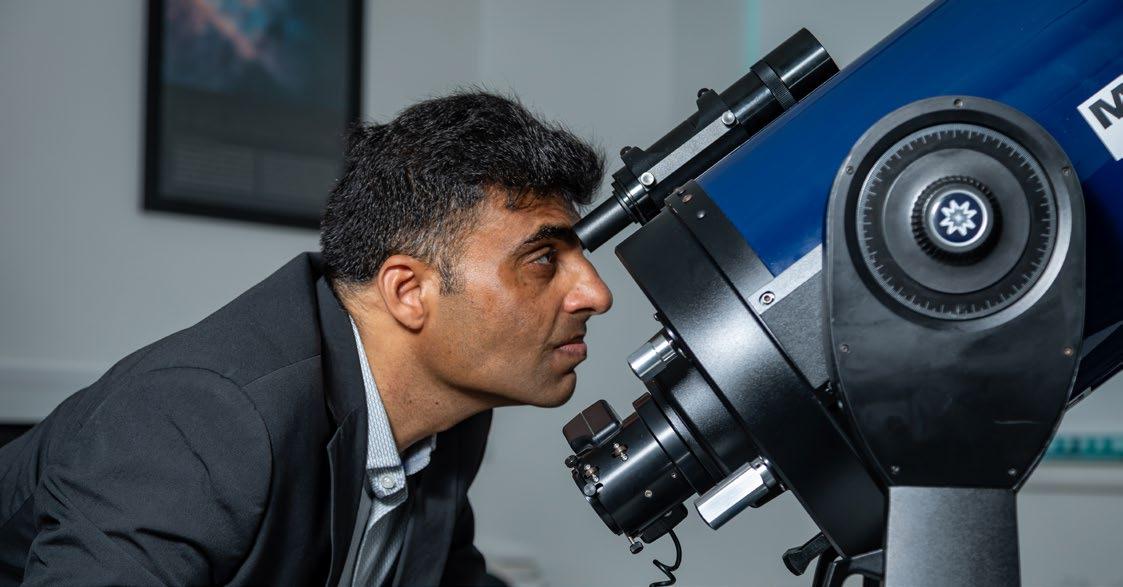
11 minute read
UAE STUDY HELPS SPACE EXPLORERS DETECT GALAXIES 37 BILLION LIGHT YEARS AWAY FROM EARTH
from The 8th Issue of the Innovation@UAE Magazine: Space is now closer with groundbreaking UAE research
The first documented records for systematic astronomical observations dated back to the Assyro-Babylonians around 1000 BCE from this cradle of civilization in Mesopotamia, in the southern part of present-day Iraq. At the time, monitoring the motions of stars and planets in the sky was the best tool to track time, which was fundamental for agriculture, religious rituals and navigation.
In the Dark ages, astronomy flourished in Asia and in the Islamic world. Extensive observations were performed in the Chinese and Indian empires, including the compilation of stellar catalogs. In the Islamic world, observations of the sky were accompanied by the study and translation of texts from ancient Greek scientists.
Advertisement
Islamic scholars built exquisite astronomical instruments to measure angles in the sky. Hence, it is no surprise that Muhammad Abdul Latif, Associate Professor, at the Department of Physics, United Arab Emirates University, is studying Population II and III stars and how and where to find them in the galaxies. All this is unveiled in the study entitled
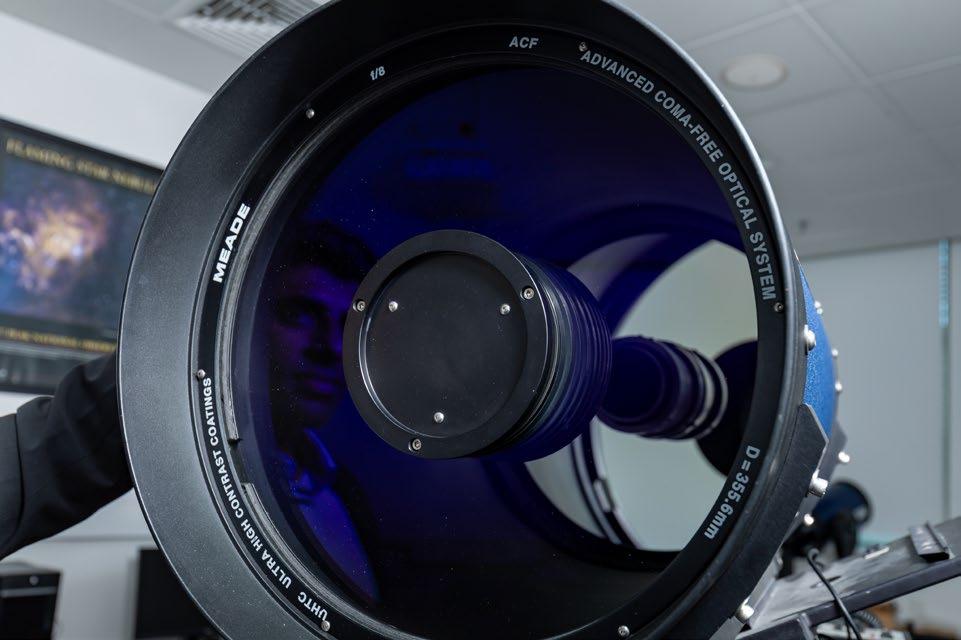
“Unveiling the Contribution of Population III Stars in Primeval Galaxies at Redshift 6”.
Importance of Population III and II stars
Abdul Latif explains to Innovation@UAE magazine what these stars are and why they are so important to study.
According to Abdul Latif, “Population III stars are the very first generation of stars that formed in the universe and brought the first light into the cosmos while Population II stars are the second-generation stars which were formed from the material synthesized within Pop III stars. In simple terms, Pop III and Pop II stars are the parents and grandparents of our sun. Our sun is a Pop I star. Pop II and Pop III stars were short lived like dinosaurs being big and massive compared to our Sun.
The importance of studying these stars is that they give us insight into how stars and galaxies were formed within the first billion years after the Bing Bang. The Big Bang Theory says the universe started with an infinitely hot and dense state that inflated and stretched, first at unimaginable speeds, and then at a more measurable rate over the next 13.7 billion years to the still-expanding cosmos that we know today.
Abdul Latif explains, “These stars tell us how heavy elements were produced in the universe (elements heavier than helium, in astronomy we call them metals). They also emitted plenty of radiation and led to the re-ionization of the universe.”
The research
The research carried out studies on how the JWST observations can detect the first stars, the Population III stars. JWST is the James Webb Space Telescope; the biggest space telescope ever launched which can detect/observe infrared light. One of the prime objectives of this mission is to detect primeval galaxies, the host of Population III and Population II stars. These observations will shed light on the formation and evolution of the first stars and first galaxies.
Previous studies have not investigated the entire possible range of halo masses and redshifts that may help in their detection. Motivated by the prospects of detecting galaxies up to z ∼ 20 ( Z scores tell you how many standard deviations from the mean each value lies. Converting a normal distribution into a z-distribution allows you to calculate the probability of certain values occurring and to compare different data sets) in the JWST early data release, the research quantifies the contribution of Population III stars to high-redshift galaxies from 6< z<30 About thousand galaxies have been detected at z > 6 (Bouwens et al. 2016; Oesch et al. 2016; Finkelstein et al. 2022; Harikane et al. 2022; Schaerer et al. 2022) with candidates up to z ∼ 20 being revealed in James Webb Space Telescope (JWST). The research results were rendered by employing the semianalytical model A-SLOTH, which self-consistently models the formation of Population III and Population II stars along with their feedback.
Abdul Latif explains that A-SLOTH is one of the most complete models which includes all the relevant astrophysical processes required to study the formation of Population III and II stars as well as to make solid predictions for JWST for the large sample of statistically significant galaxies. He adds,” A-SLOTH is computationally more efficient compared with similar models, a publicly available model and the only model which traces the formation of individual stars. It has been calibrated with observations of local galaxies.”
Results
The research results suggest that the contribution of Population III stars is the highest in low-mass halos of 107 –109 times the mass of our Sun. While high-mass halos of
1010 solar masses contain less than 1% Population III stars, they host galaxies with stellar masses of 109 solar masses as early as z ∼ 30. Overall, the apparent magnitude of Population III stars gets brighter toward higher redshift due to the higher stellar masses, but Population III dominated galaxies are too faint to be directly detected with JWST. The research results predict JWST can detect galaxies up to z ∼ 30, which may help in constraining the initial mass function of Population III stars.
Hence, what does this mean for humanity and space exploration? Abdul Latif states, “Our results suggest that the best candidates for searching for Population III stars are low-mass galaxies from 10< z <30, which are challenging to detect with JWST, and the contribution of Population III stars decreases to less than 1% in massive galaxies. We further predict that JWST can detect galaxies up to z ∼ 30 as their AB magnitudes (The AB magnitude system is an astronomical magnitude system. Unlike many other magnitude systems, it is based on flux measurements that are calibrated in absolute units, namely spectral flux densities) which lie within its range. These findings may guide observers in planning their observations and help to improve spectral modeling of highredshift galaxies.” High redshift galaxies mean the galaxies which are the most distant ones, billions of light years away from Earth. They were the first galaxies to form in the universe after the Big Bang.
Importance for UAE space exploration
The study of the farthest galaxies ever formed is extremely important for space exploration in general and the UAE’s space exploration in particular. Abdul Latif explains, “We predict that JWST can detect galaxies about 37 billion light years away from us and these galaxies were formed only about 100 million years after the Big Bang. This is unprecedented in terms of space exploration as we have never been able to detect such distant galaxies. Thanks to advancements in technology and human endeavors, we are now in a position to do that. Our work will guide the scientists working with JWST to plan their observations and what to expect in terms of properties of such objects.”
By studying this unique sample of galaxies, researchers will be able to study the statistical variations among the properties of high-redshift galaxies, such as stellar masses, luminosities, star formation rates, fraction, and contribution of Population III stars to their host galaxies. Abdul Latif concludes, “I often tell my students that JWST is a time machine which allows us to look back in time by observing distant objects. Therefore, basically, we will be looking back in the past of our universe, how it looked like when the universe was in its childhood.”
Definition of Population I, II, III stars
Astronomers grouped stars in the order they were observed, so Pop I stars are present-day stars, with Pop II stars being one generation older. Pop III stars are the hypothesized oldest stars in existence.
UTILIZING AI AND MACHINE LEARNING, THE UNIVERSITY OF SHARJAH IMPROVED DETECTION OF RADIO SOLAR BURSTS AND METEORS IN UAE
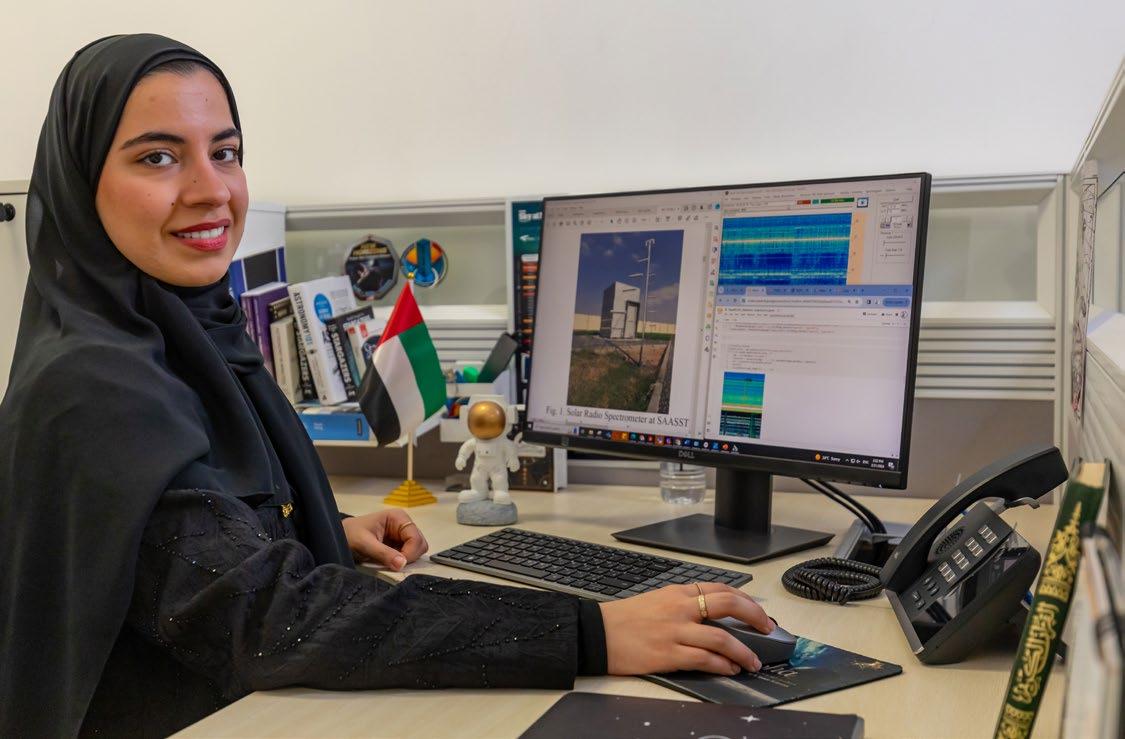
Two studies aim to make the detection of both solar bursts and meteors more efficient, accurate and effective using technologies such as Artificial Intelligence (AI), and Deep Learning, a branch of Machine Learning.
Aisha Alowais, a research analyst at the Sharjah Academy for Astronomy, Space Sciences and Technology (SAASST) of the University of Sharjah (UoS), worked on both researches, one entitled; Classification of Incoming Radio Data from a Solar Radio Spectrometer and the other entitled; Meteor detection and localization using YOLOv3 and YOLOv4.
Aisha Alowais works in the Space Artificial Intelligence Laboratory, where her focus is directed toward developing machine learning algorithms in space and astronomy-related projects. Among the laboratories she collaborates with is the Radio Astronomy Laboratory, which conducts studies concerning observing radio solar bursts to understand the behavior of the sun in the field of solar physics. Along with her team, Aisha developed a machine learning algorithm for radio solar bursts detection and classification.
Space research involves dealing with vast amounts of data, much like the work we do at the Sharjah Academy for Astronomy, Space Sciences, and Technology (SAASST).
The first study looked into the phenomena of solar bursts which are generated through the acceleration of energetic electrons during solar eruptions such as flares and Corona Mass Ejections (CMEs).
These bursts offer crucial information about the Sun›s behavior, magnetic fields, and underlying processes. They also provide insights into fundamental plasma physics and space weather.
In addition, studying them helps scientists to predict potential hazards such as geomagnetic storms and radio communication failure.
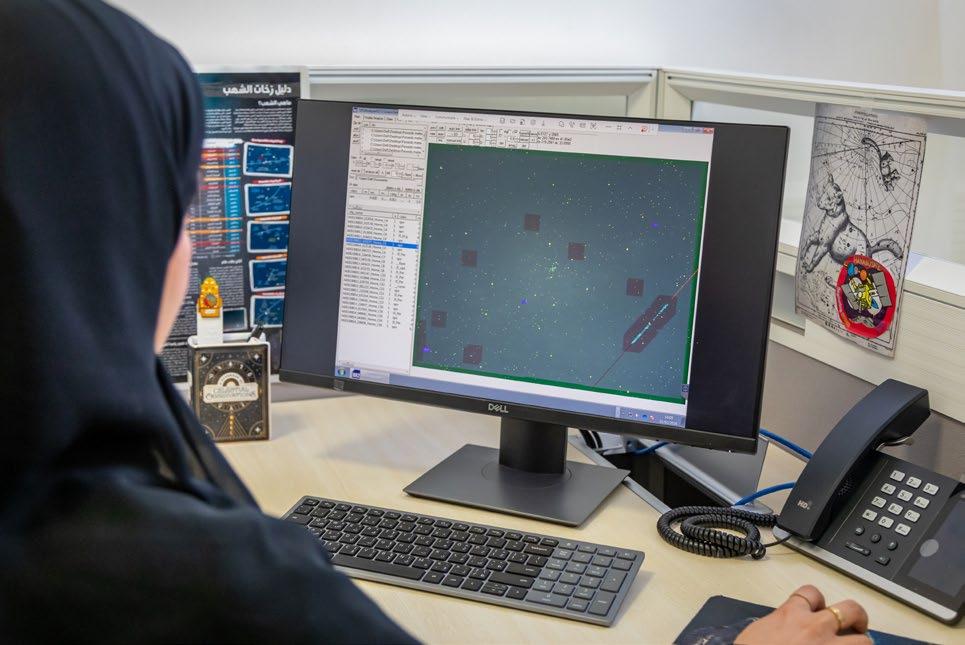
The Challenge
There are challenges with studying solar bursts, one of which is detecting them in radio spectrometers. Radio frequency interference and other observation equipment factors are challenges. One of the biggest challenges is noise interference which overshadows the actual detection of solar bursts.
Consequently, operators must spend considerable time filtering out true data from false data, impacting efficiency. Early research efforts utilized image processing techniques such as the Radon and Hough transforms to automatically detect Type II and III solar burst events, respectively.
Alowais explains, “Space research involves dealing with vast amounts of data, much like the work we do at the Sharjah Academy for Astronomy, Space Sciences, and Technology (SAASST). Our antennas, designed to capture radio waves from the sun, the Milky Way, and Jupiter, also pick up a lot of terrestrial noise in the radio background. Upon converting them to a digital format, the background noise including the phenomena of interest, the solar bursts, are together displayed on a spectrogram, needing to be processed. This is where technology, especially Artificial Intelligence (AI), plays a vital role in making our research more efficient.”
She adds, “For instance, AI helps us automatically detect and identify radio bursts amidst all the noise, significantly streamlining the process.
When trying to detect solar bursts into two classes, the researchers have divided the dataset into two classes, either “event” which represents a solar burst, or “No Event” representing noise.
The Solar Burst study
In 2022, the total number of solar burst observations was 32, which is considered a small number for training machine learning algorithms effectively, so Alowais and the team employed image processing techniques and augmentation to address these limitations and expand the dataset.
The team utilized deep learning; a sophisticated area of machine learning inspired by the structure of the human brain. It uses layers of algorithms called neural networks to process data in complex ways. In our solar burst research, we applied deep learning to quickly analyze vast amounts of data, identifying solar bursts and classifying them into their respective types.
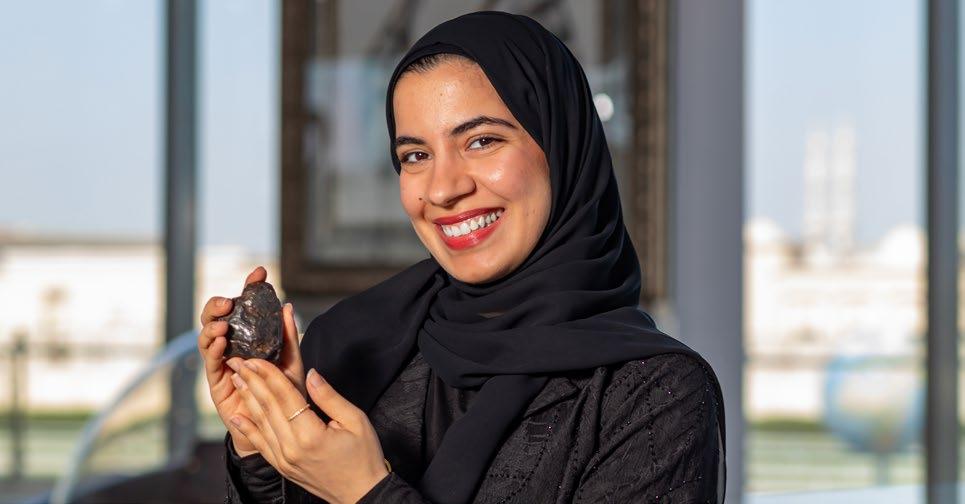
Two models were developed. Each model was evaluated separately.
For the Event Detector with LeNet-5, the model achieved performance with an accuracy of 0.96, indicating that it accurately classified whether a solar burst event existed in an image. The loss value of 0.06 was relatively low, suggesting that the model was well-tuned and effectively minimized errors during training.
For the second model with VGG-16, it demonstrated higher performance levels. It achieved an accuracy of 0.99, indicating its proficiency in correctly identifying the specific types of solar bursts present in the images. The loss value of 0.03 was remarkably low, implying that the model›s predictions closely align with the ground truth labels.
The Findings of Solar Burst Study
The findings in this study hold promising implications for both solar radio burst research and the broader field of deep learning applications. The developed models demonstrated their potential in enhancing the automated detection and categorization of solar radio burst events, a crucial task in space weather monitoring.
Given that the method of capturing data is labor-intensive, such automation processes are in high demand. In line with this, such studies will advance the understanding of solar radio bursts, ultimately benefiting space weather monitoring and prediction systems.
The study also laid the groundwork for future endeavors in refining the models, expanding the dataset, and exploring additional regularization methods.
Meteor Study
In the same respect, meteors in the UAE are observed daily using the U.A.E. Meteor Monitoring Network (UAEMMN).
By September 2022 for example more than 40,000 meteors were observed. However, due to the high sensitivity of the network, it also captured non-meteor objects such as airplanes, birds, insects, and space debris in the atmosphere.
Consequently, to accurately identify and label meteors, the study employed object detection algorithms to reduce data and accurately detect meteors and non-meteor objects.
For the research on meteor detection Alowais and the team utilized The YOLOv3 and YOLOv4 object detection algorithms, utilizing convolutional neural networks. The models were trained on both an imbalanced and a balanced dataset that consisted of thousands of images.
Alowais states, “YOLOv3 and YOLOv4 are versions of an algorithm designed for real-time object detection. YOLOv4 is an improved version of YOLOv3, featuring enhancements that increase its accuracy and detection speed. We utilized both to evaluate the impact of these improvements on our research. The results of our study show that each surpasses the other in certain aspects.”
The imbalanced YOLOv4 model yielded the highest recall score of 98.5% followed by the imbalanced YOLOv3 model with a recall score of 98%. The highest accuracy result was also achieved by the imbalanced YOLOv4 model, with a score of 90%. Overall, all the four models were successful at labeling meteors with a confidence of more than 95%.
The study represented a significant contribution to the field of meteor-related image analysis using low-cost cameras and machine learning. It had promising implications for further research and development in this area.
The researchers trained a machine learning model (ML) with thousands of images from the (UAEMMN), featuring both meteors and non-meteors. This training enabled the model to distinguish between these objects accurately. The ML model employed deep learning techniques and focused on object detection, a process that recognizes and pinpoints the location of objects within an image. This approach significantly enhanced the precision of the meteor detection efforts.
These deep learning frameworks could detect, locate, and label meteors within an image by generating bounding boxes. The use of this software would streamline the process of separating meteor images captured by the UAEMMN cameras from other data.
The results of the study demonstrated that both models effectively classify and locate meteors and not meteors in images. One key advantage of the proposed models is the high accuracy in labeling objects of both classes, achieving a correct prediction rate of up to 90% and higher.
Another advantage was the fast prediction time, with the models being capable of detecting objects in hundreds of images in a matter of minutes. Although the validation results were lower than those of other studies, the testing results indicated that YOLOv3 and YOLOv4 are highly suitable models for meteor detection and localization.
The impact on UAE Space Exploration
The first research has led to the development of an automated system for detecting radio solar bursts. Alowais states, “This system sends real-time alerts to researchers. This advancement can enhance global radio solar burst monitoring and significantly benefit the UAE›s space exploration efforts by providing crucial data more efficiently.”
In terms of the meteor detection study, Alowais believes that the research enhances global meteor detection and supports the UAE space program because it extends the capabilities of the UAEMNN by applying these methods to other data driven projects.
She explains, “We are educating future scientists by incorporating this advanced technology into student training programs. This dual approach advances our algorithm- development capabilities and contributes to positioning the UAE as an active participant in space exploration and research.”
Alowais, believes that AI, machine learning, and deep learning are revolutionizing astronomy and space exploration by efficiently processing the vast amounts of data these fields generate. This could facilitate groundbreaking discoveries, advance the understanding of the universe, and enhance space exploration capabilities.
She comments, “By automating routine tasks, researchers can focus more on innovation, driving forward the development of new algorithms and technologies. SAASST is committed to nurturing the next generation of scientists and researchers by hosting interns annually in a specialized 8-week summer program. This program is designed to equip students with the skills and knowledge they need to excel in space exploration and research, offering hands-on experience with the latest technologies and methodologies, including AI, machine learning, and deep learning.”