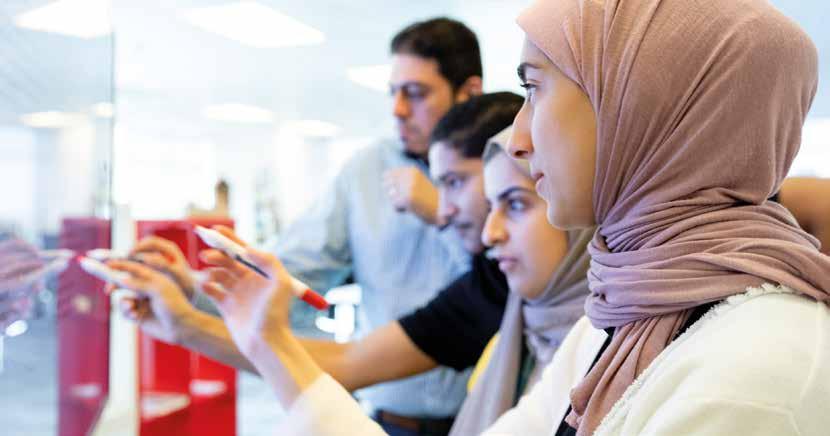
2 minute read
Protecting Data Privacy in the Age of AI
by KAUST
KAUST professor develops more secure machine-learning models
PETER RICHTARIK Professor of Computer Science
Advertisement
IT IS INCREASINGLY IMPORTANT THAT WE PROTECT THE DATA THAT THESE MACHINELEARNING MODELS REALLY THRIVE ON.
FORBES
“The Next Generation of Artificial Intelligence”, October 12, 2020
Artificial intelligence (AI) technology has grown in leaps and bounds over the past decade. It is now being used across various sectors to speed up drug development, support autonomous vehicles and help HR managers recruit more efficiently. Using AI, companies and governments can analyze large amounts of data quicker than ever before, but the risk of privacy breaches also increases with more data. Peter Richtarik, Professor of Computer Science at KAUST, is helping to develop AI models that can learn from datasets without comprising privacy.
Federated learning is one of the most promising privacy-preserving approaches to AI. It is a concept co-invented by Professor Richtarik and Google researchers that improves communication efficiency while maintaining privacy. It does so by flipping the conventional approach to AI on its head. The traditional approach to machine learning is to gather all the training data in one place, for example in a cloud server. This makes it easier to train a model on the data but also means that the data is potentially more vulnerable to privacy and security breaches.
With federated learning, the data sets are not centrally stored but rather kept separate, and the machine-learning process is completed by a loose federation of participating devices coordinated by a central server. In the event of a data security breach, the risk is isolated to just one of the devices. Federated learning is still very much in its early phases, but it has huge potential. Forbes recently named it as one of the three most-important areas in AI development, with the next 10 years being key.
Federated learning has other benefits aside from increasing user privacy. For example, AI-powered autonomous vehicles could leverage the technology to safely avoid road obstacles like potholes. Using information from the cars around it, a self-driving vehicle would be able to make better decisions on how to avoid potholes and increase passenger safety.
Professor Richtarik’s research is underpinned by the AI Initiative at KAUST, a research and education outreach program launched in 2018. Through this initiative, Professor Richtarik and other researchers have established KAUST as a regional leader in AI and machine learning, while ensuring a swath of educational opportunities for the next generation of experts coming through the university’s ranks.