Group www.phdassistance.com
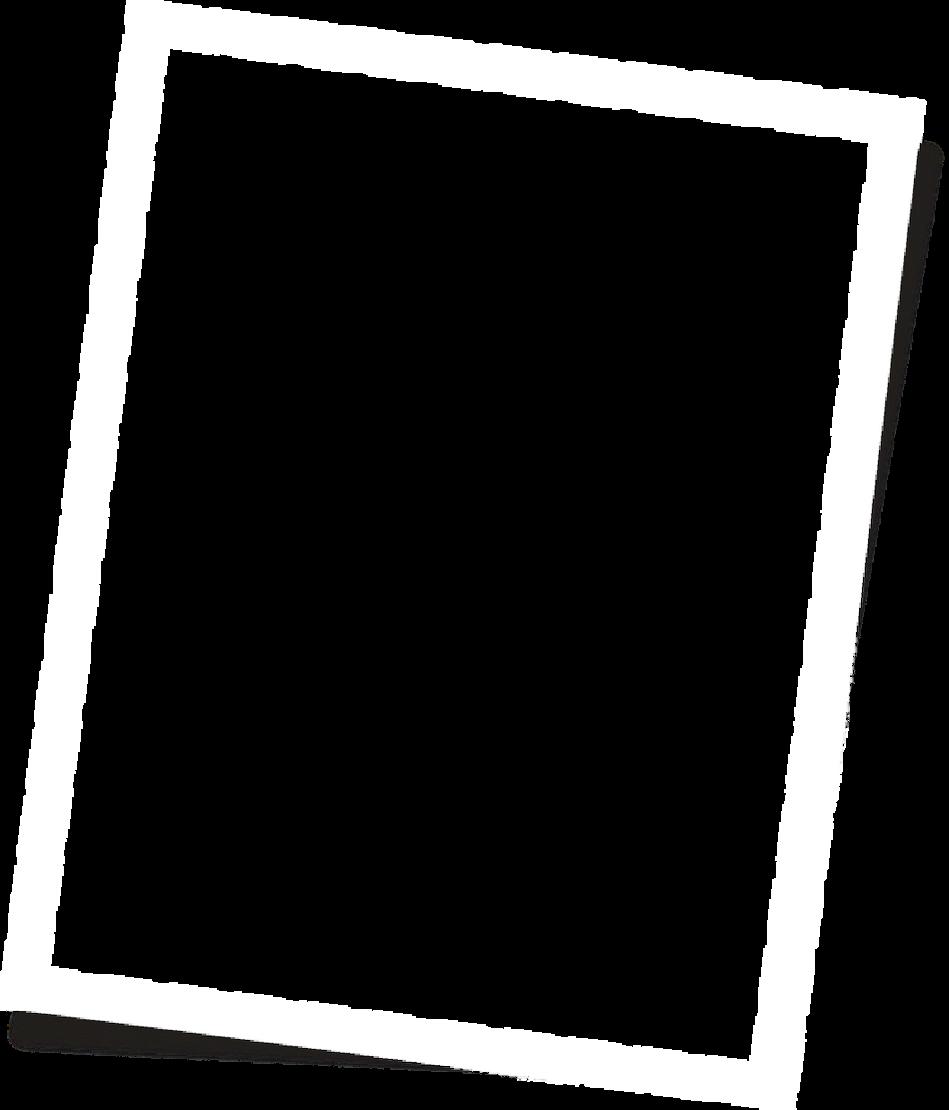
An Academic presentation by
Email: info@phdassistance.com
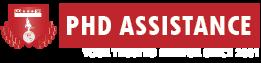
TheContributi MachineLearn inCybersecur
Dr. Nancy Agnes, Head, Technical Operations, Phdassi
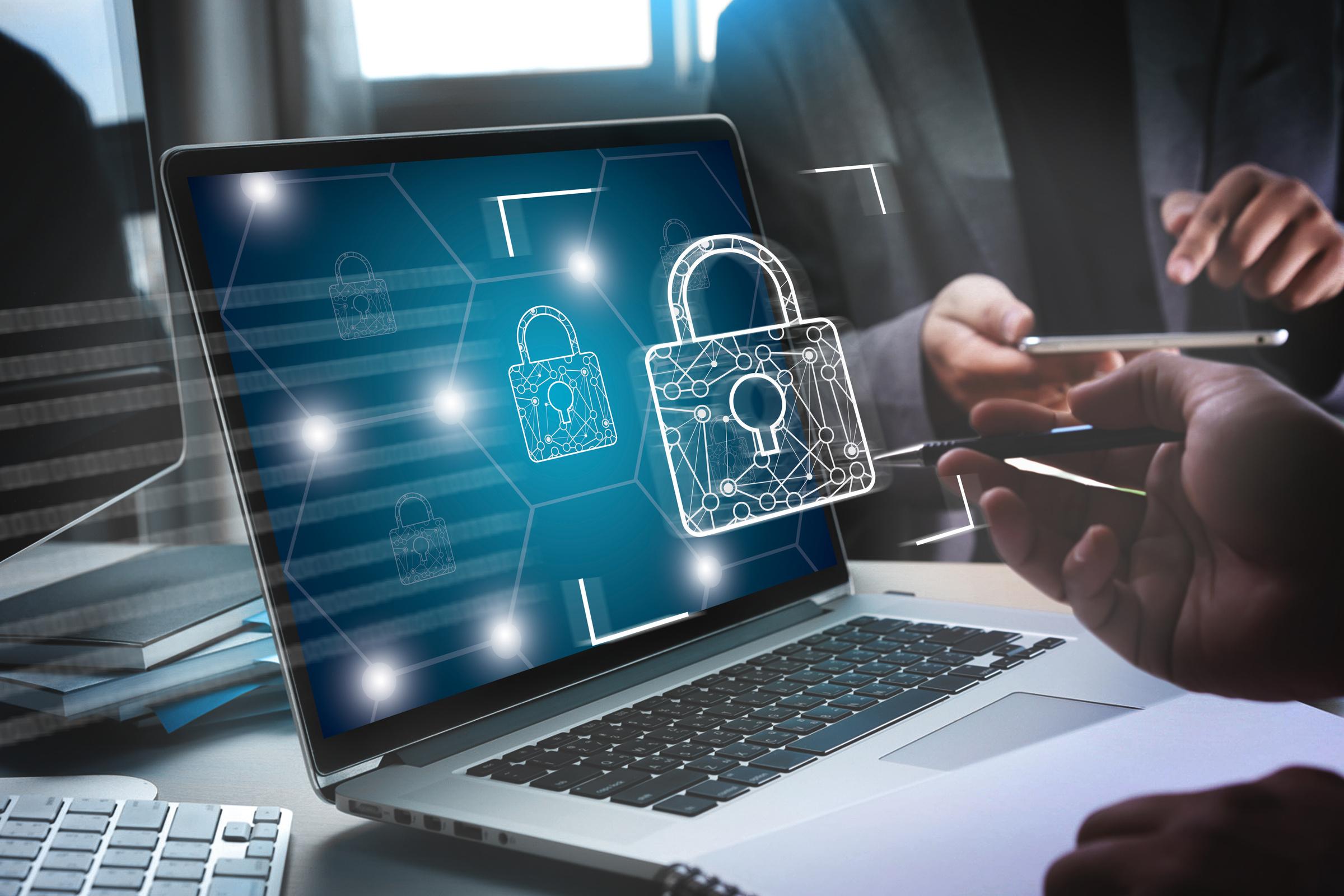
day'sDiscussion oduction hine Learning in Malware Detection hine Learning in Phishing Detection ond Detection: Additional Roles of hine Learning in Cybersecurity Future of Machine Learning in ersecurity clusion
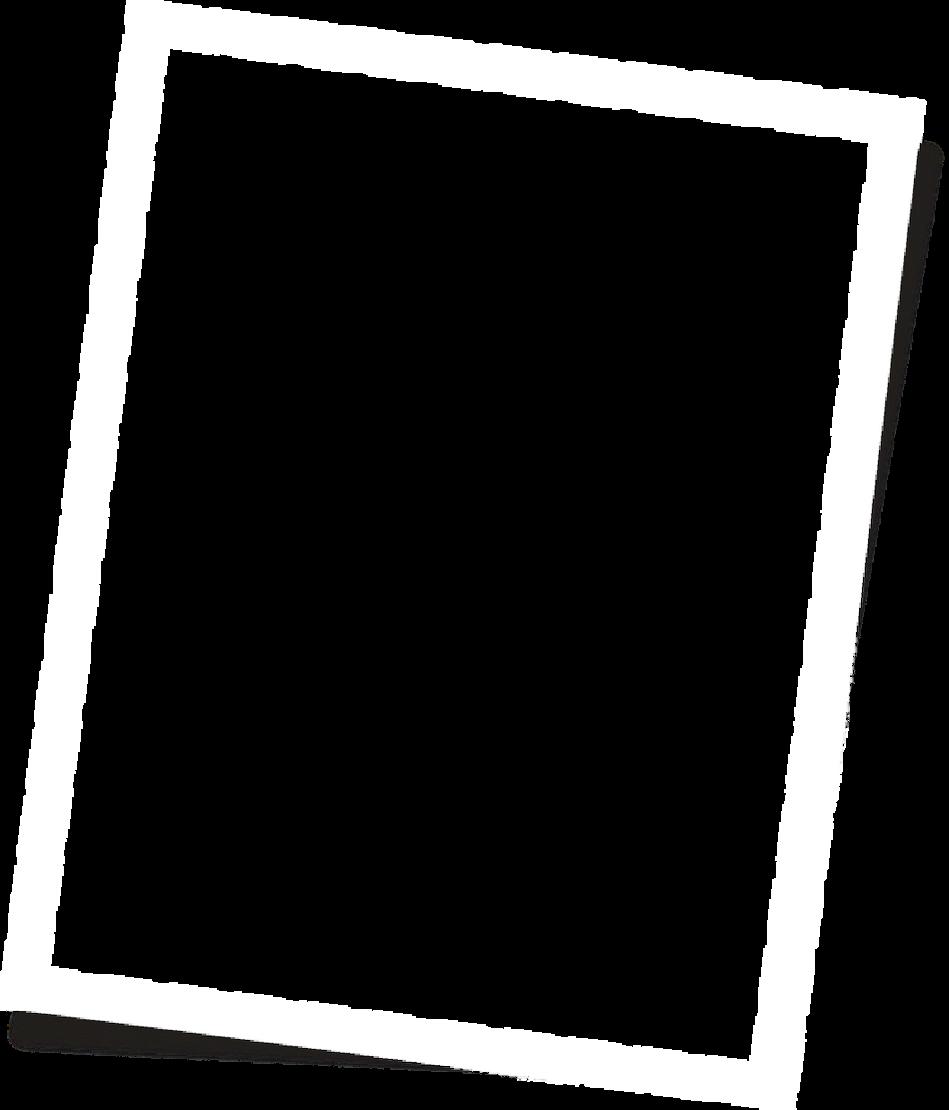
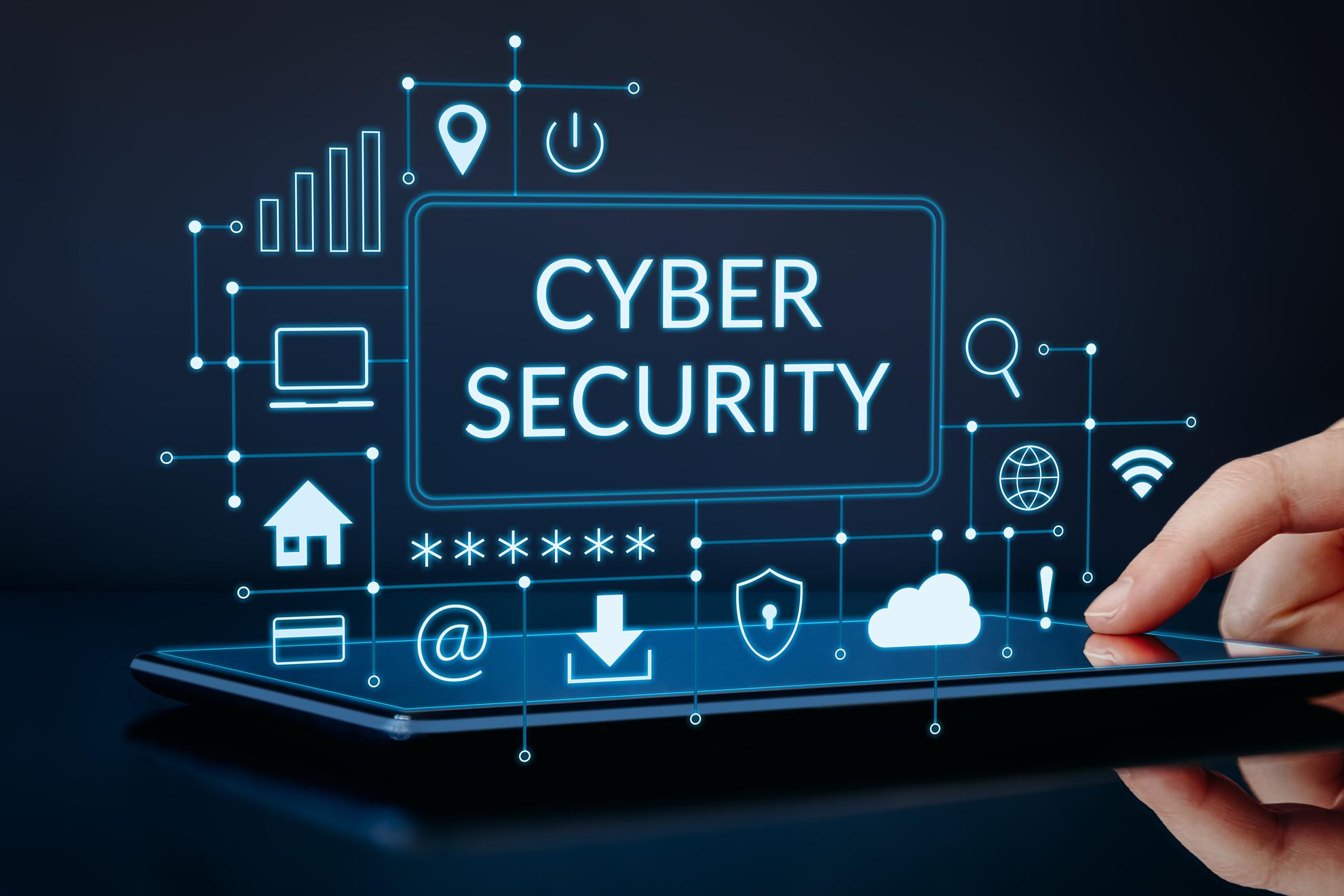
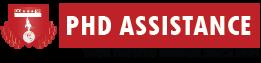
Introduction
The benefits of artificial intelligence (AI) are now broadly acknowledged as a result of the increasing complexity of contemporary informationsystemsandtheresultingeverincreasingvolumeofbigdata. Particularly with the emergence of deep learning, machine learning (ML) technologies are already being used to addressvariousreal-worldissues.
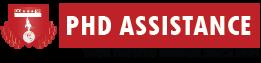
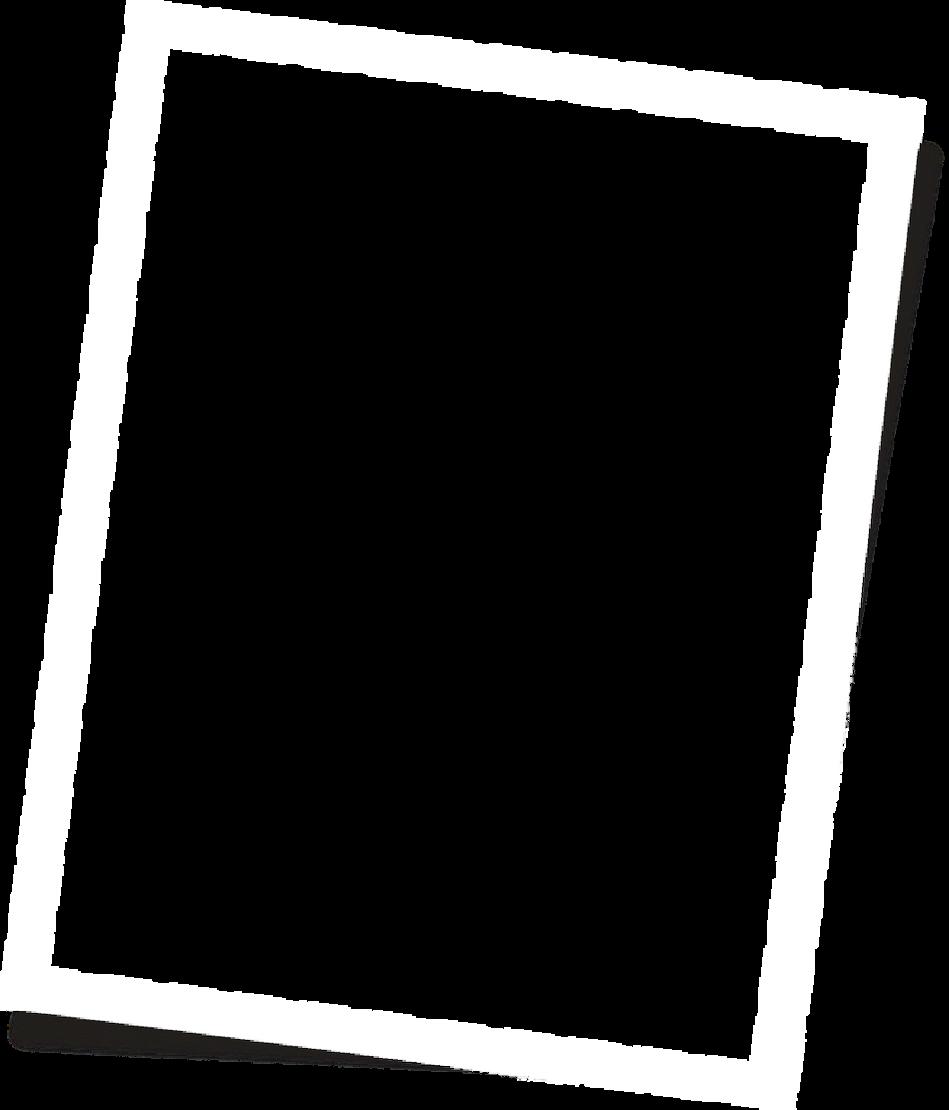
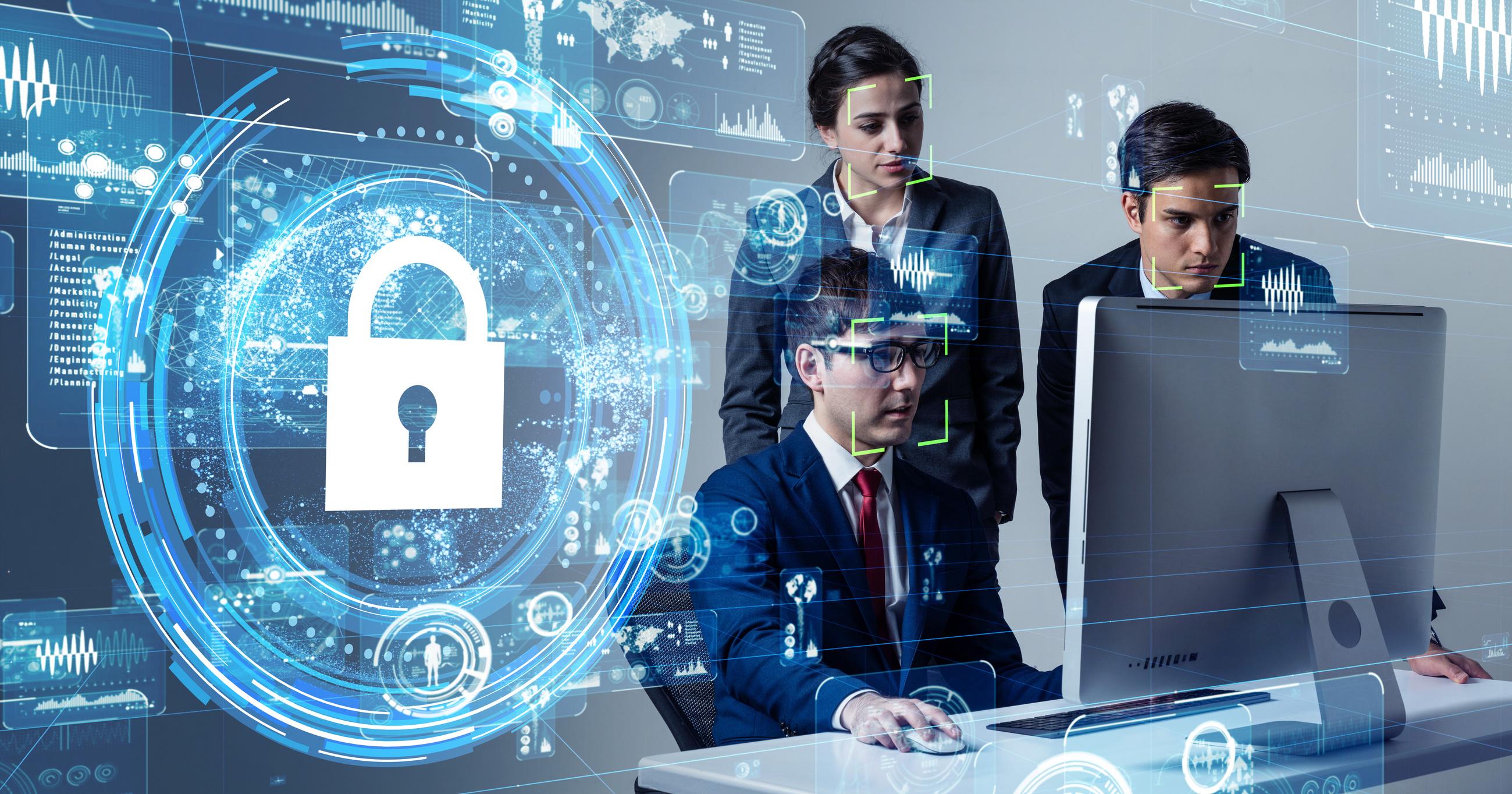
Additionally, ML is correctly regarded as a technology enabler due to the significant potential it hasdemonstratedwhenusedtoautonomousvehiclesortelecommunicationnetworks(Zhang etal.,2022).
MachineLearning,isakeytechnologyforbothpresentandfutureinformationsystems,anditis already used in many different fields. There is a huge gap between research and practise, but the application of ML in cyber security is still in its infancy. As a result of the current state of the art, which prevents recognising the function of ML in cyber security, this disagreement has its origins there. Unless its benefits and drawbacks are recognised by a large audience, ML's full potentialwillneverberealised.
Machine translation, travel and holiday suggestions, object identification and monitoring, and evenvariedapplicationsinhealthcarearefascinatingexamplesofthepracticalsuccessesofML.
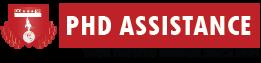
The latter call for developing a concept of "normality" and seek to identify events deviating from it under the presumption that such deviations correlate to security incidents. These two methods of detection work in conjunction with one another: misuse-based approaches are very accurate but can only identify known threats; anomaly-based approaches tend to raise more false alarmsbutaremoreeffectiveagainstnewattacks(Elsisietal.,2021).
Twoindependentmethods—misuse-basedandanomaly-based—canbeused todetectcyberrisks.Theformer,alsoknownassignature-orrule-based,calls foridentifyingparticular"patterns"thatrelatetoagivendangeronthegrounds thatsubsequentthreatswilldisplaythesamepatterns.
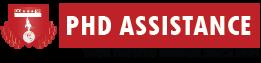
The former can serve as full detection systems but calls for labelled data that was developed under some degree of human oversight. The latter can only carryoutauxiliaryjobsanddonothaveahumanintheloop. Labelsmaybesimplertoobtaindependingonthesortofdatabeinganalysed; for example, any layperson can tell a valid website from a phishing website, whileitismoredifficulttotellbenignnetworktrafficfrommalicioustraffic.
The ability to use supervised or unsupervised ML algorithms is the distinctive feature of ML applications for cyber risk detection (schematically represented inFig.1).
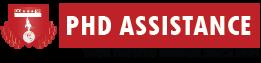
Figure1.ProsandConsofSupervisedandUnsupervisedMLforCyberThreatDetection.
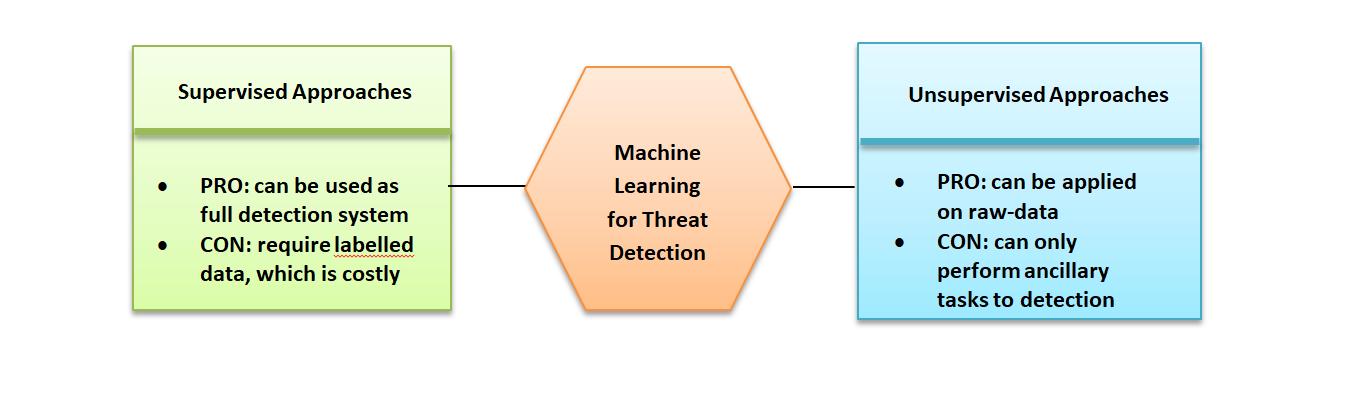
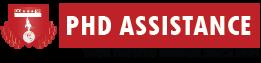
MachineLearningin MalwareDetection Oneofthemostrecognisabledifficultiesincybersecurityis the struggle against malware. Since malware only affects onetypeofdevice,itcanonlybefoundbyexamining data atthehostlevel,orthroughHIDS. Antivirus software can be viewed as a subset of HIDS, in fact. A particular malware version is designed for a certain operatingsystem(OS). Formorethan20years,malwarehastargetedWindowsOS themostduetoitswidespreaduse.Attackersarecurrently focusing their efforts on mobile devices running operating systemslikeAndroid(Annamalai,2022).
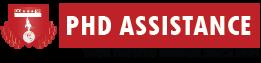
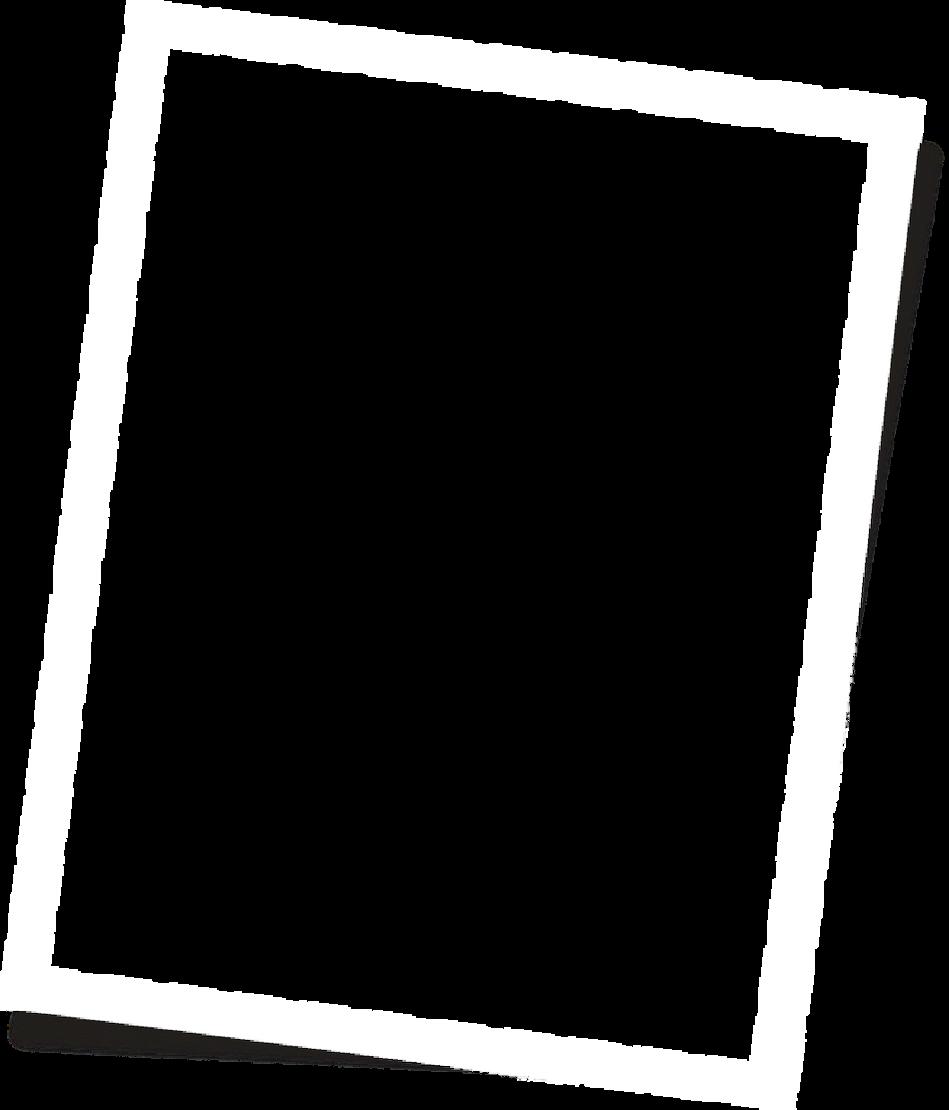
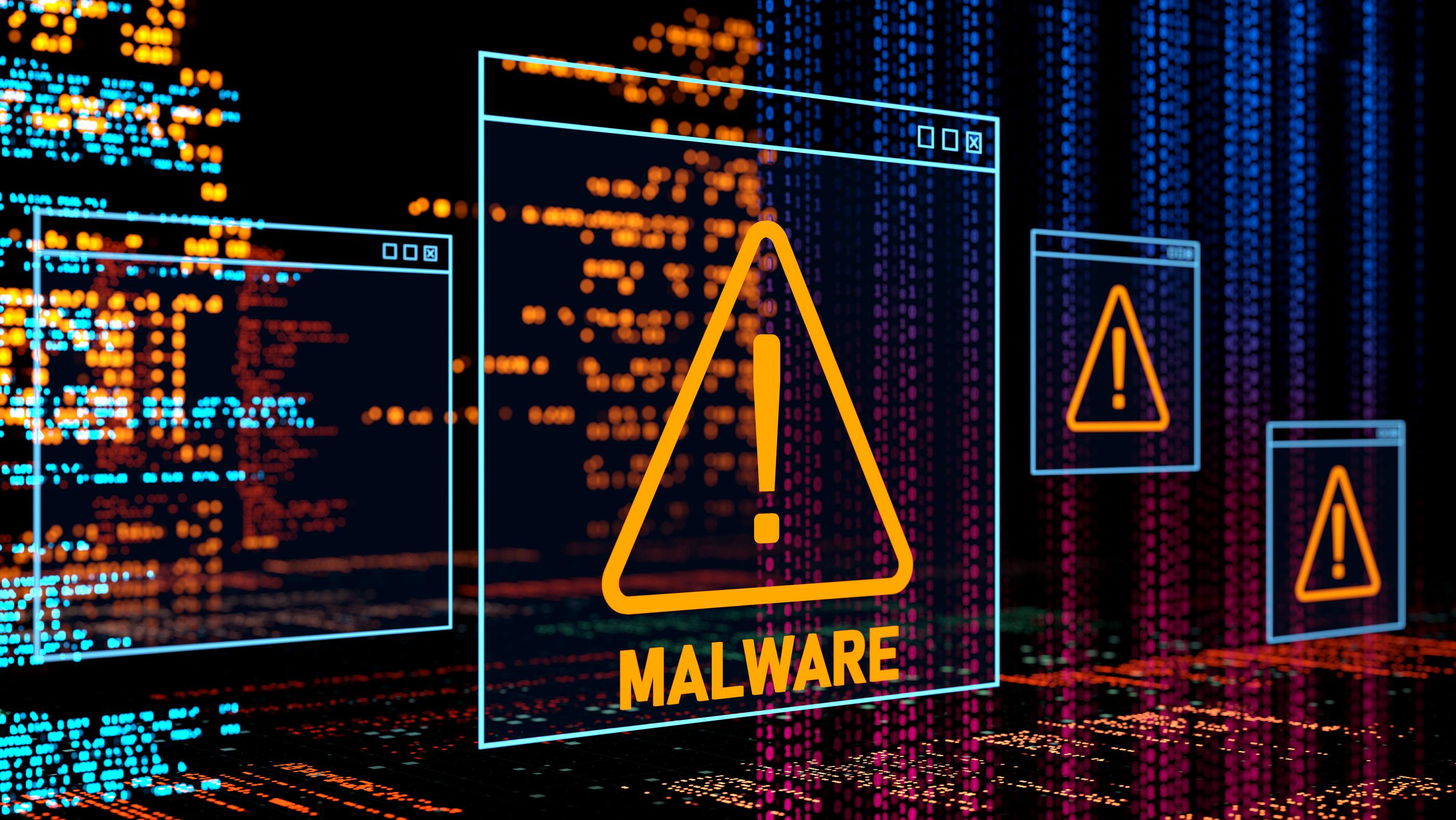
Both static and dynamic assessments are shown schematically in Fig. 2, can acquirefromML.
Static or dynamic studies can both be used to detect malware. By only examiningagivenfile,theformerseektoidentifymalwarewithoutrunningany code.
Thelatterconcentrateonexaminingapieceofsoftware'sbehaviourwhileitis being used, typically by setting it up in a controlled environment and keeping aneyeonitsoperations.
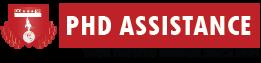
Figure2.MalwareDetectionviaML.
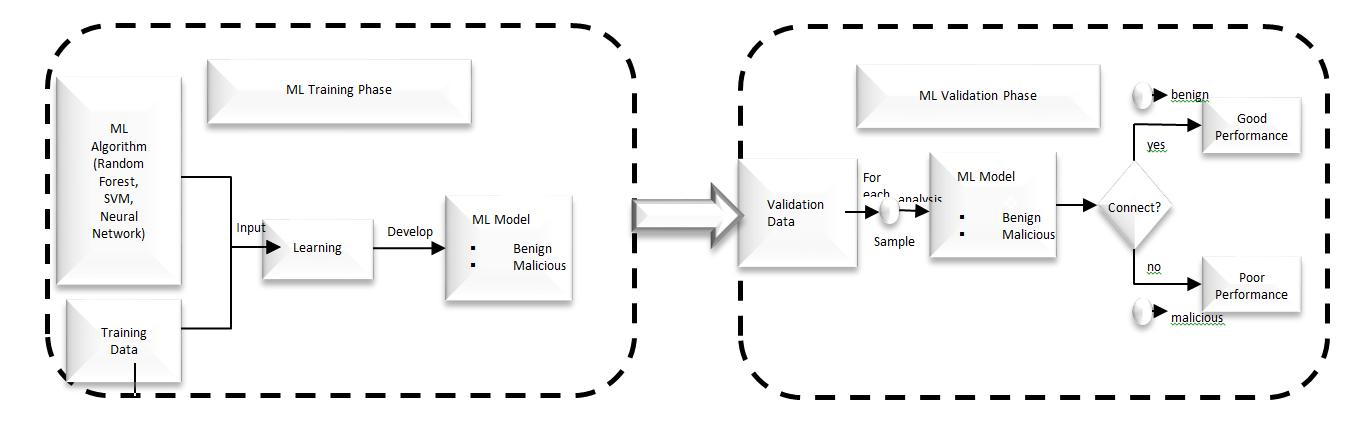
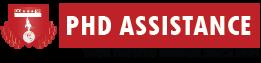
Both static and dynamic assessments are shown schematically in Fig. 2, can acquirefromML.
Static or dynamic studies can both be used to detect malware. By only examiningagivenfile,theformerseektoidentifymalwarewithoutrunningany code.
The latter concentrate on examining a piece of software's behaviour while it is being used, typically by setting it up in a controlled environment and keeping aneyeonitsoperations.
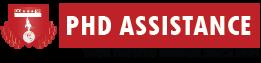
We specifically differentiate between two different uses of ML to detect phishing attempts: detection of phishing sites, where the aim is to identify web pages that are disguised to look like a legitimate website; and identification of phishing emails, which either point to a vulnerable website or stimulate a response that includes sensitive information (Geetha & Thilagam,2021).
One of the most frequent ways to infiltrate a target network is by phishing, which is still a seriousdangertoonlinesecurity.Modernenterprisesmustprioritisetheearlyidentificationof phishingefforts,whichcanbetremendouslyhelpedbyML.
MachineLearninginPhishing Detection
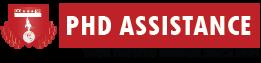
The primary distinction between these two methodologies is to the sort of data being analysed: although it is typical to examine an email's text, header, or attachments,itismorenormaltostudyawebpage'sURL,HTMLcode,orevenvisual representationsforwebsites.SuchapplicationsaredepictedschematicallyinFig.3.
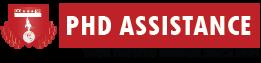
Figure3.PhishingDetectionviaML.
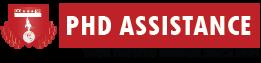
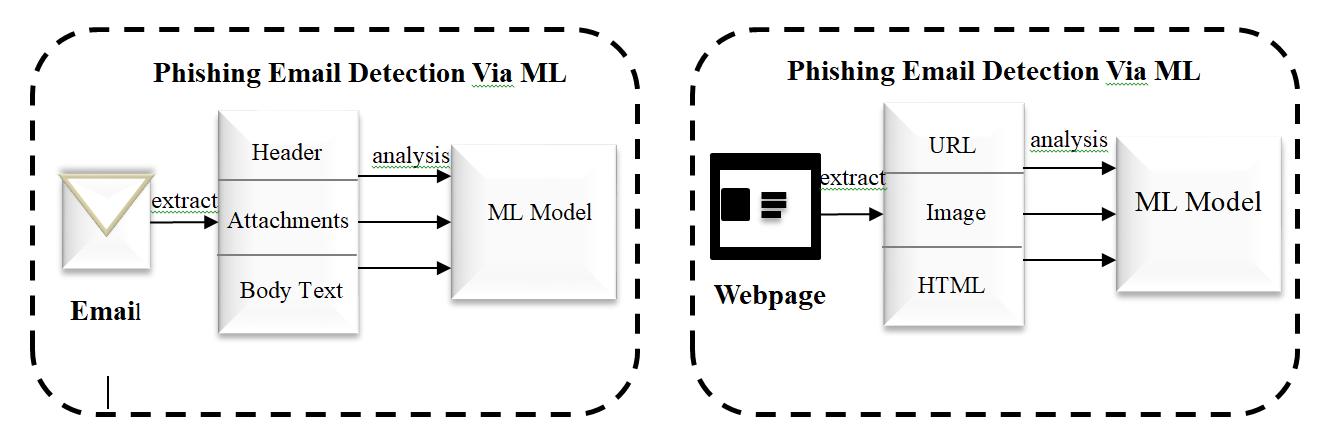
Cybersecurity
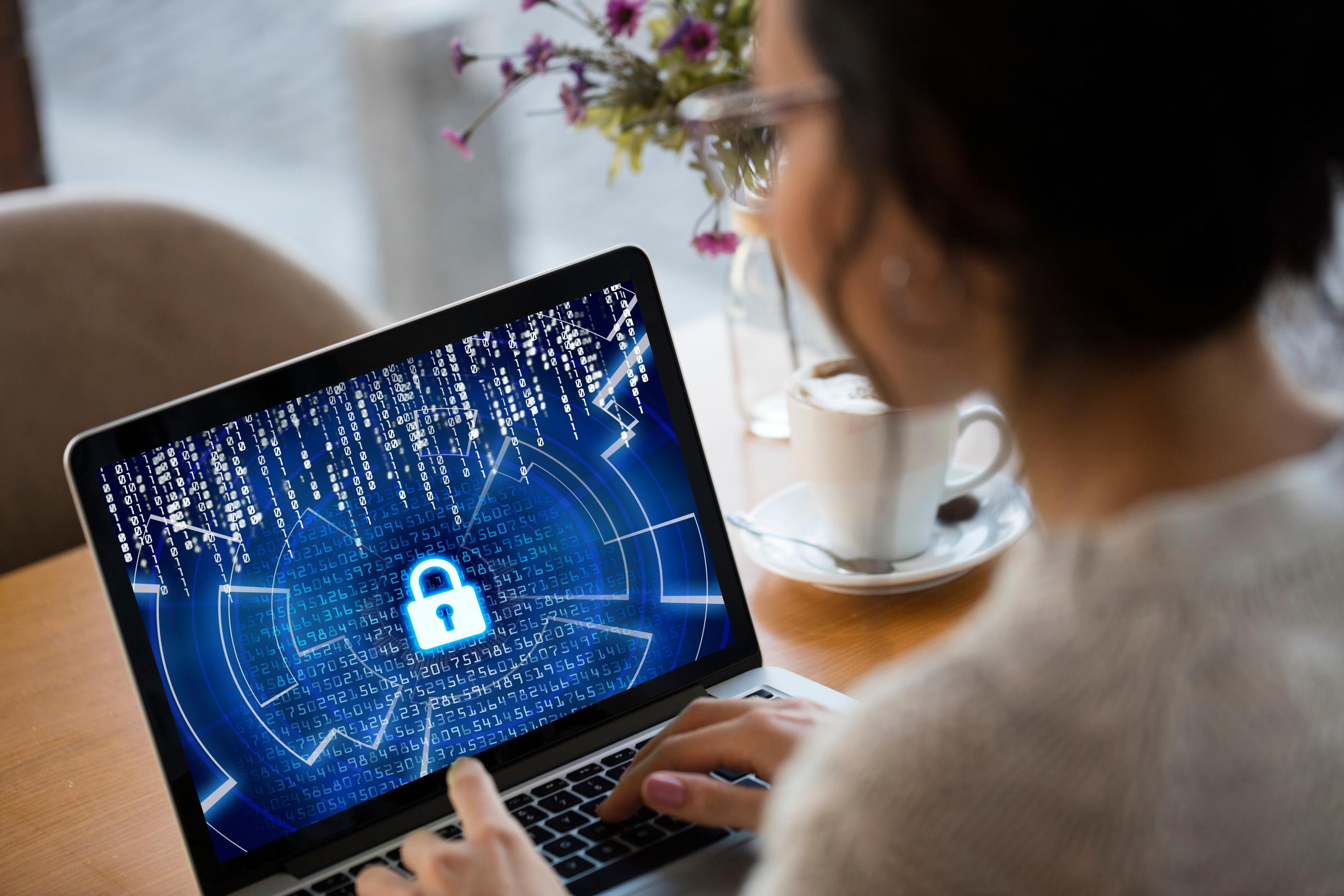
BeyondDetection: AdditionalRolesof MachineLearningin
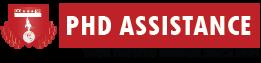
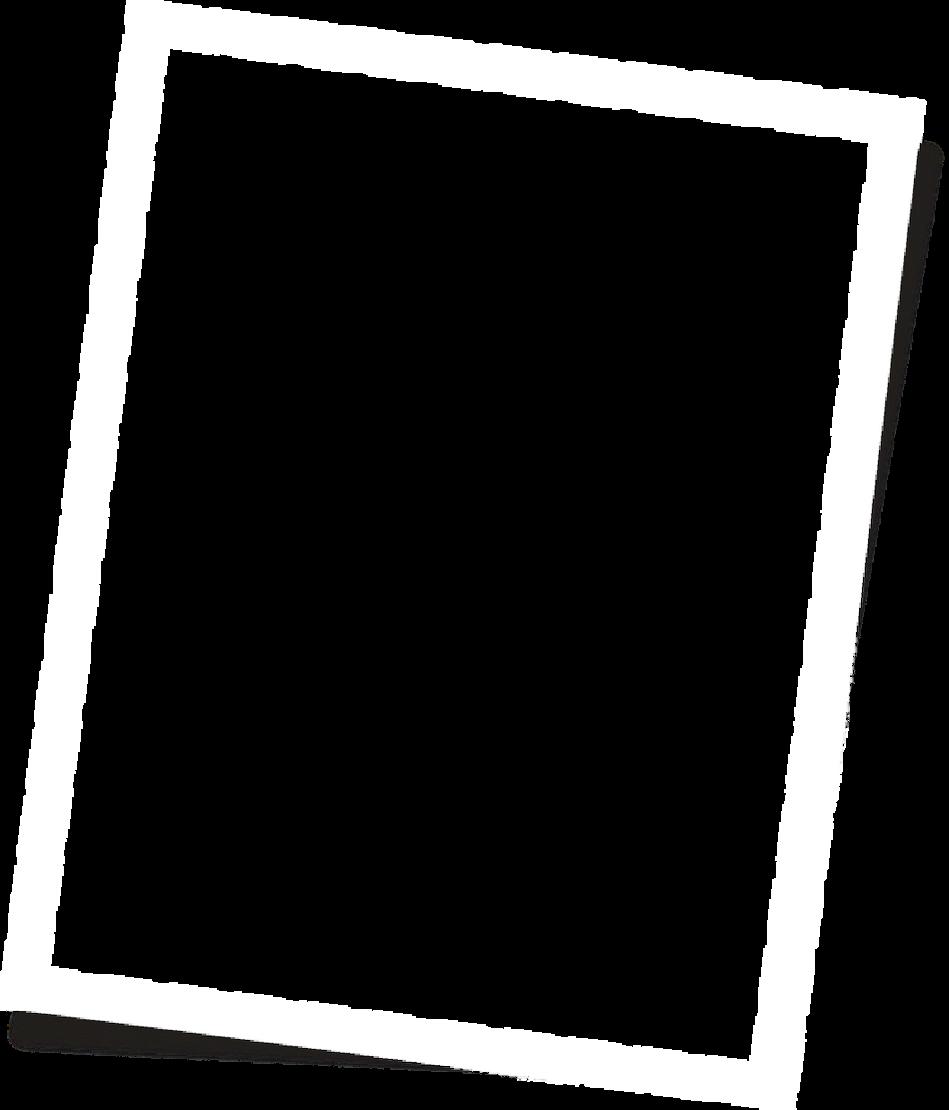
There are numerous other functions in cyber security that ML can fill in addition to threatdetection.
four tasks without losing generality: alert management, raw data analysis, risk exposure assessment, and cyber threat intelligence
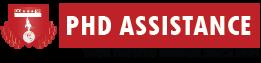
into (Hameed et al., 2021). Schematic representation of and is given in Fig. 7.
machine learning
threat detection
Modernenvironmentsdoindeedproduceenormousamountsofdataonaregular basis,andthesedatamayoriginatefromavarietyofsources,includingMLmodels. Byusing(extra)MLtoanalysethisdata,itispossibletogaininsightsthatraisethe securityofdigitalsystems.ResearcherscangroupallthesecomplementingMLjobs
Figure7.AdditionaltasksthatcanbeaddressedviaMLincybersecurity
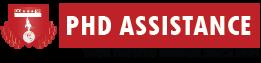
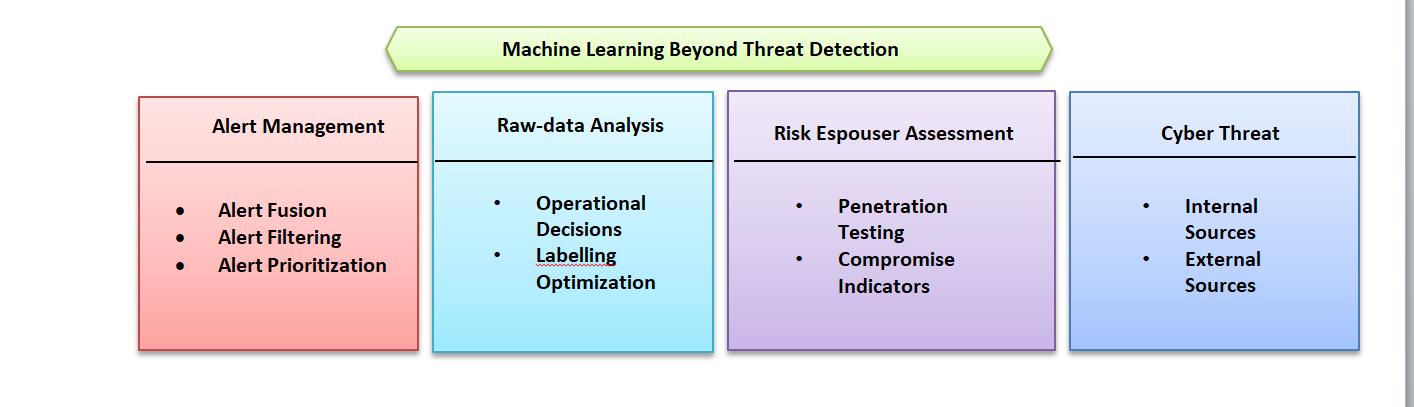
TheFutureofMach LearninginCybers
The state-of-the-art can be advanced in a c ways, including by improving current perf known problems (such the inability to expl creating cyber
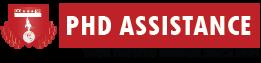
new ML-based
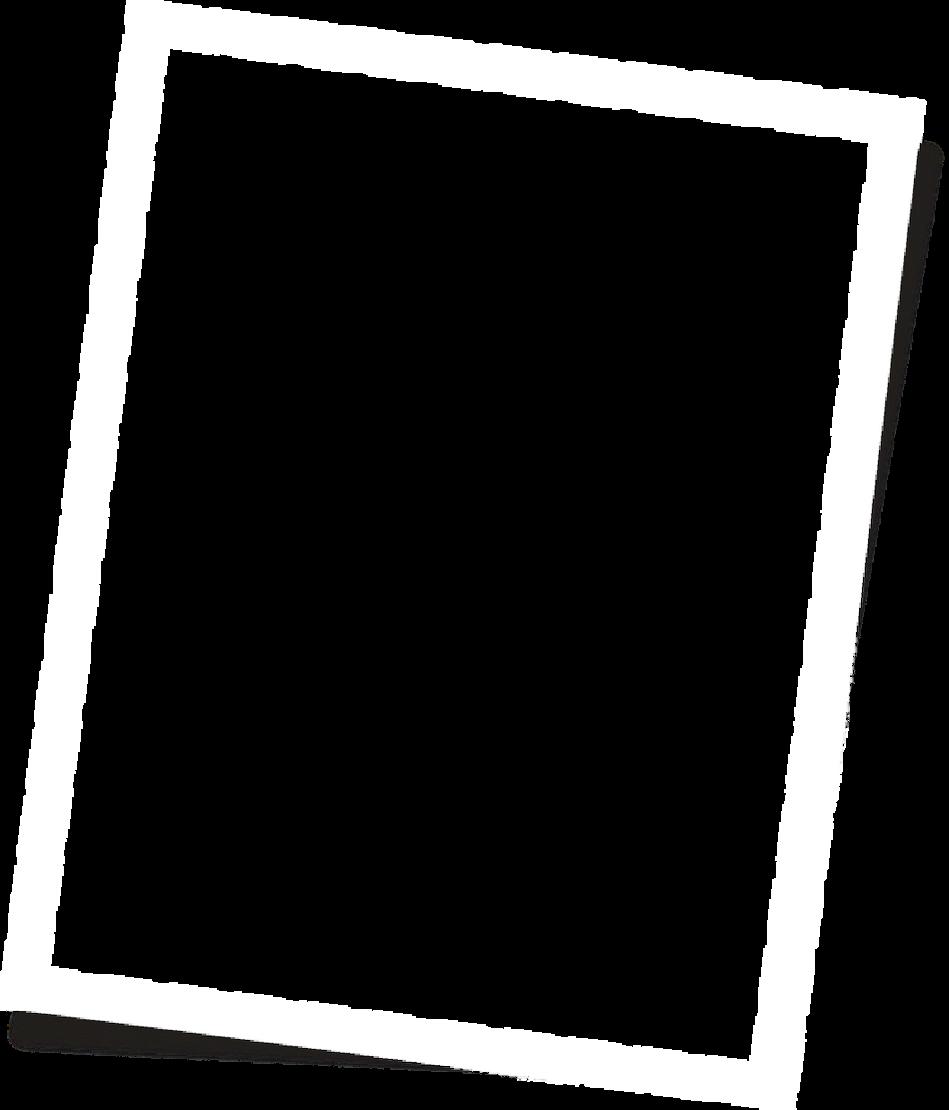
security integratingquantumcomputing). 6.1 Certification (Sovereign entities)transparency and reliability, regulatory bodie development and adoption of standardize certifytheperformanceandrobustnessofML
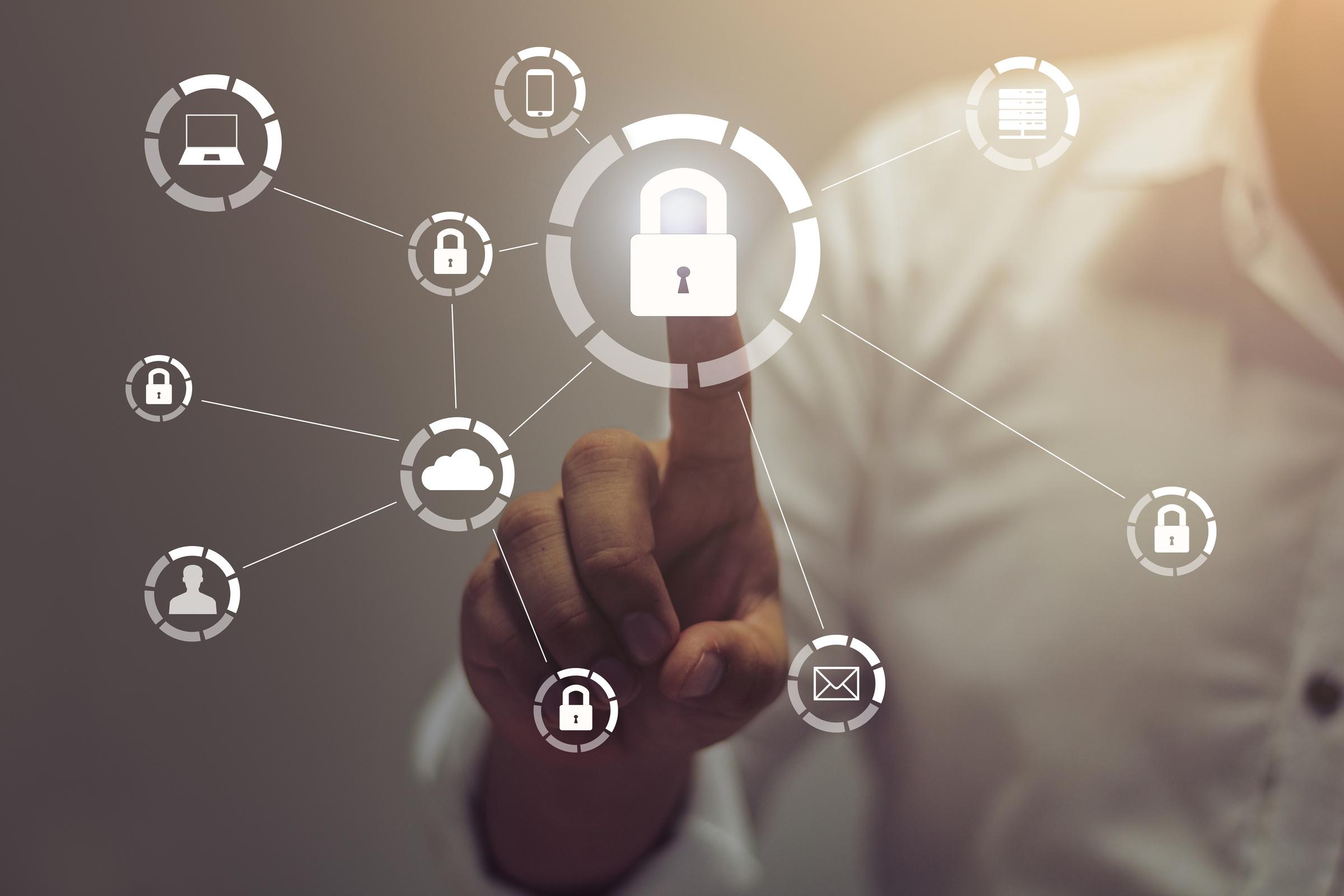
At the same time, such material should be evaluated to ensure its correctness potentially by a separatesetofreviewerswithmoretechnicalexpertise.
DataAvailability(executivesandlegislationauthorities)-Toaddresstheshortageofadequatedata, companies should be more willing to share data originating in their environments, whereas regulationauthoritiesshouldpromotesuchdisclosurebydefiningproperpoliciesandincentives.
Usable Security Research (scientific community) - The peer-review process should facilitate and enforcetheinclusionofthematerialforreplicatingMLexperiments.
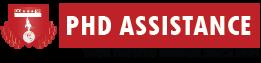
Orchestration of Machine Learning (engineers)
- Orchestrating complex systems that use (combinationsof)MLandnon-MLsolutionsisbeneficialforcybersecurity.
Hence,MLengineersandpractitionersshouldclearlyhighlighthowtocombineallsuchcomponents inordertomaximizetheirpracticaleffectiveness.
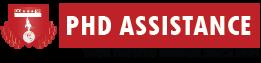
Informationtechnology(IT)systems,including autonomous ones that are also actively exploited by hostile actors, are being used by moderncivilizationmoreandmore.
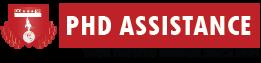
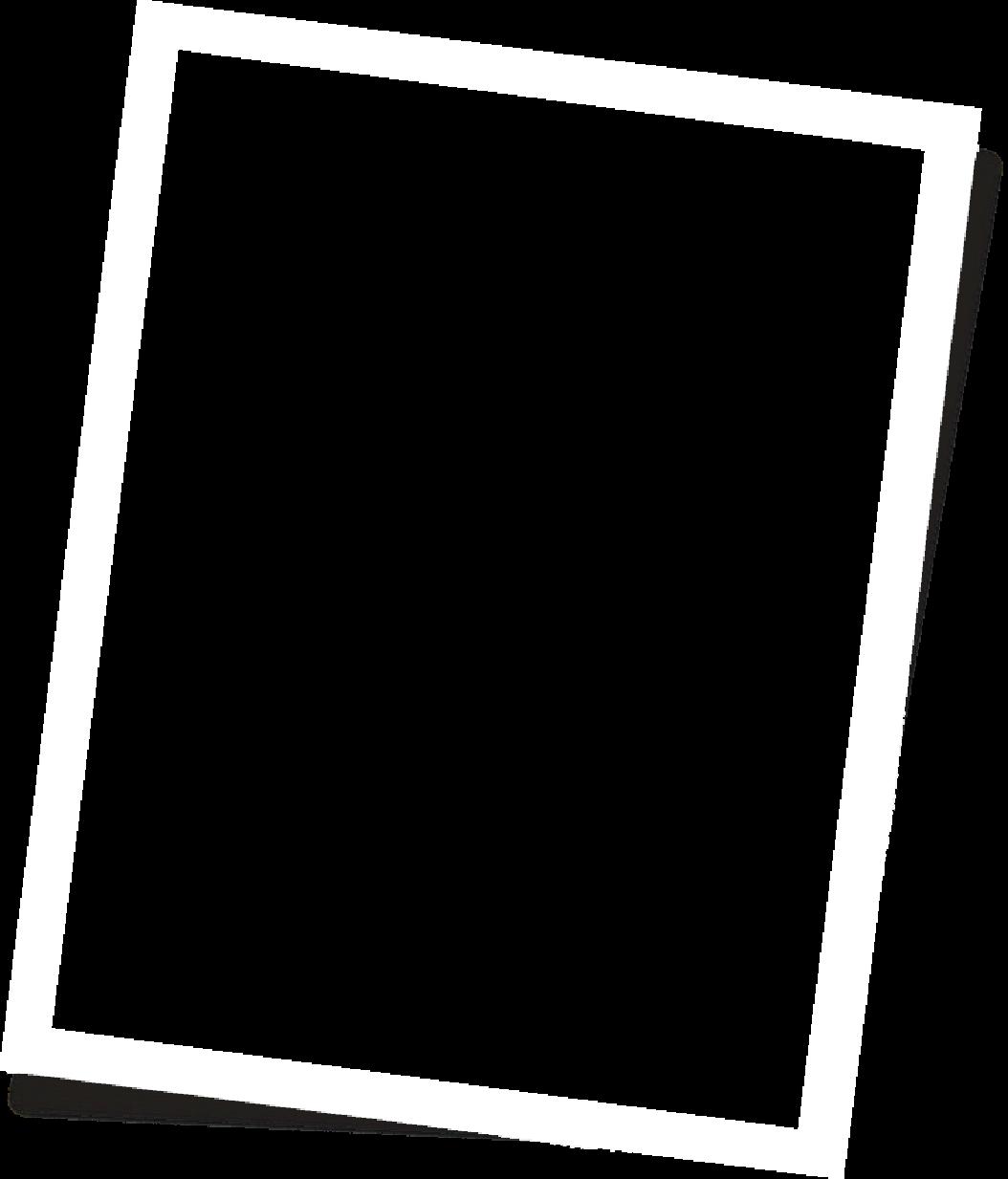
As a matter of fact, cyber threats are always changing, in the coming future attackers will have the means to seriously hurt or even kill people.
Conclusion
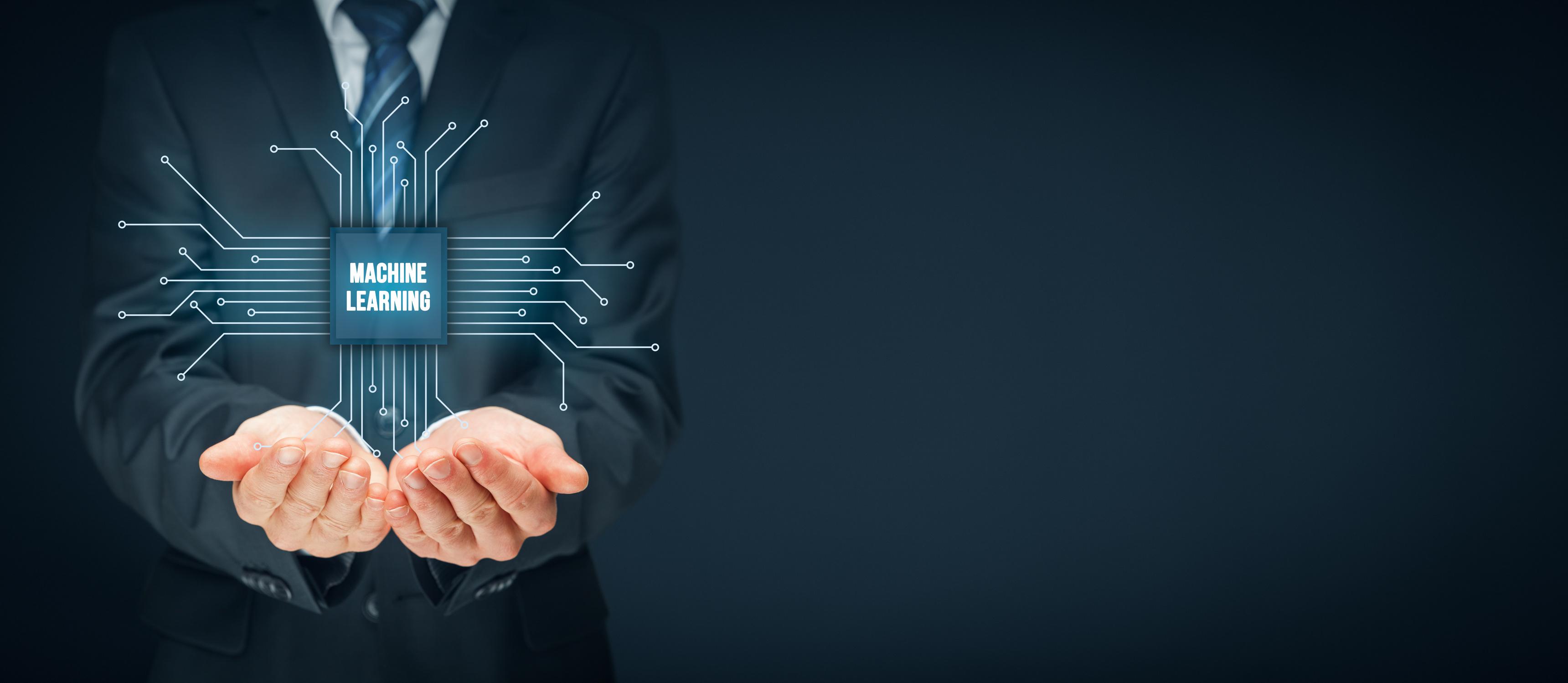
To establish the groundwork for a greater deployment of ML solutions to safeguard present and future systems, this log aims to stimulate significant improvements of machinelearning (ML) in the fieldofcybersecurity.
Defensive mechanisms need to have the ability to quickly adapt to the changing settings and dynamic threat landscape in order to prevent such incidents and reduce the myriad hazards that canaffectexistingandfutureITsystems.
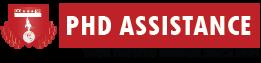
GET IN TOUCH +447537144372 UNITEDKINGDOM +91-9176966446 EMAIL INDIA info@phdassistance.com
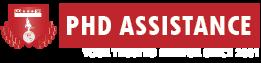
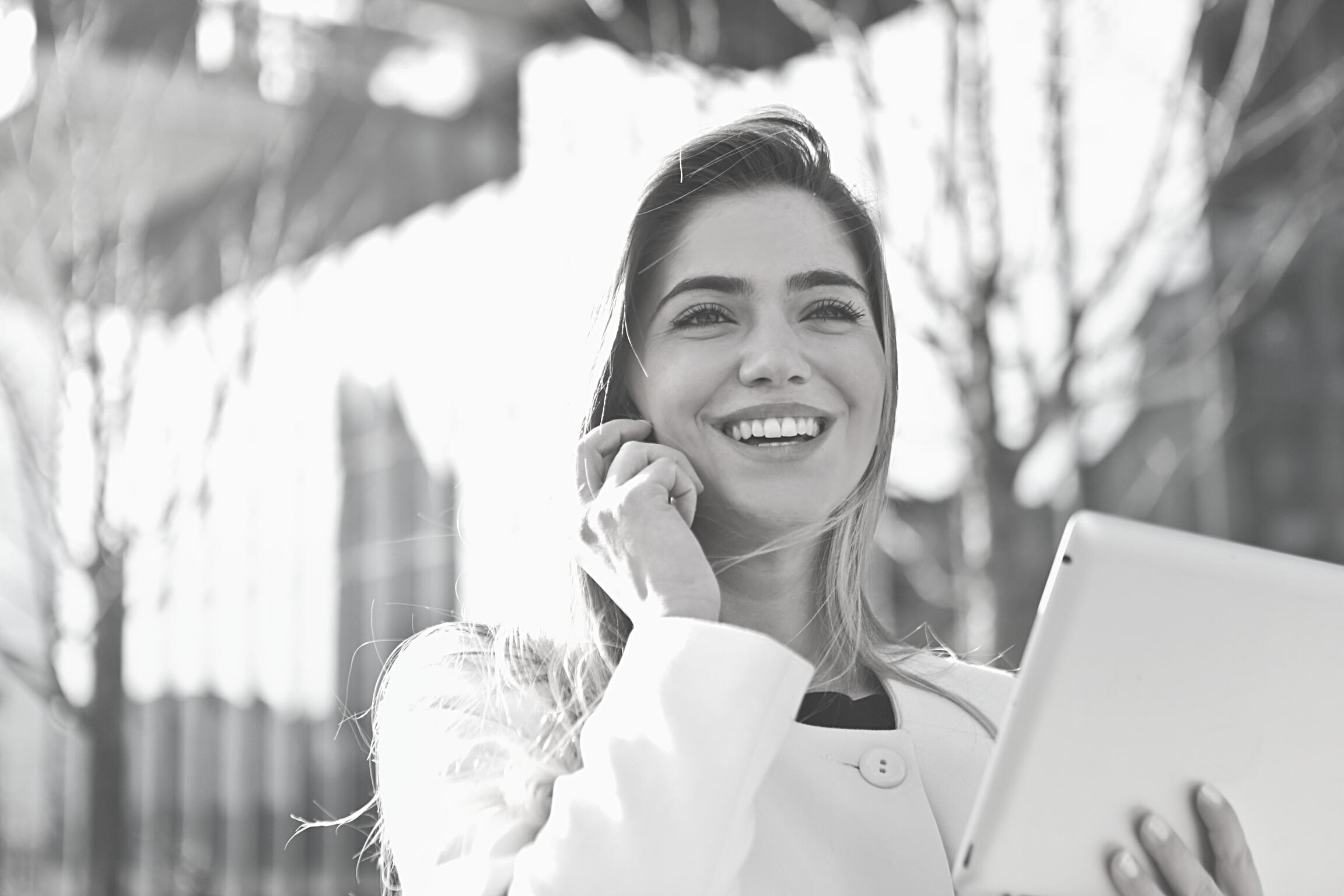