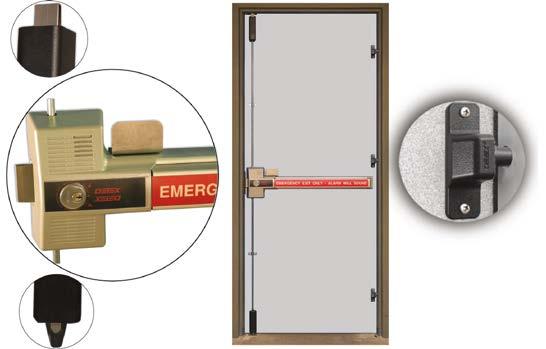
11 minute read
SOLUTIONS SHOWCASE
Is Your Back Door Protected?
Detex introduces a maximum security, multipoint lock so big and strong that it stands up to assault by the bad guys and reduces employee and customer theft. Exclusive to Detex, the ECL-230X-TDB is a heavy-duty, easy-to-install, three-bolt, multipoint lock whose construction takes panic hardware to a whole new level of toughness and eases your back-door security worries about the bad guys peeling the bottom of your back door to gain entry.
Built for maximum strength, it is designed with a larger deadbolt that goes deeper into the frame than other locks in the category. Connecting rods are solid steel rather than the less reliable, hollow rod/cable construction. Life-safety and code compliant, the new Detex ECL-230X-TDB serves as both panic hardware and a maximum-strength locking device.
The ECL-230X-TDB includes a photo-luminescent sign available in more than ten color and language combinations, a 100-decibel alarm, and three locking points per door: ■ Top deadbolt—approximately 1-inch wide by ½-inch thick, the top bolt provides additional stability to the top corner of the door. ■ Side deadbolt—at 2¼ inches tall by ½-inch thick with a 1-inch throw, the side bolt allows for ¾-inches of penetration into strike. (Detex recommends flush installation for maximum security.)
With more than 1½ square inches of bolt engagement, the side deadbolt provides superior defense against pulling and prying on the side of the door. ■ Bottom bolt—this 5/8-inch HEX bolt with ¾-inch throw engages the floor with 5/8-inch penetration and provides better attack resistance and superior defense against the “peeling up” of the bottom of the door. Together, the three bolts withstand 16,000 pounds of pull force.
Add suffix DX3 (ECL-230X-TDB-DX3) for six locking points for even stronger security, providing an additional line of defense against break-ins. Even if the exterior door hinges are compromised the DX bolts keep the door locked and secure. These passive deadbolts are easy to install and offer another layer of attack resistance. Through-bolt mounting installation improves the door’s holding strength. You choose the right application and level of protection by choosing one, two, or three bolts for a total of four, five, or six locking points.
Other 230X models include: ■ ECL-230X (dead bolt only) ■ ECL-230X-W (weatherized dead bolt) ■ ECL-230X-TB (top and bottom bolt) ■ ECL-230X-W-TB (weatherized top and bottom bolt) ■ ECL-230X-TD (top and dead bolt) ■ ECL-230X-W-TD (weatherized top and dead bolt) ■ ECL-230X-W-TDB (weatherized top bolt, dead bolt, and bottom bolt)
Additional benefits include: ■ Corrosion-resistant alloy lock body ■ Surface/flush reversible strike ■ Durable photo-luminescent sign absorbs light, then “glows in the dark” when lights are dimmed ■ Non-handed ■ Resetting the alarm can only be accomplished with a control key ■ Locking and unlocking the dead bolt always arms and disarms the alarm ■ Accepts 5-pin through 7-pin standard and interchangeable core rim cylinders without using a cylinder collar ■ Optional inside pull handle
Almost all hardware and accessories can be customized with different finishes, colors, sizes, and more. Ask us how this new generation of life-safety and security hardware can make a powerful difference at your back door.
For more than a century, Detex has earned the trust of architects and owners who rely on Detex products for the life safety and security of people and property. A USA company, Detex designs, manufactures, markets, and ships products from New Braunfels, Texas. Detex is known internationally for life-safety and security-door hardware, loss prevention and architectural hardware, integrated door-security systems, and guard tour verification.
Visit Detex’s website at detex.com or call us at 800-729-3839.
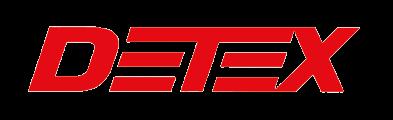
Machine Learning and the Evolution of Exception Reporting
Recent years have seen an explosion of consumer products that incorporate artificial intelligence (AI). Self-driving cars, Alexa, Siri, chatbots, Google search, and smart traffic lights are examples of how artificial intelligence is impacting the world. While artificial intelligence has gained mainstream attention recently, AI applications have been “hidden” for many years in a variety of business applications, such as credit scores, credit card fraud detection, direct marketing, and more.
Retail loss prevention has also seen applications of AI in technologies such as facial recognition and return fraud authorization. In this article, we extend the loss prevention professionals’ toolkit and show the results of a machine-learning algorithm (a specific type of AI) developed to detect fraudulent and abusive employee activity.
The ultimate objective for developing machine-learning models is to obtain collective intelligence to curb employee deviance. Appriss Retail serves more than ninety retailers worldwide, and this experience provides a solid foundation for such model development.
From Rules and Queries to Predictive Models and Machine Learning
Today, most loss prevention departments use traditional exception-based reporting (EBR) tools to monitor their employees by running queries on point-of-sale data that flags suspicious activities. EBR tools used by retailers are similar to the tools used by the credit card industry in the 1980s to detect fraud. In the early 1990s, the credit card industry mostly abandoned the EBR approach in favor of methods of detection based on predictive modeling and machine learning. A similar trend was seen with health-care fraud about fifteen years ago. Our prediction is that retail loss prevention will evolve in a manner comparable to credit card fraud detection.
In a traditional EBR process, a query is run to look for anomalous behavior, and its output is provided to an analyst in the form of a list of transactions or employees to investigate. Then the analyst or investigator reviews the exceptions presented and determines, sometimes based on looking at additional information, if the transaction or employee in question warrants further review. Some of the exceptions will result in an action against the employee, such as arrest or termination of employment, which we label as “success” in the flow chart below.
The goal of machine learning is to apply a complex mathematical model to the data and learn, using thousands of variables, the most useful elements in an employee’s transactions for identifying cases that are most likely to result in an action. With a machine-learning model, the top-ranked exceptions will have a much higher rate of success than those that are generated from traditional EBR. Over time, an approach with a higher success rate will make loss prevention departments far more efficient and will decrease employee fraud rates.
Query Based Investigations Machine Learning Based Investigations
– Department Store Divisional VP of Loss Prevention
EBR Query on POS Data Investigation/ Review of Exceptions Success
Found Something to Correct/Termination
Failure Found Nothing to Correct
Machine learning exploits the differences between successes and failures
Overview of Artificial Intelligence and Machine Learning
Artificial intelligence can cover a wide range of tasks. The next diagram illustrates some frequently used components of AI.
Predictive Models and Machine Learning. Predictive models and machine learning are shown in the diagram as almost completely overlapping circles and are very close in definition and purpose. The primary difference between the two is machine learning originated in computer science, whereas predictive modeling was developed in the field of statistics. In the past decade or more, the two fields have essentially merged and now share a highly overlapping definition. Both are concerned with using data to predict or model outcomes, and both are tools used in AI.
Deep Learning. Deep learning is a process that uses highly complex model structures to learn patterns in the raw data without any human intervention, thus eliminating the need for derived variables. The difficulty in deep learning is that the more complex models are often more difficult to fit accurately.
Dynamic Learning. There are two very different types of model categories: static models and dynamic models. A static model is fit to a static pattern in data. The predictions from a static model can change as the data it is presented with changes, but if the model is presented with the same exact data, it will produce the same exact answer. By contrast, a dynamic model’s prediction can change from day to day even if it is presented with the same information. Dynamic learning is a process by which models are refit or updated dynamically as new data presents itself. This approach is useful when a system is continually bombarded with additional information and the relationships in the data are dynamic and the model itself needs to change to adapt.
Artificial Intelligence
Predictive Models
Machine Learning
Deep Learning Self Driving Cars, Voice Recognition, Text Recognition
Dynamic Learning
Collective Intelligence
Machine-learning models allow developers to use data from many retailers to create a more powerful solution, and Appriss Retail’s worldwide retailer base is a significant benefit. Each retailer is unique in the exception-based approaches and investigation techniques that result in employee terminations and prosecutions. Combining retailer strategies leads to better detection, including: ■ Variables resulting from shared questions ■ Models built on many successful strategies
Using Dynamic Learning and Feedback
Beyond the collective-intelligence model described in the prior section, a final objective of an AI-based employee-deviance detection system is a feedback loop from the investigations back into the models. During an investigation, the investigator will perform many actions that can be captured in a system and later used to improve system accuracy. For example, the investigator will click on related transactions and individuals, will open a case, will abandon a case or a transaction, and so on. The captured usage activity can be converted into information that a machine-learning model can utilize to improve the models. This type of feedback loop ensures that as new fraud schemes are detected at one retailer, that pattern is captured in a broader model that propagates across all retailers.
Employee-Based Variables 3,000+
Known Successful Investigations Across Many Retailers Machine Learning / Predictive Models
Cases to Investigate
Feedback loop from investigations improves models and keeps up with new fraud
The Ultimate Objective
As stated above, the ultimate objective for developing machine learning models is to combine many learnings and obtain collective intelligence to curb suspicious employee behavior. In this application of machine learning, a department store chain saw at least a 25 percent increase in fraud-related terminations above what would normally be captured by EBR alone. Visit apprissretail.com to learn more.
The True Value of Partnership
Perhaps one of the most commonly used yet often misunderstood terms in business today is the word “partnership.” Partnership is a term commonly used to convey a set of mutual expectations in a relationship. It is intended to foster and promote unselfish levels of cooperation and mutual support with the goal of delivering reciprocal value for all parties involved. In a meaningful partnership, each party should feel benefitted by the other and rewarded by a genuine commitment and desire for collective success.
All too frequently, however, the word “partnership” is conveyed as more of a wish versus a mutual commitment—a term used in a one-sided manner to solicit a spirit of cooperation yet producing a selfish and one-sided outcome. Needless to say, partnerships of this kind typically result in abusive business relationships and are often short lived.
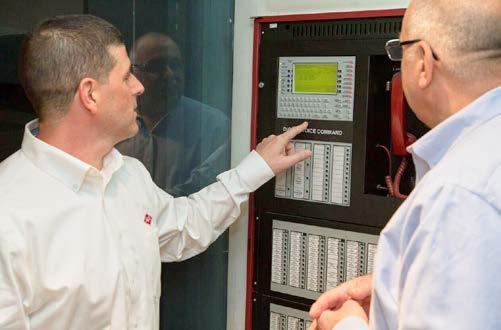
At AFA, the Word “Partnership” Means More
It carries a more profound and special meaning and has done so for nearly 150 years. It is not a wish or a goal but the foundation by which we interact with every one of our valued customers, every minute of every day. When it comes to life safety and asset protection, partnerships matter—partnerships that value the importance of delivering practical and cost-effective solutions, creating a safe environment for employees and customers while ensuring valuable merchandise and assets are kept properly protected and secure. These types of partnerships encourage open and honest communication and include a thoughtful willingness to listen and challenge each other to achieve optimum levels of business results. In addition, these partnerships carry a sense of urgency when responding to all matters regardless of perceived importance. These partnerships result in long lasting relationships.
AFA, a True Business Partner You Can Count on
Thoughtful and Innovative Fire and Security Solutions. At AFA, we find the right technology, best approach, and solutions to help you ensure your most valuable assets are fully protected.
Exceptional Service, Responsiveness, and Attention to Detail. We take pride in our tireless attention to detail and follow up with our clients and partners. We deliver exceptional service with prompt and forthright response, so you can have confidence that your people and assets are protected.
A Heritage of Experience and History of Meaningful Client Partnerships. AFA’s heritage as the nation’s first central-station fire-alarm company is a great source of pride for us. What’s most important are the long-term business relationships we have built with our clients, partners, and employees over the decades. History and relationships matter. It’s the difference between being a number in a computer versus having a long-time personal contact you can take at their word. It’s the stability and reliability that comes with nearly 150 years of experience protecting and serving our clients.
Visit afap.com to learn more.
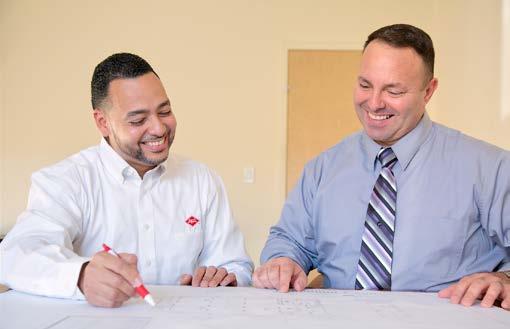
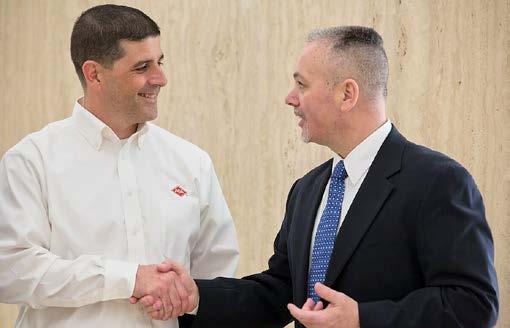
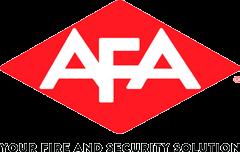