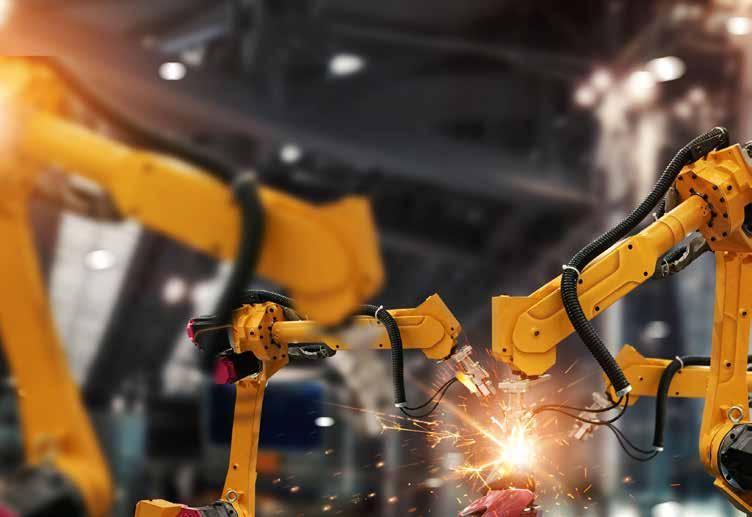
8 minute read
WHAT ARE THE EASY AI
WHAT ARE THE EASY AI WINS FOR MANUFACTURING?
Colin Elkins, VP, Manufacturing Industries, IFS discusses why AI is about to drive more value in more parts of a manufacturing enterprise than is generally thought
Advertisement
Manufacturing has been an early adopter of artificial intelligence (AI) at least in some areas like predictive maintenance. Reliability and quality are both essential for delivering a successful customer experience, so an intelligent approach to enterprise asset management (EAM) have been a natural fit particularly for repetitive manufacturing and regulated sectors.
In a 2019 IFS study, 90 percent of respondents had some plans to implement AI in various parts of their business. Industrial automation was the most commonly reported area of investment with 44.6 percent planning AI projects, while customer relationship management (CRM) and inventory planning and logistics tied for second place at 38.9 percent. According to a Deloitte study, 93 percent of manufacturers believe AI will be a pivotal technology to drive growth and innovation.
It is obvious that well beyond maintenance and factory automation, AI is about to drive more value in more parts of a manufacturing enterprise than many industry executives suspect. Just as automation revolutionized the plant floor, AI will bring dramatic changes for middle management and administrative roles.
In our work at IFS, we see that this technology will need to be at first assistive … imagine the way a smart tax form anticipates your entries. This predictive approach becomes more powerful when it is combined with the business rules underlying an enterprise resource planning (ERP) application. In time, where use cases warrant and where trust has been built up, processes can be automated entirely.
INTELLIGENT PROCESSES Intelligence must not just be bolted onto the outside of manufacturing ERP. It must be woven into the fabric of how an organization operates and creates value.
A cognitive service in that ERP application can, as you release an order to manufacturing recognize the product as a reciprocating compressor. It knows what the finished goods deliverable should probably look like based on the contract, order history, the NAICS code of the customer, their geological location which can affect the regulatory environment and taxation and other factors. The application can then route a manager through administrative steps with mostly pre-populated data. The ERP software will be able to spot
discrepancies in a shop order based on factors that were overlooked by the sales engineers who entered it.
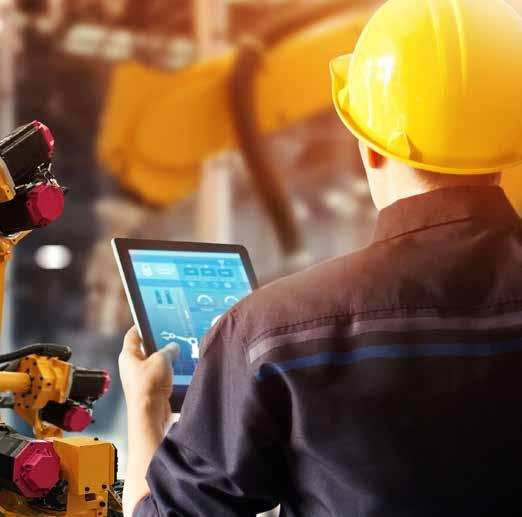
Further upstream, AI will already have been used to qualify the lead from the prospect, and machine learning services examine resulting conversions and adjust the lead qualification algorithm to meet corporate goals.
TO ERR IS HUMAN One thing that AI will excel at is processes that require more consistency and attention to detail than humans are capable. All of us can remember times human error had high cost—something as simple as entering a purchase order in Yen pounds instead of British pounds, which would snarl cash management in ways that may be difficult to track down. AI will spot things that are incongruent because it is not thinking about having lunch, or being yelled at by the boss or the next task to be completed.
Matching invoices to purchase orders is also typically not on anyone’s list of favorite things to do, and AI can take on this work and do a better, faster job than people who frankly would rather be performing some other function that is more meaningful for them and uses them for their unique abilities and aptitudes.
At one point in my career, I was sent to a company to unravel why they had matched only 7 percent match on their receivables and accounts payables to purchase orders versus 97 percent which was common in the rest of the company. It turns out that users had configured the system to match on purchase order and advice note. Unfortunately, the goods inwards clerk was never told so he entered any old number in the advice note box so nothing matched.
It took some doing but in a short space of time, more than 4,700 goods received notes (GRNs) were matched to purchase orders. This eliminated serious problems including payments generated automatically to suppliers that had already paid and allocating GRNs to the incorrect purchase orders and projects. AI can match received goods and payments to purchase orders faster and more reliably, and simple but impactful steps like this represent the low hanging fruit for manufacturers’ digital transformation efforts.
PICKING YOUR FIGHTS WITH MACHINE LEARNING Enterprise software vendors can automate broad swaths of the business all at once, but the smarter approach may be regular automation package releases to address one part of the business at a time.
As AI is introduced into a given function, and as a transactional record accumulates to demonstrate the effectiveness of the algorithm, machine learning bots can suggest or make changes to that process to optimize it for performance or around changing conditions or corporate goals. It will be easier to automate a slice of the business than to apply machine learning to that automation, so machine learning will probably be applied more judiciously, starting with higher value use cases. Executive teams will want to apply tests of criticality and constraints to determine where machine learning should be applied
That long run paper converting line that, in the event of failure, only produces a few unusable baby wipes may not need a lot of machine learning. That food and beverage line however could optimize its production and maintenance schedules around hypoallergenic and organic production runs could stand quite a bit of machine learning to squeeze more productivity and higher quality out of fixed resources. A semiconductor manufacturing operation may benefit from extremely detailed analytics of quality to maximize yield by spotting excursions, and computing their monetary cost against the cost of systemic improvements required to resolve them.
Colin Elkins VP, Manufacturing Industries, IFS
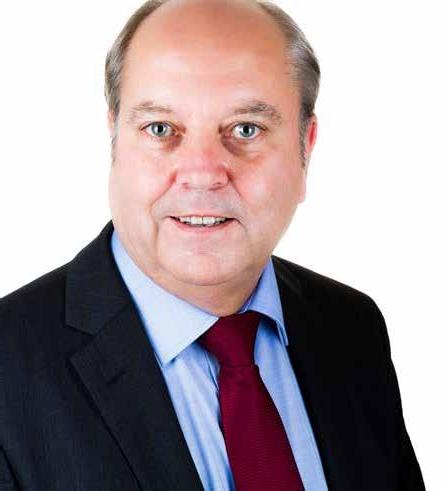
This situation is too dynamic and multivariate for humans to, in real time, compute an optimal approach. Machine learning may be a wise investment here.
OPTIMIZED PRODUCT LIFECYCLES FROM DESIGN TO SERVICE Manufacturers think in terms of products, but really deliver to customers something more ephemeral—customer experiences that consist of moments of service in terms of delivery, product performance, and support, through end of life and decommissioning or replacement and recycling. Each step in this product lifecycle is related to the other
In one manufacturing planning process I was involved in, we manufactured axles that extend down a mine shaft with a small, sealed gear box. The seal on the gearbox was essential because it would ensure performance by preventing dust and other contaminants from getting into the gears in the harsh mining environment.
On a hot, humid day, there are certain chemicals in the seal that would not cure properly. Gear boxes made on that day would often fail because of an inadequate seal, and moisture and dust would accumulate into a soupy slurry.
In manufacturing large cast-iron pinion gears, I have seen them distort in a furnace to the point that you then need to use a gear press to press them in place. AI could predict how that gear would move and then you could redesign the product blank, so it distorts into the proper shape. This makes for a more performant and reliable gear, less machining time and less waste due to grinding.
More grinding makes a weaker gear, and moist weather makes an unreliable gear box. AI can help connect the dots between earlier stages in the product lifecycle and the experiences customers have with them, the cost of warranty repair or as part of an annual service plan.
A cognitive service in ERP software can capture data on every gear that failed, including photos, records of machines that touched it, what furnace was used, the supplier of the steel and make meaningful conclusions on how to profitably improve the customer experience. In short order, an executive team could ascertain why certain gears lasted for decades and others could fail within a few months. Maybe a furnace was not calibrated and was not forming the adequate crust of hardened steel on the part. Maybe the HVAC or building automation in one plant controls humidity better than another, leading to a more performant seal. AI can separate the relevant few data points from the inconsequential many.
Whether sending a technician to perform service on a customer site or ensuring adequate supply to a retail channel, AI will be able to solve problems better and faster than people can.
The director of service in a machinery manufacturer may benefit from AI optimized scheduling that can compute the likelihood of solving a problem in a single trip, and move these more difficult service calls up earlier in the day so the entire service cycle can be completed within a service level agreement. A food manufacturing planner can shore up their educated guesses on what inventory should be sent to which retailer in a synergized inventory system based on past order history, weather and anticipated traffic patterns. Planners are smart, but AI is smarter, can look at more variables and lay for itself through increased sell-through, reduced spoilage and a more satisfied and loyal customer.
START WITH THE LOW HANGING FRUIT Think about enterprise software and AI long enough, and you can imagine a broad and all-encompassing collection of bots and machine learning—an autonomous business. We may realize elements of that sooner than later, but most businesses will want to take a gradual approach—simple and fast wins with immediate ROI and ongoing opportunities for process improvement.