
6 minute read
Using Machine Learning to Predict Surgical Site Infections

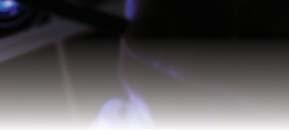
Results of Two Studies Conflict on Generalizability of Algorithms

By MONICA J. SMITH
While most health systems are at the beginning of the journey in using artificial intelligence and machine learning to predict surgical complications, surgeons at the forefront of this science are expanding our knowledge by investigating ways to overcome the obstacles to AI, as described in two recently published studies.
“These articles pose different questions, but what they have in common is that they’re both examining the challenges of bringing AI to bear on the problem of surgical site infection,” commented Philip S. Barie, MD, MBA, a professor emeritus of surgery at Weill Cornell Medicine, in New York City, and the executive director of the Surgical Infection Society Foundation for Education and Research.
“Studies of SSI prevalence are challenging to perform and interpret if not done prospectively, using trained observers inspecting each incision,” Dr. Barie told OR Management News. “Retrospective studies always leave doubt as to what exactly was observed, whether patients were omitted inadvertently because of sporadic reporting from the outpatient setting, or if data reporting is incomplete. Moreover, thousands of patients are required to achieve adequate statistical power to study clean operations owing to the low prevalence of infection.”
One study, conducted by researchers at Mayo Clinic in Rochester, Minn., addressed the problem of missing data, which can skew retrospective analyses and subsequent prospective predictions of SSIs.
“The nice thing about machine learning is that it allows the system to refine a model as it evolves, as long as you can get data for the system to look at; we wanted to know what the impact of missing data is on the ability to model infections,” said Robert Cima, MD, a professor of surgery at Mayo Clinic College of Medicine and Science, in Rochester, Minn.
“What we found is that unless you do certain corrections, your model is going to suffer from it.”
To evaluate a method for handling missing data, Dr. Cima and his colleagues compared a Bayesian-Probit regression model with multiple imputation (BPMI) with a generalized linear model
(GLM) in predicting colorectal deep organ-space SSIs (C-OSIs, e.g., postoperative intraabdominal abscess). Among the 2,376 elective colorectal resections performed at Mayo Clinic between 2006 and 2014, the C-OSI rate was 4.6% (108). The BPMI model identified 57 of these patients: a sensitivity of 56%, compared with the GLM’s sensitivity of 47%. The BPMI model lost its advantage when the model was built to use extra-institutional data (i.e., based on the American College of Surgeons National Surgical Quality Improvement Program), which reduced its sensitivity to 47%. They concluded that for optimal performance, the BPMI model should be built using “data specific to the individual institution” (Surg Infect 2021;22[5]:523-541). ‘My concern is that somebody will “We’re going to be seeing more develop a big model based on a very and more of these models, and people need to understand the limitaheterogeneous data set that may not tions of them, and how to use them reflect the risk profile or the patient in their institution,” Dr. Cima said. “My concern is that somebody profile of an individual hospital. I’d hate will develop a big model based on to see them penalized or made to look a very heterogeneous data set that may not reflect the risk profile or the like they’re not performing well when patient profile of an individual hosthe model was never designed to be pital. I’d hate to see them penalized or made to look like they’re not perused in their environment.’ forming well when the model was —Robert Cima, MD never designed to be used in their environment,” Dr. Cima said. Because retrospective chart review is cumbersome, other investigators have sought to automate the process using machine learning and natural-language processing. The other study, which specifically investigated the generalizability of SSI-detection machine learning–generated algorithms, found that machine learning models designed at one center worked just as well at another. “We’re at the beginning of an acceleration of having machine learning and AI used more widely in health care, but the work to validate models isn’t always done optimally. In many instances, we expect it to be like a ‘plug-and-play’ technology, where you install the solution in and it works. But the truth is, in some cases there is a degradation in performance or the need for more optimization,” said Genevieve Melton-Meaux, MD, PhD, a professor of surgery and Institute for Health Informatics core faculty at the University of Minnesota Medical School, in Minneapolis. continued on page 13
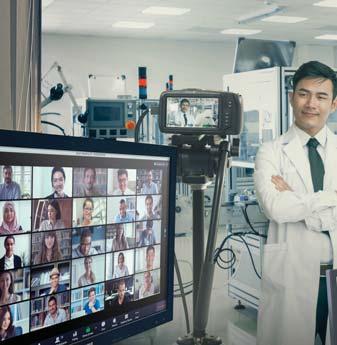
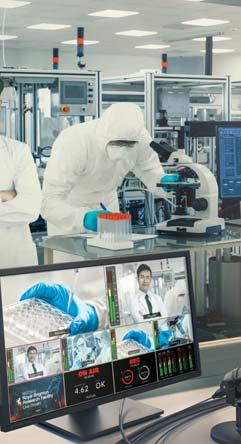
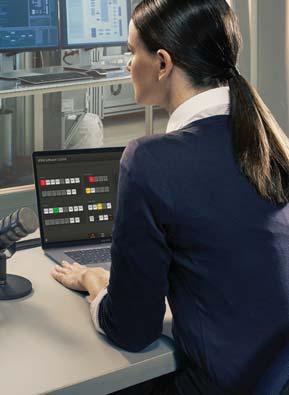
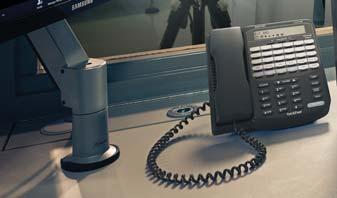
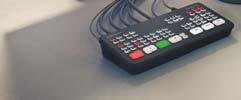
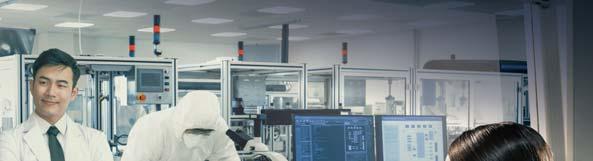
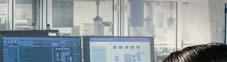
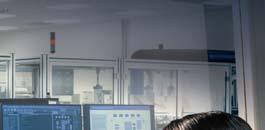
Introducing ATEM Mini Pro
The compact television studio that lets you create presentation videos and live streams!
Blackmagic Design is a leader in video for the medical industry, and now you can create your own streaming videos with ATEM Mini. Simply connect up to 4 HDMI cameras, computers or even technical equipment. Then push the buttons on the panel to switch video sources just like a professional broadcaster! You can even add titles,picture in picture overlays and mix audio! Then live stream to Zoom, Skype or YouTube!
Create Training and Educational Videos
ATEM Mini’s includes everything you need. All the buttons are positioned on the front panel so it’s very easy to learn. There are 4 HDMI video inputs for connecting cameras and computers, plus a USB output that looks like a webcam so you can connect to Zoom or Skype. ATEM Software Control for Mac and PC is also included, which allows access to more advanced “broadcast” features!
Use Professional Video Effects
ATEM Mini is really a professional broadcast switcher used by television stations. This means it has professional effects such as a DVE for picture in picture effects commonly used for commentating over a computer slide show. There are titles for presenter names, wipe effects for transitioning between sources and a green screen keyer for replacing backgrounds with graphics! Live Stream Training and Conferences
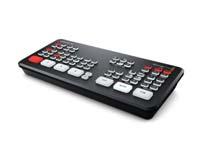
The ATEM Mini Pro model has a built in hardware streaming engine for live streaming via its ethernet connection. This means you can live stream to YouTube, Facebook and Twitch in much better quality and with perfectly smooth motion. You can even connect a hard disk or flash storage to the USB connection and record your stream for upload later!
Monitor all Video Inputs!
With so many cameras, computers and effects, things can get busy fast! The ATEM Mini Pro model features a “multiview” that lets you see all cameras, titles and program, plus streaming and recording status all on a single TV or monitor. There are even tally indicators to show when a camera is on air! Only ATEM Mini is a true professional television studio in a small compact design!
ATEM Mini ..........$295 ATEM Mini Pro..........$495 ATEM Mini Pro ISO..........$795