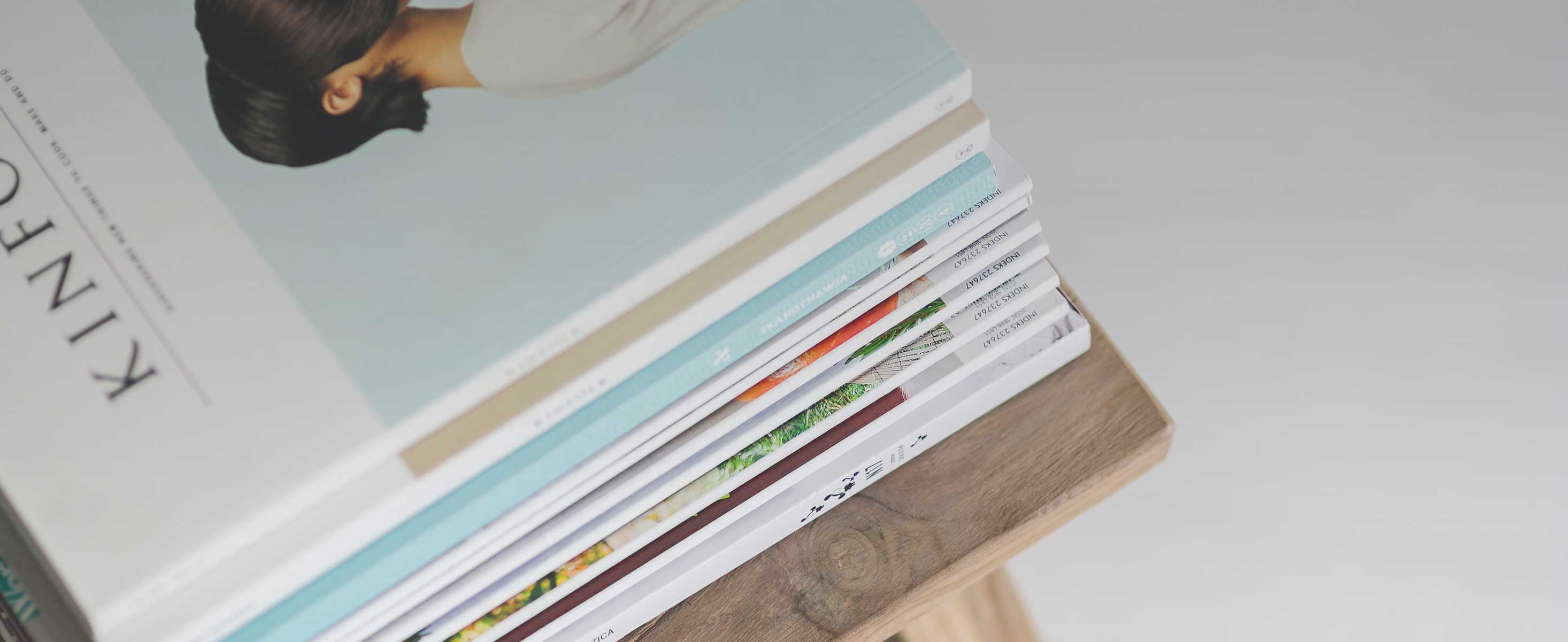
6 minute read
constraints, 2005
late 1980s and early 1990s, yet the scores on the release of data by their governments show a discernible increase. In this sense, the release of information by governments is clearly not based on current incomes. For these five transition countries, then, the significant increase in the release of information from the early 1990s suggests an (initial) optimistic attitude on the government’s part surrounding their country’s future prospects.
(ii) Political constraints:
Advertisement
If income does not appear to be primarily driving the release of information, then perhaps it is the prevailing political institutions that are: Figure 3.4 shows the relationship between the RI index and the degree of constraints on the executive (taken from the POLITY IV database) in 2005. As can be seen, as these constraints get larger, the release of information is also higher.vii Overall, the correlation between the two (in 2005) is 0.56. Of course, correlation is not causation, but this is certainly suggestive that governments tend to release more information when there is pressure on them to do so, not because they want to.
RI Index
RI, 4, 0.61 RI, 5, 0.61 RI, 6, 0.65
RI, 1, 0.45 RI, 2, 0.51 RI, 3, 0.50 RI, 7, 0.71
Executive Constraints (POLITY IV, 2004)
Figure 3.4 The Release of Information and degree of executive constraints, 2005
Source: Author’s calculations, Marshall and Jaggers (2002).
(iii) Other factors affecting the release of information
The interaction between the political/institutional constraints and the release of information is not the only consideration here. There are other plausible reasons why countries may (or may not) release information.
One potentially important factor may be the level of education enjoyed by citizens. Education may be associated with the release of information through a number of channels. Firstly, a better educated population may demand better information from its government, and may also have a greater ability to use and evaluate the information itself, both in an economic and a political sense. For example, a better educated population would be able to see, through this information released, whether the government was doing a good job with respect to economic policies, and vote accordingly at the next available juncture. In a more practical way, a higher level of education may also improve both the quantity and quality of data. For example, the government may be able to hire qualified statisticians to process the information. In a more general sense, Rauch and Evans (2000) have shown that the quality of a country’s bureaucracy depends importantly on the level of education attained by these public officials.viii
It has been suggested that countries with an abundance of natural resources (particularly energy and mineral resources) have experienced, on average, lower economic growth over the past thirty years (Sachs and Warner, 1997). One hypothesis for this slow growth is that these resources promote rent-seeking behavior on the part of public officials, which fosters poor institutions through higher corruption, patronage, and so forth. As Kolstad and Wiig (2008) outline, there are a number of ways that a lack of transparency can create or exacerbate the problems associated with resource-rich economies. This lack of transparency can: (i) make corruption more attractive, in that it can reduce the probability of discovery; (ii) make it easier to capture rents; (iii) create principalagent problems (between governments and its citizens, but also between elected officials and the bureaucracy); and (iv) can undermine democracy via these resource rents by reducing the need for domestic taxation (and hence reduce demands for accountability), increasing spending on patronage, and/or increasing spending on the direct oppression of dissenting voices.
Empirical Analysis
Because it is highly likely that there are a number of factors influencing the release of information, it is therefore important to take a more rigorous statistical approach to this issue. The sample consists of 91 countries. The panel data estimation being employed is based on nine five year non-overlapping periods between 1960 and 2004 (unless otherwise stated all variables are taken as averages over each five year period). Because I am initially interested in the causal nature of the relationship between transparency and these other factors, all variables have been lagged by one period (including the lagged value of the transparency variable). Based on the theoretical considerations above, the core specification is:
RIit = â1RIit−1 + â2LNYit−1 + â3XCONSTit−1 + â4Zit−1 + λ t−1 + ηit−1 + vit−1 (1)
where RI is the Release of Information index (proxying transparency), LNY is the log of per capita income, XCONST is the degree of executive constraints, while Z are additional factors that were hypothesized to be important possible determinants of transparency above (the full list of variables and their sources can be found in the Appendix).
There are two specification issues that require some initial attention. The first is that the lagged dependent variable will be endogenous to the fixed effects in the error term, which means the results will be biased. There are two possible methods to correct this. The first is to difference the data to remove these fixed effects (or equivalently to include dummy variables for each country). A second method is to instrument the lagged dependent variable and any other potentially endogenous variables in the regression with other variables uncorrelated with the fixed effects. First differencing accompanied with using the level of past values as instruments gives rise to the well-known “difference GMM” estimator from Arellano and Bond (1991). A third method is to first difference the instruments themselves in order to make them exogenous to the fixed effects. The combination of first differencing the variables and instrumenting with lagged levels of these variables, and using the levels of the variables with the first differenced instruments, gives us the ‘System GMM’ estimator, developed by Blundell and Bond (1998). This estimator also
assumes that, whilst there will be first-order serial correlation in the error term, there should not be second-order correlation. Lastly, the validity of the instruments can be tested for using the Hansen, and HansenDifference tests.
Table 3.4 summarizes the initial results with the RI index as the dependent variable. Column 1 includes only the income and executive constraints variables, and both have a positive and significant effect on the index. However, the size of the coefficient for the income variable decreases substantially (and, indeed, even changes sign) once additional variables are included in Columns 2–4. Education appears to have only a marginal effect on the release of information (Column 3), however energy and mineral resource exports have a highly significant effect on the release of information. The negative coefficient on the export variable strongly suggests that countries that have a heavy reliance on energy and mineral exports subsequently also release less information.ix It is not altogether clear from this table, however, why this might be so. If the only reason for this lack of information is because of an increased propensity for rent-seeking or corrupt behavior, then the addition of the corruption variable in Column 4 should substantially reduce the coefficient on the resource export variable. As can be seen, however, the coefficient is virtually identical. Nevertheless, the inclusion of the ICRG corruption variable also demonstrates, for the full sample at least, that an increase in corruption leads to a decrease in the release of information.x Lastly, as a check to see whether these results are purely a function of the inclusion of the high-income (and largely high-transparent) OECD countries, I have removed these countries. Executive constraints and energy and mineral exports continue to have a significant causal impact on the release of information, however, it appears as though the significance of the corruption variable was due in large part to the high scores achieved by these OECD countries. Once these countries are removed, there is no evidence that corruption affects the release of information for developing countries.
Having briefly looked at what some of the determinants of the release of information are, the final step is to see what effect (if any) this has on economic growth. Column 1 of Table 3.5 includes the variables employed in the Table 3.4, as well as population growth, which, along