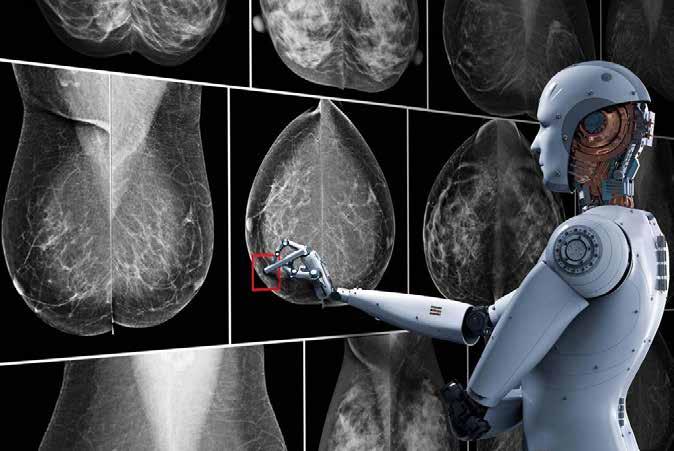
6 minute read
High hopes for AI in cancer detection
Artificial Intelligence can help detect one of the most common forms of blood cancer - acute myeloid leukaemia (AML) - with high reliability. AML is a life-threatening disease that should be treated as quickly as possible.
Cancer is the deadliest of all diseases and the second leading cause of death globally, accounting for an estimated 9.6 million deaths in 2018.
Advertisement
Early detection of cancer boosts survival chances of patients tremendously but, unfortunately, most victims are diagnosed in the final stages of the disease, leaving them with little hope for recovery. By then, the tumor would have spread excessively, leaving no treatment option either for stopping the metastasis or complete removal of the affected cells.
Of late, experts are exploring the scope of Artificial Intelligence (AI) and deep learning in addressing the issue. Both Artificial Intelligence and machine learning have long been there, hugely affecting our everyday lives by bringingmajor changes in communication, transportation, and media. Researchers are now showing a keen interest in using advances in the field of AI to improve diagnosis, management and better therapeutics to treat chronic diseases, especially cancer.
The application of Artificial Intelligence for betterment of healthcare is booming with each passing day. With advances in algorithms and hard-
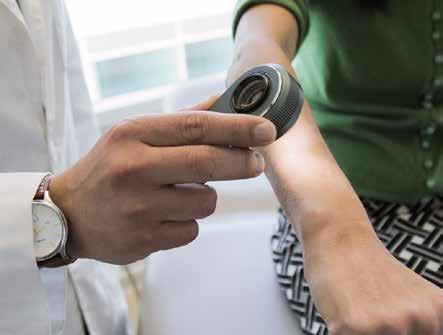
According to the researchers, at present ultrasounds can tell if a nodule looks suspicious, and then the decision is made whether to do a needle biopsy, but fine-needle biopsies only act as a peephole, they don't reveal the whole picture. As a result, some biopsies return inconclusive results as to whether the nodule is malignant, or cancerous in other words.
ware, machine learning and Artificial Intelligence can learn and analyze an enormous amount of data.
A vast data regarding cancer-diagnosed patients and those who availed treatment is being collected over the years. Experts vouch that early diagnosis of cancer is possible through Artificial Intelligence if the huge database is properly utilised.
Researchers at the University of Texas Southwestern Medical Centre in Dallas have developed a software tool that uses Artificial Intelligence to recognize cancer cells from digital pathology images - giving clinicians a powerful tool to predict patient outcomes. The spatial distribution of different types of cells can reveal cancer’s growth pattern, its relationship with surrounding micro-environment and the body's immune response.
A major technical challenge in systematically studying tumor micro-environment is to automatically classify different types of cells and quantify their spatial distributions, says Dr. Guanghua 'Andy' Xiao, corresponding author of a study published in EbioMedicine. The AI algorithm that Dr Xiao and his team developed, called 'ConvPath', overcomes these obstacles by using AI to classify cell types from lung cancer pathology images.
The ConvPath algorithm can 'look' at cells and identify their types based on their appearance in the pathology images using an AI algorithm that learns from human pathologists. The algorithm helps pathologists in making accurate cancer cell analysis in quick time.
"It is time-consuming and difficult for pathologists to locate very small tumour regions in tissue images, so this could greatly reduce the time that pathologists need to spend on each image," says Dr Xiao.
A study, published in the journal PLOS Pathogens, suggests that automated machine learning shows promise as an additional diagnostic tool that could improve efficiency of thyroid cancer diagnosis.
"Machine learning is a low-cost and efficient tool that could help physicians arrive at a quicker decision on how to approach an indeterminate nodule," said the study's lead author John Eisenbrey from Thomas Jefferson University in the US. In order to improve the predictive power of the first-line diagnostic, the ultrasound, researchers looked into machine learning or AI models developed by Google. They applied a machine learning algorithm to ultrasound images of patients' thyroid nodules to see if it could pick out distinguishing patterns.
The researchers found that their algorithm performed with 97 per cent specificity and 90 per cent predictive positive value, meaning that 97 per cent of patients who truly have benign nodules will have their ultrasound read as 'benign' by the algorithm, and 90 per cent of malignant or 'positive' nodules are truly positive as classified by the algorithm. The overall accuracy of the algorithm was 77.4 per cent.
Thyroid nodules are small lumps that form within the thyroid gland and are quite common in the general population, with a prevalence as high as 67%. The great majority of thyroid nodules are not cancerous and cause no symptoms.
Though preliminary, the study suggests that automated machine learning shows promise as an additional diagnostic tool that could improve efficiency of thyroid cancer diagnoses. Once it becomes more robust, the approach could give doctors and patients more information in order to decide if thyroid lobe removal is necessary.
A team of researchers from Beth Israel Deaconess Medical Center of Harvard Medical School led by Dr.
Machine-learning algorithm was also tested for diagnosis of Melanoma, a type of cancer that mostly occur in the skin, by the researchers of Stanford University. Apart from checking the diagnostic skills of the tool, the researchers also compared these results with certified dermatologists. The study showed that Artificial Intelligence can classify skin cancer as accurately as the dermatologists.
Similarly, researchers tested the commercially available tool for the detection of colorectal cancer, ColonFlag (in Europe) or LGI Flag (in the US), and found that the machine-learning algorithm identified individuals with tenfold higher risk of undiagnosed colorectal cancer at curable stages (0/I/II).
Recently, researchers from the Viterbi School of Engineering at the University of Southern California trained a machine-learning algorithm to differentiate between benign and malignant tumors in breast cancer. They used synthetic data instead of real data to train the algorithm. The results were fascinating, as the lead researcher Prof. Assad Oberai said, "We had about an 80% accuracy rate. Next, we continue to refine the algorithm by using more real-world images as inputs."
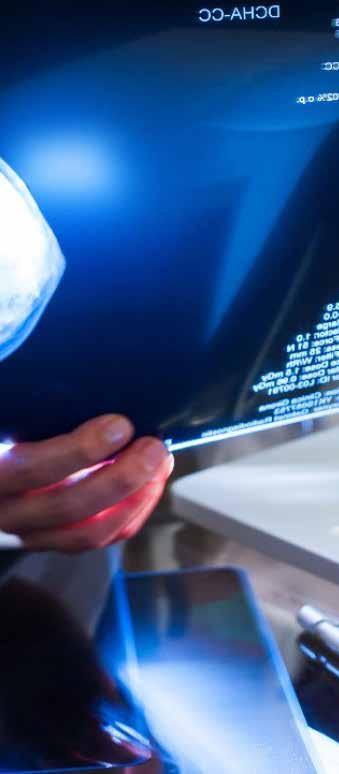
Artificial Intelligence can help detect one of the most common forms of blood cancer - acute myeloid leukaemia (AML) - with high reliability, new research has found. The approach, based on the analysis of the gene activity of cells found in the blood, could support conventional diagnostics and possibly accelerate the beginning of therapy, said the study published in the journal iScience. In the early stages, the symptoms of AML can resemble those of a bad cold. However, AML is a life-threatening disease that should be treated as quickly as possible.
"With a blood test, as it seems possible on the basis of our study, it is conceivable that the family doctor would already clarify a suspicion of AML," said Joachim Schultze, a research group leader at the German Center for Neurodegenerative Diseases (DZNE).
"And when the suspicion is confirmed, the patient is referred to a specialist. Possibly, the diagnosis would then happen earlier than it does now and therapy could start earlier," added Schultze, who is also Head of the Department for Genomics and Immunoregulation at the LIMES Institute of the University of Bonn in Germany.
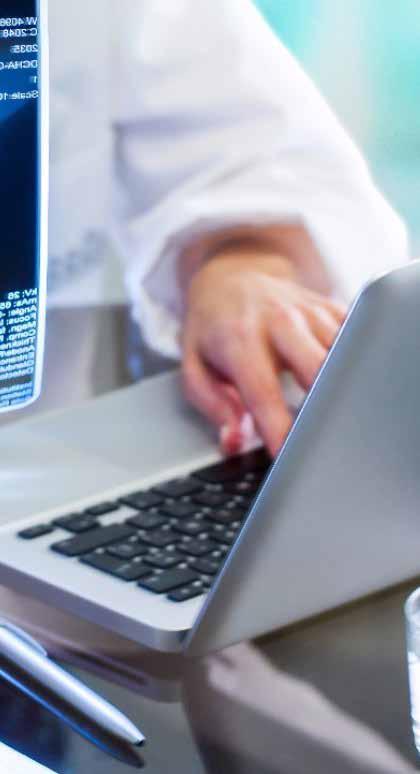