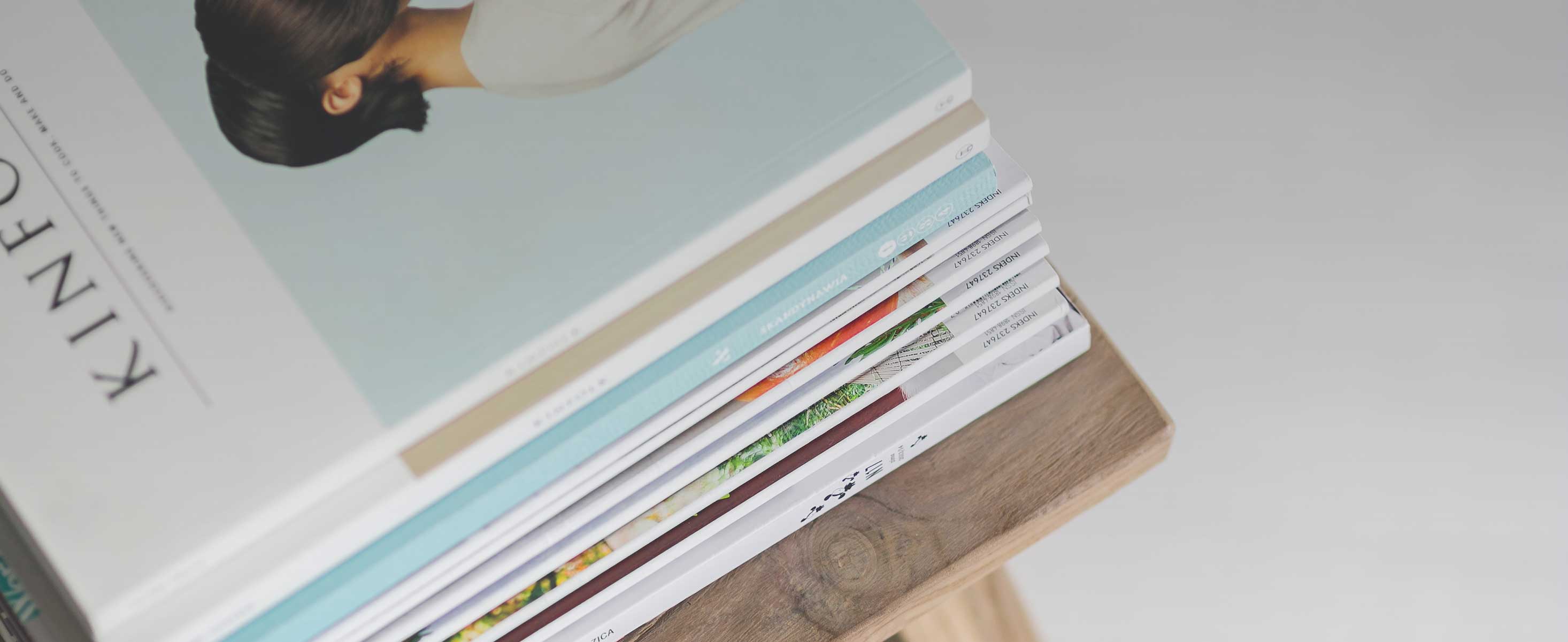
6 minute read
HOW MANAGING THE RISKS OF AI IS KEY TO HARNESSING ITS POTENTIAL
Generative AI is becoming a strategic imperative with its vast potential, but companies must also navigate a unique set of risks.
By Ritin Mathur, Partner, Consulting - Data and Analytics, Ernst & Young Advisory Pte. Ltd and Marie-Claude Ferland, Associate Partner, Consulting - AI Strategy, Ernst & Young Advisory Pte. Ltd.
Generative artificial intelligence (gen AI) came into the spotlight globally when a language-based AI chatbot was launched in November 2022, with over a million users within the first week of its launch Gen AI is an innovative form of AI that is capable of creating sophisticated content — such as images, text, and audio — by leveraging large amounts of internet data and cutting-edge computing power. Many businesses today across all sectors are seeking to fully understand gen AI to embed its use into their operations and develop the right strategy to harness its potential.
Potential of gen AI
Gen AI eliminates the need for laborious data labeling through a semi-supervised or unsupervised technique. Trained with data scraped from the internet, a gen AI app can quickly generate content — such as poems, programming codes and contextually-aware legal briefs — in response to a few short queries. This facilitates content creation and lowers its cost.
Gen AI’s use cases currently extend across many sectors, and it is already performing mundane tasks like customer service, freeing up manpower for higher value-adding work. In creative and entertainment industries, such as moviemaking, music production and gaming, gen AI has delivered convincingly original scripts, images, music compositions and even performances. In e-commerce, gen AI-enabled virtual assistants with access to shoppers’ profiles as well as purchasing and browsing histories enable targeted recommendations.
Limitations of gen AI apps
While gen AI may be seen as the next digital phenomenon, its commercialization poses challenges. At its core, a large language model (LLM) is a statis - tical machine trained to predict the most likely word to follow a given sequence of words in a sentence. Unlike humans, gen AI lacks a comprehensive understanding of its output’s meaning, implications, and nuances. In domains where accuracy reigns supreme, such as medicine and law, gen AI impresses with its ability to produce technical content. But outside of these domains, its use of syntax and phrasing can often lead to “hallucinations”: presenting incorrect or unrelated information in a convincing manner.
Another limitation of gen AI is its inherent language bias. An LLM is limited by the training data’s quality where the quality of its output is only as good or bad as its training data. Because most of the internet is in English, a language-based gen AI chatbot is still subject to language bias even when supported by a massive LLM. As a result, it can only produce more accurate responses in English than in other languages.
It is also impossible to create gen AI models as know-it-all oracles. While gen AI can comb the internet and consolidate all available information from it on any topic of interest, such content only represents a small fraction of human knowledge.
This is compounded by the time factor. For example, a language-based gen AI chatbot trained with a cutoff period of July 2022 possesses no memory of knowledge and facts after that period. However, a gen AI can become more sophisticated when its LLM is further trained although training LLMs requires massive and expensive resources. Without further training, there will always be knowledge gaps when using gen AI apps.
Despite the potential of gen AI, its rate of commercialization will likely differ across sectors, subject to data availability, industry regulations and technical maturity.
Navigating challenges and risks
The unique capabilities of gen AI present a unique set of risks that includes misinformation and the consequences of “deepfake” impersonations, where its ability to create unreliable content quickly, cost-efficiently and semi autonomously can lead to erroneous decisions by unsuspecting businesses.
The use of gen AI tools has raised privacy issues, where user information is shared with third parties without permission. Before the General Data Protection Regulation became prevalent, the internet operated on the assumption that digital content is freely available and leading LLMs have been trained by scraping a lot of such data.
Like all digital apps, gen AI is also susceptible to hacks, such as “prompt injection” that manipulates an LLM to perform unauthorized actions. Hence, the issues of cybersecurity and “hallucinations” present challenges when using gen AI in business operations.
Mitigating these risks requires strong data governance practices, including data anonymization and encryption with regular audits. Monitoring gen AI processes to identify misinterpretations and errors as well as keeping abreast of regulations can help businesses navigate potential risks.
Four key strategic actions for implementing gen AI
Given its vast potential, incorporating gen AI into business operations is becoming a strategic imperative. Businesses can begin their gen AI journey by taking four key actions:
1. Increase digital literacy and awareness within the organization with training programs, workshops, and industry partnerships.
2. Build a team with the right mix of in-house and external AI specialists, business strategists, legal experts, ecosystem partners and ethicists to produce near-term and long-term gen AI strategies.
3. Drive a dynamic and flexible implementation strategy by aligning use cases with business requirements where gen AI can offer tangible benefits in a realistic schedule.
4. Conduct regular reviews and risk assessments on how gen AI is improving business processes and integrating operations where relevant for greater efficiency and cost-effectiveness.
Unlike other digital transformation journeys that may have daunting requirements, the lack of need for data labeling in gen AI means that leading tech companies can pool their resources to train general-purpose models. This makes it easier for businesses to build semantically relevant AI apps.
With its superior learning and adaptation capabilities, gen AI offers a myriad of use cases and has the potential to drive productivity gains and reshape the business landscape. By monitoring its performance, having a sound implementation strategy, building the right competencies, keeping up to date on regulatory developments and implementing strong data governance, companies can tap into such potential for significant business value.
The views reflected in this article are the views of the authors and do not necessarily reflect the views of the global EY organization or its member firms.
About The Company
EY exists to build a better working world, helping to create long-term value for clients, people and society and build trust in the capital markets. Enabled by data and technology, diverse EY teams in over 150 countries provide trust through assurance and help clients grow, transform, and operate. Working across assurance, consulting, law, strategy, tax and transactions, EY teams ask better questions to find new answers for the complex issues facing our world today. EY refers to the global organization, and may refer to one or more, of the member firms of Ernst & Young Global Limited, each of which is a separate legal entity. Ernst & Young Global Limited, a UK company limited by guarantee, does not provide services to clients. Information about how EY collects and uses personal data, and a description of the rights individuals have under data protection legislation are available via ey.com/privacy. EY member firms do not practice law where prohibited by local laws. For more information about our organization, please visit ey.com.