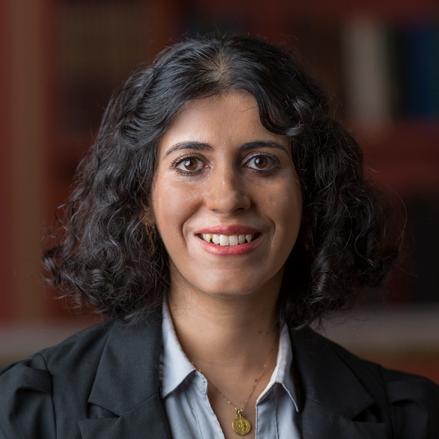
2 minute read
“Engineering” Solutions for Healthier Brains
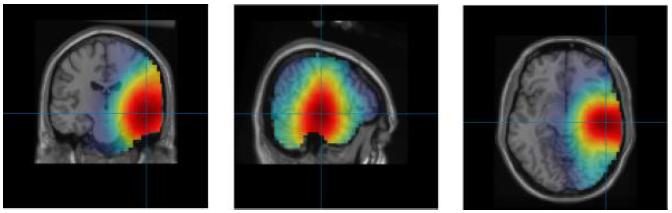
Could artificial intelligence help solve the mental health crisis? What if an algorithm allowed neurologists to know the area affected by a brain seizure?
These are just two of the questions that Maryam Ravan, Ph.D., assistant professor of electrical and computer engineering, aims to address. Working on two separate studies, she proposes the use of cutting-edge algorithms and techniques that could provide noninvasive solutions for clinicians to treat neurological and psychiatric conditions more effectively.
Normal brain activity, including mood and thinking processes, relies on a balance of fast and slow brain waves. Imbalanced brain wave activity is associated with neurological issues such as epilepsy and psychiatric conditions like bipolar disorder and major depressive disorder.
One technique clinicians use to detect brain wave imbalances is electroencephalography (EEG). This diagnostic test uses sensors to measure the electrical activity on a patient’s scalp, which is representative of the brain wave activity taking place underneath. Brain wave data collected through an EEG holds valuable information regarding an individual’s neurological and mental health. Existing software models that process EEG brain wave data use a mathematical technique called exact low-resolution brain electromagnetic tomography (eLORETA).
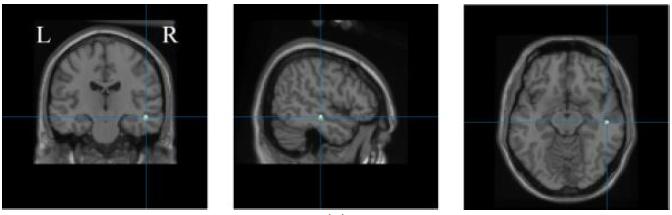
In collaboration with McMaster University psychiatrist Gary M. Hasey, M.D., Ravan co-authored two studies that leverage machine learning, a form of artificial intelligence (AI), to improve the treatment of mental health conditions.
In one study, Ravan helped develop a machine learning algorithm to analyze patients’ brain waves and categorize their patterns as biomarkers for bipolar disorder or major depressive disorder.
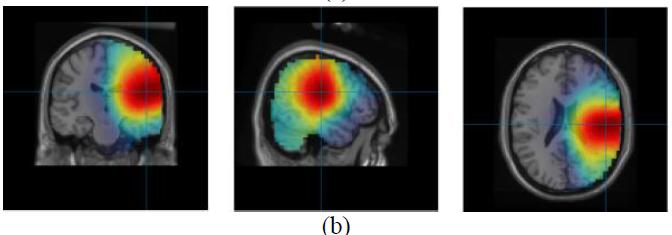
The researchers pose that, given its abilities, machine learning could be useful in analyzing a patient’s EEG results and determining whether a patient is experiencing bipolar disorder or major depressive disorder. With Ravan’s expertise, the team developed a new algorithm called ReLORETA, which accounts for multiple variables known to impact brain source estimates, including head geometry and misaligned brain electrodes, among other factors.
“The accuracy of our algorithm, which was derived from a large sample of patients, successfully distinguished bipolar disorder from major depressive disorder more accurately than the existing methods available to clinicians,” says Ravan. “This suggests that our method may hold significant promise as a clinical tool.”
A second mental health study explores how brain wave data could be used to predict how patients with major depressive disorder will respond to the antidepressant sertraline (sold under the brand name Zoloft) versus a placebo treatment.
While antidepressants are often the first line of treatment for major depressive disorder, it can take months of trial and error and multiple medications before reaching an effective treatment. If predictive biomarkers can inform how a patient will likely respond to a certain treatment, clinicians may be able to eliminate this frustrating process and fast-track a patient’s recovery time.
The study’s other co-authors include College of Engineering and Computing Sciences alumni Thomas Oakley (M.S. ’22), Jonathan Coskuner (M.S. ’21), and Andrew Cadwallader (M.S. ’22).