
4 minute read
Machine Learning on the Smart Farm
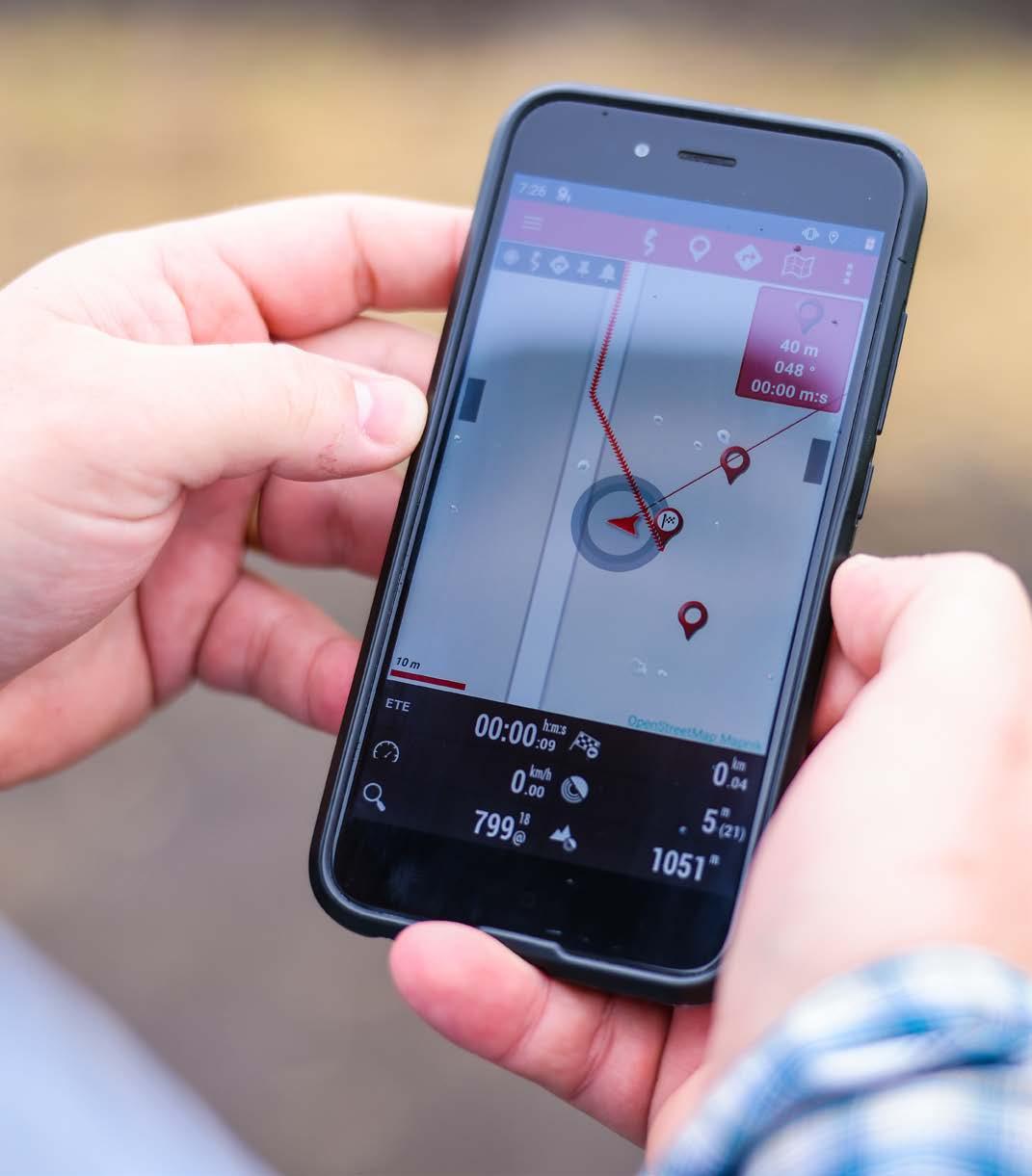
Smart farms and technology innovation have a critical role to play in this ‘global grand challenge’ of feeding a growing population with fewer resources. This creates opportunities for technology, data, and artificial intelligence to help crop and livestock producers sustainably meet increasing demands and manage their risk.
Advertisement
Digital Ag Collaborations
Machine Learning on the Smart Farm
According to the United Nations, the world’s population will increase by two billion people by 2050, and food productivity will need to rise by approximately 60 per cent. With only one growing season per year, farmers are on an accelerated timeline to meet the demand for food — all while addressing food sustainability, plant and animal welfare, labour shortages, supply chain challenges, and climate change.
Throughout this growing season, Olds College Center for Innovation (OCCI) is collaborating with industry partner AlgoRythmn Corp. to apply machine learning and data-driven solutions to enhance market and financial risk management innovations, so producers can make informed decisions to maximize profitability and minimize risk.
Machine Learning Explained
Machine learning is the application of data and algorithms to help machines mimic the way humans learn and make decisions. It identifies patterns in the data to generate structure and predictions without the need for human intervention. Using various datasets, machine learning algorithms autonomously improve their performance.
Machine learning already enhances many everyday tasks. It is the underlying technology powering most smartphone apps including virtual assistants like Siri, traffic prediction patterns on Google Maps, and Netflix recommendations. It also controls autonomous vehicles, machines that diagnose medical conditions, and robo advisors who manage financial portfolios.
At its core, machine learning uses data to answer questions. ‘Using data’ is typically referred to as ‘training’, while ‘answering questions’ is referred to as ‘making predictions’. What connects these two parts together is the model. The model is trained to identify trends and correlations using a dataset. Then, a new unseen dataset makes predictions based on what it learned during training.
Machine learning can be simplified into the following steps:
1 CHART-SIMPLE
Gathering Data:
This is the first step in machine learning. The quantity and quality of data is very important and will determine the predictive ability of a model.
2 ARROW-DOWN-UP-ACROSS-LINE
Data Preparation:
The data is prepared to be used in the model, and examined to identify and mitigate errors. The data is also split into two parts: a ‘training’ set used to train the model and a ‘testing’ set used to evaluate the model’s performance.
3 MICROCHIP
Model Training:
The model is given the training data which consists of inputs and their corresponding classification. Using this data, the algorithm constructs its first prediction.
4 NETWORK-WIRED
Model Testing:
Once a prediction has been made, an error function evaluates the accuracy of this output by comparing the prediction value with the known value of the training data.
5 COGS
Model Parameter Tuning:
Depending on the discrepancy between the estimated value and the known value, weights are adjusted within the model to improve the prediction accuracy until there is a low margin of error.
6 CHECK-DOUBLE
Model Evaluation:
The machine learning model is given the testing data set in order to evaluate the model’s predictive ability on new, unseen data.
Machine Learning in Agriculture
The agriculture industry has seen rapid changes in methods of data collection, analysis, and interpretation as technology such as electronic sensors, processors, and drones become increasingly accessible and affordable. As the volume of farm data continues to increase, there is a growing opportunity to adopt machine learning and datadriven models to all stages of agricultural production from pre-harvest to post-harvest.
Smart farming and precision agriculture is the first step towards farm management that utilizes data to increase farm productivity and decision making. Rather than basing decisions per field, these technologies allow decisions to be made per square meter, management zone, or even as granular as per plant and animal. Precision agriculture predicated on machine learning technology has the potential to increase production, improve product quality, lower yield variation, and reduce labour and environmental impact.
Examples of machine learning applications in agriculture include yield prediction and mapping, satellite imagery for analyzing crop conditions, soil property analysis, disease risk estimation, crop identification, and autonomous farming equipment operation.
Financial Risk Management on the Smart Farm
As data becomes increasingly available from crops, the environment and the market, there is an opportunity to apply machine learning towards developing data-driven financial risk management plans to help producers make informed decisions affecting the profitability, productivity and long-term viability of their farm.
OCCI and Algo-Rythmn Corp. are working together to enhance market and financial risk management innovations, and provide actionable insights and tools for farmers. One area of focus is addressing the variability of input and output prices using data-driven price prediction and hedging decisions.
Stay tuned for more machine learning insights from the Smart Farm at oldscollege.ca/smartfarm.
Algo-Rythmn Corp. is a technology integration company focused on the application of advanced analytics, such as big data and artificial intelligence algorithms, to provide data-driven solutions.
At the beginning of June 2022, the team calculated the missions and acres completed for OMNiPOWER so far this growing season. OMNiPOWER had already completed:
21
Missions spreaded 1,115
acres seeded over 635
acres sprayed over 325
acres
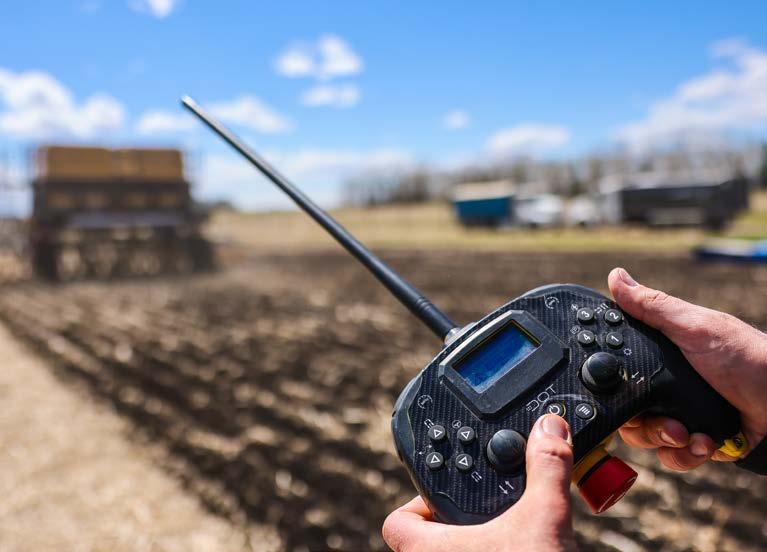
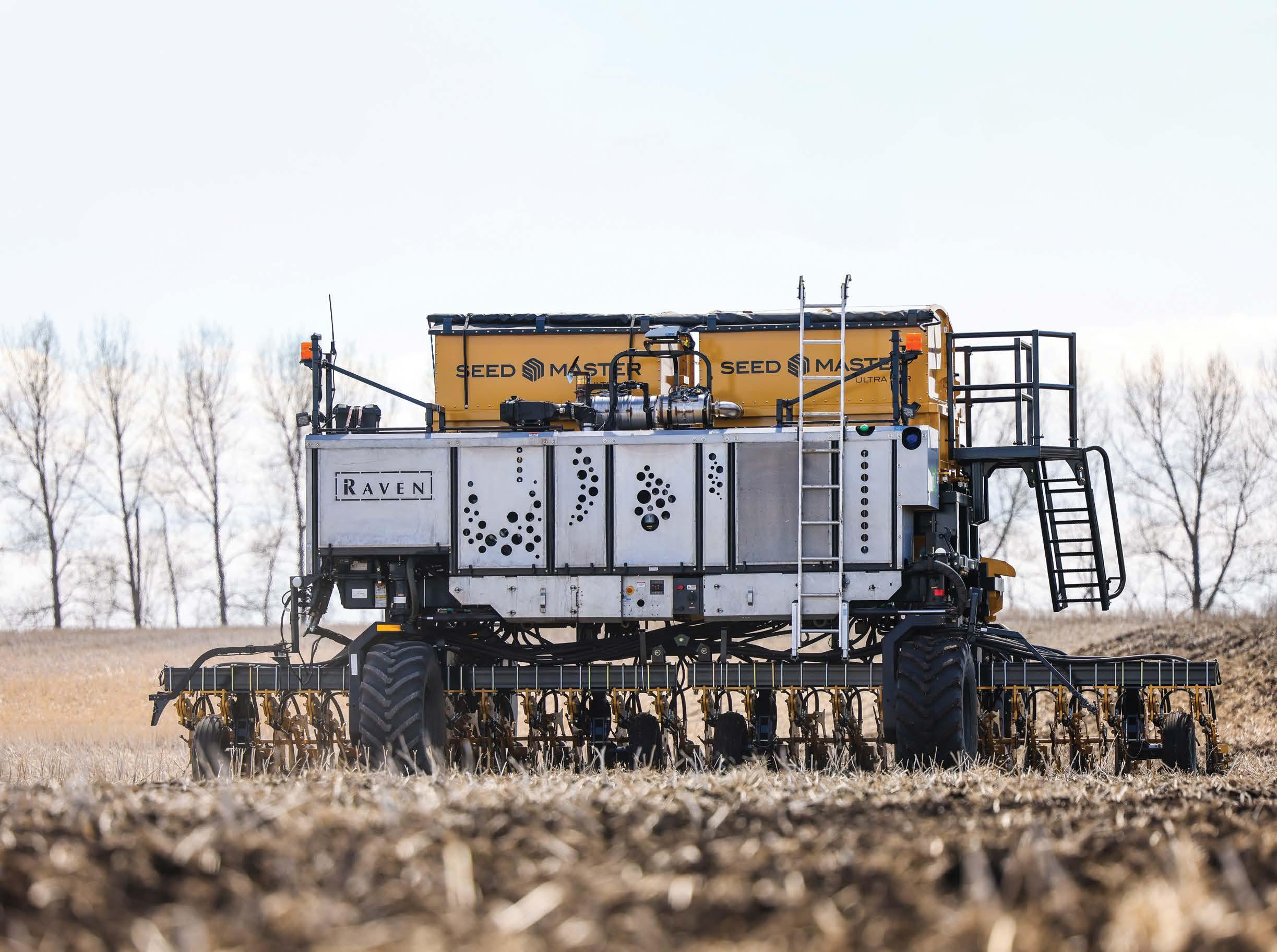
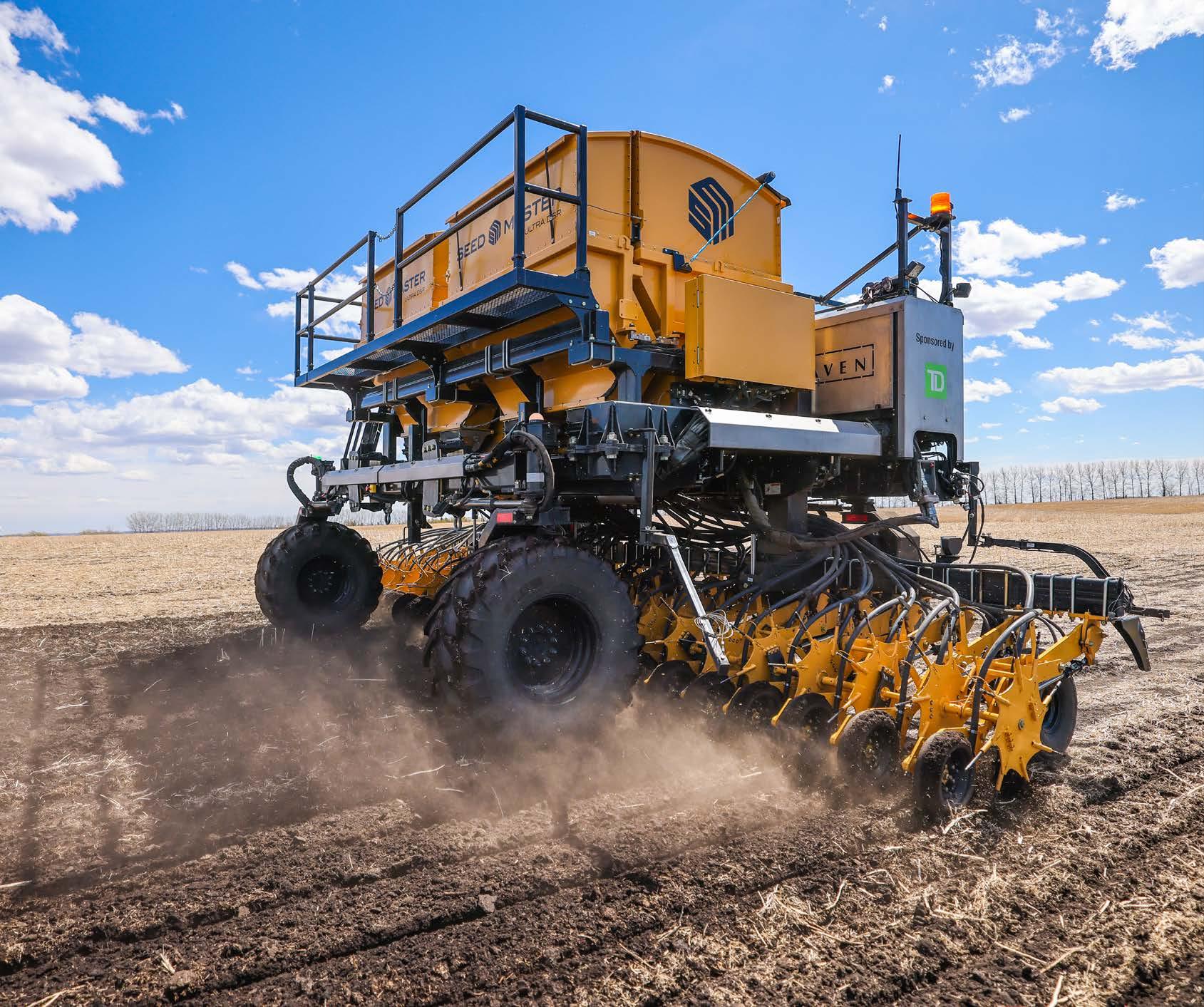
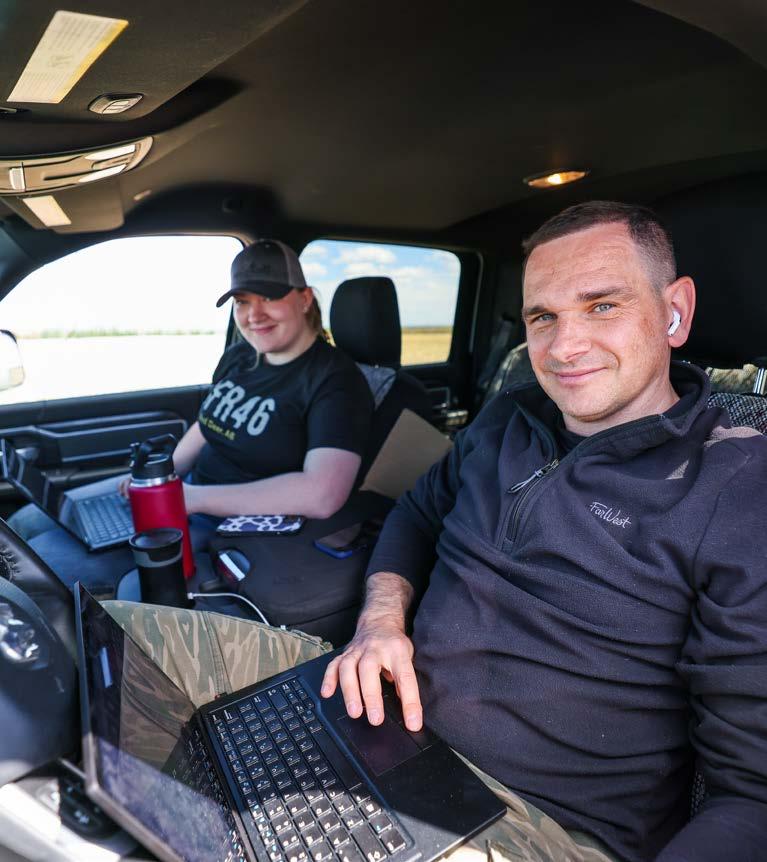