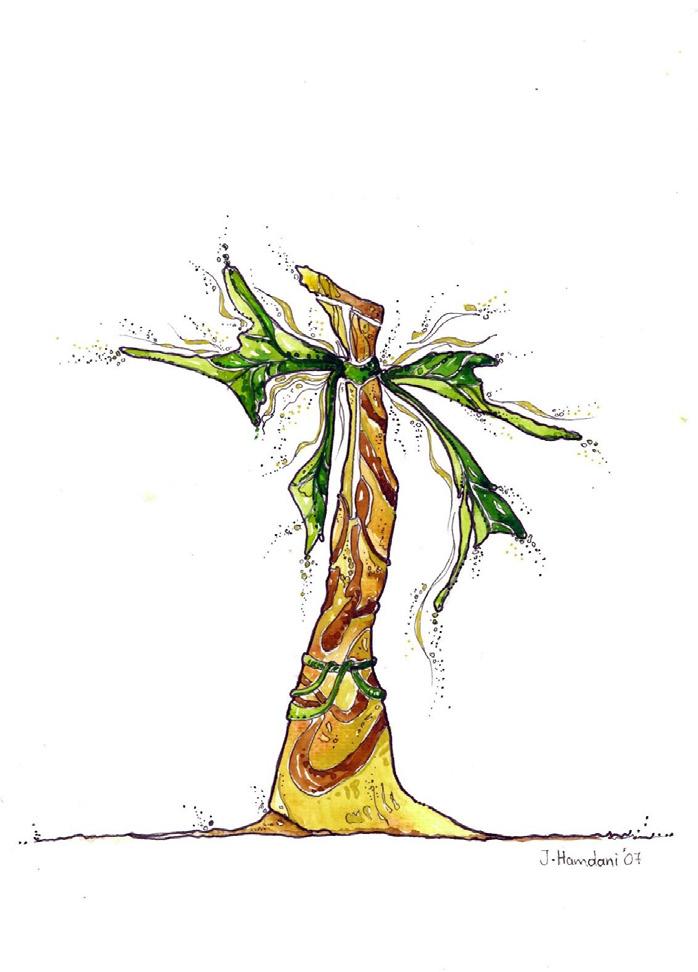
RESEARCH TRIP 23
Date: 2022
Location: Valldaura Self-Sufficient Labs - IAAC, Barcelona, Spain
TOVA
Spain’s first 3D printed building using earth and Crane WASP
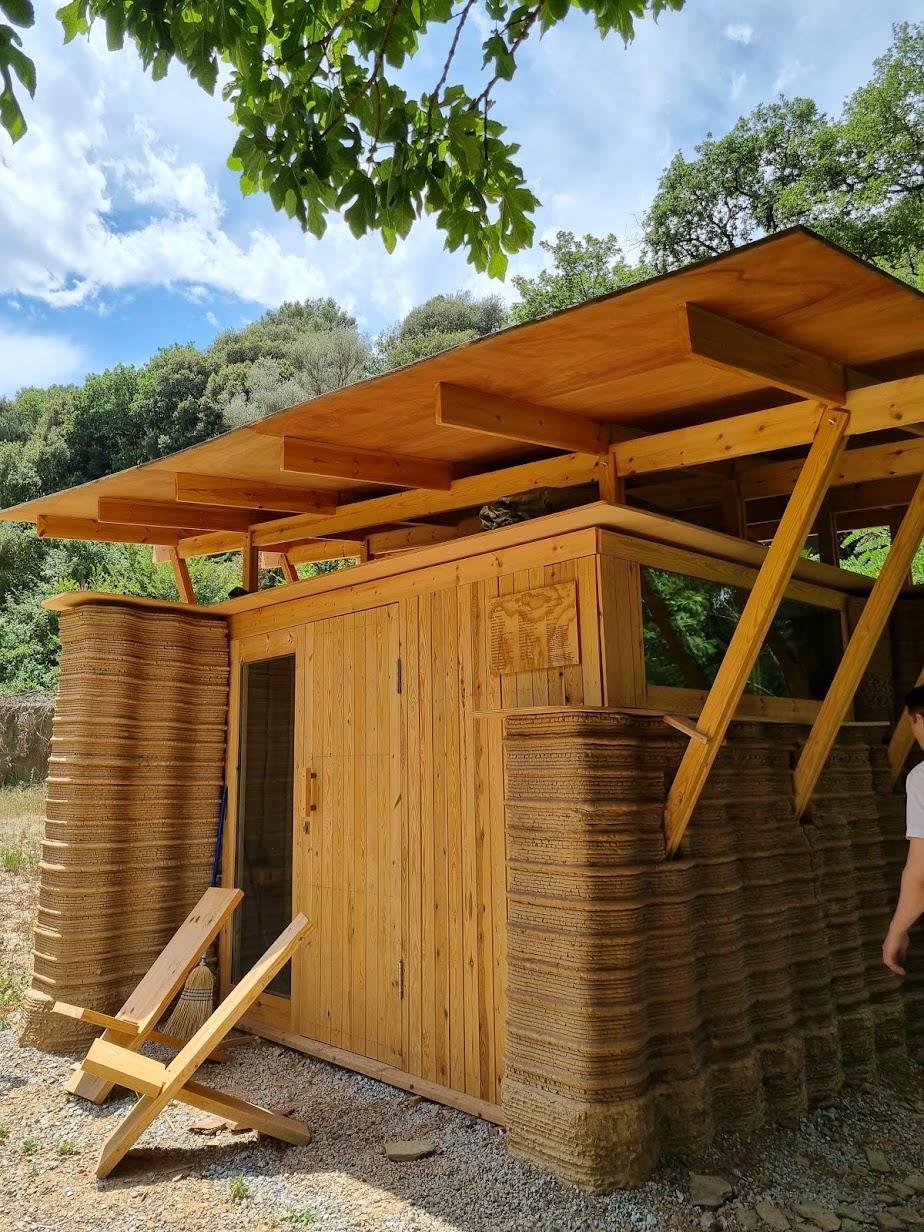
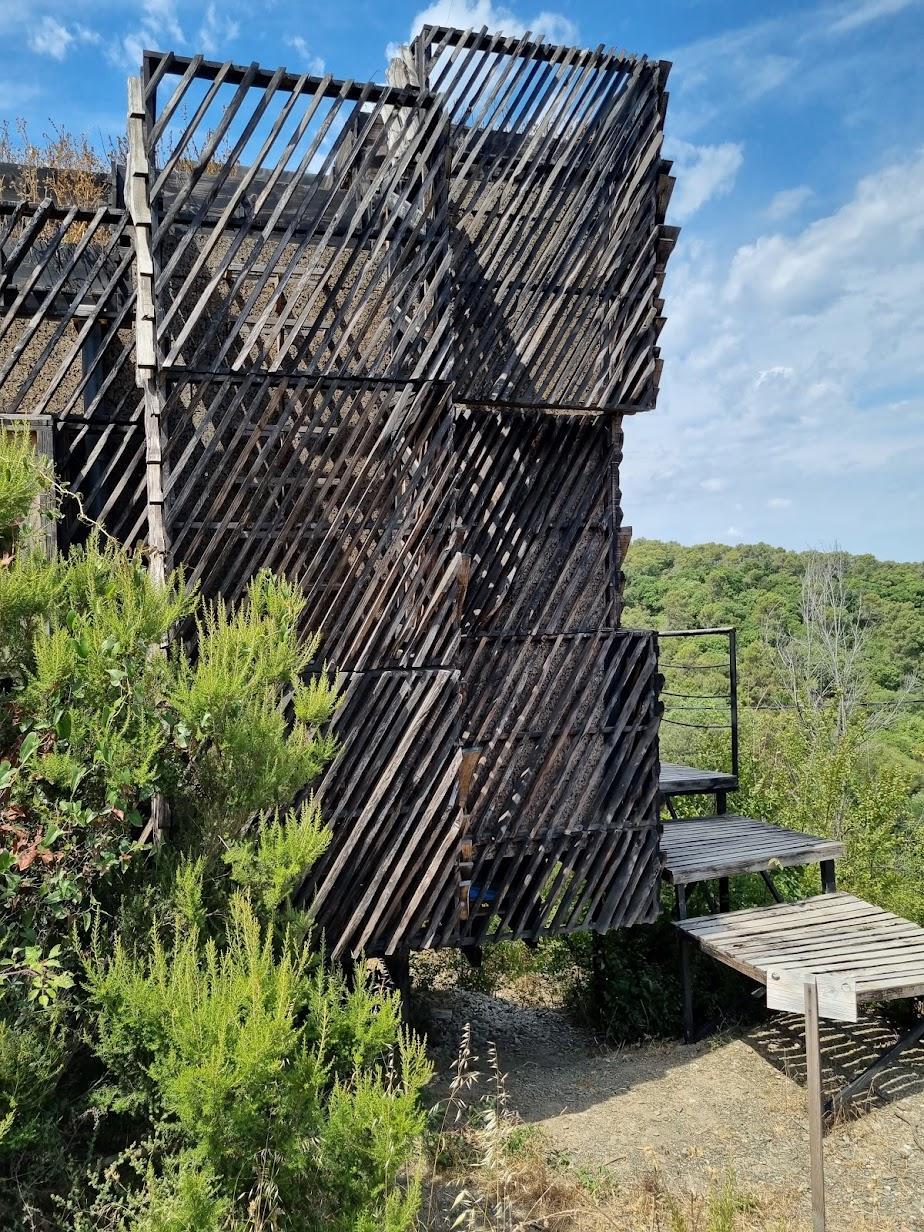
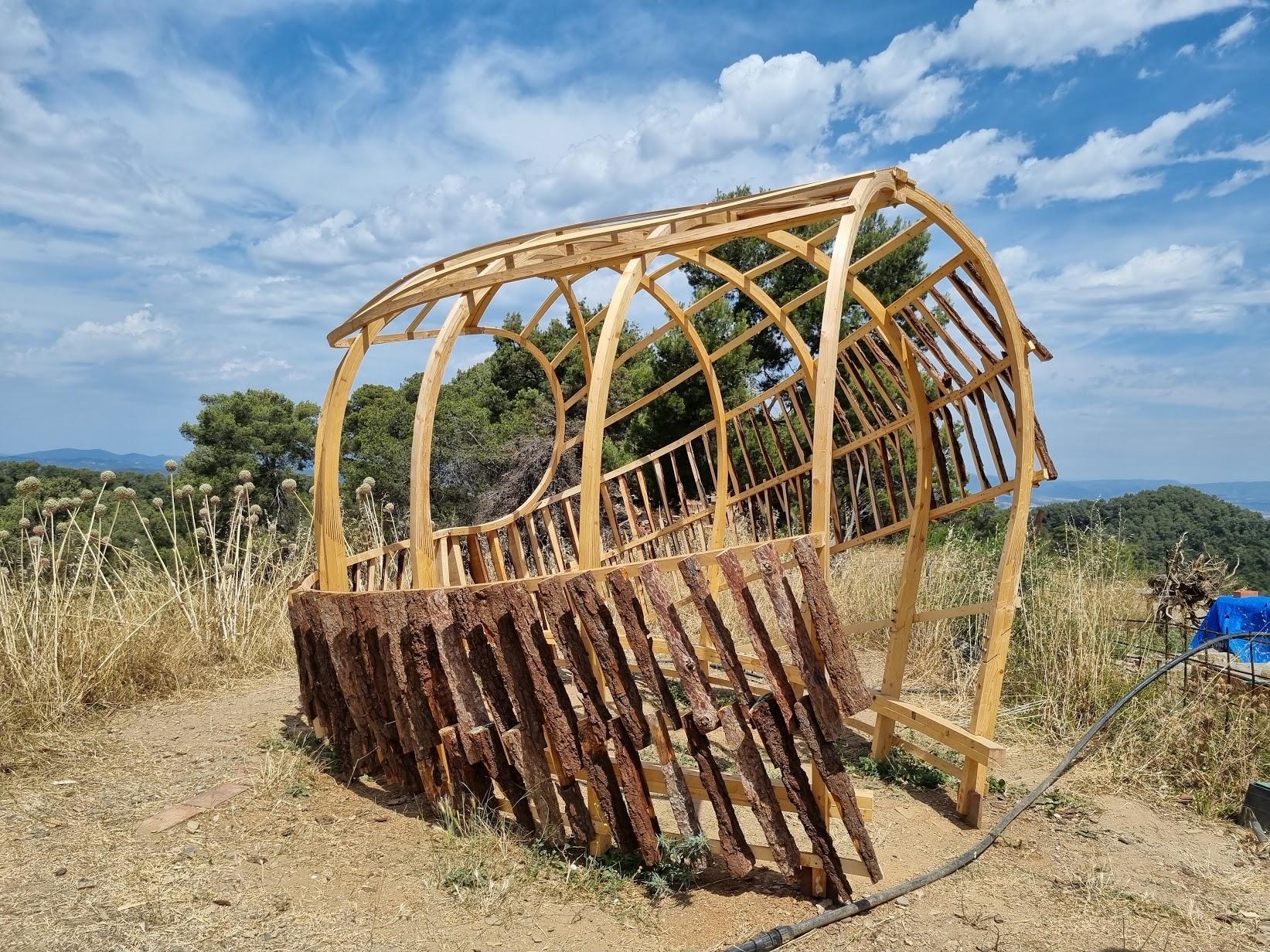
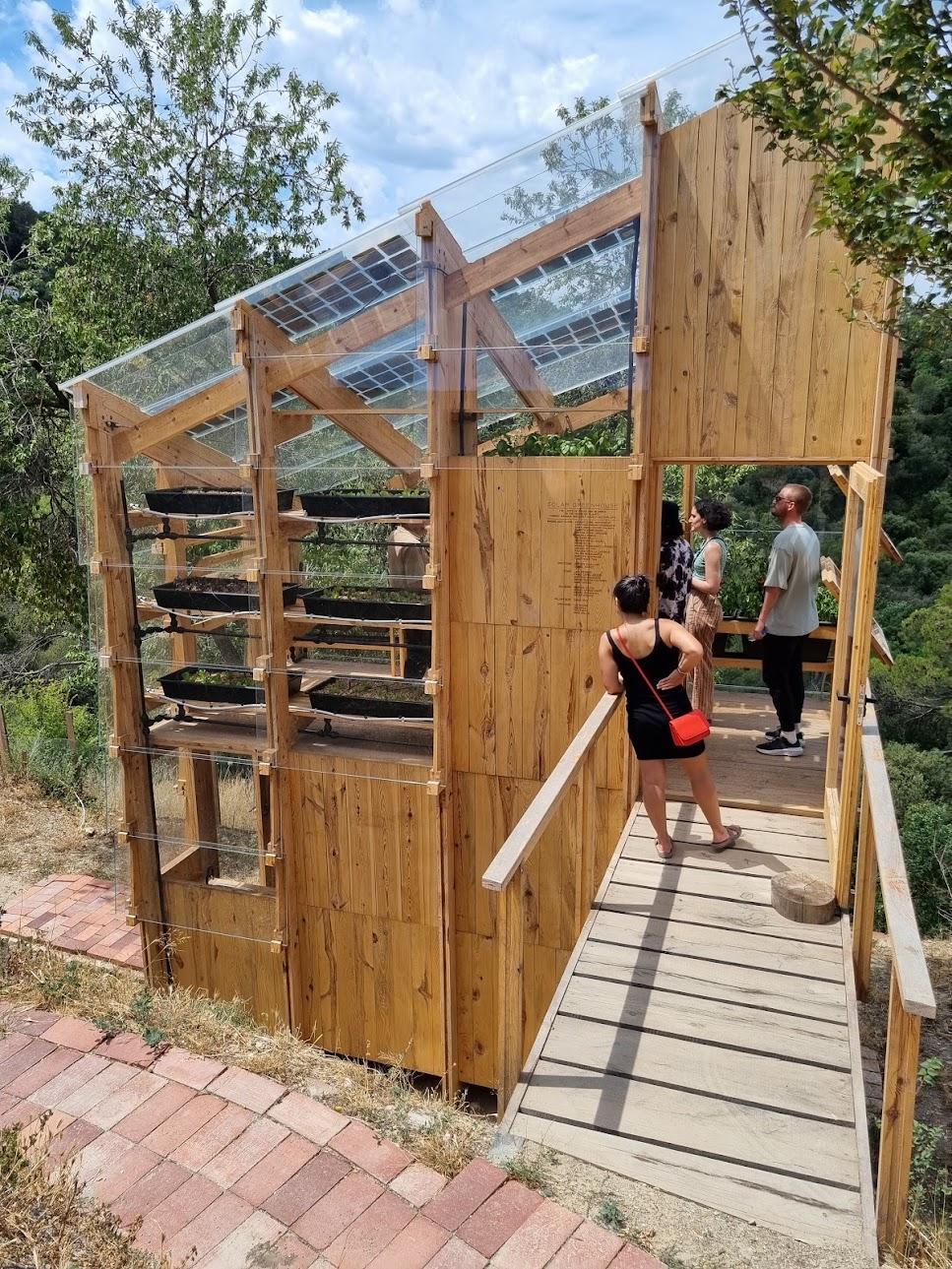
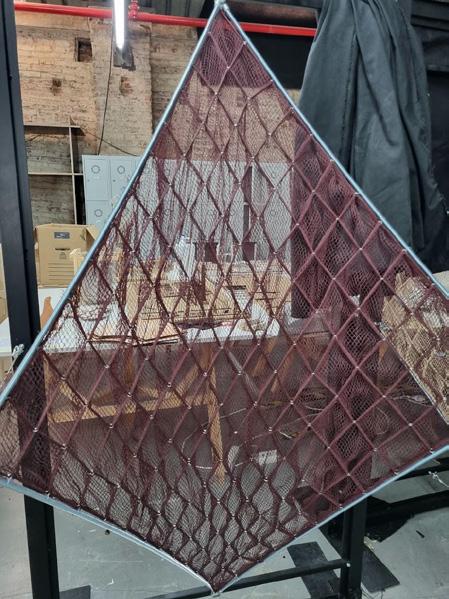
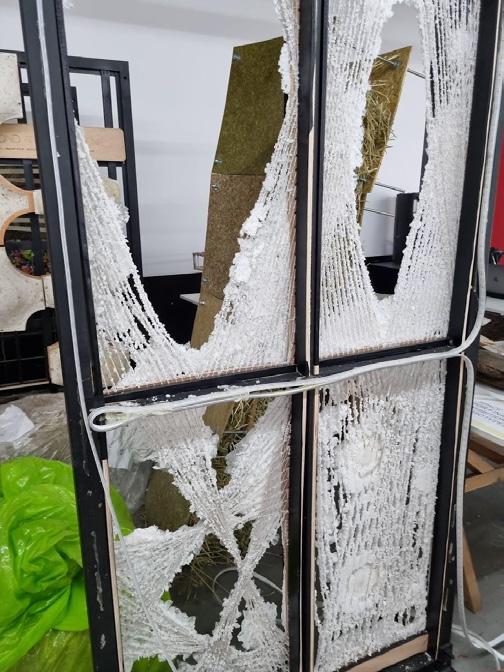
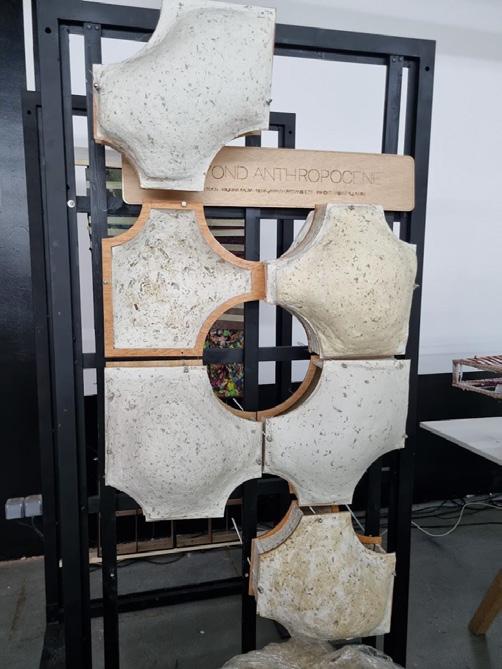
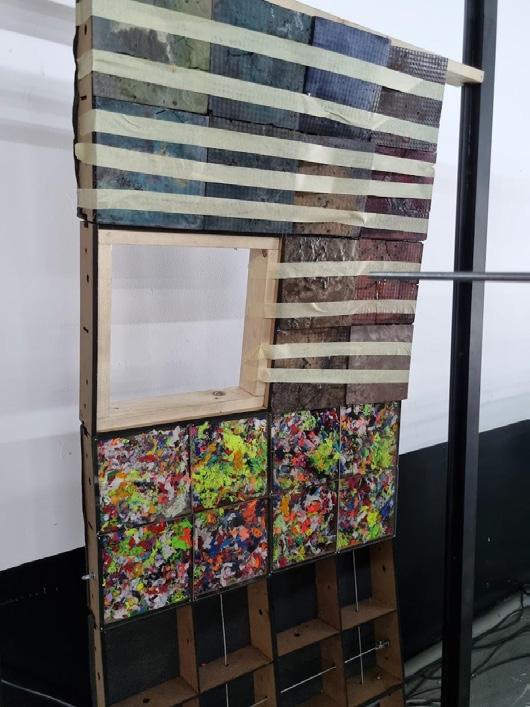
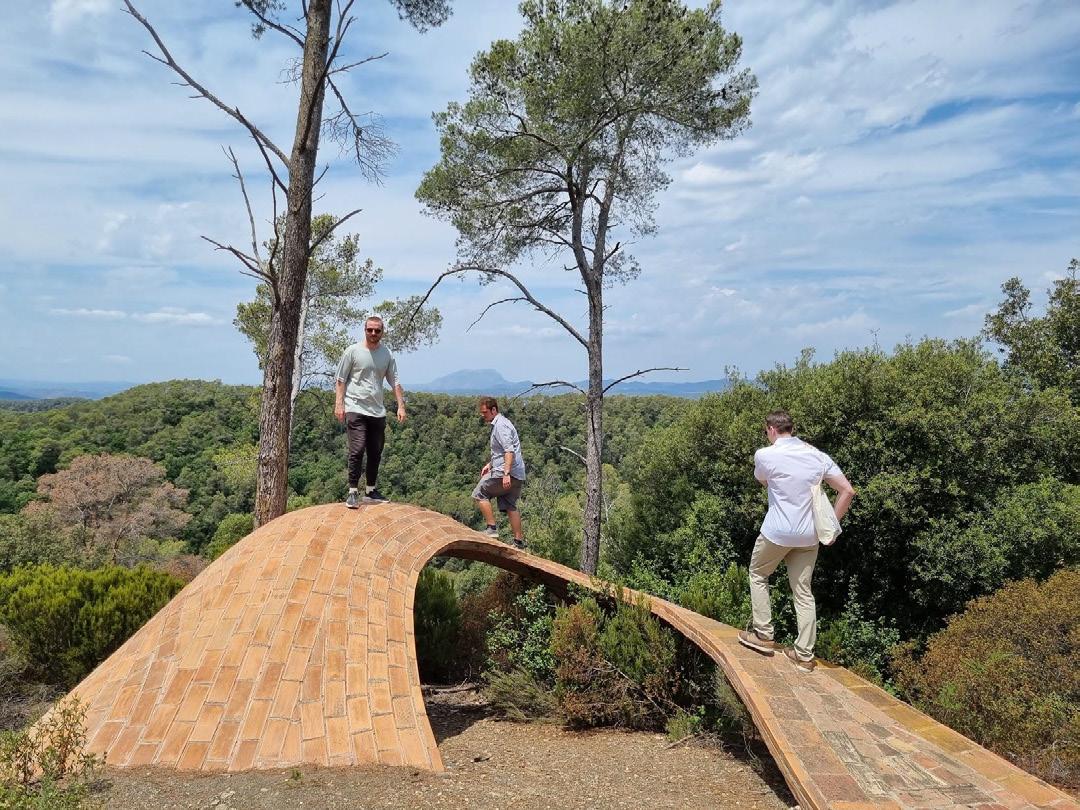
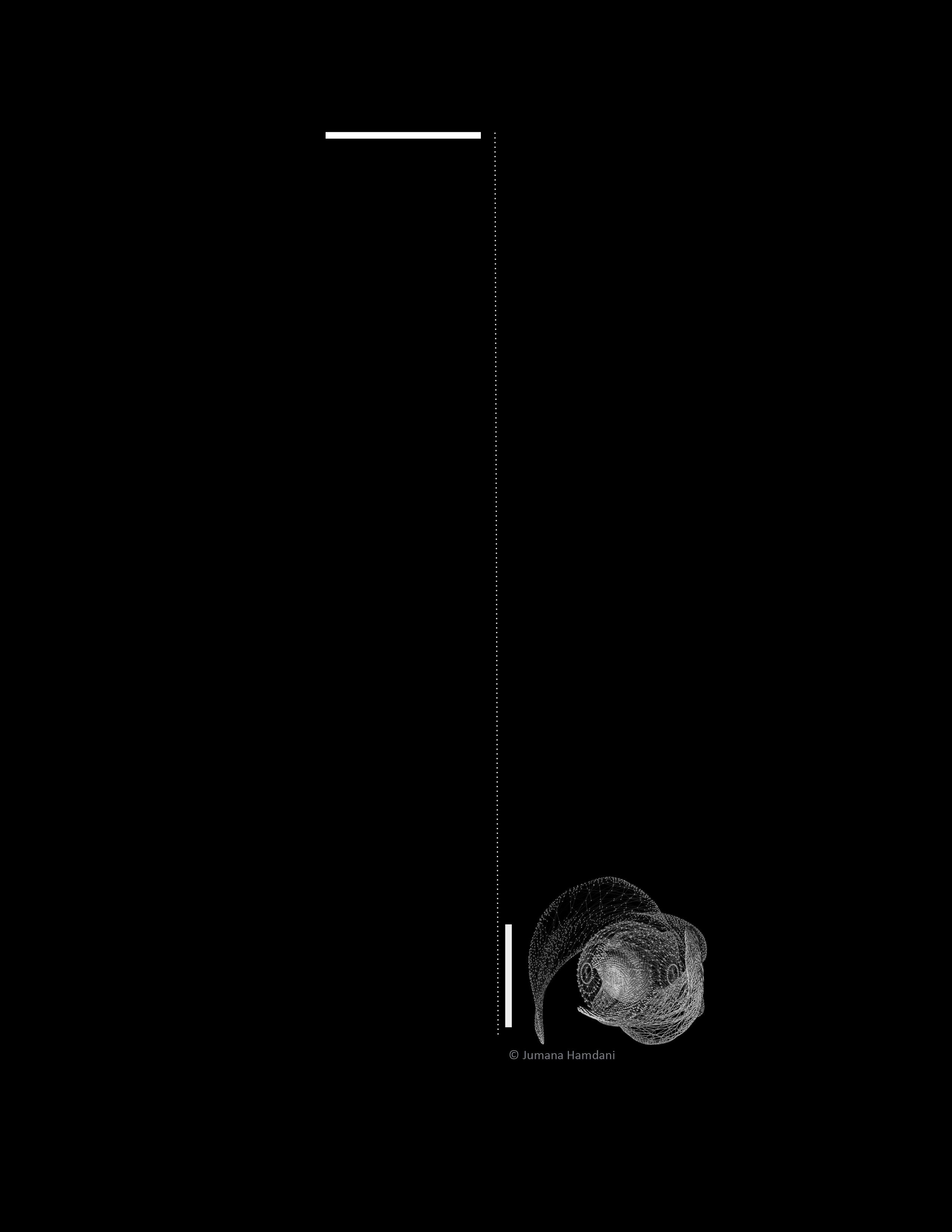