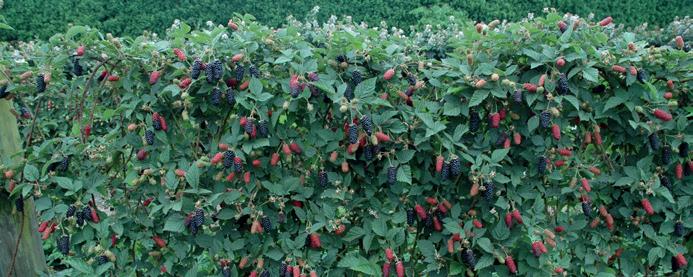
2 minute read
Selecting blackberries at the optimum stage of maturity
Title: Augmented berry vision
Funder: Innovate UK
Advertisement
Partners: Opposable Games (Lead), Berry Gardens Growers Ltd, University of the West of England
Term: September 2020 to September 2022
Selecting dessert blackberries at the optimum stage of maturity is key to ensuring that the final product purchased by the consumer is of high quality, looks good and, most importantly, tastes good. Consumer satisfaction is essential for repeat purchasing, but so much depends on the harvest team selecting the right berries at the right time, every time! Selection of perfect berries is challenging due to subtle colour changes that occur during ripening. Blackberry can be a particularly difficult crop, for although many berries might have turned black, they are not all at the same stage of maturity in terms of flavour development. Removing every berry that is black can lead to considerable variation in taste and flavour, and consequently consumer satisfaction. For pickers to select ripe fruit, fast, consistently and accurately, requires considerable skill, which takes time to acquire (Figure 1). Pickers, therefore, need a more reliable method of selecting uniformly ripe berries.
The project
This feasibility project set out to develop technology that can be used by harvesting teams to help them differentiate between blackberries which are fully ripe and those that are black but haven’t yet developed optimum flavour. With the help of Berry Gardens Growers, over 500 blackberries of varying ripeness were collected from member farms. Hyperspectral imaging of the fruit was conducted alongside laboratory assessments to determine berry ripeness and other metrics. From the analysis of the spectral images, key electromagnetic wavelengths were identified to provide significant differentiation between ripe and unripe fruit.
Results
Using the results of these analyses, a berry detection algorithm has been developed to detect and assess berries within a video feed. As berries are detected, their images are analysed to determine their ripeness. Machine learning was used to create the berry ripeness detection system. A convolutional neural network (CNN) was trained with multi-spectral images of blackberries of known maturity. The resulting algorithm showed a 95% accuracy in ripeness detection. During the project prototype hardware and software were developed. The hardware was tested in the field by experienced pickers (Figure 2) providing valuable insight to improve future versions. The system employs augmented reality (AR) glasses, which are worn by the pickers. Augmented Reality is the overlaying of visual digital information onto the real world through the lenses. Bespoke multispectral imaging cameras and the machine vision algorithm determine the ripeness of each berry, which is relayed to the picker via the AR glasses. This allows the pickers to pick berries that are uniformly ripe and to leave any berries, which have not developed optimum flavour, still on the cane to be harvested on another occasion.
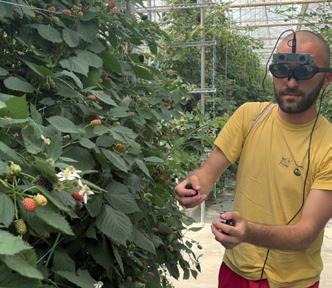
The concept was successfully demonstrated, and the partners are looking for routes to further develop the system into a product suitable for commercial use.