
5 minute read
Buyer Beware: Do advanced analytics really deliver?
Buyer Beware
Do advanced analytics really deliver?
by Steve Hanawalt
These days, you can’t avoid the hype touting all the ways advanced analytics (AA) optimizes the performance of solar and wind power assets. “Advanced analytics” is a broad term for a range of techniques and tools that takes data and uses it to generate valuable business insights, and make predictions and recommendations. For the purposes of this article, the umbrella term “advanced analytics” refers to a range of techniques and tools, including artificial intelligence (AI) and machine learning (ML).
If the buzz about advanced analytics is to be believed, you simply plumb your operating data up to a shiny new web service and the insights pour forth—saving you millions! But is it really that easy? What kind of real-world results can you expect? What data infrastructure and pre-processing do you already need to have in place for these applications to deliver reliable ROI? Before moving into advanced analytics, what about making standard industry analytics work properly? Are you confident you’re capturing all the asset performance improvement opportunities available?
Buyer beware—there are a lot of claims out there from software vendors and consultants looking to sell unproven or misapplied technology in the renewable energy space. On the other hand, some AA solutions generate significant opportunities for improvement in project returns—when properly applied. Therein lies the challenge: What is the right application of AA in the renewable energy space?
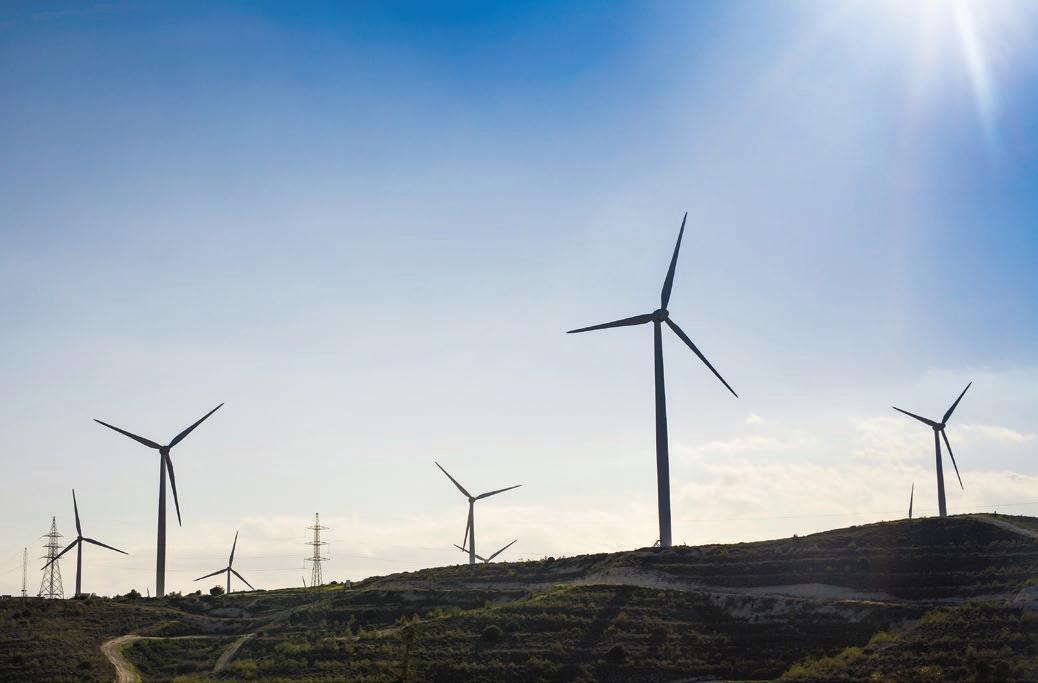
The Five Keys to Successful Implementation
To help find the answer, let’s look at the five keys of successful AA implementation in solar and wind:
1. The right data platform: Do you have a robust and scalable data platform with a fully integrated timeseries, transactional, and metadata layer? If you don’t, you won’t be able to meet the requirements of the following two keys.
2. A robust data cleansing process: Operating data from renewable energy plants is high in volume, frequency, and noise (meaning it’s full of missing and bad or corrupted data). Many advanced analytics run just fine in a laboratory, but fail miserably when they encounter the mess of real-world operating data. A robust and automated data cleansing pre-process is mandatory. There is no direct flight from raw data to insights.
3. A powerful event detection and classification engine: When you’re pulling millions of data points from your plants over the course of a year, you need a reliable tool to help you separate worthless data from data you can use. Manually classifying every event and failure type used in the analytic and anomaly detection process would require an army of data analysts - your data platform needs an industrial-strength, automated event detection and classification engine that’s designed by experts in solar and wind power. General-purpose algorithms won’t be able to associate events with renewable energy asset failure modes and effects. They might alert you to a problem, but that won’t be much help when it comes to determining the cause, and prioritizing the issues that expose you to the most risk.
4. The right tools: Make sure you’re applying the right set of tools to your problem. AA relies on a diverse set of tools (models) to classify data and events or to find performance anomalies. It is not always necessary, and sometimes not even a good idea, to use an advanced algorithm when a linear regression will suffice.
5. Dedicated subject matter experts (SMEs): Most often, generating corrective and preventive actions from the insights raised by AA is neither easy nor obvious. It requires solar and wind power operational SMEs to interpret. These technicians, analysts, and engineers know how the equipment really works, its failure modes and causes, and the proper actions for resolution. You won’t reach your software ROI goals without a skilled team dedicated to the process of post-AA analysis and resolution.
When to Consider Advanced Analytics—and When Not To
Advanced analytics should be considered for anomalies that aren’t or can’t be detected using the plant SCADA or traditional monitoring applications. For example, you don’t need AA to tell you your wind turbine or solar inverter is offline, and you shouldn’t have to use AA to tell you that a tracker has stopped tracking. However, if your plant SCADA system isn’t pulling tracker control setpoint data, advanced analytics can detect that a tracker has stopped tracking. In other words, before you invest in moving up the asset optimization pyramid, make sure you have each of the five keys to a successful implementation.

Consider the typical utility-scale solar power plant: It has thousands, even millions of non-instrumented electric generators. Sensors well downstream of these generators don’t have the sensitivity to detect performance issues associated with these generators. So how can we know if our solar generators are performing optimally? We have three choices:
• Manually inspect them—a very cost-prohibitive option.
• Use thermal imagery—a good, but periodic, option.
• Use advanced analytics—a good continuous option because the typical solar power plant does not have sensors located close enough to the generation equipment to detect subtle shifts in operating performance.
Advanced analytics solves other problems specific to our industry as well, such as using machine learning to detect subtle changes in a turbine’s power curve, or to catch the temperature derating of a solar inverter. Additionally, automated workflows incorporating AA can generate a work order with all details about failure mode, failure cause, and repair code recorded by the software. By integrating the event detection, insight, and action steps into an automated workflow, we can reap the benefits of advanced analytics.
To Buy or Not to Buy?
That’s the big question. Is advanced analytics worth the investment? It can be. But if you’re trying to solve the wrong problem, or if you don’t have the right data platform, business processes, and subject matter experts in place, you’re far better off investing your money getting the basics done right first.
Steve Hanawalt is co-founder and executive vice president of Power Factors. Power Factors consolidates multiple operational data sources, asset hierarchies, and metadata frameworks to create a single cloud-based remote asset management platform that works with today’s large-scale portfolios.