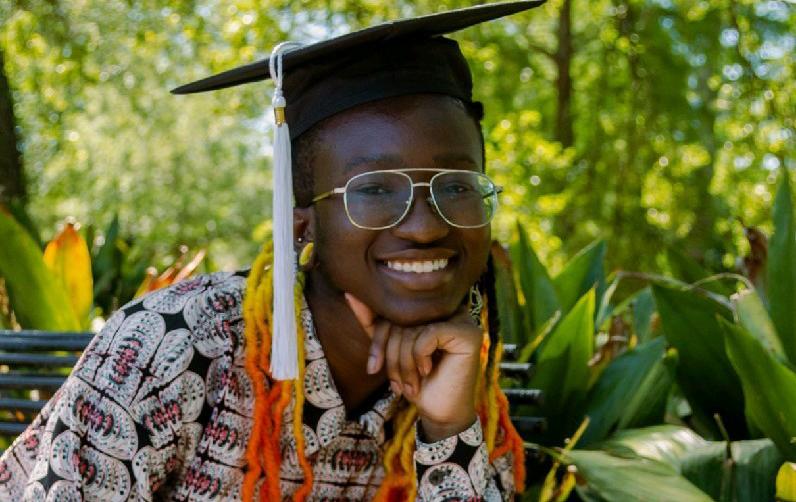
2 minute read
Student Voices, Student Action
By Lucien Mensah (SSE ’21, LA *22)
Lucien Mensah is a graduate student at Tulane University pursuing his Master of Computational Linguistics degree. He is also a graduate assistant for Digital Humanities at Newcomb Institute and the Office of Gender and Sexual Diversity at Tulane.
Working at the intersection of humanities and technology is something that I’ve always strived to do, and this has been something I was able to achieve through Newcomb Institute’s Tech Lab. I began working with Newcomb as a senior on the Digital Research Internship team. This internship was an experience that was particularly awesome for me and was specifically intriguing even during the interview process, where there were a variety of ways that we could talk about ourselves and express our interest in the team. As I became a team lead during my second semester and a Product Developer this year, I’ve been able to have many experiences where I got to display my technical knowledge with tangible products, learn a variety of technologies, have powerful discussions about race and gender in tech, and think critically about the impact of the work that we do. One of the ways I was able to talk about race and gender in tech was through my zine article from last year, where I was able to discuss an issue that I am passionate about: linguistic underrepresentation in technology. Natural Language Processing (NLP) is taking the tech world by storm, as everyone wants to create chatbots or somehow get computers to understand language. Where we are and how far we’ve come is amazing. My issue, however, is with the lack of focus on people and languages left out of this innovation. Languages rich in written history are often the languages with the most developed NLP innovation. On the other hand, for languages based on oraliture such as proverbs, legends, traditional songs, and more, there is a bias to not work as much with these languages, as spoken speech is a more difficult task than working with written texts. Additionally, innovations hardly ever account for signed languages that also require a video component. Most software is developed in English in the first place, which gives us another problem of this bias. Bias in and of itself is a more significant problem within the fields of Data Science and Machine Learning, and constantly thinking of ethics within this field has also been a prominent discussion, as human rights problems become more mainstream discussion. I bring this all up because Black, Hispanic, and Indigenous people remain the most marginalized in technology, meaning our languages are also marginalized. When you begin to look at the intersections of gender, ability, race, class, you begin to see who is left out of the dialogues and how replications of offline, societal inequities are perpetuated through technology. I end my article by asking those of you reading this question: What are you doing to stay aware of these inequities and make space in Newcomb and beyond for those who are not included?