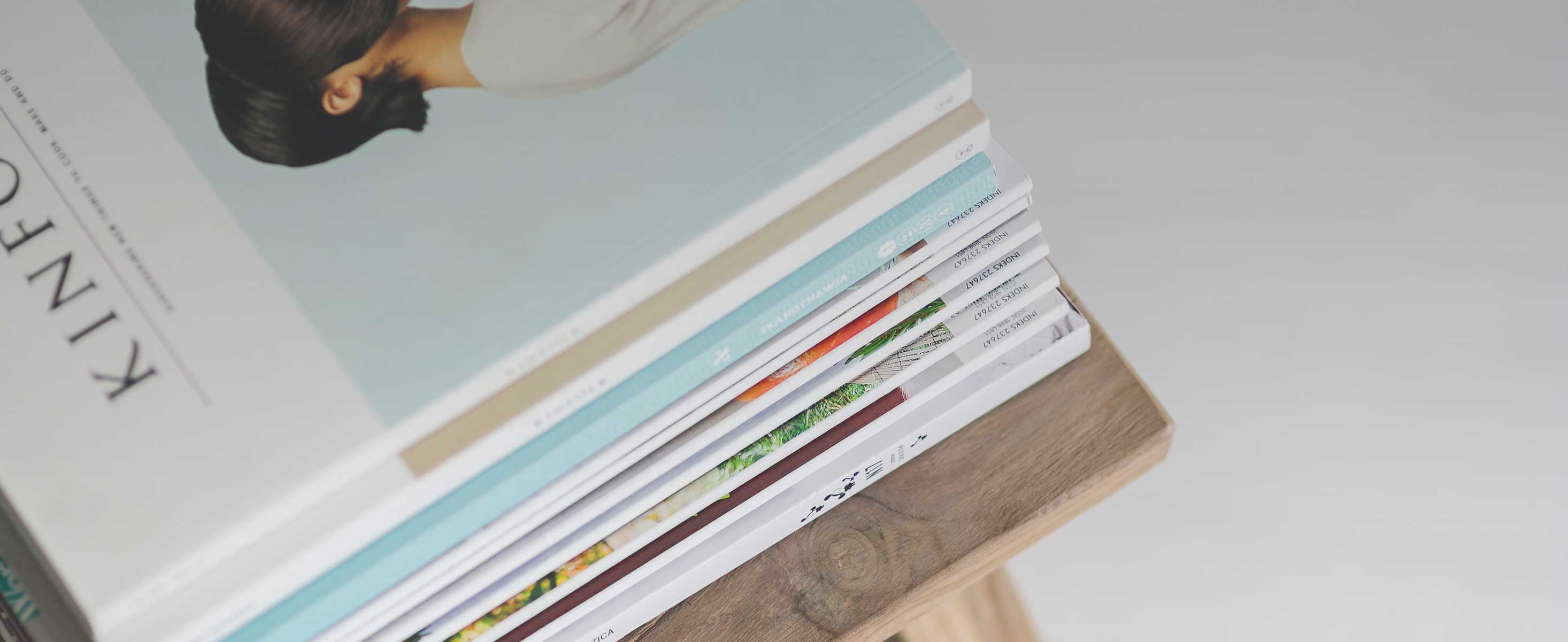
2 minute read
More Fleets Are Turning to Machine Learning to Improve Driver Safety
According to IBM, machine learning “is a branch of artificial intelligence (AI) and computer science which focuses on the use of data and algorithms to imitate the way that humans learn, gradually improving its accuracy.”
Although it is a branch of AI, machine learning is not the same thing. In a recent webinar sponsored by the Truckload Carriers Association, Brian Filip, chief technology officer with insurance company Idelic, explained the difference.
Advertisement
“People often conflate or use those terms in the same way,” he said. “They’re absolutely not the same thing.” AI uses machines to do things that humans would normally do, such as charting a course on a map and then giving step by step directions: “Siri, give me directions to…”
Machine learning, on the other hand, is technology that learns by itself. For the trucking industry, machine learning is a game changer. Many fleets are already employing machine learning though they may not know it.
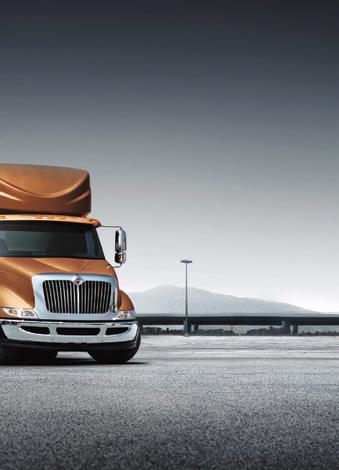
One such machine learning technology monitors driving performance and creates a risk profile for each driver in a fleet. The key to using machine learning is to have all the systems used by truckers communicate with each other.
“You’re probably running a separate system for ELDs, for telematics, for cameras,” Filip said, “and getting them to talk to one another doesn’t usually happen, so you spend an inordinate amount of time going through all these different places.”
Machine learning software, such as those produced by Idelic and other companies, can collate data and present it to decision makers who can act on that data.
On its website, Idelic says, “Through the combination of process and technology, Idelic helps fleets predict and mitigate driver risk, reduce preventable accidents, and lower losses.”
Managers can use machine learning to identify high-risk drivers, which enables a fleet to provide targeted coaching to that driver, according to TForce Freight’s director of health and safety, Scott Reagan.
“We’re able to identify those drivers … helping everyone— the operation, the local leadership, the driver,” Reagan said, adding, “We need to get ahead of it instead of talking after the crash,” said Reagan.
Machine learning software can compile a driver scorecard which can point out faults but can also identify good driving. Reagan said, TForce drivers “all of a sudden wanted that information. We saw that risk behaviors start to diminish.”
Michael Lasko, a former driver who is now Director of Environmental Health and Safety at Boyle Transportation said adopting new technology can assist in retention efforts as long as drivers are included during every step of the process. Technology used at Boyle is beta-tested by company drivers.
The use of machine learning, however, is time consuming. The benefits may not be seen immediately but will occur over time.
“You can’t draw conclusions on what happens in an hour, or a day, or even a week,” Filip said, because crashes are statistically rare, and carriers can’t look at that granular of a level of data. “You have to measure these things over the course of months, quarters, and even years to really see whether or not you’re having a statistically significant impact on outcomes.”