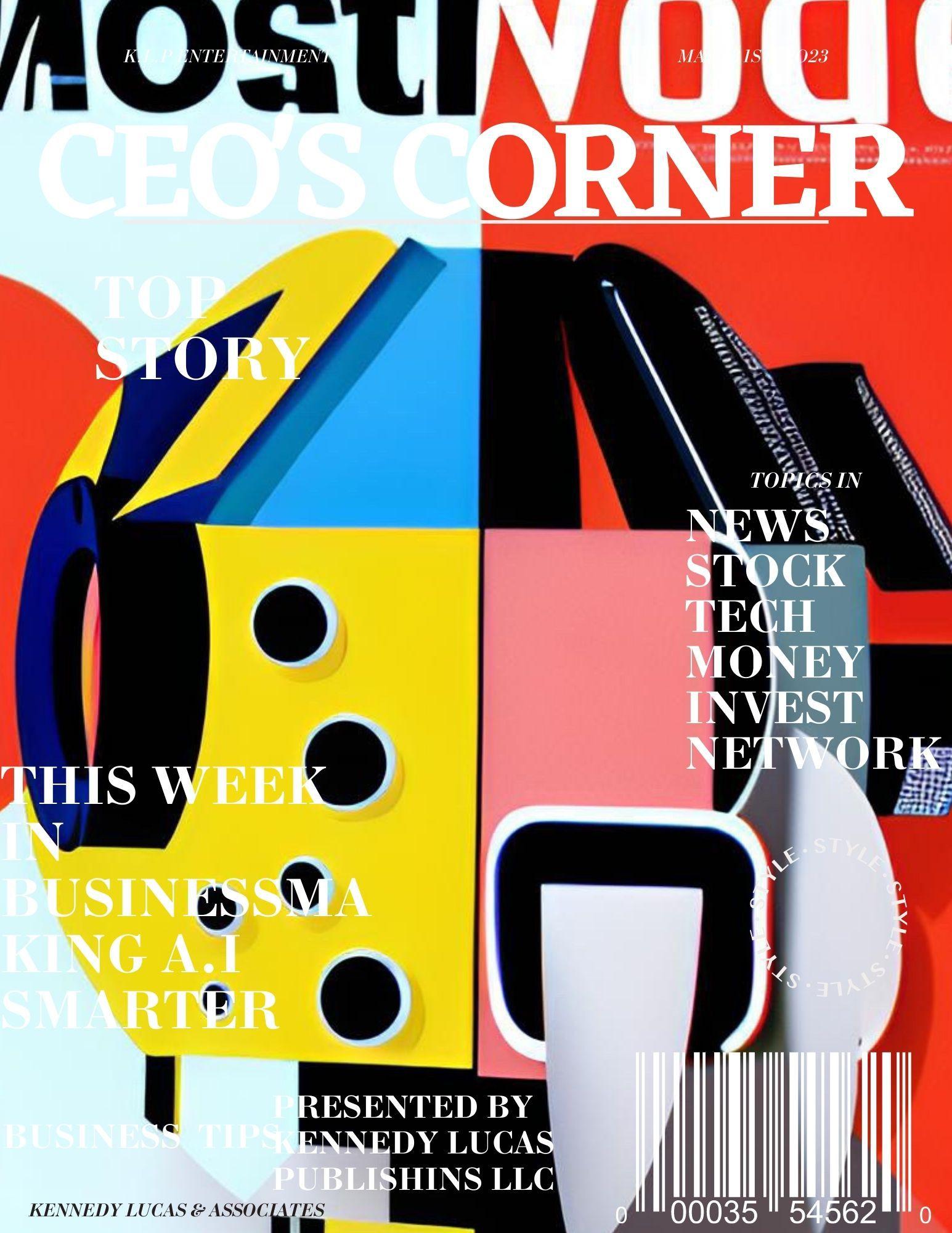
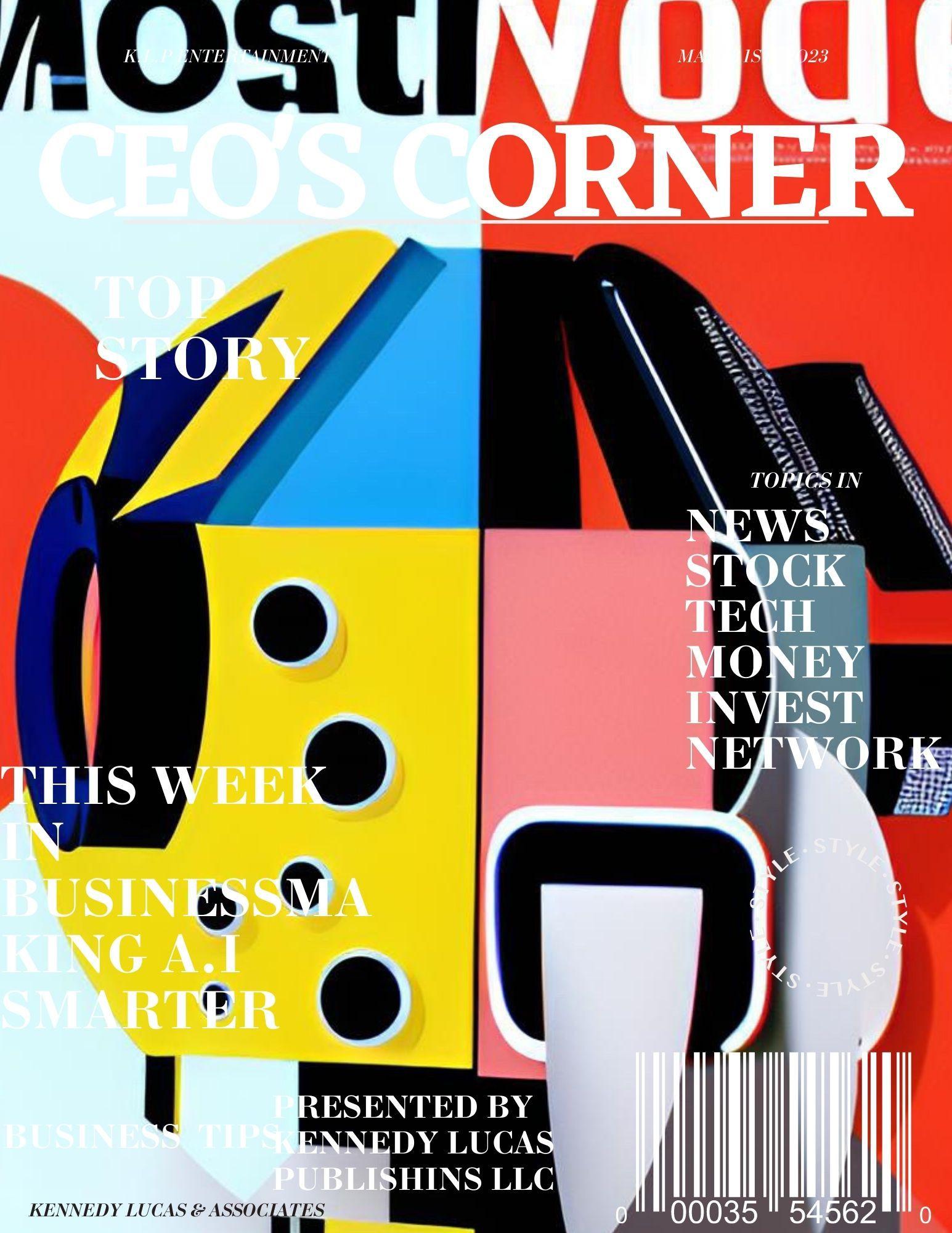
CEO’S CORNER The
Race To Make A.I Smarter
Large language models may sound more human if they are taught fewer words. Bigger is always better when it comes to chatbots with artificial intelligence.
As they are fed more data, large language models like ChatGPT and Bard, which produce conversational, original text, get better. Every day, bloggers use the internet to describe how the most recent technological developments, such as an app that summarizes articles, podcasts produced
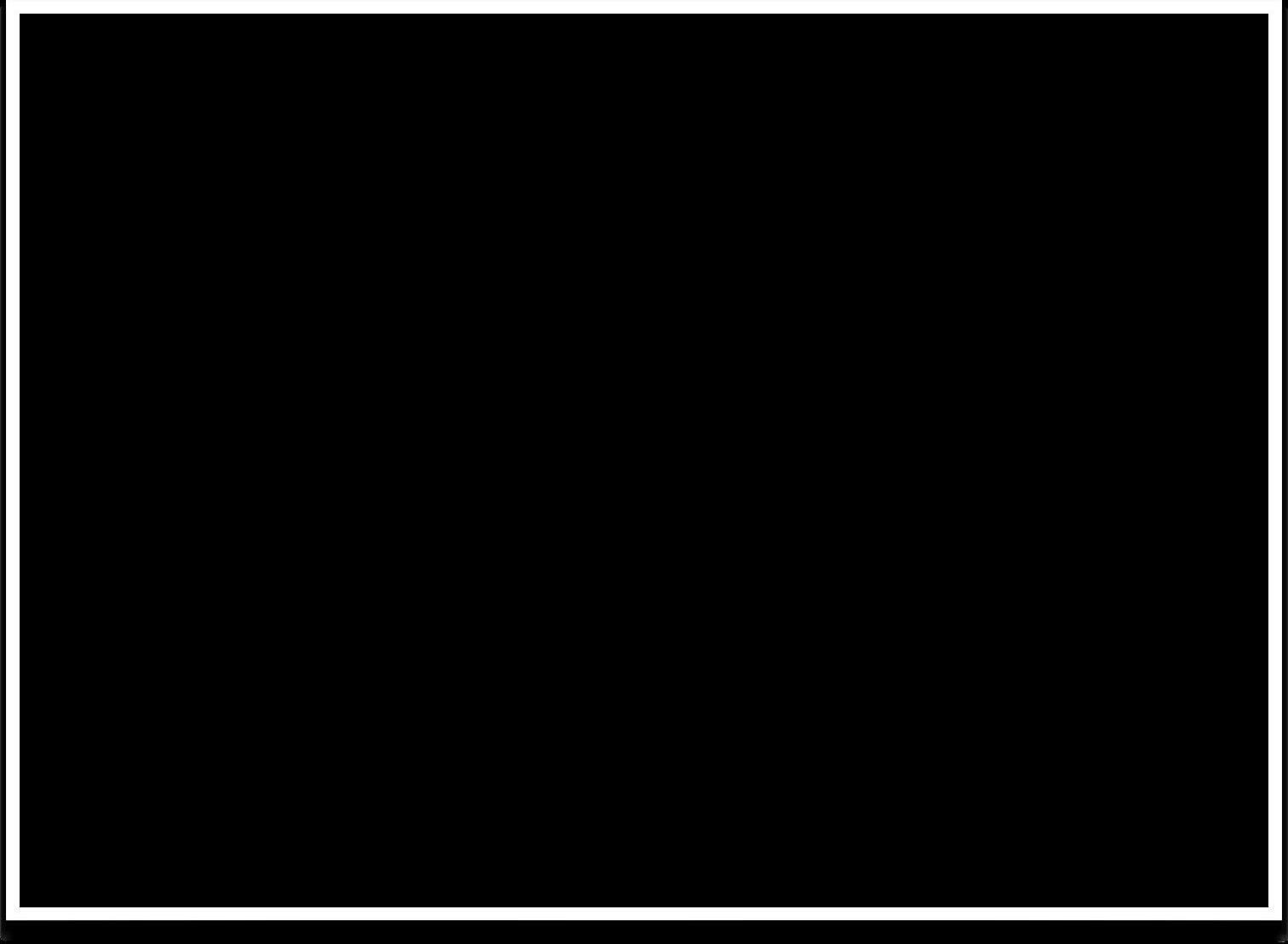
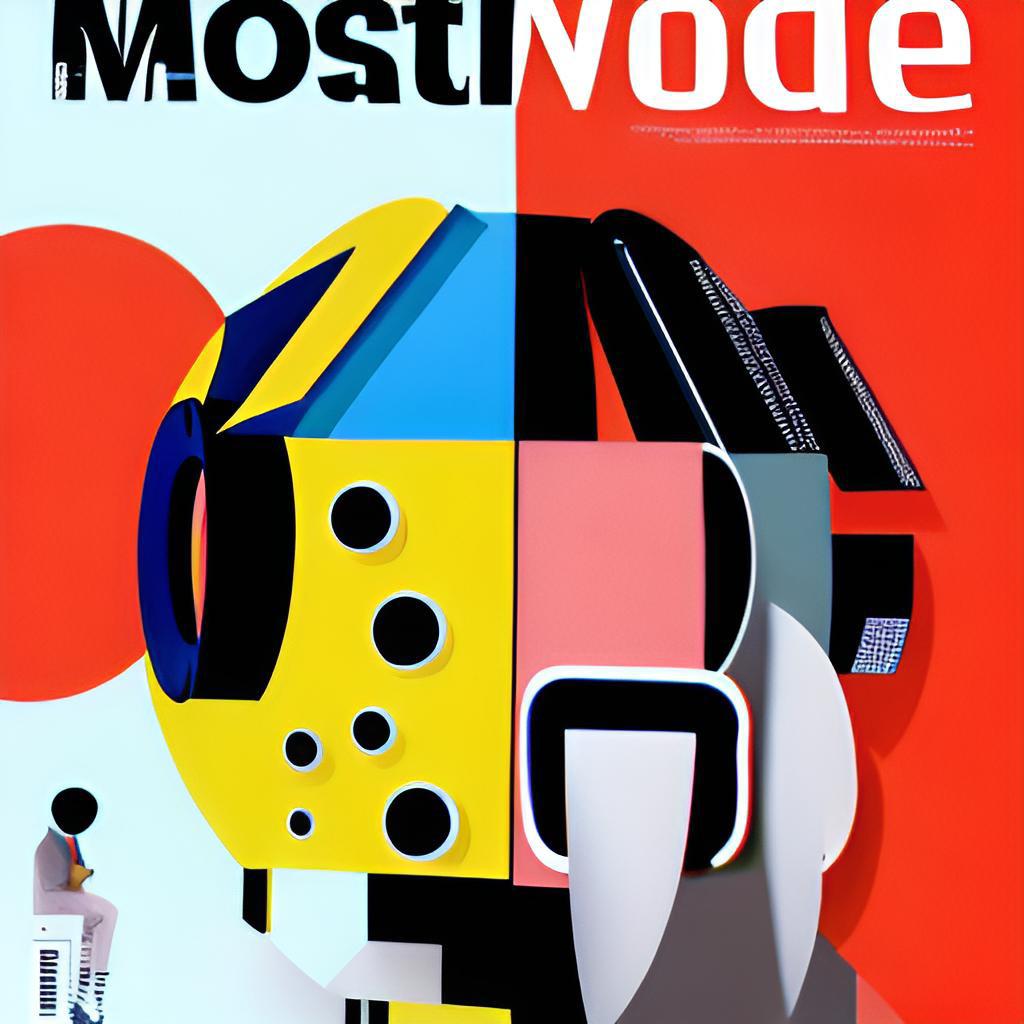
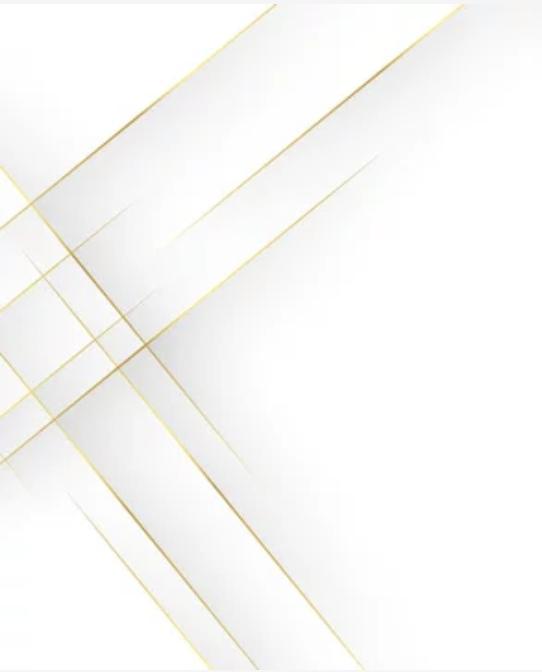
cial intelligence, and a ned model that can answer any question about professional basketball, will "change everything."
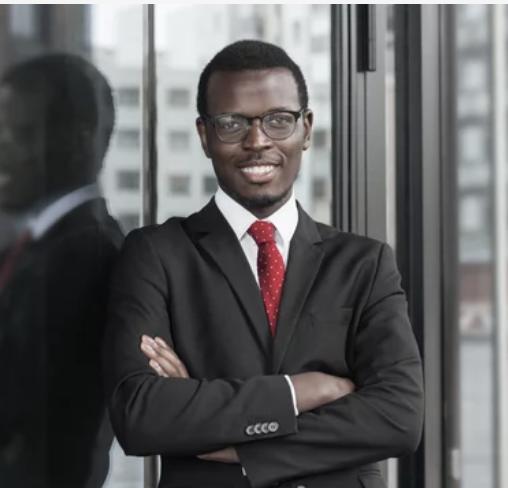
A growing number of people are worried that a small group, including Google, Meta, OpenAI, and Microsoft, will have almost complete control over the technology since developing larger and more capable A.I. demands
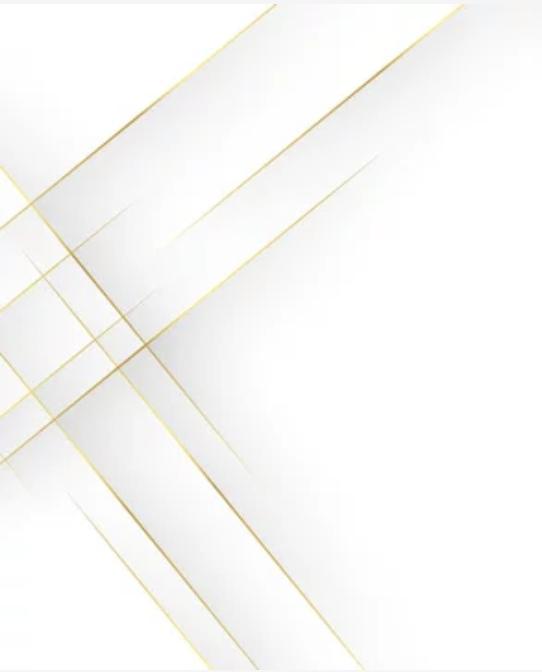
processing capacity that only a limited number of corporations have.
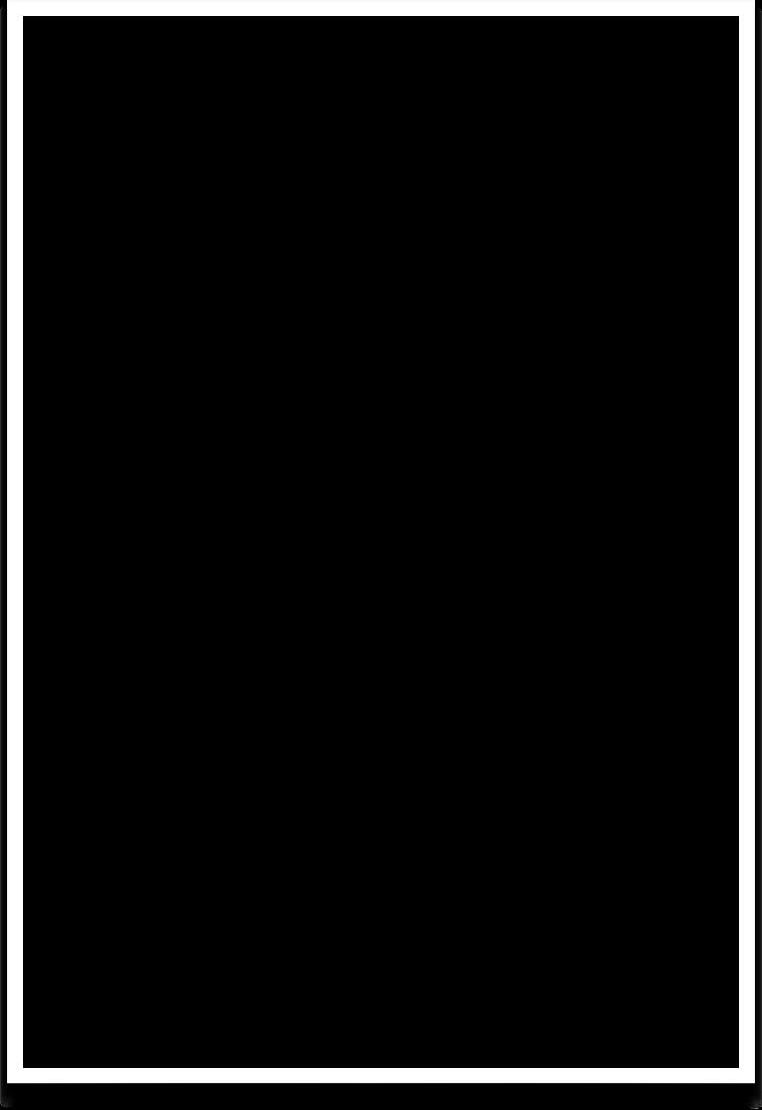
Nulla nunc lectus porttitor vitae pulvinar magna. Sed et lacus quis enim mattis nonummy sodales.
Presented By Kennedy Lucas Publishings LCLarger language models are also more difficult to comprehend. They frequently Even their designers have called them "black boxes," and top researchers have expressed concern that A.I.'s ultimate aims may not be the same as ours. If bigger is better, it also tends to be more secretive and exclusive. Natural language processing is the area of artificial intelligence that focuses on verbal comprehension. In January, a group of young academics issued a challenge to attempt and challenge this paradigm. Teams were tasked with developing functional language models from data sets that were less than a tenth the size of those
utilized by the most University and one of the BabyLM organizers. The project's other organizer and computer scientist Alex Warstadt stated, "The challenge puts questions about human language learning, rather than 'How big can we make our models?' at the center of the conversation."
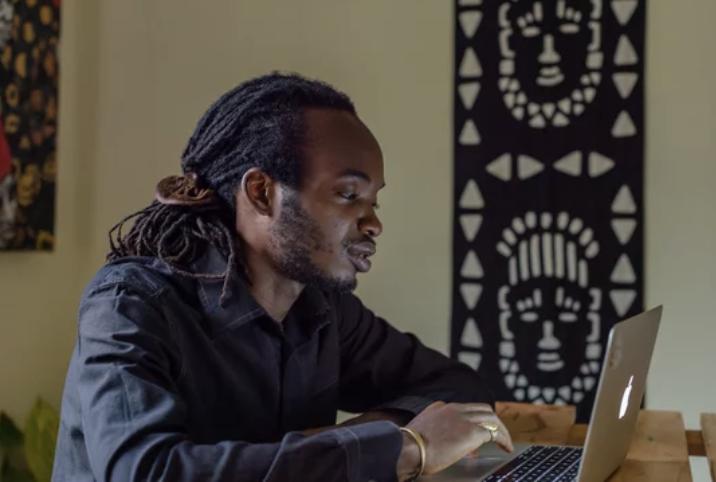
NEWS STOCK MONEY BUSINESS
Nulla nunc lectus porttitor vitae pulvinar magna. Sed et lacus quis enim mattis nonummy sodales.
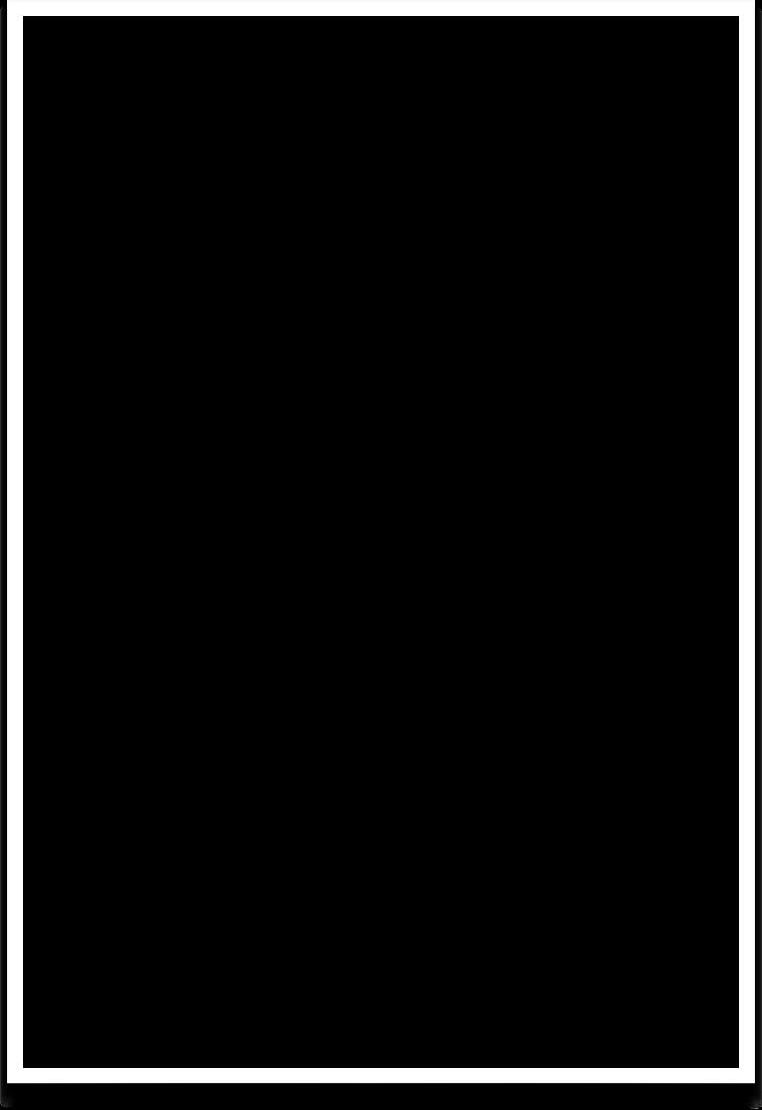
The next word in a sentence or phrase can be predicted by neural networks called large language models. Using a corpus of words generated from transcripts, websites, books, and newspapers, they are trained for this duty. On the basis of example sentences, a typical model draws assumptions.
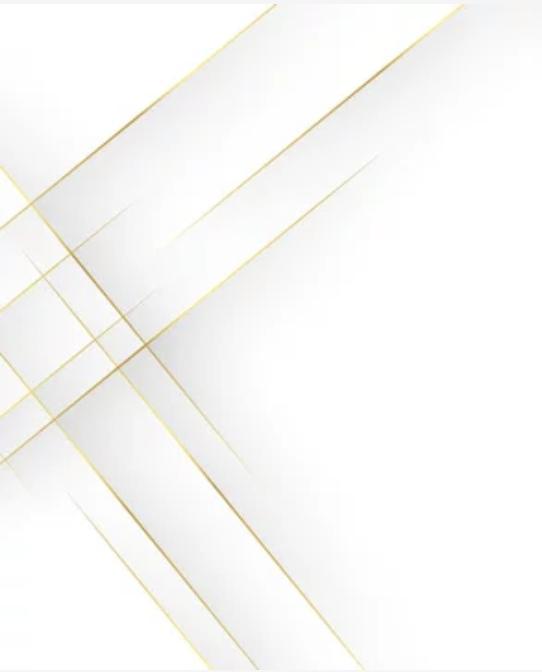
and based on how near it is to the correct response, adjusts itself. A model is created by repeatedly performing this process to create maps of the relationships between words. In general, a model will get stronger the more words it is trained on since each phrase gives the model context, and more context translates to a more detailed understanding of what each word means. 200 billion words were used to train OpenAI's GPT-3, which was published in 2020; a trillion were used to train DeepMind's Chinchilla, which was released in 2022.
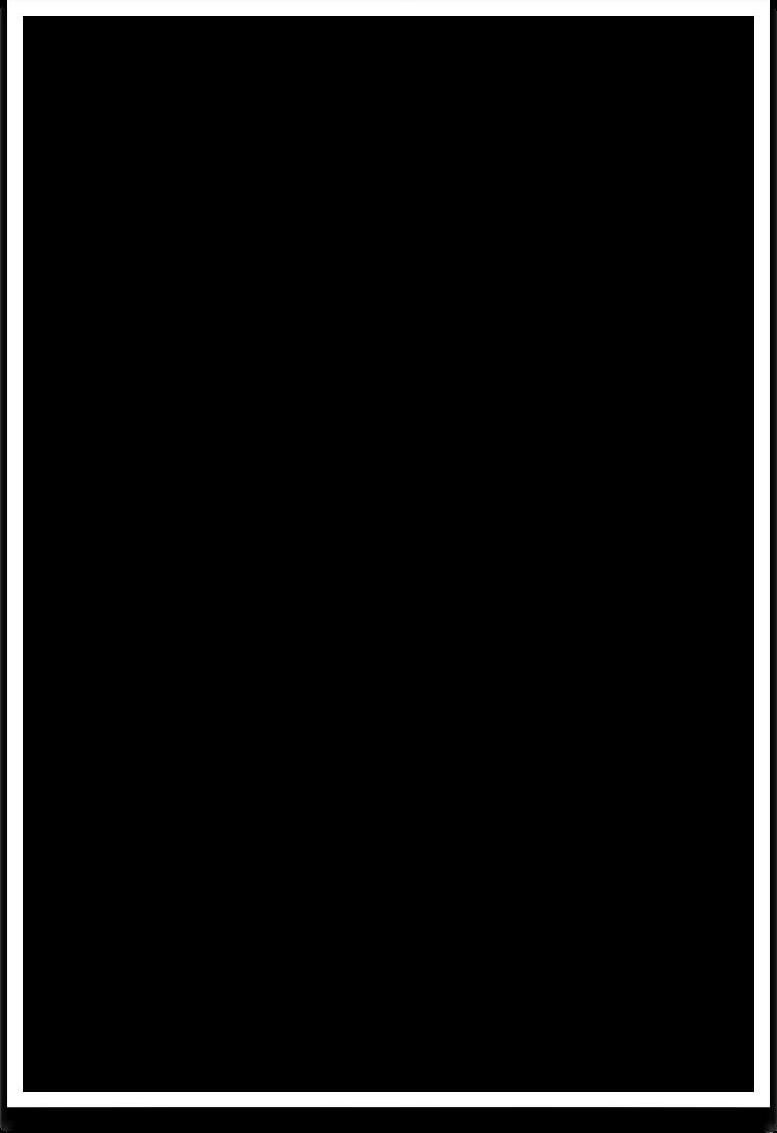
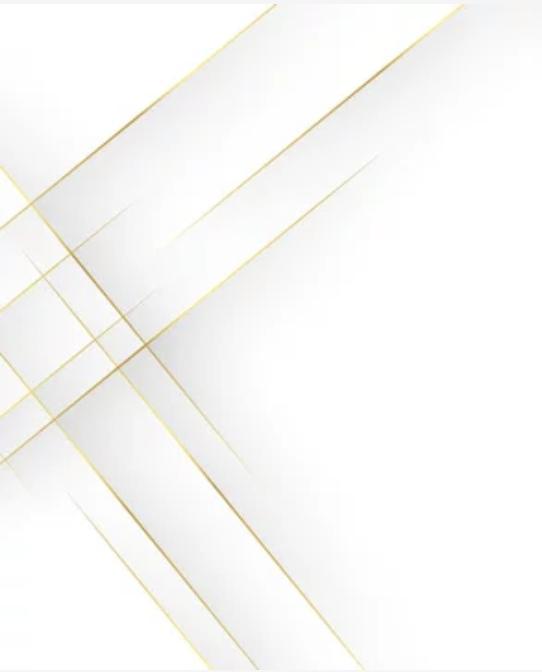
The ability of something other than humans to produce language excites linguist Ethan Wilcox, who works at ETH Zurich: Would it be possible to examine how people learn language using AI language models? As one example, nativism, a According to a well-known hypothesis that may be traced back to Noam Chomsky's early writings, humans learn languages effectively and fast because they are born with a basic understanding of how language functions. However, language models also pick up language quickly and appear to lack any innate knowledge of how language functions, suggesting that nativism may not be true.
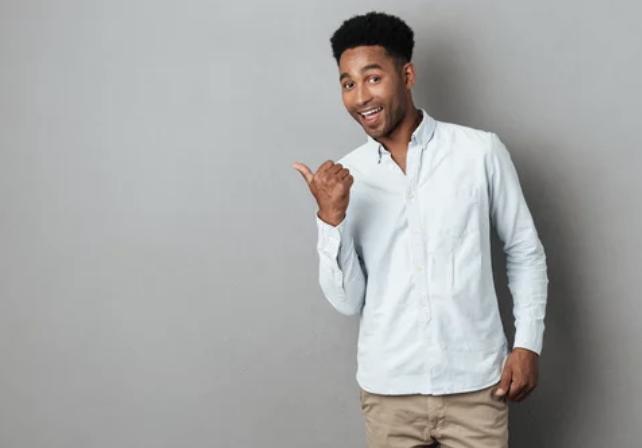
“Write quickly and you will never
The problem is that language models pick up knowledge very erently from people do. Humans have complex sensations, social lives, and physical bodies. We can taste peppermints, feel the vanes of feathers, and smell mulch. Early on, simple spoken phrases and syntaxes that are frequently absent from written language are introduced to us. Dr. Wilcox came to the conclusion that a computer taught on zillions of written words can only produce language to a certain extent. regarding the way we use language.
Nulla nunc lectus porttitor vitae pulvinar magna. Sed et lacus quis enim mattis nonummy sodales.
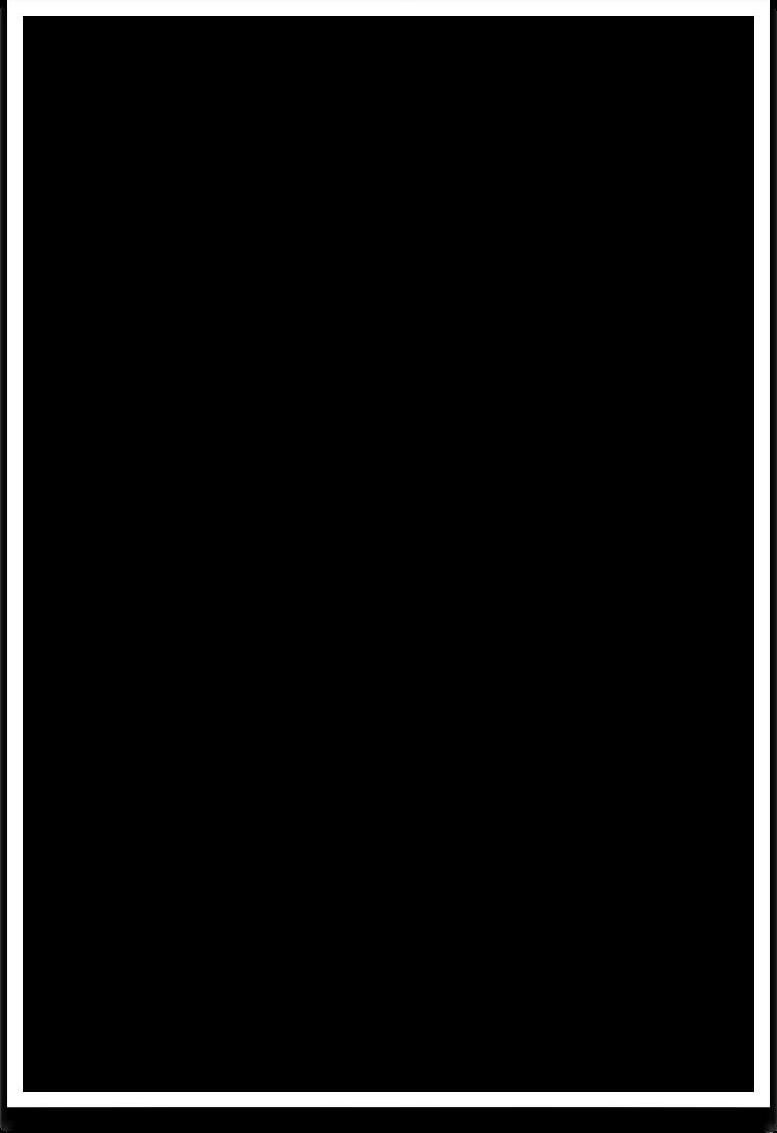
However, a language model might interact with language in ways that could answer some of the questions we have about our own capacities if it were only exposed to words that a young human encounters.
In an effort to bring language models a little bit closer to human understanding, Drs. Wilcox, Mueller, and Warstadt created the BabyLM Challenge with the help of six other researchers. To train language models on the roughly 100 million words that a 13-year-old human might encounter, they issued a call for
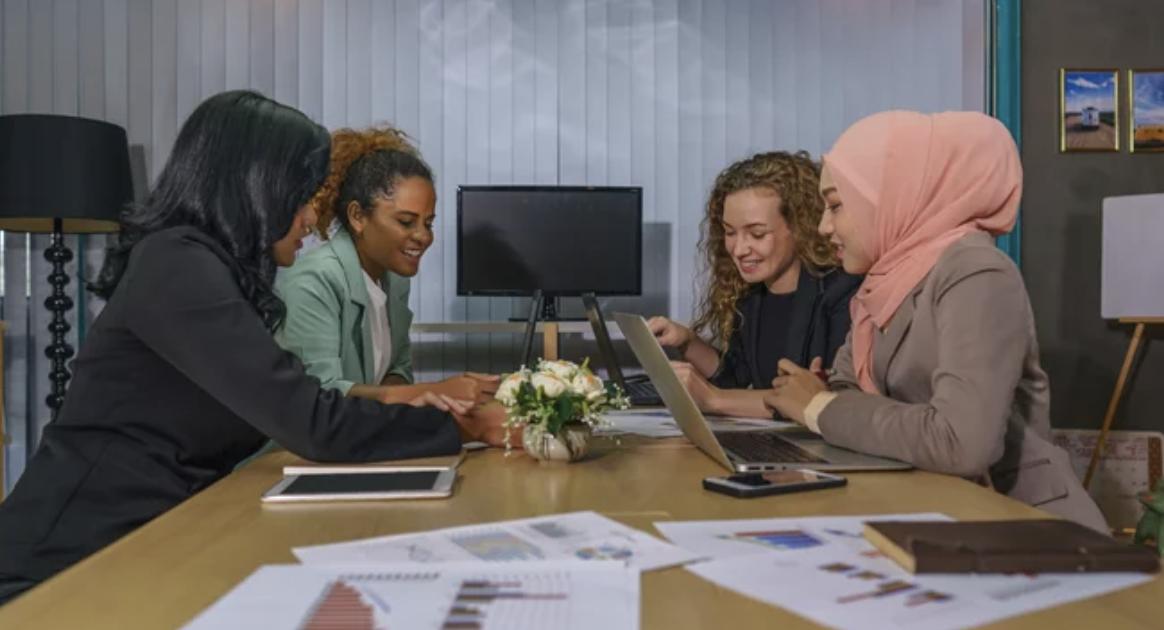
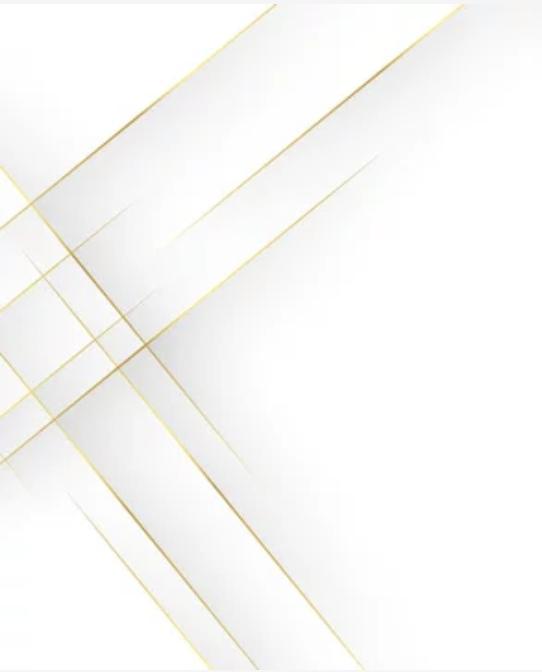
“Write quickly and you will never
teams in January. Candidates would be evaluated on how well they produced and understood linguistic nuance, and a winner would be named.
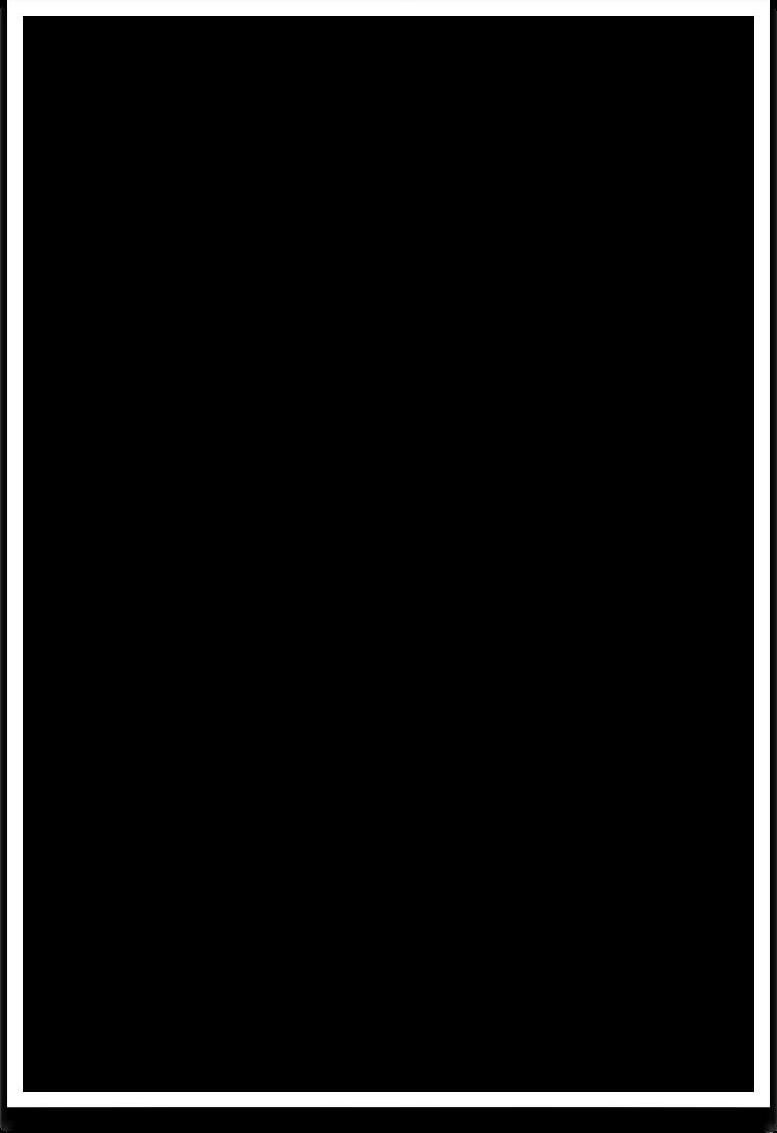
A linguist from McGill University named Eva Portelance arrived the day the challenge was made public. Her work crosses the frequently hazy boundary between linguistics and computer science. The ambition to simulate human cognitive abilities in computers led to the earliest attempts at arti intelligence (A.I.) in the 1950s; the "neuron" is the basic unit of information processing in A.I., and early language models developed in the 1980s and 1990s were directly influenced by the human brain. However, as processing power increased and businesses focused on creating goods that could be sold, computer scientists discovered that it was frequently simpler to train language models on vast volumes of data than to push them into psychologically informed structures. Dr. Portelance explained that as a result, "they provide us stuff that seems like it was written by a human, but there's no relationship between us and how they are effective.
These massive models provide little information for scientists who are interested in learning how the human mind functions. Furthermore, few academics have access to them because they demand a lot of computing power. Only a few large-scale industry labs can afford
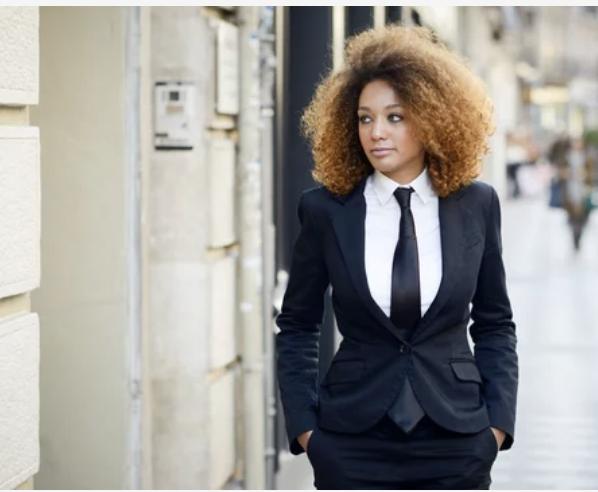
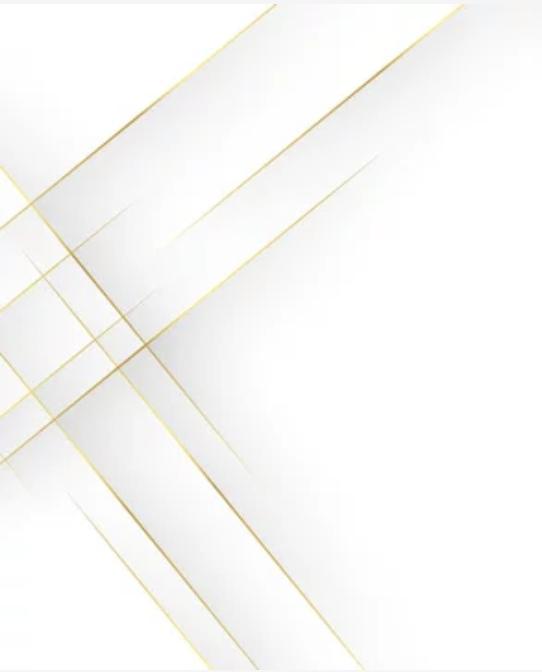
“Write quickly and you will never
to train models with trillions of words and billions of parameters, according to Dr. Wilcox.
"Or even to load them," Dr. Mueller continued. Research in the subject has recently felt a little less democratic as a result of this.
According to Dr. Portelance, the BabyLM Challenge might be considered as a step away from the competition for larger language models and toward more approachable, intuitive A.I.
Larger industry labs have not overlooked the possibility of such a research program.
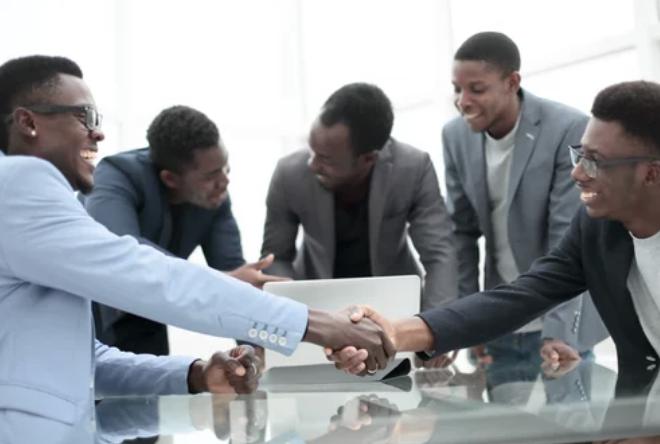
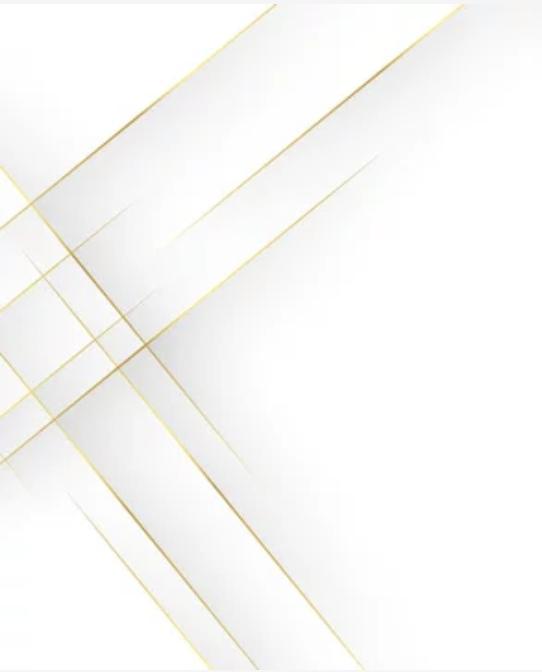
Chief Sam Altman A recent statement by an OpenAI executive claimed that enlarging language models would not produce the same kind of advancements as in previous years. Additionally, businesses like Google and Meta have been funding research towards language models that are more effective and are based on human brain architecture. After all, it is possible to scale up a model that can produce language when trained on less input.
Nulla nunc lectus porttitor vitae pulvinar magna. Sed et lacus quis enim mattis nonummy sodales.
Despite any potential financial gain, the challenge's backers' objectives are primarily scholarly and abstract. Even the prize undermines the useful.
"Just pride," Dr. Wilcox replied.
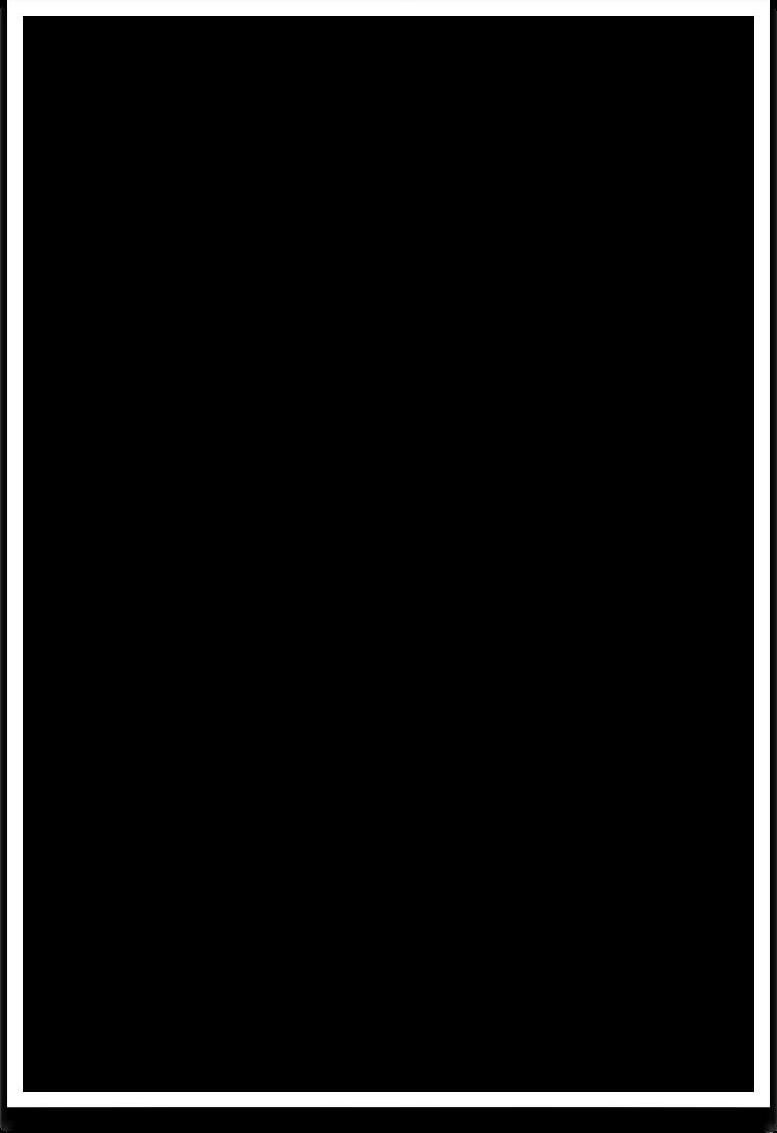
“Write quickly and you will never
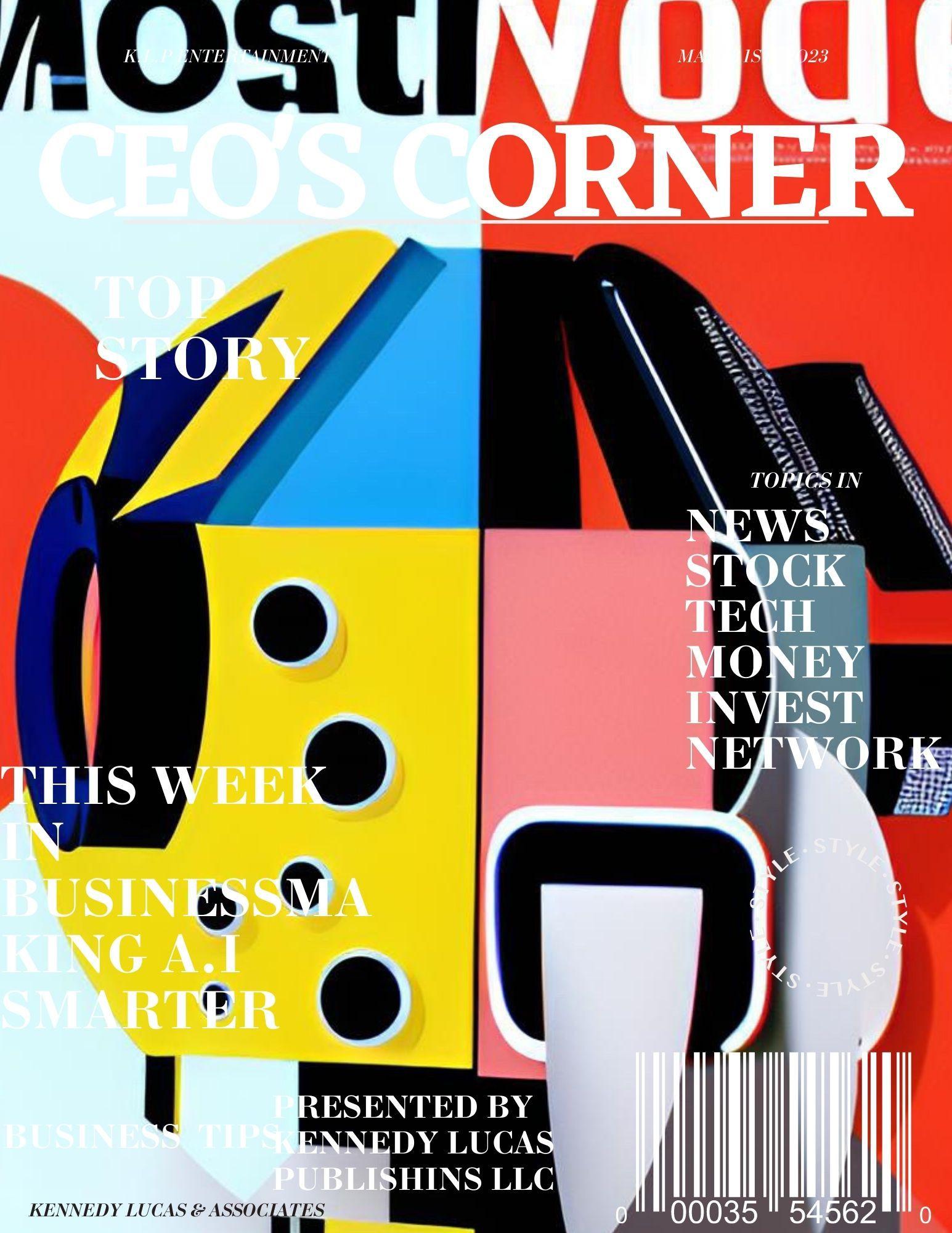