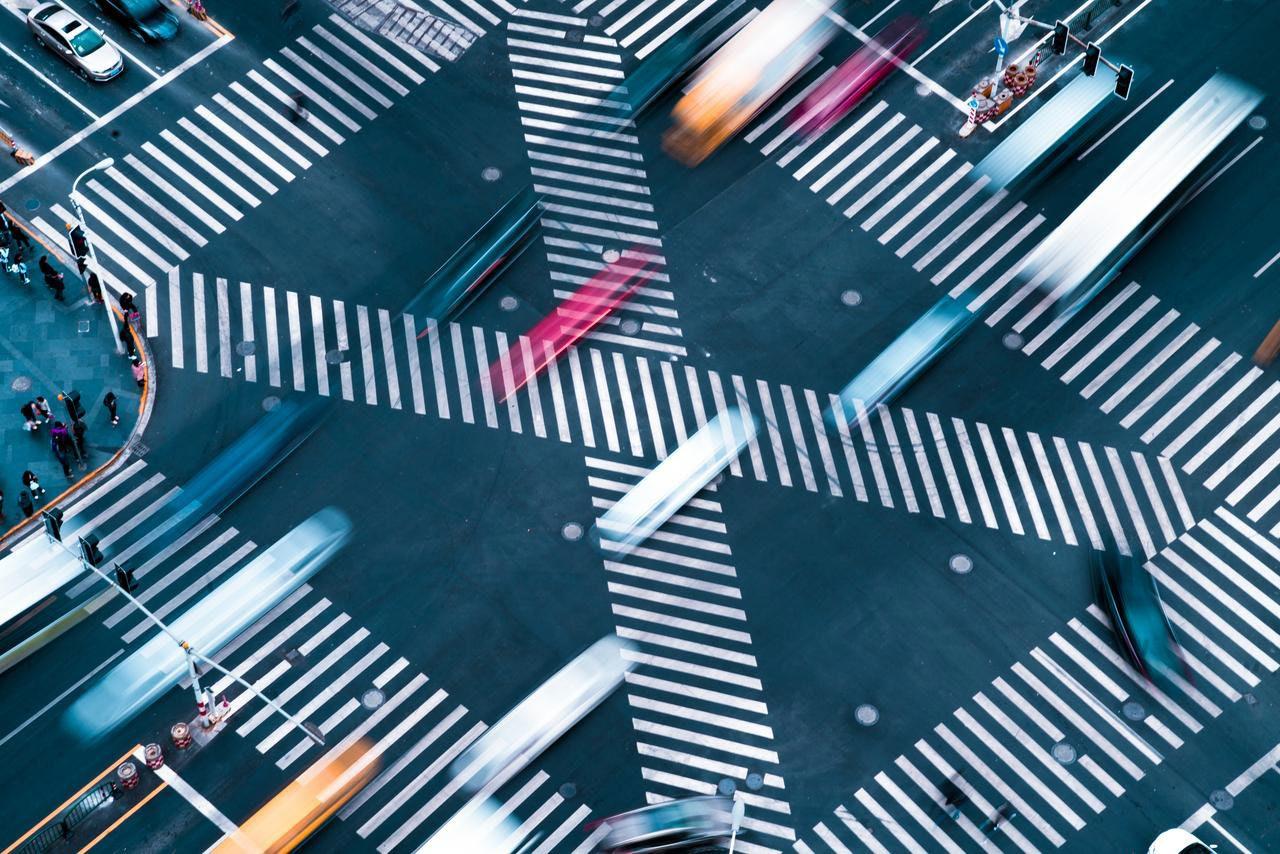
6 minute read
HOW FAR ARE YOU ON THE PATH TO BECOMING A DATA DRIVEN COMPANY?
by Steve Nunez Head of Data & AI, Zühlke Asia
Many companies aspire to continuously generate value and competitive advantage through data and artificial intelligence (AI) technology. What does this journey towards being a data-driven company look like? And what barriers do companies face along the way?
Advertisement
The potential of data and artificial intelligence is vast and beyond dispute. By 2030, AI technologies are expected to contribute around $15.7 trillion to the global economy. Most companies invest in data and AI projects, but many report that the potential of these projects is only partially exploited.
We surveyed over 110 international businesses in a study to understand how decision-makers view the potential of data and AI, how far companies have advanced towards becoming a data-driven company, and what obstacles they are encountering.
5 typical barriers companies face in becoming data-driven
1. An inactive data innovation pipeline
A key element for a data-driven company is an active data innovation pipeline. This refers to the continuous planning and implementation of data and AI projects that generate value for the organisation. However, this pipeline is not active in many companies due to the misalignment between business goals and technology or the lack of a defined strategy.
43.6% of respondent companies thought that AI initiatives failed because there was too little consideration of business success or too much emphasis on technology. The second-most common driver was the lack of a company-wide data and AI strategy (33.6%).
2. Proof-of-concepts falling by the wayside
Proof-of-concept (PoC) studies act as a checkpoint where the feasibility of projects is reviewed. Many projects remain stuck at this phase due to a narrow PoC or insufficient expertise for operationalising use cases.
32.7% of respondent companies indicated that their AI initiatives faced problems associated with PoCs. The most frequently cited reason (40.9%) was the poor quality of data and models.
In an example at a plant construction company, we found a lack of infrastructure for operationalisation. Although the project team developed good models, it was unable to progress beyond the PoC as there was no direct access to the customer’s systems. Creating access would mean integrating multiple systems across the globe, costing millions.
3. Technically perfect solutions are not used as planned
A user group may fail to use an application as planned. This may be caused by a solution that is insufficiently tailored to real-life practice, prejudice against AI solutions or user experience difficulties.
Failing to integrate AI solutions was the most common reason for the users failing to adopt the solution (52.7%). Further reasons cited were insufficient training of end users and distrust towards AI solutions.
In one project with a vehicle manufacturer, a pilot MVP AI solution was developed to forecast demand for replacement parts. However, it emerged that the predicted parts were either so small that inventory was always available on hand anyway or so large that they had to be ordered. This meant that the solution delivered no added value for its users.
Technical competencies represent a major barrier for successful implementation of data and AI projects. Some companies are unclear about what skills is required. Others may have the right job profiles, but their employees are unable to apply their skills because of sub-optimal structures.
55.5% mentioned that the lack of collaboration was a major issue. This shows the importance of deploying in-house data consultants who have experience in implementation projects and can build bridges between teams.
4. The data itself
While many companies already have valuable datasets, they don’t make them available in a structured way. Together with availability, data quality is often cited as a barrier.
49.1% said that while the necessary data had been gathered, it was not readily accessible.
Another frequent statement was that the relevant data was of poor quality (45.5%).
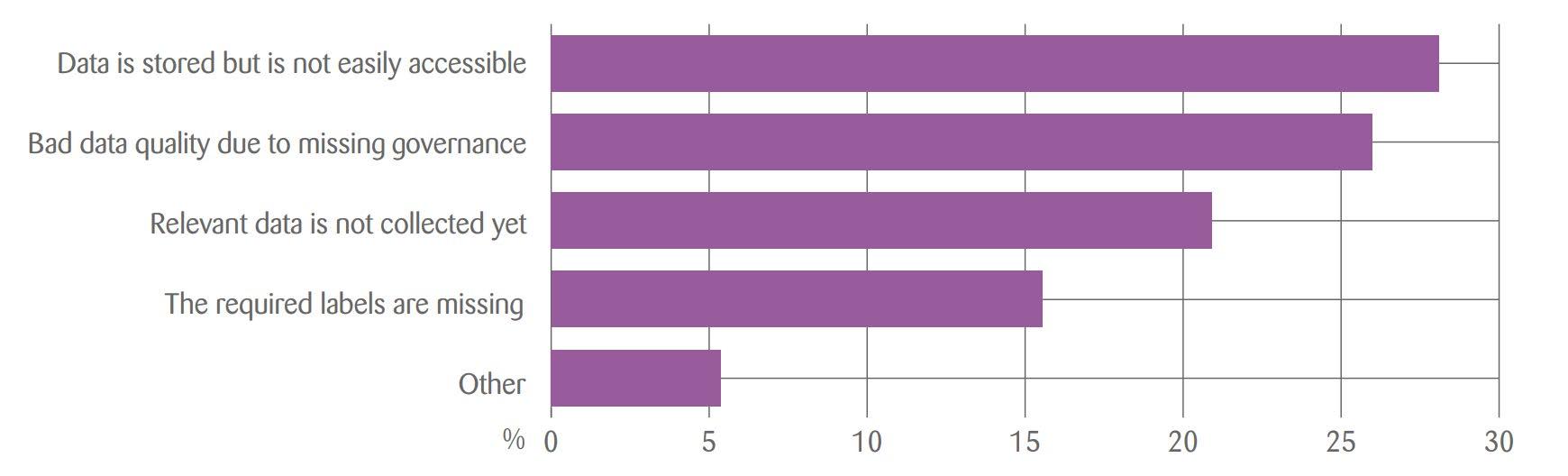
How far have companies progressed on their journeys?
These companies recognise the potential and significance of data and AI. However, there was a lack of holistic implementation concepts.
1. “Innovation” – They face challenges across all five barriers
We identified 3 levels of maturity during a cluster analysis to understand how these companies progressed to becoming a data-driven company.
2. “Foundation” – They experience challenges in the last two barriers
These companies can be found further along the journey, but they face challenges in establishing foundations commonly associated with data-driven companies.
3. “Data-driven” – They can already be described as data-driven
These companies are the most advanced along the journey to a data-driven organisation but continue to anticipate an uptrend in data analytics and AI use in the near future.
Becoming a data-driven company
From the barriers identified, we derive the following success principles for data and AI projects:
• Company-wide planning and orchestration
• Business orientation
• Early user involvement of AI solutions
• Fast and agile execution, plus a willingness to learn as an organisation
Organisations can incorporate these principles within a data-driven company framework in 3 main steps:
1. Determine the vision at the C-Level
Set up a core team at the management level to align decision-making, develop a strong vision and formulate outcomes that the company aims to achieve. This ensures that the whole company can be aligned on this journey.
2. Define the data strategy and establish AI portfolio management
Develop a data strategy using the company strategy as a starting point, then operationalise it through a portfolio of concrete projects and initiatives. Furthermore, a portfolio process in place can function as an impulse generator for the project pipeline.
3. Create the foundations on an ongoing, incremental basis while implementing value-adding solutions
Establish the foundations step by step while projects are implemented in parallel. These foundations include data platforms, structures and processes that are based on concrete use cases and fit for real-world use. For initial implementa- tion, choose use cases with sound prospects for success. These projects will serve as a beacon and strengthen internal acceptance of the company’s transformation.
“The era of experimentation in AI is over. You expect to see tangible results from AI investments. And we believe that an integrated and holistic approach is the way to achieve this.”
By laying the right foundations and fostering an open culture, companies can progress on their journey to be more data-driven, paving the way for organisations to operate efficiently, compete effectively and navigate the digital future.
About the company
Zühlke is a global innovation and technology service provider with over 50 years of leading expertise in strategy and business innovation, digital solutions and application services. Founded in Switzerland in 1968, Zühlke operates in 16 locations worldwide, supported by a talent pool of over 1,600 technologists and innovation experts. Groupwide, we support businesses on over 10,000 successful projects, working with clients from large Forbes 100 companies to innovative start-ups across diverse industries: from financial services, healthcare and medtech, enterprise and public sector and more. Visit https:// www.zuehlke.com/asia/en