
7 minute read
Digital twins: the next frontier in asset management
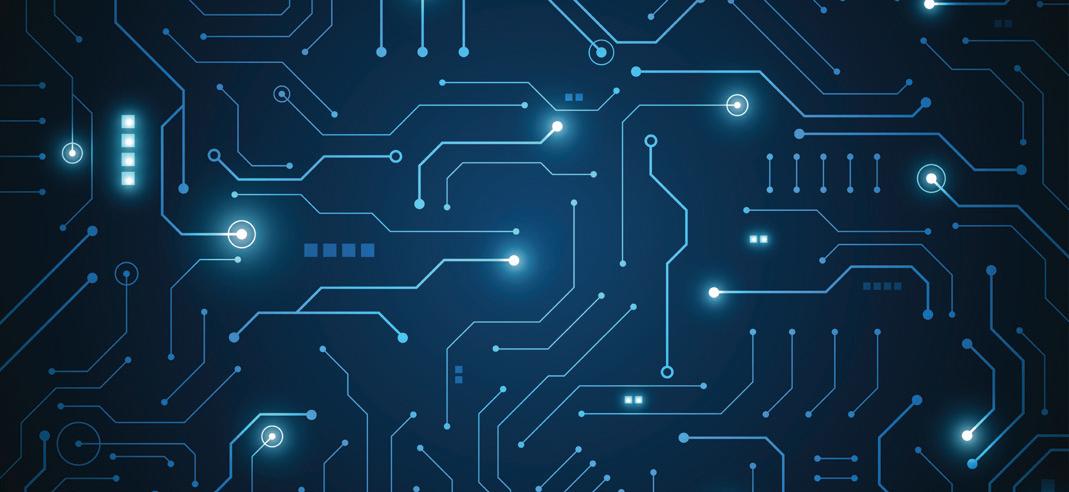
By Angelo Fiumara, Digital Asset Integration Manager, Essential Energy
Advertisement
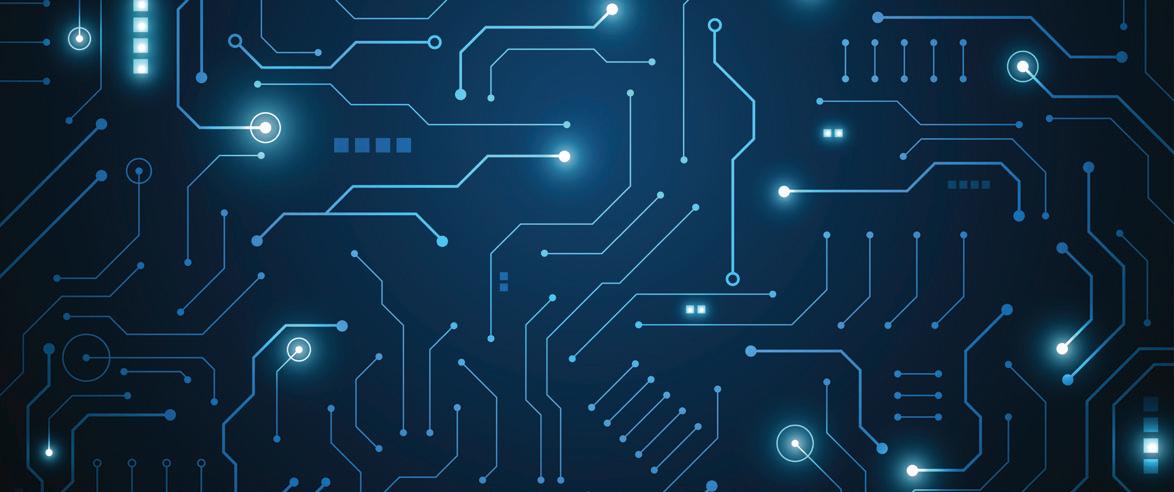
Essential Energy is an electricity distributor (sometimes referred to as Distribution Network Service Provider or DNSP) responsible for operating and maintaining the electricity distribution network supplying 95 per cent of NSW and parts of southern Queensland. The business is utilising a digital twin to uplift its asset management and operational practices, unlocking ways of working not previously possible. By building a digital model of the network and its surroundings, many insights that were impractical due to the laborious and manual calculations involved, are now easily within reach, because assets can be accurately modelled to replicate how they will perform in reality and interact with the real world.
Essential Energy has more than 870,000 customers and manages over 183,000km of powerlines. This markedly low ratio of customers to assets means that it is critical that Essential Energy finds ways of working that are not only industry best practice but are also agile enough to continuously improve and deliver long-term value to its customers.
While there are many definitions of digital asset management, at Essential Energy, digital asset management refers to the use of digital data sources to provide asset management insights and operational efficiencies.
It encompasses a multitude of techniques that leverage rich sources of intelligence that, at its most basic level, involves the use of digital imagery to determine what assets exist and what condition they are in.
More advanced applications include the building of an accurate digital twin of the network that is supported by an engineering-grade mechanical and electrical analytics engine to provide ‘whole of network’ insights.
Network-wide risk and resilience assessments as well as the compatibility of the network to connect renewable energy supplies are some of the applications made possible by this modelling capability.
The development of the digital twin that can be applied at a large scale is seen as a core capability required to efficiently manage one of the largest and most geographically dispersed distribution networks in the world.
HARNESSING THE POWER OF BIG DATA FOR DIGITAL TWIN DEVELOPMENT
Essential Energy’s digital twin journey began in 2014, when Essential Energy began carrying out Light Detection and Ranging (LiDAR) scans of its service area for the purposes of compliance reporting.
The acquisition of this data laid the foundations for Essential Energy to be able to enhance its network design function. The LiDAR data, once ingested into a technology solution that is used for network design, enabled the automatic creation of Building Information Model (BIM) objects. These BIM objects replicate the way the network is actually constructed in the real world which creates a 3D digital twin of the ‘as built’ network.
This accurate ‘as built’ record provided by the digital twin had never previously been available. This automatic creation of digital twins for portions of the network has significantly improved the efficiency of the network design process, which has initiated the transition away from 2D construction plans to 3D BIM-based network designs.
The use of auto-generated 3D digital models of the network has also enabled a rapid production of compliant and consistent approach to network designs.
It has also uplifted the skill levels for both network designers and accredited service providers who design new connections based on digital twin objects on behalf of customers connecting to the network.
One of the key components of designing with BIM objects is the implementation of built-in compliance checking. This allows consistent, accurate and fast design reviews for internal and externally produced designs, creating high levels of compliance with Australian Standards and Essential Energy guidelines, and reducing technical certification assessments timeframes by up to 50 per cent.
This network design application of digital twins has in turn provided the foundation for Essential Energy to expand its application to the entire network to support asset management and operations.

THE THREE LAYERS OF DIGITAL ASSET MANAGEMENT CAPABILITY
Essential Energy considers three key layers of capability when considering digital asset management: • Digital data sources • A platform to visualise the digital twin and linked asset data • Applications that use digital data, the digital twin to provide asset management insights
The visualisation platform provides the means by which the digital twin and other digital data and content can be linked and digitally brought to life to demonstrate the network and its surroundings.
This provides a means of associating all asset and contextual information to a digital twin asset and, for such a geographically dispersed network, provides the means of carrying out ‘virtual site visits’ and reducing travel using an accurate digital desktop model
The availability of rich digital datasets, the development of a configurable digital twin and the visualisation platform provides the basis for what Essential Energy sees as the third and most powerful layer in digital asset management capabilities – digital asset management applications.
These applications are targeted at specific asset management needs. The outcomes can then be used to enable other asset management applications and be passed back to the visualisation platform as a record of how the outcome was derived from the digital imagery.
For instance, digital asset imagery combined with the digital model can be used to determine asset attribute and condition information.
Similarly, applications may make use of the digital twin and its configurable mechanical analytics engine to provide insights such as the ‘as built’ conductor tensions and mechanical loading of power poles which can be used to inform decisions relating to pole reinforcement or replacement.
The outcomes provided by these applications can similarly be passed back to the visualisation platform so that asset and operational managers are able to see the basis of what has contributed to the modelled insight.
AN ASSET-BY-ASSET APPROACH TO UNDERSTANDING NETWORK HEALTH AND PERFORMANCE
Some powerful but fundamental value use cases are already apparent for the application of a large-scale digital twin to enhance asset management outcomes.
In the electricity distribution industry, many processes for assessing the serviceability of assets rely on the application of rule-based approaches rather than specific approaches for specific assets. A tailored approach to specific assets is made possible through digital twin modelling.
Using the actual measured mechanical loading of assets in place of assumed values is a key area of potential for a digital twin that has a mechanical ‘analytics engine’ at its heart.
For Essential Energy’s assets, the decision to replace or reinforce timber poles relies heavily on assessing the mechanical loading of poles and the remaining strength required in the pole to safely withstand that loading.
Using the digital twin to determine the actual mechanical loading on power poles can better inform the asset decisionmaking process.
Similarly, mechanical loadings can be derived from the digital twin to impact the shift from reactive to planned maintenance. This shift – when applied to power line components such as poles and cross arms – is made possible by using the digital twin to determine the mechanical loading on each asset.
The mechanical loading on these pole and cross arm assets is determined primarily through the assessment of the impact of wind forces on conductors, meaning that the combination of known conductor tensions, historical weather conditions and asset condition information has significant potential to predict asset failures and assess the overall resilience of the network.
VISIBILITY AND FLEXIBILITY KEY TO A SUSTAINABLE ENERGY FUTURE
The applications of digital twins in electrical distribution goes beyond ‘static’ mechanical modelling. The growth in customer connections of embedded generation and the growing role for the network to manage complex energy flows means that there is an increasing need to understand how its performance and capacity changes throughout the day.
The addition of real-world sensory data to a static digital twin provides the opportunity to determine the network's true capacity to manage highly variable energy flows. Feeding this sensory data into the mechanical analytics engine of a digital twin would provide accurate assessments of electrical ratings.
The addition of an electrical model of the network into the digital twin means that the overall impact of new connections – including intermittent renewable energy sources – can be fully modelled to make maximum use of network capacity.
This future of new and intermittent renewable energy sources connecting to the network is one that must be prepared for so that customers continue to benefit from a network that provides a platform for energy exchange.
As technology improves and becomes more cost efficient, the network needs to be able to safely and reliably manage complex energy flows – something a mature digital twin can support.