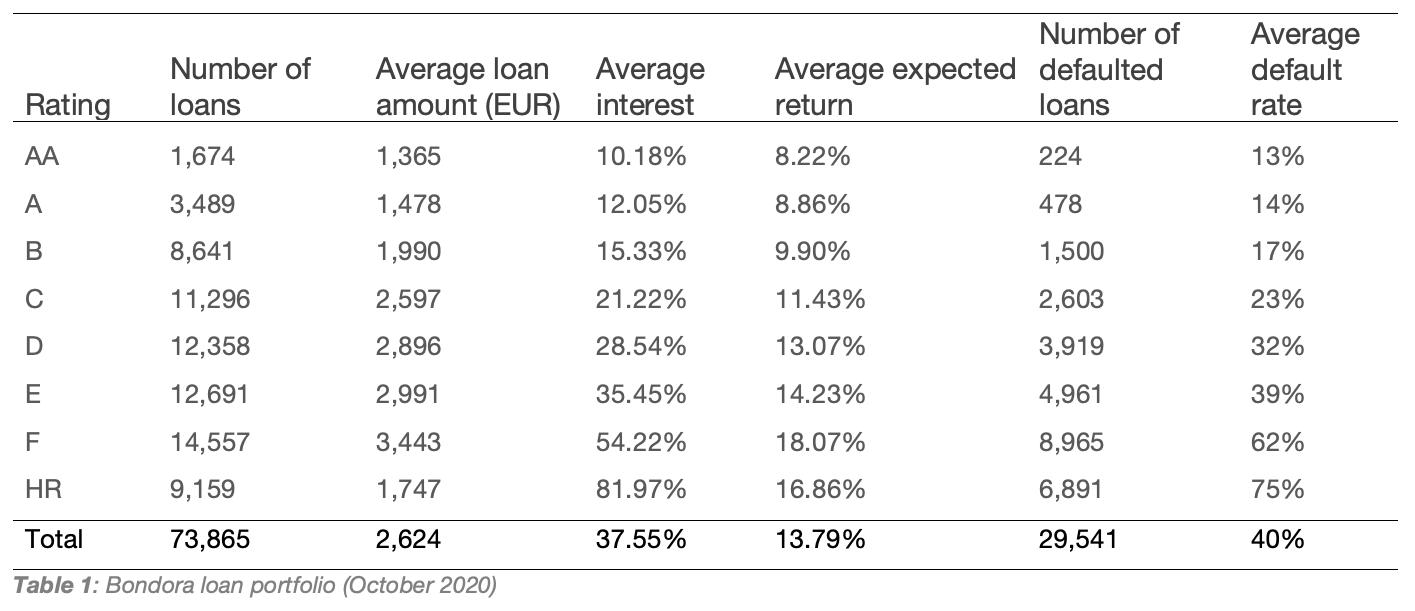
7 minute read
function 4: monitoring and information processing
Banks play a very important role in reducing the information asymmetry between borrowers and investors. It is more cost-effective for the intermediary to gather information, and its own exposure is an indication of the debtor’s creditworthiness (Leland and Pyle, 1977). The sophisticated rating models and the available information, such as the customer’s account history, reduce information asymmetry.
Platforms also analyze customers’ sociodemographic and income data, although they have less access to the information of previous financial behavior. However, soft information voluntarily provided by customers can be an alternative source.
Advertisement
Online intermediaries usually started as technology start-ups, so they rely heavily on innovative digital solutions such as machine learning or artificial intelligence. These solutions can improve the process of gathering and processing information, and thus the efficiency of the credit assessment.
What then is the role of P2P lenders?
Table 1 below summarizes the main characteristics of the loan portfolio of Bondora as of October 2020. The majority of the loans are rated C or worse and the average default rate is 40%, which is extremely high. The default frequency is in line with the ex-ante rating of applications. Our ongoing research (with Ferenc Illés and Tímea Ölvedi) on the performance of P2P lending shows that ratings are based more on hard information, with no convincing evidence that platforms can benefit from alternative information and reduce information asymmetry more than banks.
The loan amounts are small on average, and the interest rates are high, making P2P loans similar to loan shark creditors.
In the above-mentioned research, we also investigate the performance of P2P investments and how investors benefit from P2P participation. We found that although the default frequency is high, a huge fraction of the defaulted loans are recoverable later and fewer than 10% of investors realize a negative rate of return.
Based on the above, peer-to-peer lending is not comparable to bank lending, as such high default probabilities are unacceptable for traditional financial institutions due to the high capital burden and reputational risk. Thus, peer-to-peer platforms seem to operate in the part of the credit market which banks avoid. It could be argued that platforms serve as a legal alternative to loan sharks and allow financial inclusion of the bank-ineligible segment. On the other hand, this inclusion costs an interest rate of about 40% on average and leads to a default rate of up to 75%. Di Maggio and Yao (2021) found that borrowers on P2P platforms became more indebted, counting their original bank loans, shortly after origination.
References
De Roure, C., Pelizzon, L., & Tasca, P. (2016). How does P2P lending fit into the consumer credit market?
Di Maggio, M., & Yao, V. (2021). FinTech borrowers: Lax screening or cream-skimming? The Review of Financial Studies, 34(10), 4565-4618.
Dömötör, Barbara Mária, and Tímea Ölvedi. “A személyközi hitelezés létjogosultsága a pénzügyi közvetítésben.” peer-reviewed by
Közgazdasági Szemle 68, no. 7-8 (2021): 773-793.
Dömötör, B., & Ölvedi, T. (2021). The Financial Intermediary Role of Peer-To-Peer Lenders. In Innovations in Social Finance (pp. 391-413). Palgrave Macmillan, Cham.
Freixas, X., & Rochet, J. C. (2008). Microeconomics of banking. MIT Press.
Jagtiani, J., and Lemieux, C. (2018). Do fintech lenders penetrate areas that are underserved by traditional banks? Journal of Economics and Business, June 2018.
Leland, H. E., & Pyle, D. H. (1977). Informational asymmetries, financial structure, and financial intermediation. The Journal of Finance, 32(2), 371-387.
Carl Densem
Author
Barbara Dömötör
Barbara Dömötör is an Associate Professor of the Department of Finance at Corvinus University of Budapest. She received her PhD in 2014 for her thesis modelling corporate hedging behavior. Her research interest focuses on financial markets, financial risk management and financial regulation. She is the regional director of the Hungarian Chapter of Professional Risk Managers’ International Association.
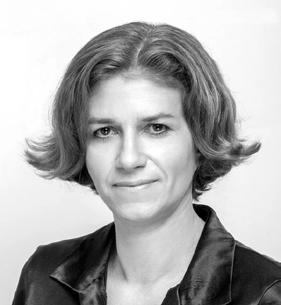
Synopsis
The rise of machine learning algorithms, especially in credit scoring and bankruptcy prediction, has overshadowed traditional methods of late and, simultaneously, raised challenges and attracted academic research. From this emerging body of research comes best practices and guardrails that encourage better use of ML algorithms and awareness of their downsides.
machine learning and credit risk: challenges and insights
by Dr. Nawaf Almaskati
The vast proliferation of machine learning (ML) applications in recent decades due to the surge in available computing power as well as the introduction of several new ML algorithms and their relative commercial success in tasks such as credit scoring and bankruptcy prediction have contributed to the growth of various research strands that look at ML applications in the field. ML algorithms have been popular in the field of bankruptcy prediction and credit scoring, showing superior classification performance compared to classic methods such as logistic regression and discriminant analysis1 .
The popularity of ML algorithms in this field arises from the fact that they tend to perform better in imbalanced and smaller datasets which is an important advantage in bankruptcy and credit scoring applications 2. Results from current research and applications of ML to bankruptcy and credit scoring suggest that the selection of the estimation method is as important as the selection of the input variables in determining the performance of credit and bankruptcy prediction models. Barboza et al. (2017) find that using ML algorithms instead of traditional models lead to an average improvement in the classification performance by 10%.
challenges
1 / The classification performance is a statistical concept that measures the accuracy (actual versus forecast) of the model when assigning the different cases to the different groups (e.g., bankrupt versus non-bankrupt).
2 / Imbalanced datasets are sets where some groups are much smaller than others as is the case with bankrupt versus non-bankrupt firms, where the former group is much smaller than the latter. Classic methods such as logistic regression and discriminant analysis do not perform well when applied to such datasets due to the lack of sufficient observations in one group.
This challenge, and other related questions regarding the required complexity and potential applicability or suitability of the increasing number of available ML algorithms, makes the task of applying ML algorithms to real life problems both interesting and difficult.
For instance, should one forgo the relative simplicity and structure offered by standard regression models in favour of the more complex and ‘black-box like’ algorithms such as neural networks or gradient boosting? Also, are there always additional benefits from adding more complexity to the estimation process through utilizing more complex models or combining estimations from multiple ML algorithms? Perhaps the simplest answer to these questions is that there is no clear rule on what one should do and that, like each dataset or situation is different, each ML algorithm is different too.
Therefore, the job of any ML user is to use the available tools and metrics to find the ML algorithm that provides the best answer to the question being researched while keeping an eye on any algorithm’s pitfalls or shortcomings. In their no free lunch theorem, Wolpert and Macready (1995) state that if algorithm A beats algorithm B in some cases, then it is likely that there is a similar number of cases where algorithm B beats algorithm A, which suggests that there is ‘no free lunch’ or ‘one-size-fits-all’ when it comes to applying ML algorithms.
Insights And Trends
There are a few observations and trends that arise from current ML applications.
First, while the majority of past applications have focused on analysing and comparing the performance of individual ML algorithms, several studies have advocated using a hybrid approach where an ML algorithm is used for feature selection followed by another ML algorithm to addresses the classification or regression problem. Advocates of this approach argue that using an ML algorithm to select the most important features of the input variables makes the model more robust and mitigates the issue of overfitting3. This approach also reduces the time and computing power needed to run the model and allows for the inclusion of more input variables.
Second, current research also appears to suggest employing recommendations from multiple ML algorithms rather than a single one (Tsai et al., 2004; West, 2000; Zhang et al., 1999). This approach is similar to relying on a group of analysts with different approaches (i.e. algorithms) instead of making decisions based on the recommendation of one analyst. The approach allows the production of more robust estimates and reduces volatility in the final recommendation.
Third, ML algorithms also allow the analysis of text-based sources such as management discussion and analysis (MD&A) and other regular disclosures which brings more value and insights into the prediction process. The systematic analysis of MD&As using ML algorithms will allow more robust capturing of management’s sentiment and views about the company’s performance, industry trends and financial health.
Last, more research needs to be done on the ability of ML algorithms to detect the impact of ‘black-swan’ events on credit conditions. Most of the research done so far focuses on the performance of ML algorithms across time with no attention being given to the fact that the performance of such algorithms may change significantly during periods of high stress or unforeseen events.
Concluding Remarks
While ML techniques bring numerous opportunities to the credit risk field, there are still several difficulties with their implementation in practice. First, discussion regarding the period and frequency (e.g. monthly, quarterly, annually, etc.) to be used for the calibration of the model is still inconclusive. Second, concerns about the ‘black-box’ nature of many of the ML algorithms which prevent proper auditing of the calibration process may continue to pose challenges to the wider utilization of such algorithms in the field. Last, but not least, some of the algorithms may require massive computing power to run, especially when using a large dataset or a large number of inputs. This puts restrictions on the ability to recalibrate the model frequently in order to incorporate changes in the underlying patterns or trends.
References
Barboza, F., Kimura, H., & Altman, E. (2017). Machine learning models and bankruptcy prediction. Expert Systems with Applications, 83, 405-417.
Tsai, C.-F., Hsu, Y.-F., & Yen, D. C. (2014). A comparative study of classifier ensembles for bankruptcy prediction. Applied Soft Computing, 24, 977-984.
West, D. (2000). Neural network credit scoring models. Computers & Operations Research, 27(11), 1131-1152.
Wolpert, David H. and Macready, William G., (1995), No Free Lunch Theorems for Search, Working Papers, Santa Fe Institute.
Zhang, G., Y. Hu, M., Eddy Patuwo, B., & C. Indro, D. (1999). Artificial neural networks in bankruptcy prediction: General framework and cross-validation analysis. European Journal of Operational Research, 116(1), 16-32.
peer-reviewed by
Dan diBartolomeo, Carl Densem
Author
Nawaf Almaskati
Dr. Nawaf Almaskati is an active researcher in machine learning and artificial intelligence, financial markets and ESG with several high impact publications and working papers in the field. Dr. Almaskati’s current research focuses on utilizing machine learning and advanced econometrics methods to address the challenges facing the financial industry.
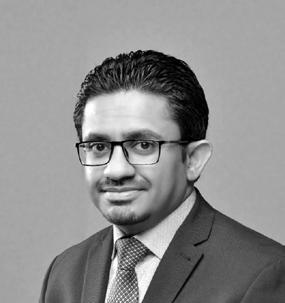
Projects geared at climate change and energy risk must undergo clear and precise project risk management to attain their stated goals. By capturing individual risk categories in a stage-wise manner and treating these independently with established methods, risk management can better assure shareholder value and project completion. This important step cannot be overlooked.