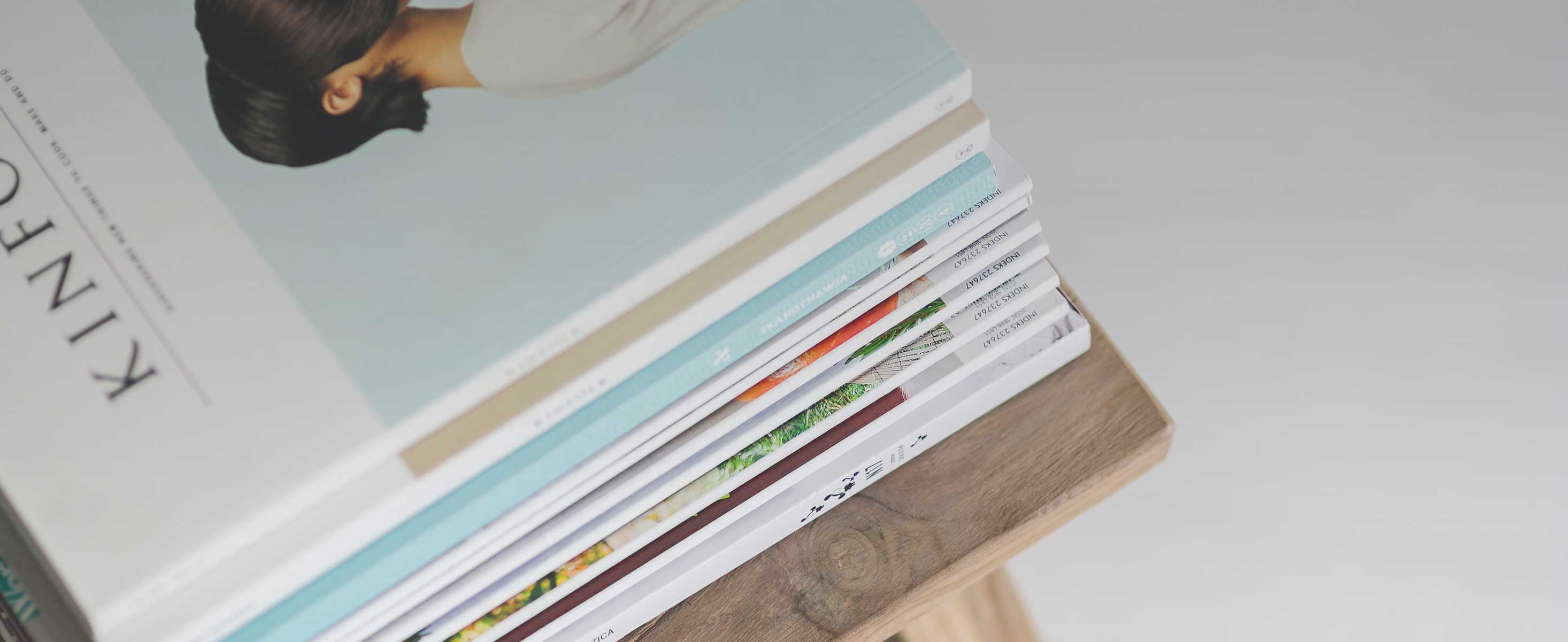
3 minute read
PACS/IT
BY MARK WATTS
A CODE OF ETHICS FOR ARTIFICIAL INTELLIGENCE AND IMAGING
iwas reviewing a portable X-ray contract last week when the salesperson sent an addendum that listed an add-on for $14,870. I picked up the phone and called the salesperson.
I asked, “What does this add-on do?” The reply was, “It diagnoses a pneumothorax.”
I did not order the add-on.
If there is a positive finding, 1 in 1000 chance, who and how will a notification be sent out to act? Is it ethical to sell a product like this knowing that medical decisions can be made from this unreviewed “diagnosis.”
Of all the industries romanticizing artificial intelligence (AI), health care organizations may be the most smitten. Hospital executives hope AI will one day perform health care administrative tasks such as scheduling appointments, entering disease severity codes, managing patient lab tests, managing referrals, and remotely monitoring and responding to the needs of an entire cohort of patients as they go through their daily lives.
By improving efficiency, safety and access, AI might be an enormous benefit to the health care industry.
But caveat emptor, buyers of health care AI need to consider not only whether an AI model will reliably provide the correct output – which has been the primary focus of AI researchers – but also whether it is the right model for the task at hand. I believe we need to think beyond the model.
This means executives should consider the complex interplay between an AI system, the actions that it will guide and the net benefits of using AI compared with not using it. This should be done before executives bring any AI system on board. They should have a clear data strategy, a means of testing the AI system before buying it and a clear set of metrics for evaluating whether the AI system will achieve the goals the organization has set for it.
Here is the simple rule of thumb, “In deployment, AI ought to be better, faster, safer and cheaper. Otherwise, it is useless!”
I have been involved with some AI vendors. I was pleasantly surprised to find how efficient their models could be trained. I was reviewing the results with a data scientist. As we discussed a CT AI algorithm, I asked the scientist, “How long have you tested this product?” The reply was 18 months. I next asked, “What were the protocols utilized to generate the data that was consumed by the algorithm?” The engineer stated, “One-millimeter slices overlapping at 0.75 millimeters provided the outcomes.” This is a key point – an AI engineer does not understand ALARA.
Each CT chest, abdomen and pelvis exam would be 10,000 slices. This is not how the health care world works. The software engineer said he is not responsible for the performance of the algorithm if I did not give it the right datasets.
This reminded me of a professor at the University of Texas that I met at a Society for Imaging Informatics in Medicine (SIIM) conference about 15 years ago. He said, “I can train a robot to mow the lawn. I can also train a robot to change a baby’s diaper. What my robot will never be able to do is care if it is changing the lawn or mowing the baby.”
To them, it is a mathematical exercise to produce the most favorable output. It is up to us – as the consumers of artificial intelligence in imaging – to ask the right questions.
A salesperson will tell you about the wonderful things AI can do if you use it the way it was designed by the engineers of the world. A salesperson wants to make a sale. It is up to the leadership in imaging to safely deploy well understood and real-world tested AI in imaging. •
Mark Watts is an experienced imaging professional who founded an AI company called Zenlike.ai.