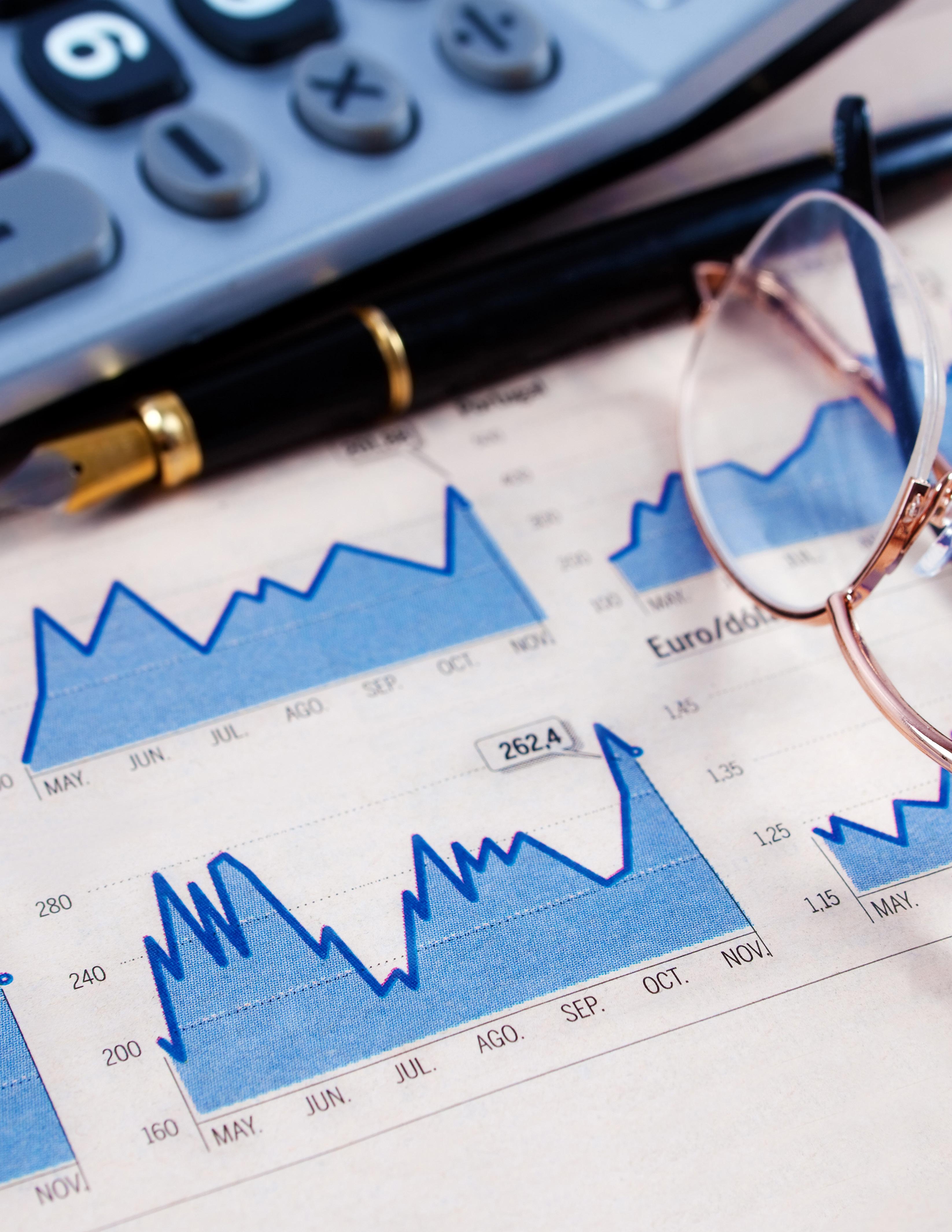
7 minute read
Making sense of models: a parsimonious solution by Dr. Gary Nan Tie & Dr. Bob Mark
making sense of models: a parsimonious solution
by Dr. Gary Nan Tie & Dr. Bob Mark
Advertisement
models shape decisions
We all need to understand how scientists, economists and doctors use models. This is especially true today since models are used to guide our public health policy for pandemics, and we are in the early stages of receiving vaccinations against the SARS-CoV-2 virus1. A subway map, a wind tunnel aircraft mockup, an architect’s virtual preview are simple examples of models. A more sophisticated model consists of mathematical distillations of relevant features of a problem that we want to solve.
Mathematical models guide us in understanding and predicting actual behavior for the purpose of making a decision. In particular, a mathematical model is a flexible mechanism which is adaptive and enables inference which:
Mathematical models enable both qualitative and quantitative reasoning. Quantitative reasoning endeavors to numerically estimate a quantity along with how much give and take there is in an estimate. Qualitative reasoning typically describes how networks of relationships interact with one another, inferring possible cascades of consequences that are directionally correct but not necessarily temporally or quantitatively accurate. Qualitative models may arise, for example, because of a lack of data to parameterize a model but their logic is nonetheless useful.
• can explain and predict phenomena • is consistent
• can be vetted
• is reproducible • can be updated upon arrival of new information
• allows us to extrapolate • articulates our risk performance • can be perturbated and stress tested • permits ‘What-if?’ investigation • allows comparison with alternative views
• guides rational decision making
1 / As we go to press, more than 245 million doses have been administered across 107 countries, according to data collected by Bloomberg. The latest rate was roughly 6.79 million doses per day. See https://www.bloomberg.com/graphics/covid-vaccine-tracker-global-distribution/
2 / See Nan Tie, G. & Mark, B., Sept 2020,’Parsimony;’A Model Risk Paper’, PRMIA Institute
what is a parsimonious model
We had touched upon the difference between statistical dependence and causation in our parsimony paper but it’s worth reminding ourselves of the ramifications. Anecdotally, we can observe the correlation between the kitchen barometer and the weather outside. We all know that changing the barometer does not change the weather3 .
Selecting a germane model which informs us in the context of decision making under uncertainty calls for a model that faithfully describes historic behavior and is robustly predictive. Moreover, the model needs to be neither too simplistic nor overly elaborate but be just right4! Experts need to be able to clearly communicate model results.
A layperson does not need to know how the watch was made in order to tell the time. Most can relate to receiving some medical test results and asking ourselves ‘Is this good or bad?’ Some steps for better communication of model results include:
1. Provide intuition, by analogy or simple example.
2. Stress the significance of results. How concerned should we be?
3. Put things in perspective. Relative to other risks, where is this?
4. Are the results actionable? What do we do with this information?
5. Give a recommendation with caveats and describe how one arrived at this conclusion.
We now explore in more detail the notion of parsimonious model selection that is fit for purpose5. Models are used to explain data as well as to make predictions. Data is usually collected from observations or experiments. Associated with each data point is a label value or outcome. Put another way, each input is associated with an output.
The prediction problem is to construct a function from inputs to outputs which behaves reasonably on existing inputs and will make plausible predictions of outputs on as yet unseen inputs. The reasonableness and plausibility are where parsimonious model selection comes in.
A regression problem is one in which the output values are from an interval, for example numbers between 6.5 and 29. When the output values are a discrete handful, like 0 and 1, we call this a classification problem. Notice the quantitative nature of regression (we care about the precision of the output) and the qualitative nature of classification (we care about the correctness of the output).
3 / In general, we could estimate observational probability p where p(Y |X = x) is the distribution of Y given that we observe X taking on the value x. We can jointly observe X and Y at random times to estimate p(Y| X) = p(X,Y)/p(X). Y and X are statistically dependent but Y is not necessarily caused by X, so setting X to x may not affect the distribution of Y. 4 / Ibid Nan Tie, G. & Mark, B, ’Parsimony, A Model Risk Paper’ 5 / For example, in the commons problem we can ask fit for whom?
Moreover, in terms of fitness for purpose, many machine learning applications naturally fall into either regression or classification type problems.
Machine learning algorithms typically construct the best fitting function from inputs to outputs from a given class of functions6. So, although we can explicitly describe what the algorithm is doing, we may not necessarily have intuition about the resultant function chosen. Communication of model results can begin with narratives such as analogies and heuristics. The narrative should be followed by a summary analysis of the algorithm giving perspective and context. In particular, the analysis should highlight the significant differences to alternative approaches with numerical examples demonstrating the sensitivity of results to perturbation, and conclude with recommendations, caveats, and applications.
To illustrate these ideas, suppose for example our purpose was to understand the economic impact of potential interventions to temper the number of people infected with the coronavirus, so as not to overwhelm hospitals. There is a spectrum of risk. One extreme is do nothing, the epidemic spreads exponentially, hospitals are overwhelmed, and many die as a result of lack of hospital beds and ventilators. The other extreme is a prolonged lockdown, people lose their jobs, demand for goods decline, businesses fail, and a recession sets in. Neither risk is desirable, perhaps there is a middle ground, because the health of people and economic prosperity are linked. For example, too many sick employees reduce the workforce and productivity.
What if core workers were allowed to keep the economy alive and non-core workers temporarily locked down to reduce the spread of infection? Fortunately, authors at Cambridge University in partnership with two researchers at the US Federal Reserve7, combined macroeconomics with aspects of epidemiology in a model to study the economic consequences of social distancing. They found there is no absolute tradeoff between the economy and human health. It is possible to have your cake and eat it too. A parsimonious model allows you to make an optimal decision in advance to avoid adverse outcomes as opposed to an afterthe-fact seat of the pants knee jerk reaction.
A structured lockdown is smart by parsimoniously balancing economic and health intervention. Yes, all models are wrong in the sense they are representations of reality. Nonetheless, they are useful in making policy. Smart lockdowns are possible. It is not a binary choice.
The scientific method of systematic observation, measurement, and experiment, and the formulation, testing, and modification of hypotheses as new information becomes available also applies to mathematical modeling.
lockdown – a parsimonious example
what we can do
6 / For the technically inclined, the Representer Theorem.
7 / Bodenstein M., Corsetti, G.and Guerrieri L., 2020, ‘Social Distancing and Supply Disruptions in a Pandemic’, Cambridge-INET Institute Working Paper Series No: 2020/17
As new data arrives, we can update or rethink models. So, all models are provisional and how we select a model fit for purpose is on the basis of parsimony. As we pointed out earlier, not too hot, not too cold, but just right is the key to finding a parsimonious solution.
Mathematical models are being used to make decisions, sometimes on your behalf without your knowledge. We all need to be savvy consumers and educate ourselves by critically asking questions, scrutinizing evidence, examining chains of reasoning, checking that model selection is parsimonious and fit for purpose, appreciating whether model results are qualitative or quantitative and most importantly realizing model results are provisional. Things change, as can our minds!
authors
Dr. Gary Nan Tie
Mu Risk LLC
Dr. Gary Nan Tie engages in cross-disciplinary mathematical research, discovering connections across disparate fields to bring new insight in bridging theory with practice. In the beauty of nature there is wisdom. Always the beginner’s mind!
Dr. Bob Mark serves on several boards, led Treasury/Trading activities and was a Chief Risk Officer at Tier 1 banks. He is the Founding Executive Director of the MFE Program at UCLA, co-authored three books on Risk Management and holds an Applied Math PhD. Bob is a cofounder of PRMIA and GARP Risk Manager of the Year.
Dr. Bob Mark
Managing Partner, Black Diamond Risk Enterprises
PRMIA Sustaining Members are invited to read more on this topic by downloading the complete paper Parsimony – A Model Risk Paper.
If you are not currently a Sustaining Member and would like a copy of the paper, join now.