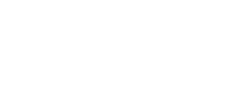
6 minute read
PERSPECTIVES
How Festo Applies Artificial Intelligence for Predictive Applications
By David Greenfield
Editor-In-Chief/Director of Content, Automation World

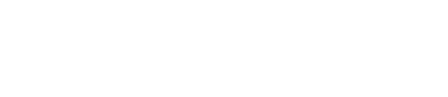
As part of Festo’s exhibit at the 2021 virtual Hannover Messe event, the company featured its Festo AX software, developed by Festo and Resolto (a Festo subsidiary specializing in artifi cial intelligence).
Dr. Frank Melzer, member of Festo’s management board for product and technology management, noted that Festo uses condition monitoring and machine learning to monitor the behavior of its customers' components, machines, and systems. “AI is the enabler that takes the automation business to a new level of effi ciency,” he said. “We continuously monitor machine data and check it against our AI model, which describes the good state of a component or machine. The AI algorithms then detect deviations from the normal state and can also predict them. Particularly in industrial intelligence, the mixture of algorithms paired with the specifi c expertise of an engineer is a decisive success factor for the implementation of AI-based automation applications.”
Highlighting the open architecture of Festo’s AI technologies, Dr. Melzer said Festo's AI technologies can “be easily integrated via IoT gateways and standardized protocols with other components.”
To help demonstrate how the use of AI can vary depending on its application, Festo presented two use cases in di erent industry verticals. In one application for the automotive industry, Festo explained how a German automobile manufacturing customer uses predictive maintenance to improve the maintenance and reduce the downtime of its servo-pneumatic welding guns. Data from the welding guns is continuously collected and evaluated using Festo’s AI algorithms to predict failures of the welding guns. Evaluation of this data allows failures to be identifi ed before they occur. According to Festo, this translates into about a quarter of the downtimes currently expe-
machines, and systems. “AI is the enabler that takes the automation business to a new level of effi ciency,” he said. “We continuously monitor machine data and check it against our AI model, which describes the good state of a component or machine. The AI algo- rienced in this company’s production operarithms then detect deviations from the normal tions being avoided and allowing repairs to be scheduled during non-production times. In the other application highlighted at the event, Festo used a predictive energy application of AI for a customer in the food packaging industry. Here, the packager's goal was to reduce the energy consumption of its pneumatically operated bottling plant to reduce CO2 emissions and costs. After examining the plant’s compressed air processes, Festo applied its C2M energy e ciency module to monitor compressed air consumption, provide information about possible leaks, and prevent the system pressure from falling below a defi ned standby pressure level. The C2M module includes a pressure regulator, on-o valve, sensors, and fi eldbus communication in a single unit. According to Festo, the application of the C2M module has worked so well for the customer that they now want to use the data collected by the C2M for potential predictive maintenance applications.
Highlighting the open architecture of Festo’s AI technologies, Dr. Melzer said Festo's
Festo uses predictive maintenance in the automotive industry to increase e ciency in the maintenance of servo-pneumatic welding guns by avoiding unforeseen downtimes and service and complaint costs. Source: Festo.
rienced in this company’s production operations being avoided and allowing repairs to be
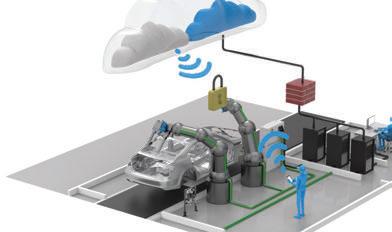
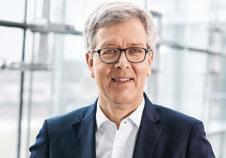
Simulation Platform for IoT Network Testing
By David Miller
Senior Technical Writer, Automation World
Among the biggest challenges of the Industrial Internet of Things (IIoT) is the installation, management, and integration of an unprecedented array of data-producing connected devices. The increased loads of data created by the IIoT’s proliferation of connected devices places a great strain on industrial networks.
To date, several technologies are being used to ease this burden. For example, MQTT (message queuing telemetry transport), an industrial communication protocol which uses a publish/subscribe transmission paradigm to limit the volume of data exchange on a network, has significantly reduced bandwidth usage. In addition, the rise of more sophisticated simulation technology—often driven by artificial
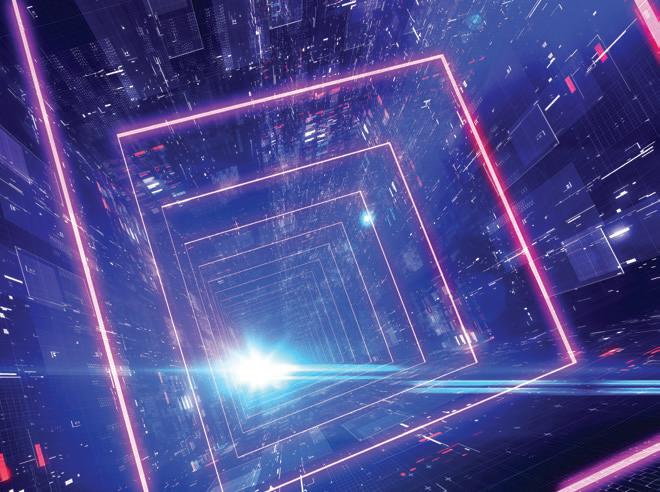
intelligence (AI) and machine learning—has allowed for explosive advances in the prediction and forecasting of issues that may arise within networked systems as they become more complex. This applies in many areas, including: Supply chain management, in which parallel planning for various possible scenarios has been made possible by virtual optimization models; predictive maintenance, where machine learning algorithms trained on large quantities of data can help operators anticipate equipment failures before they occur; and in the use of interactive digital twins that can help plant managers spatially optimize their facilities.
Now, the combined capabilities of simulation and MQTT are being extended to the testing of large-scale IoT networks via the use of Swarm, a new offering from HiveMQ, a provider of MQTT broker software. HiveMQ’s Swarm is a distributed platform that can create hundreds of millions of virtual network connections that simulate devices, messages, and MQTT topics. From there, the Swarm software is able to develop scalable and reusable scenarios that mirror real-world device behaviors.
According to Mary Brickenstein Ofschen, technical writer at HiveMQ, the custom scenarios created by Swarm allow end-users to scale the number of devices involved in a simulation by changing simple parameters and observing how individual assets will behave within the overall system as a result. This can help determine how to distribute a load intelligently across a network for the purpose of stress testing.
“For many companies, it is very important to understand exactly how their current development plans impact the entire system. For example, what will the backend infrastructure look like if the number of products sold each year doubles. The same is true for the industrial IoT space. Let’s say you want to expand your gateway infrastructure, add a new SCADA system, or increase the primary and secondary applications in your Sparkplug space,” Ofschen said. “You need to discover the potential bottlenecks in your system, but it’s hard to do. The ability to build reusable, declarative scenarios that HiveMQ Swarm provides [means that you] can build scenarios that truly match your planned use case, put the scenarios on version control, and copy and modify your testing parameters as needed. You can even build your own library of different test scenarios and use them to automate the testing of your entire solution.”
Swarm features multi-cloud functionality and can also be deployed on a local machine with no additional requirements. Currently, the software supports up to 10 million MQTT connections. In addition to its simulation capabilities, Swarm offers built-in monitoring, logging, and reporting, as well as REST API compatibility, making it possible to integrate with a centralized infrastructure.
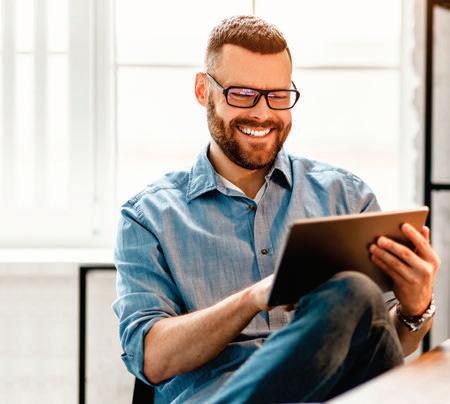
XX Ultrasonic Sensors with NEW easy-to-use software. Ideal for level detection & control, mobile equipment, material handling and hoisting applications.
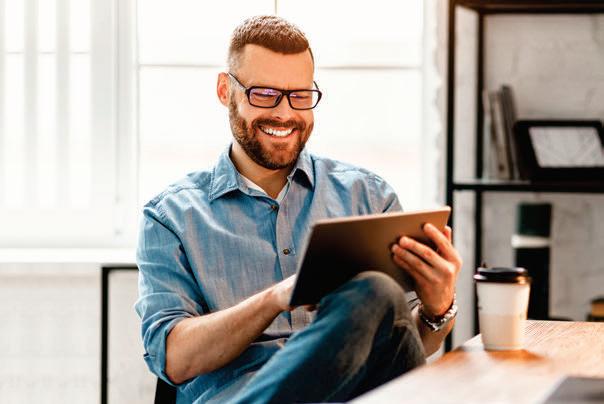
WOW! That was “Simply easy!”
This engineer just set up several ultrasonic sensors for a new machine line. Despite the varying ranges he had to set, he used a single software application. He set the distances. He adjusted gain. He filtered out anomalies. And those settings will remain for future replacement sensors.