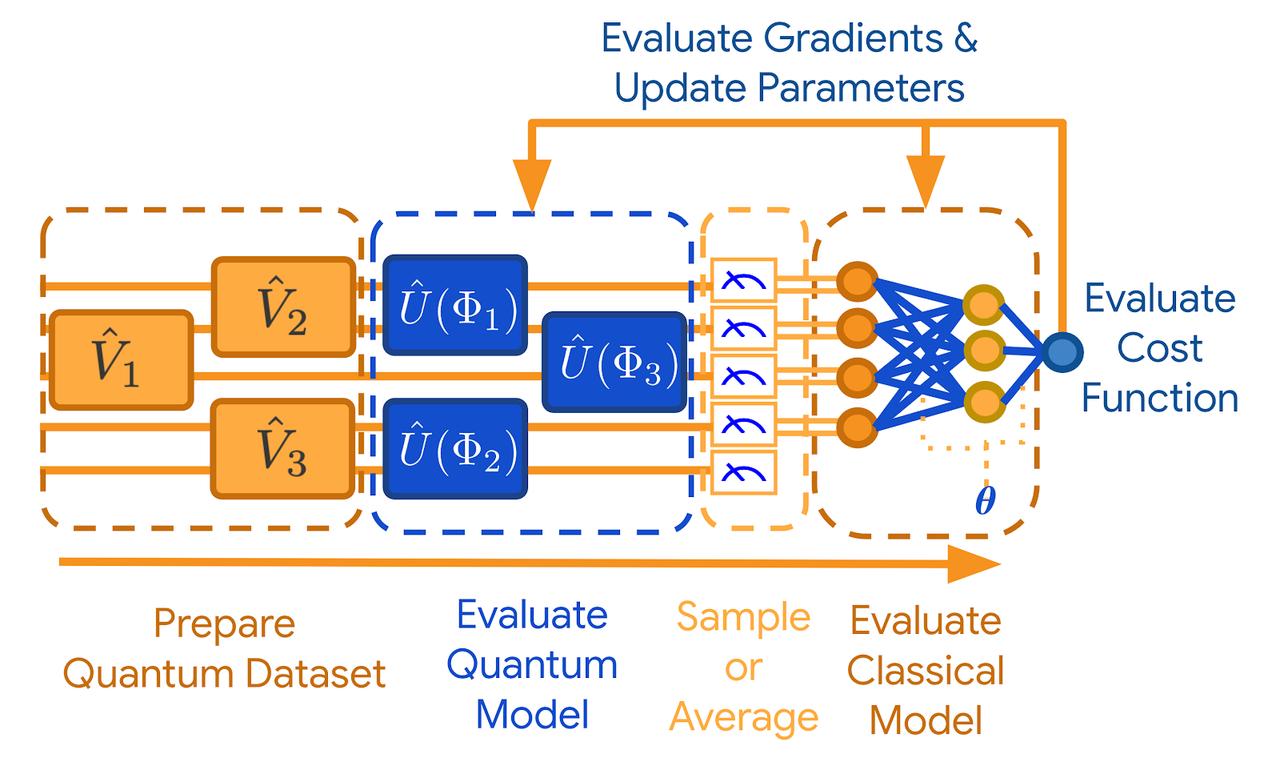
1 minute read
Whyquantummachinelearning
There are various reasons to use quantum computing in machine learning realm, few of the reasons are listed below:
Quantum computing can be used in Deep learning and machine learning problems to reduce the time taken train the deep neural network. Many authors are currently performing these techniques to solve the training time issue when considering the size of dataset and deep learning model architecture learnable parameters.
Advertisement
The quantum state of a qubit in a quantum computer is a vector in a 2a-dimensional complex vector space. In this area, numerous matrix transformations take place.
The Fourier Transform, identifying eigenvectors and eigenvalues, and resolving linear sets of equations over 2-dimensional vector spaces can all be solved by quantum computers in polynomial-time.
As discussed before about the eigen values and vectors, The computation of eigenvectors and eigenvalues is another problem with traditional computers. The set of related eigenvectors and eigenvalues increases in proportion to the input's higher dimensionality.
Quantum Random Access Memory (QRAM), which selects a data vector at random, enables quantum computers to handle this problem quickly and efficiently. It employs qubits to translate the vector into a quantum state. Logarithmic qubits are included in the summarized vector that results from quantum principal component analysis. A dense matrix is created by the specified random vector.