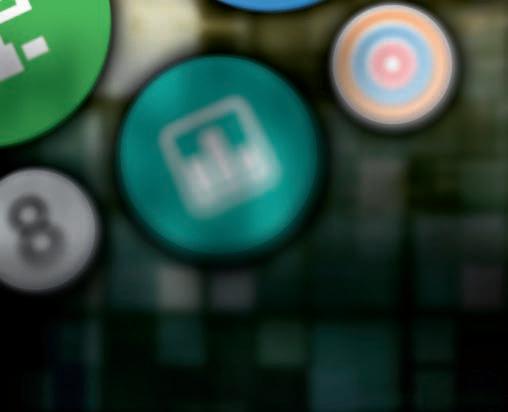
25 minute read
INTERVIEWING
It’s All about Context: Part 2
In our last column we began the discussion of how context affects the conversation and the questions that can be asked during it. It’s important to understand that context changes as a conversation evolves. What might begin as a discussion about fast-food restaurants evolves into a discussion about the different restaurants and finally focuses on a single restaurant chain. Because the context has evolved into a discussion about a single chain, it might be appropriate to ask the other party, “How many times have you eaten at McDonald’s in the last month?” There is a tacit admission from the second party that he has eaten at McDonald’s even though he has not verbalized it. So asking the question assumptively is clearly appropriate. Lacking a context for the conversation about restaurants, it would be better to ask, “Have you ever eaten at McDonald’s?”
Context is not only about providing the subject matter of the discussion, but also revolves around the individual’s mindset as the conversation evolves. For example, an individual with something to hide will be threatened by a conversation where a truthful person views the topics and questions with nothing more than interest.
The Individual’s Mindset
Context is not only about providing the subject matter of the discussion, but also revolves around the individual’s mindset as the conversation evolves. For example, an individual with something to hide will be threatened by a conversation where a truthful person views the topics and questions with nothing more than interest.
In a recent case relating to a large fraud, a manager was asked to have a conversation with one of the authors of this column. Since being asked to come to the corporate part of the facility was unusual, he was at first suspicious and extremely defensive. However, it wouldn’t necessarily be unusual for the individual who is innocent to be somewhat nervous until he discovers the context of the meeting. But in this situation, the individual was concerned that his fraud had been uncovered, and instead of mere nervousness, he exhibited suspicion and defensiveness in his questions and answers.
As the interview developed the conversation moved from biographical information to background information relating to his promotions and business-development practices. His overall attitude changed from suspicious and defensive to boastful as he talked about how he had brought about the significant increase in business over the last several years.
As the conversation wound its way closer to the topics relating to the fraud, his attitude changed from boastful to fearful with his answers becoming shorter and more evasive as the conversation moved closer to the fraudulent activity. So an interviewer must manage the changes in emotional and psychological mindset of the subject as he progresses through the conversation and its contextual change.
The non-confrontational interview we advocate does several things extremely well. First, it provides a context for the conversation relating to the investigation of company matters. Second, it affords the interviewer an opportunity to assess the subject’s behavior during the presentation of a monologue describing what the investigator’s job is. During this monologue the same words are viewed very differently by innocent or deceptive individuals. The innocent person has done nothing wrong and perceives the investigator’s monologue as a simple description of what he does for a living. The innocent person views the conversation with interest, and this is supported by his open posture and non-threatened demeanor. However, the dishonest individual experiences the conversation in an entirely different fashion. For him the conversation is a disquieting and fearful experience as he comes to the realization his dishonesty may have been discovered.
by David E. Zulawski, CFI, CFE and Shane G. Sturman, CFI, CPP
Zulawski and Sturman are executives in the investigative and training firm of Wicklander-Zulawski & Associates (w-z.com). Zulawski is a senior partner, and Sturman is president. Sturman is also a member of ASIS International’s Retail Loss Prevention Council. They can be reached at 800-222-7789 or via email at dzulawski@w-z.com and ssturman@w-z.com.
© 2015 Wicklander-Zulawski & Associates, Inc.
Biographical Information
Although the admission-seeking interview can take many paths, the process almost always begins with confirming biographical information and establishing a behavioral norm for the individual. Confirming the biographical information is essentially a neutral context that is collaborative in nature. The interviewer and the
subject are simply working to confirm information that is already known. For the interviewer there are several other purposes that are in play.
First, the interviewer is looking to establish the behavioral norm of the individual under this given set of circumstances. Essentially, the interviewer is looking to confirm how the subject answers truthful biographical inquiries. Here the interviewer develops a behavioral context that he will use to compare against later topics, questions, and words.
Second, the interviewer is looking to identify how the person looks, talks, and acts when retrieving short- and long-term memory information.
Finally, these inquiries allow the interviewer to overcome any initial nervousness and to establish control over the direction of the conversation.
For the subject this portion of the conversation is collaborative in that he is simply working with the interviewer to confirm what in most cases is already known. This might not be true during a field interview where an officer has approached an unknown individual with little or no background information available to him about the person. In that case it is possible that the subject is not providing accurate information because he has something to hide. With the exception of the field interview, other investigative interviews will already have accurately identified the subject and developed some biographical information about him or her.
It is possible that the subject may exhibit some level of nervousness whether he is truthful or untruthful at this point in the conversation. Often because the full context of what is going to be discussed has not been laid out, there is a level of uncertainty that manifests itself as nervousness. One might equate this to going to the dentist. Very few people enjoy their visits to the dentist because of the possibility of potential unpleasantness in the form of filling a cavity. However, the initial nervousness and uncertainty diminishes almost immediately after the dentist informs his patient that he has no cavities. Just a few minutes of discomfort while cleaning the teeth, and the visit is over until the next scheduled appointment.
Rapport Building
The context now shifts from a neutral collaborative discussion of biographical information to eliciting more personal data. Depending on how this shift is done, it may elicit rapport, suspicion, defensiveness, or even aggression in the subject. The purpose of this conversation is to begin the process of rapport building.
Rapport involves establishing trust between two parties and begins by identifying common experiences between the individual and the interviewer. The time spent here can be valuable for the interviewer in several ways.
First, it establishes reciprocity between the subject and the interviewer. Reciprocity is essentially an obligation that requires a repayment. For example, if you are invited to dinner by a friend, there is an unspoken obligation to repay that debt at some point. Here the debt is allowing the interviewer an opportunity to speak about himself after the subject has had his opportunity; the introductory statement is introduced.
Second, it provides the interviewer a context relating to the individual’s personal life and what is important to him or her.
Third, it may also suggest rationalizations that minimize the seriousness of what the person has done, thus protecting the subject’s self-image.
Creating a context of trust and safety occurs over time and is generally not something that happens in a few short moments. Rather it is like putting building blocks in place. True rapport occurs in the moments just before the subject makes his first admission and is the result of making sure all the building blocks are in place. At that point the individual finally feels comfortable enough with the interviewer to share derogatory information about himself. An example of this would be how we might tell a story that is embarrassing about ourselves, but we only do so when we are comfortable with the people around us.
At the beginning of rapport, the context is one of sharing information that is not embarrassing or threatening to the individual’s self-image. The interviewer is simply expressing interest in some portion of the person’s life experiences that may in some way match his own. This could be as simple as a discussion of the inclement weather the subject had to travel through to get to the interview. A shared experience, which could be a person, place, or thing, creates a linkage between the subject and the interviewer providing the foundation for full rapport to develop.
Your favorite person in the world is yourself, and to have another be interested enough to inquire about your life experience can be quite flattering. At the end of the rapport building, the interviewer hopes to achieve a context where he is viewed by the subject as either neutral or in a somewhat favorable light.
As we have seen, the context between two individuals will vary as the conversation precedes and different topics are covered. It will also vary based on the perception of the individual and the threat the topic being discussed poses to their self-image. In part three of our discussion, we will focus on the transition of context that occurs during the admission-seeking interview and how it is viewed by both the interviewer and subject.
Your favorite person in the world is yourself, and to have another be interested enough to inquire about your life experience can be quite flattering. At the end of the rapport building, the interviewer hopes to achieve a context where he is viewed by the subject as either neutral or in a somewhat favorable light.
FINISH LINE IS WINNING BIG WITH BIG DATA
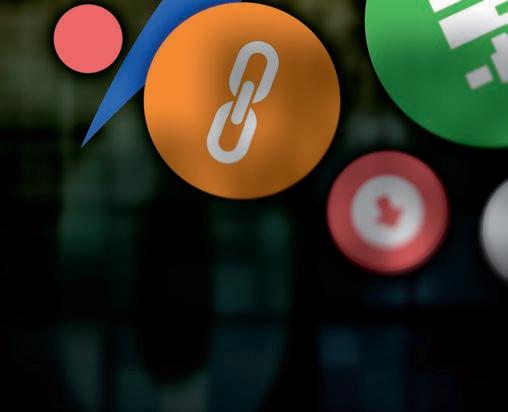
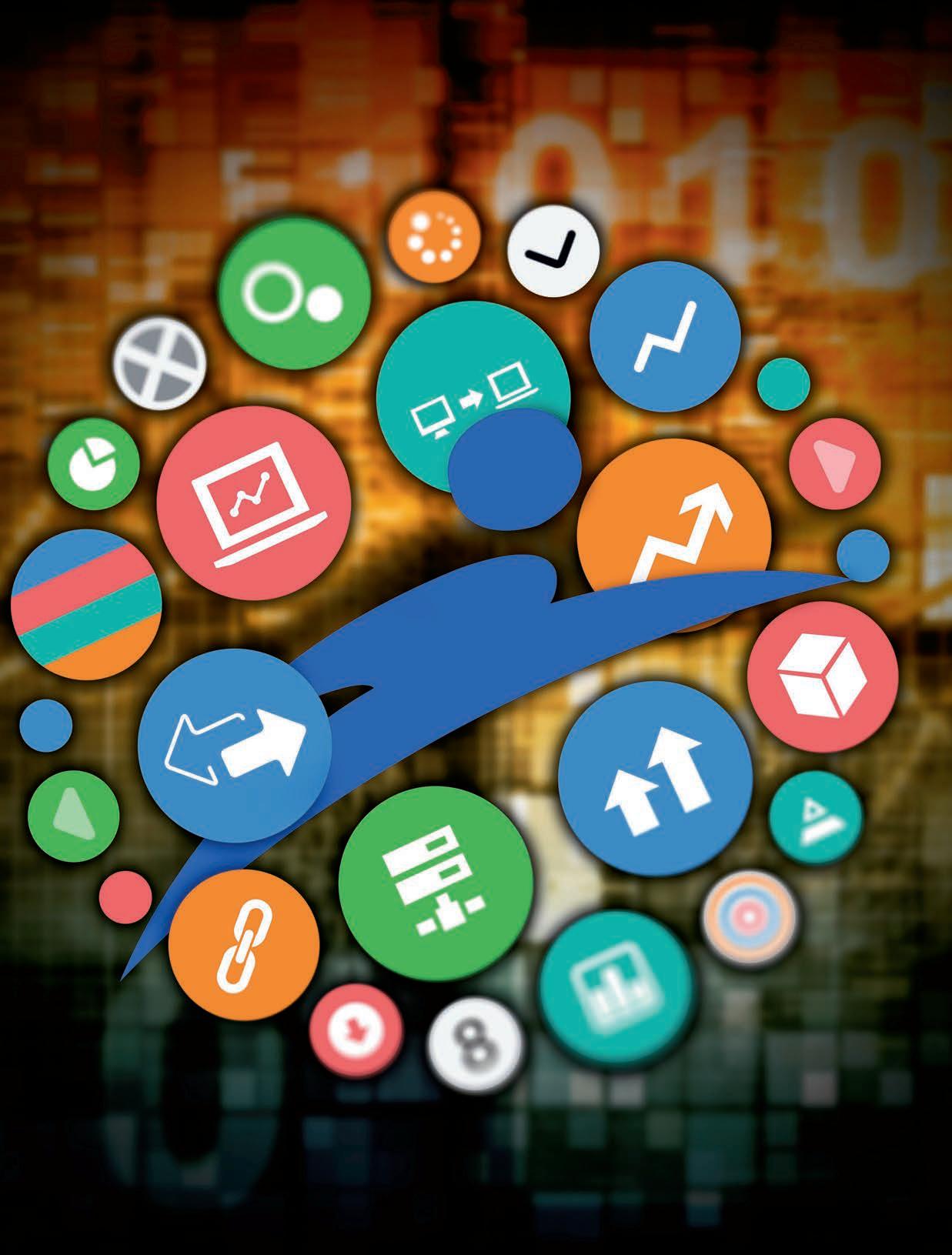
By Mike Smith and David Speights, PhD
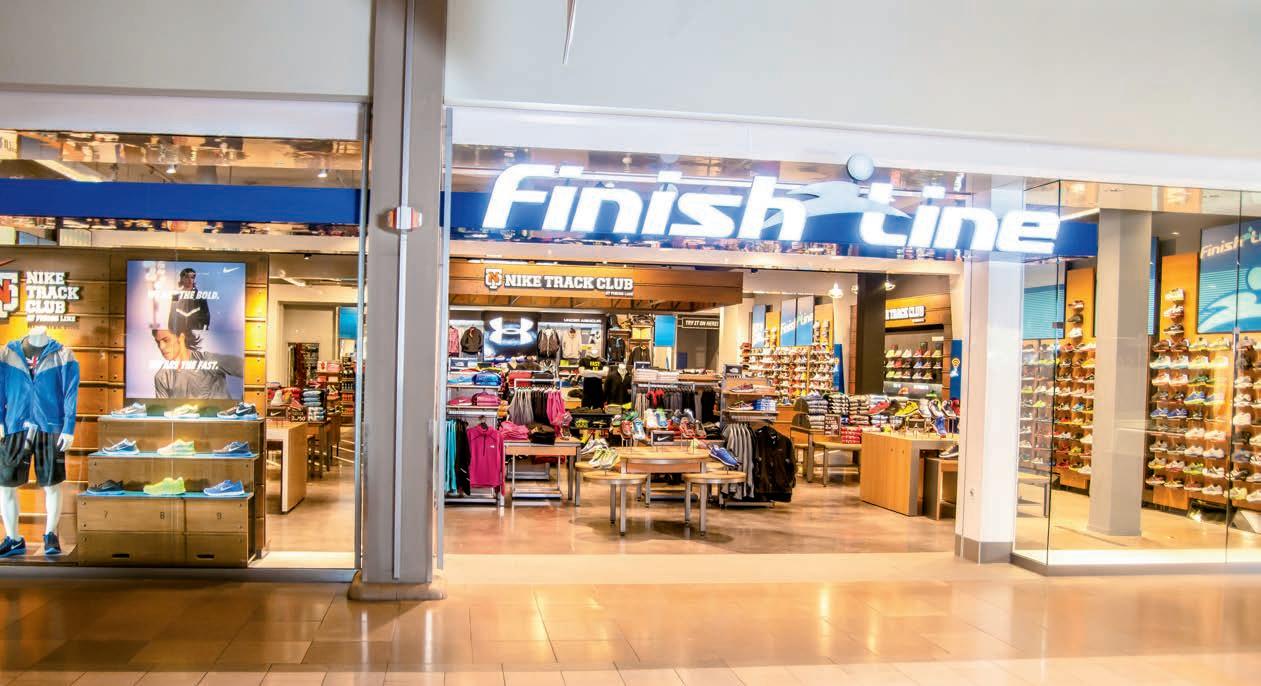
Return fraud and abuse continues to cost retailers billions of dollars every year—$16 billion to be exact. But some retailers like Finish Line, a $16 billion premium retailer of athletic shoes, apparel, and accessories, are fighting back and combatting return fraud head on with winning results.
Headquartered in Indianapolis, Finish Line operates approximately 1,400 branded locations primarily in US malls and shops inside Macy’s department stores and has a strong online retail presence. With a mission to connect to young, fashion-conscious individuals through a premium-brand experience, Finish Line’s retail stores play an important role in delivering this premium experience. More than 14,000 “sneakerologists” are employed across the country, so maintaining a consistent loss prevention strategy and process is paramount to preserving that premium customer experience.
Seeking to protect the company from return fraud while continuing to provide an inviting customer experience, in 2005 Finish Line turned to a real-time, consumer-based return authorization solution called Verify from The Retail Equation. It helps prevent fraudulent and abusive transactions from occurring by using predictive analytics to identify and stop high-risk returners, increasing net sales, and reducing return rates, while ensuring all legitimate returns can be made.
Creating an effective returns policy that protects the company from loss without negatively impacting sales or customer loyalty requires a careful balance; it’s both an art and a science. This solution quickly became a valuable tool to streamline the return processes at the point of sale, help enforce the return policy, and provide management discretion to evaluate suspect returns as needed.
A Powerful Resource for the Whole Team
Understanding that the return authorization solution could bring far more benefit to the company than just within the loss prevention department, we collaborated with Finish Line’s IT and operations departments through the selection and implementation processes. Our LP team had the foresight to know that a technology spending decision should not be made solely through the scope of loss prevention because the success of this implementation would depend on—and benefit— virtually every department.
Several years after we implemented the returns management technology, our foresight has proved to be true. By understanding return patterns through the data captured in the system, we have learned about merchandise categories, product groups, and specific items that can help loss prevention, merchandising, operations, and even our vendors. We have a tool that can truly curb return fraud without alienating loyal customers and deterring future sales. And we were able to integrate the system with our existing exception-based reporting application to provide a clear picture of what’s happening in the business.
Furthermore, for operations executives and in-store teams, the solution has helped tighten POS processes and controls. In effect, we
have helped improve the consumer’s return experience and engender their loyalty through a quick, efficient, and easy-to-manage return process that eliminates all guess work from our store employees.
A Historically Strong Offense against Return Fraud
The solution helps quickly determine whether a return is accepted or declined—guiding the salesperson and removing the guesswork. While each retailer can choose the best way to identify consumers, previously with every return at Finish Line, the customer was required to present a valid government-issued identification, which was then swiped at the point of sale (POS). Pertinent data was captured in the system, encrypted for security, and instantly run through a series of predictive algorithms and statistical models. Almost immediately, the system generated one of three recommendations for the return—accept, warn, or decline.
The automated returns tool improved the consistency in Finish Line’s return procedures. The solution’s analytics backbone consistently uses the same parameters to apply the return policy guidelines, as opposed to a cashier having to make a subjective decision with each return—a situation that can escalate emotions and lead to customer dissatisfaction or accepting fraudulent returns in a panic, both of which are very damaging to a retail company.
Taking a proactive, analytics-driven approach to return authorization helped deter fraudsters and shoplifters who often attempt to return stolen merchandise for cash. Any attempt to return merchandise with frequency is detected, and immediately the transaction is flagged, and the store associate is notified to deny the transaction. Prior to this system, we were unable to capture that data, much less make it available in real-time.
By understanding return patterns through the data captured in the system, we have learned about merchandise categories, product groups, and specific items that can help loss prevention, merchandising, operations, and even our vendors. We have a tool that can truly curb return fraud without alienating loyal customers and deterring future sales.
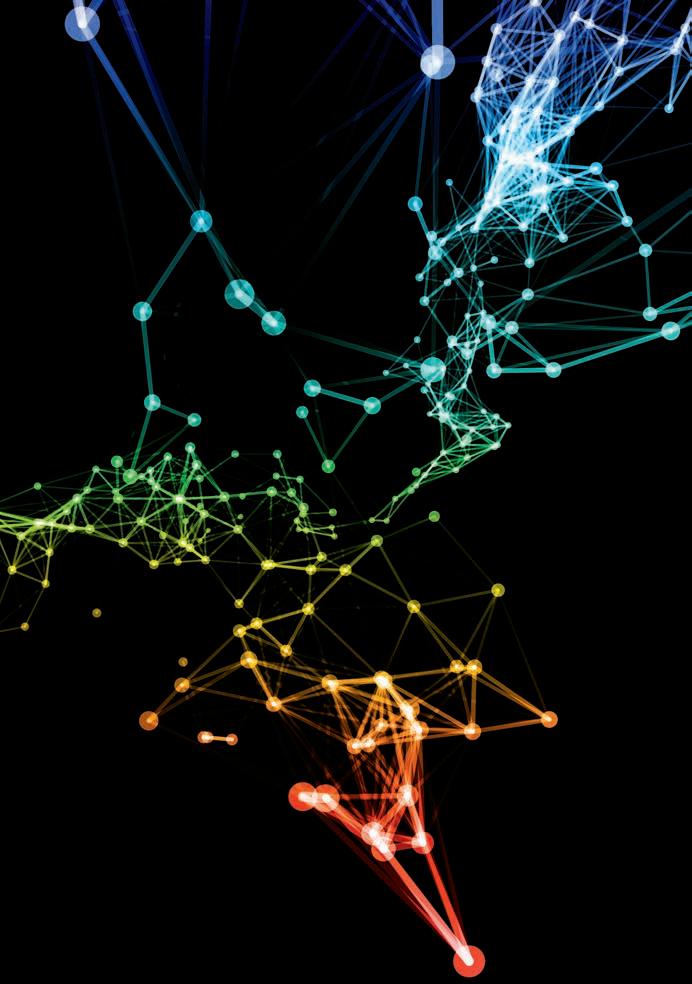
Customer Linking Mechanics
Information gathered from a single return can be tied to more data that becomes useful across the organization.
LOYALTY NUMBER ID COLLECTED ON RETURN
TRANSACTION INFO
MERCHANDISE INFO CARD HOLDER DATA
CUSTOMER RETURN
ORIGINAL SALE ASSOCIATE DATA
eCOMMERCE ORDER
SHIPPING DATA INVENTORY VARIANCE
It’s important to increase awareness about overall return activity now and as policies and procedures change. Store operations can reinforce policies with all store associates and be actively engaged in the shaping of new, profitable customer behaviors. Marketing is enhanced with a better loyalty program. Merchandising can be more creative. E-commerce is streamlined.
With this increased insight and intelligent decision making come cost savings. We recognized a return on investment through an improved rate of return within one year of implementation, and we expect to again see a decrease in return rate year-over-year when we re-deploy the solution with our new POS system. Shrink was also down markedly over the same time period. Additionally, there were improvements in customer experience, greater POS efficiency, and greater profitability thanks to reduced loss.
Leveraging the Future to Create More Loyal Fans
The most exciting part of Finish Line’s new solution deployment is our ability to directly enhance the return experience for our loyalty customers. Very soon, members in our Winners Circle program will need to present a government ID at return only once, and then every time afterward they will be identified by their loyalty info. This provides multiple benefits: ■ It makes the return process easier and more streamlined for Winners
Circle members, ■ It creates an “easier returns” selling benefit to attract and sign up new loyalty members, ■ It gives Finish Line associates an additional reason to ask a customer for their Winners Circle number at time of purchase, which gives us even better overall purchase history, and
Customer Linking Details
The data type in each column has key variables that allow it to be connected with other data types.
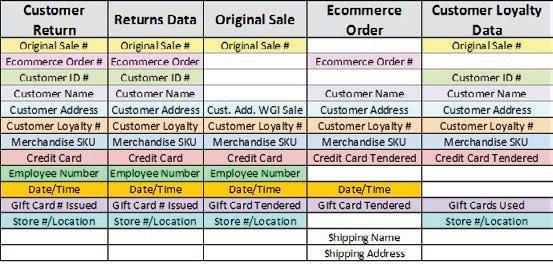
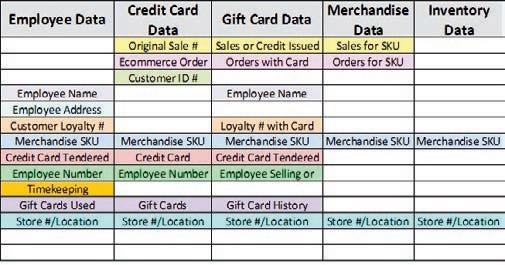
■ The collection provides more big-data transaction information tied to specific consumers to help my business partners in store operations, marketing, e-commerce, and merchandising.
By capturing this data in real-time at POS, we will provide greater insight into shopping trends and patterns and even better strategies to best reach customers and maintain loyalty. Additionally, an intelligent return authorization solution can help treat different consumer segments uniquely. We could add even more value to our loyalty program when these best shoppers can be quickly recognized and given a more lenient return policy. Ultimately, it creates a more premium return experience for Winners Circle customers.
We are also creating an omnichannel environment by building a seamless return process between all business channels, as well as an expedited return/exchange process at the POS for both the customer and associate. Imagine implementing a “buy online, return in-store” policy with an easy way to validate that the purchase was actually made from your e-commerce site. This is very important for Finish Line since a majority of returns made from e-commerce sales are performed at stores and not sent back to
DELIVERING SOLUTIONS FROM SOURCE TO SHOPPER.
FROM THE SOURCE TO YOUR SHELVES.
Checkpoint solutions enable you to increase sales by ensuring that the right merchandise is available at the right place and time. • Manage inventory e ciently • Increase on-shelf availability • Secure high-theft merchandise • Improve the shopper experience

VISIT OUR BOOTH AT NACDS!
Booth #3348 Denver, CO August 22-25
the distribution center. The risk of these returns is greatly diminished, and the store can now focus on the shopper experience as opposed to fraud mitigation.
These features are just scratching the surface. Knowing shoppers and their behavior in real-time, in-store is a huge advantage that adds value to the business.
The Power of Connected Big Data
When it comes to gaining insight into shopping habits and trends, there is no question that knowledge is power. Finish Line and other retailers are embracing data tools like exception-based reporting, predictive modeling, digital video, and ROI analysis to help identify previously hidden relationships to take decisive action to improve customer service and reduce the specters of fraud and shrink.
Here’s how it works. Using analytics on top of linked data, we can build connections that reveal more about the customer. The better informed a retailer is about its shoppers, the better it can create strategies to market to them to generate future sales that meet the buyers’ needs and expectations, and therefore minimize returns. In Finish Line’s case, detailed data captured during a return transaction can ultimately reveal trends and preference that can help us provide better incentives, greater customization in the shopping experience, or additional merchandising flexibility.
For example, let’s say a new shoe is hitting the market that is flashier and more colorful than shoes in the past. We decide to merchandise the shoe prominently on a display at the front of the store. As a result, the new shoe flies off the shelves, but then gets a lot of returns. The return data—what sizes, colors, styles were returned more—can be analyzed to separate potential fraud from other consumer issues, and ultimately provide insight to other departments to help determine, for instance, whether certain colors are less popular and should no longer be
Big Data Is Reshaping Retail LP Operations
by David Speights, PhD
Businesses, including retailers, are losing 5 percent of revenues to fraud every year according to the Association of Certified Fraud Examiners’ 2014 Global Fraud Study. This is a staggering number, especially when applied to the 2013 estimated gross world product, which translates to nearly $3.7 trillion in potential projected global fraud loss.
Many retailers are taking action to combat fraud by employing new technologies to monitor high-risk retail transactions. This is helping reshape retail loss prevention operations to deliver a better customer shopping experience, while effectively protecting company bottom lines.
Technology Enhances the Collection and Monitoring of Data
Retailers collect data from many sources, including store sales transactions, store video, traffic counters, alarms, merchandise movement, loyalty programs, e-commerce click paths, and much more. A large retailer collects millions of transactions and hundreds of millions of line items per day. To that, they add 30 to 60 gigabytes of video per store, per day. For a 1,000-store retailer, this could total 22 petabytes per year, or the equivalent of 23,068,672 gigabytes.
Conventional systems like exception-based reporting and data-mining systems uncover direct relationships that occurred in the past on a single identifier. But big-data analytical tools take analysis to a new level by detecting the connections among seemingly unrelated identifiers to reveal underlying larger groups of transactions and individuals. For example, a return authorization technology like The Retail Equation’s Verify-3 solution helps prevent fraud and abuse in real-time during the return process, not the day after, while simultaneously linking in all related information to an individual. This type of response is not possible with conventional systems because they simply cannot process the complex analytics and deliver accurate answers fast enough to authorize a transaction in process.
Many companies and solution providers have approached the data size or analytics problems by investing in a small number of bigger, faster hardware machines. This works up to a point. However, the massive amounts of data building up in the system and the complex analytical methods required to unearth the information is more than most traditional computing architectures can handle.
Big data-oriented companies achieve high-processing speeds by using special tools like the Hadoop platform to split data into thousands of chunks and distribute the load across a very large number of machines. We have developed custom software to operate in this environment to tackle very tough analytical challenges. Additionally, tools like IBM’s PureData (formerly Netezza) data-warehouse appliances are also used for added throughput and operate on a similar parallelprocessing architecture. This architecture decreases processing time by more than 90 percent versus more traditional hardware/ software deployments. A query that may take five hours to process using SAS on a conventional single-server system takes only minutes on a Hadoop or PureData parallel-processing architecture. In the field a return authorization completes in milliseconds.
Knowing Precisely What to Look For
Predictive algorithms and machine learning techniques rely on big-data tools to quickly improve the shopping experience and reduce return fraud and shrink simultaneously. Companies can process the data from all the
No. IDs = 72 No. Credit Cards = 122 No. Loyalty Cards = 84
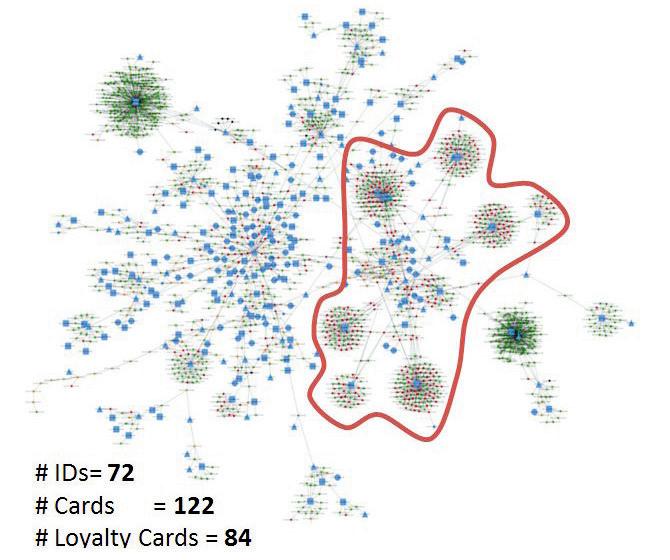
transactions in the chain and identify suspicious behavior indicative of any form of return fraud or abuse, including wardrobing, returning stolen merchandise, receipt fraud, price arbitrage, price switching, double dipping, check fraud, and tender fraud. When an individual attempts to make a return, systems perform calculations and in a fraction of a second predict the likelihood of whether the return is fraudulent.
While the vast majority of consumers (about 99 percent) are approved, those whose actions are highly suspicious are warned or denied. This is an important point to notice. The system allows and supports generous return policies, so profitable consumers enjoy a fast and pleasant return process, including those who make numerous returns. In fact, the most valuable consumers tend to have a very high number of returns, which is why it is best to not rely solely on simple returnvelocity calculations, but rather use big data to identify fraudulent patterns of behavior in real-time.
Complex queries can also be used to identify organized retail crime rings and fraudulent returners by linking seemingly independent events. The diagram above shows a cluster of suspicious purchase and return events.
At the center of each dandelion-like cluster is one person (or one ID). The thin lines connecting the clusters show a “hidden” connection, such as a gift card being passed among conspirators. On their own the clusters may appear to be legitimate (high-volume buyers often return many items), but the value of the returns exceed the value of purchases, and the connections indicate probable fraud by a group or an individual using multiple identities. Software-as-a-Service applications can halt the group’s returns immediately, and special reports can provide investigators with the information necessary to pursue a case. The bigdata tools allow companies to maintain and update the linked identities on more than a billion linkages each day.
The amount of return-related fraud is a staggering $9 to $16 billion problem according to the 2013 Consumer Returns in the Retail Industry report released in January 2014 by the National Retail Federation. Many of these losses are preventable using the technology available today. Fraudsters depend on system delays and lapses in judgment by the cashiers and associates on the front lines.
However, when big-data analytics replace subjective decisions, fraud and shrink diminish substantially, reducing return rates by an average of 8.2 percent and shrink by 12.95 percent. A $2 billion retailer would see about $15 million in savings per year, and retailers see a steep decline in return rates beginning immediately after the system is live.
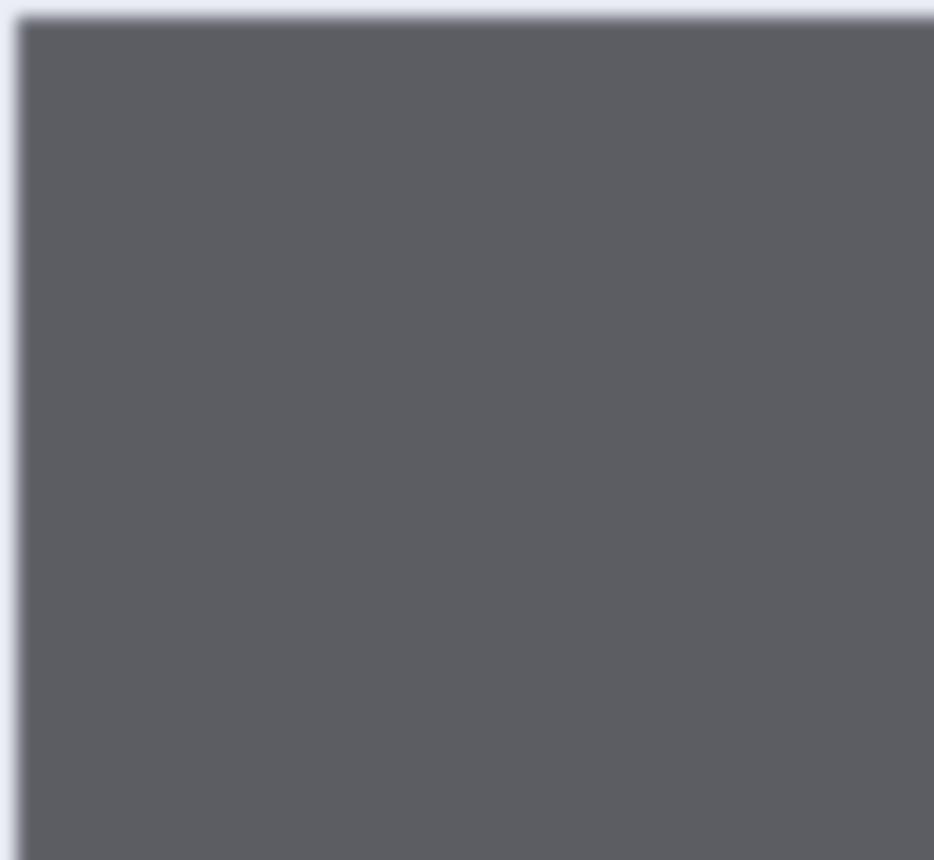
continued from page 20
carried, or how merchandising displays might be designed differently to entice and help the shopper make a lasting purchase.
Our primary objective with implementing this technology was to create a better consumer experience in our stores, while at the same time to get a handle on fraudulent returns, which are expensive for retailers. We then started to see other opportunities emerge by looking at the data captured in the system to help modify other processes by adjusting to meet customers’ interests and expectations.
Collecting data and impacting business on a greater scale creates significant benefit. At Finish Line, the visibility all starts with the customer’s return. Over time you learn enough to link all of the data and have a holistic view of the customer. The customer linking mechanics illustration on page 18 shows the many different ways to build the connections from the original consumer return transaction. It is then possible to drill down even further through common threads of data to link disparate points.
Each data type has variables allowing it to be linked with other data types. As shown in the customer linking details chart on page 19, beneath each high-level data type listed as a column heading are keys that you can link from one type of data to another. For example, employee number can be easily linked to customer name and then to other disparate data. The ability to take this additional information and tie it into existing and future analytics tools is very important.
For Finish Line these links add significant value to the analysis effort already in place with our
With infinite ways to use this data to improve business and gain intelligence, we have a tremendous cross knowledge of returns with inventory and employee behavior. To fully realize the benefits, it’s important to educate your business partners in store operations, marketing, e-commerce, and merchandising about customer behavior.
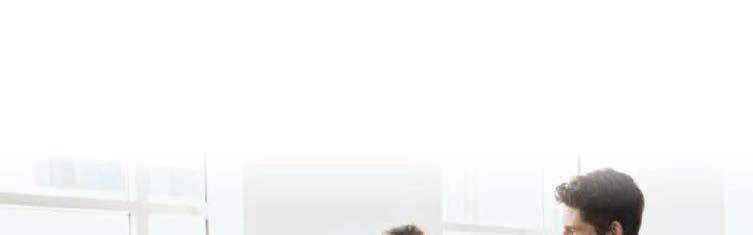
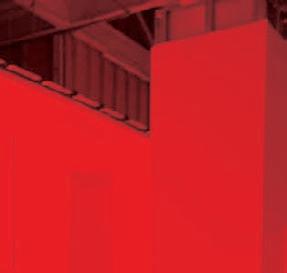
16
September
Retail Council of Canada The International Centre Mississauga, ON 1/2 Horizontal Page
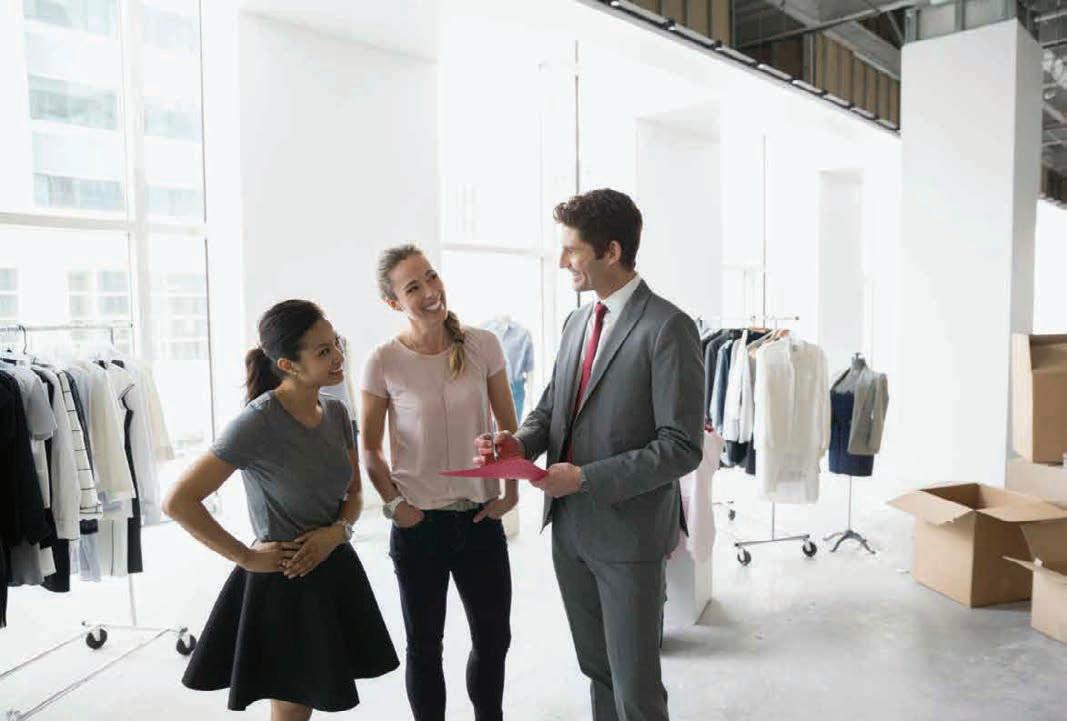
Canada’s Leading Retail LP Conference REGISTER TODAY!
RCCLPConference.ca
Presented by Produced by
exception-based reporting tool. In one case we started with a SKU that was missing in a physical inventory. We linked that SKU to a refund transaction during that inventory period and then connected it to the employee performing that refund.
With infinite ways to use this data to improve business and gain intelligence, we have a tremendous cross knowledge of returns with inventory and employee behavior. To fully realize the benefits, it’s important to educate your business partners in store operations, marketing, e-commerce, and merchandising about customer behavior. With everyone on the same page, the real benefits are achieved, including an improved customer experience while shaping customer behavior.
The Benefits to the Bottom Line
The more you know about a customer, the more you can affect your bottom line. Authorizing returns may have started as both a customer service improvement and fraud prevention initiative, but it has proven to be so much more.
Everyone in the organization will benefit from this scientific, analytics-based understanding of overall customer behavior. It’s important to increase awareness about our overall return activity now and as our policies and procedures change. Store operations can reinforce policies with all store associates and be actively engaged in the shaping of new, profitable customer behaviors. Marketing is enhanced with a better loyalty program. Merchandising can be more creative. E-commerce is streamlined.
At Finish Line this is much more than a loss prevention initiative; it involves the entire company. And the entire company has and will continue to benefit greatly from big data.
MIKE SMITH is the senior vice president of loss prevention for Finish Line. With more than thirty-seven years of experience in retail loss prevention, Smith has held various LP positions with Montgomery Ward, Maison Blanche Department Stores, and Mercantile Department Stores. He holds a bachelor’s degree in English from the University of South Alabama. He can be reached at msmith@finishline.com.
DAVID SPEIGHTS, PhD, is the chief data scientist at The Retail Equation. He has more than fifteen years of experience applying advanced analytical methods to solve business problems in multiple industries. Speights holds a PhD in biostatistics from the University of California, Los Angeles and holds several patents. He can be reached at dspeights@thereturnexchange.com.
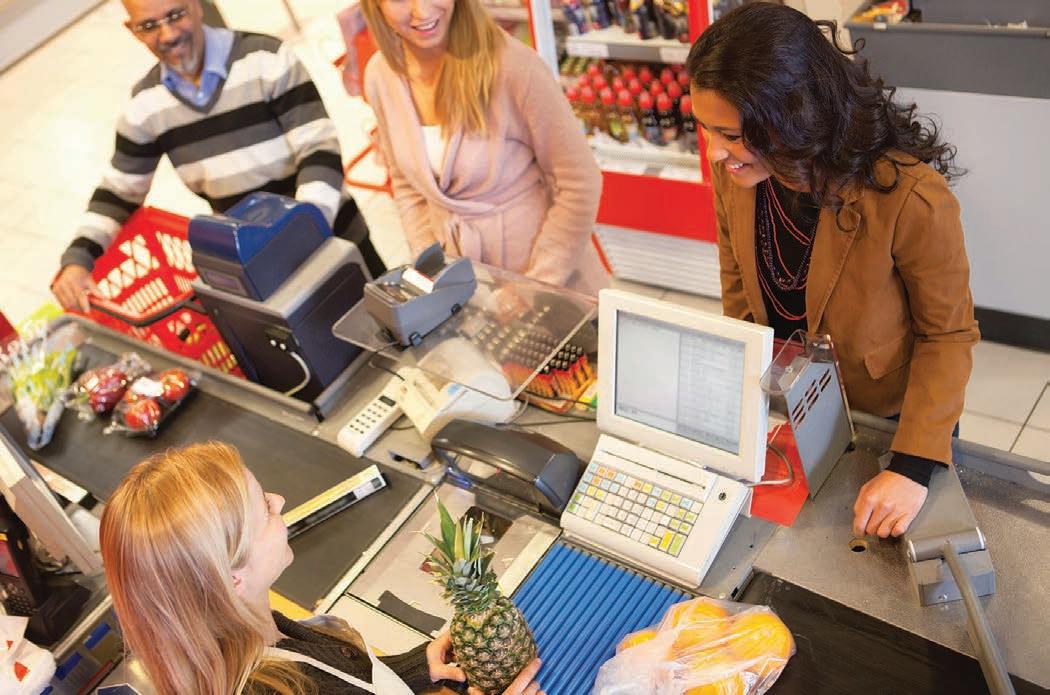
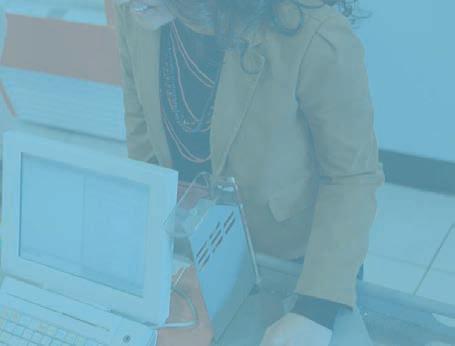