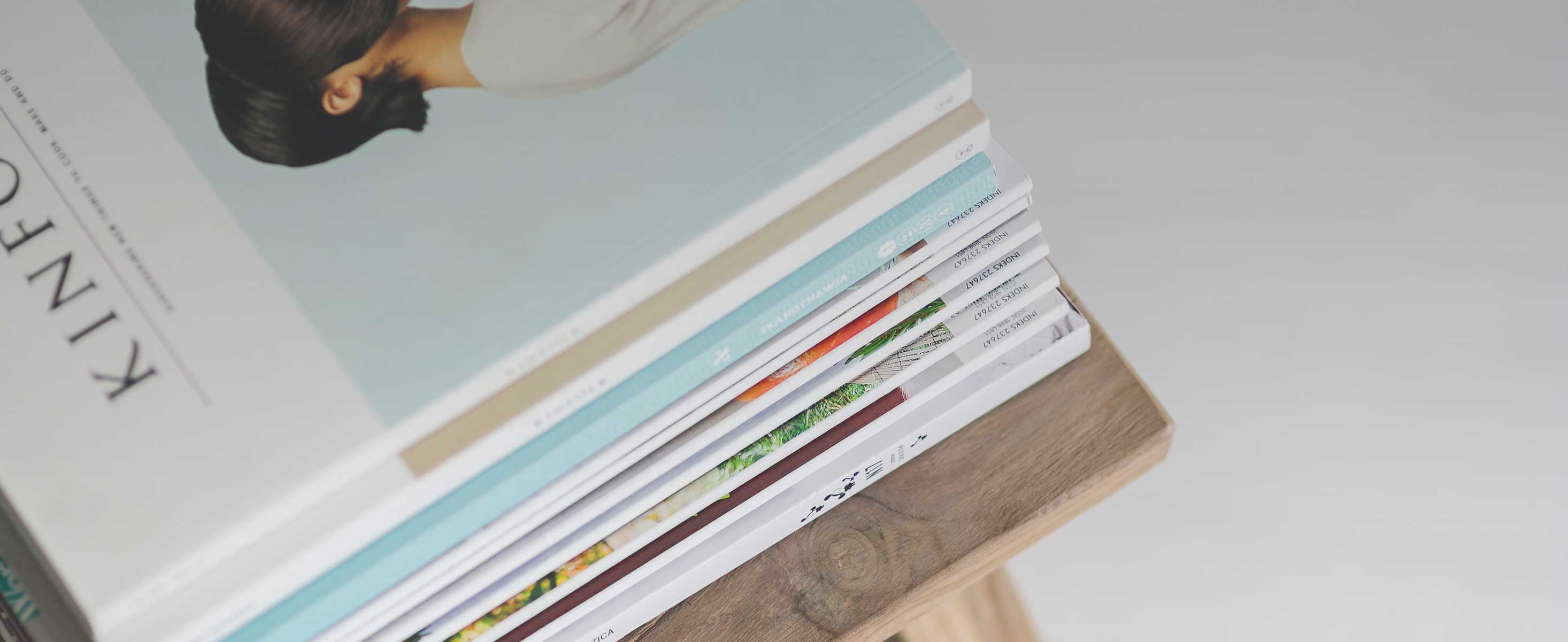
4 minute read
New appointments
A number of new senior members have joined the Optimize team enhancing the fi repower of the business.
Rachel Riley has been appointed brand ambassador to drive market reach and brand recognition. Riley, best known for her role on television’s Countdown as well as 8 out of 10 Cats and other television hits, is a graduate of applied mathematics and understands the crucial role algorithms play in enabling organisations to be more effi cient and to decarbonise. “Optimize has the technology and software in place to deliver a signifi cant impact for fleet operators, supporting operational effi ciency and the journey to a zero carbon society,” Riley explained. “I’m looking forward to working with the Optimize team and playing my part in improving the effi ciency of the sector.”
Advertisement
George Garnett joins the business as Chief of Staff and will support CEO, Colin Ferguson, developing the organisational and operational capacity at Optimize. Yale graduate Garnett brings a powerful skill set to the business to unlock the domestic and international growth potential of the business: “This is a unique time for the company. The combination of high energy prices, unique macroeconomic and geopolitical factors and an increasing emphasis on climate, means we are well placed to execute both for our clients’ operational ef fi ciency, but also to help deliver on the common goal to optimise our processes, cut emissions, and ultimately decarbonise our economy for the next generation.”
Lucy Allen joins the business, as Key Account Director, and will lead the business on account management and customer success. Allen is a proven and experienced senior technical sales lead and brings market knowledge and insights to the business. “The team at Optimize are highly focused and committed to delivering results for the fleet and logistics sector,” she explains. “It is great to be on board with the opportunity to drive growth.”
Dr Ross Conroy joins Optimize as Head of Research & Development after working on the Knowledge Transfer Partnership at Teesside University with Optimize. Conroy is an AI specialist bringing a deep knowledge to the organisation: “I am excited to explore the opportunities for AI in an active market,” he explains, “and I believe this will bring amazing solutions for the fleet transport sector.”
Dr Sarah Bee is the new Strategic Projects Director at Optimize, bringing her wealth of experience from the energy and fleet transport sectors to drive new projects at the company. Bee has worked on a variety of government-backed and customer-led projects. “I can see many new applications for the Optimize services across the fleet sector,” she explains. “We are focused on innovation and transforming our clients’ businesses as they prepare for the new net-zero carbon future.”
Richard Smith has been appointed Product Development Director and brings his vehicle telematics and application engineering knowledge and experience to compliment the senior management team. “This is an inspiring business and I am looking forward to driving the product development roadmap,” he explains. “We are in a new age of innovation across the sector as digital connections offer new solutions to drive operational efficiency – what a time to be alive!”
Artificial intelligence expert Dr Ross Conroy is head of Research and Development at Optimize and has been working on the new algorithm which will drive huge improvements for the fleet transport sector. To get a better understanding of his work and the new services being created, we sent our reporter to explore.
“Artificial Intelligence (AI) and machine learning are the skills I specialise in,” Conroy explains. “There are various approaches used in AI, ranging from traditional techniques like rule-based systems to more advanced techniques like deep learning. However, many existing transportation optimisation products do not qualify as AI, as they rely on trial-and-error methods or heuristics to find a solution.
“In contrast, AI or rather machine learning, is focused on learning from data rather than making assumptions about what it‘s trying to plan for. Our approach to this problem solving is based on data-mining, where the software examines past solutions that worked and uses that information to continuously improve its decision-making. This involves recognising patterns in the data and reapplying solutions when similar problems arise.
“With most machine learning techniques, the more data you‘ve got, the better the predictions. What we‘re doing now means we‘ve got lots of data constantly coming in, and the idea is the algorithm will continuously improve, the more it sees.”
AI techniques
It’s well-known Optimize is launching a suite of new algorithms which take the latest technology to deliver transport efficiency for its clients, but perhaps you can explain a little bit more about the theory behind the developments?
“There are two sorts of AI techniques that we‘re developing at the moment,” he explains. “One is the Monte
Carlo Tree Search (MCTS). This is applied when there is a particular sort of problem that you‘re trying to solve. There are multiple configurations that can be used and the software tries to find the best solution, given the current inputs.
“It can periodically switch between configurations to figure out if others are better. For instance ‘I‘ve been using this configuration for the last 10 minutes. I‘m gonna try this one for one or two tries and see if it’s better.’ It’s a technique commonly used in chess AI where MCTS has been applied.”
Best sub-algorithm
The second AI technique Conroy mentioned is a very new solution he and his team have been working. “We‘ve been using machine learning to predict the best sub-algorithm for each of many different types of problem,” he continues.
“So you could have data from very dense urban multi-drop and other data from longer distance activities. You’ll want to use a very different solver for each of those problems. You can‘t really write one solver to cover everything well. So instead, this super-algorithm uses features of the input model - such as the density of the jobs, how many there are, the type of geographical area – and tries to pick the best solver for that situation.”
Initially the application will be for optimisation, but Conroy explains there will be other opportunities: “The super-solver is something that will help with the mobile and transient hubs as well. Our strategic aim is to have one black box solution which can intelligently direct itself to whichever set of algorithms it requires to solve the problem the user has presented. A director of operations, or super-solver. All the algorithms which we currently use for separate discrete products will be available to it as needed,” he concludes.