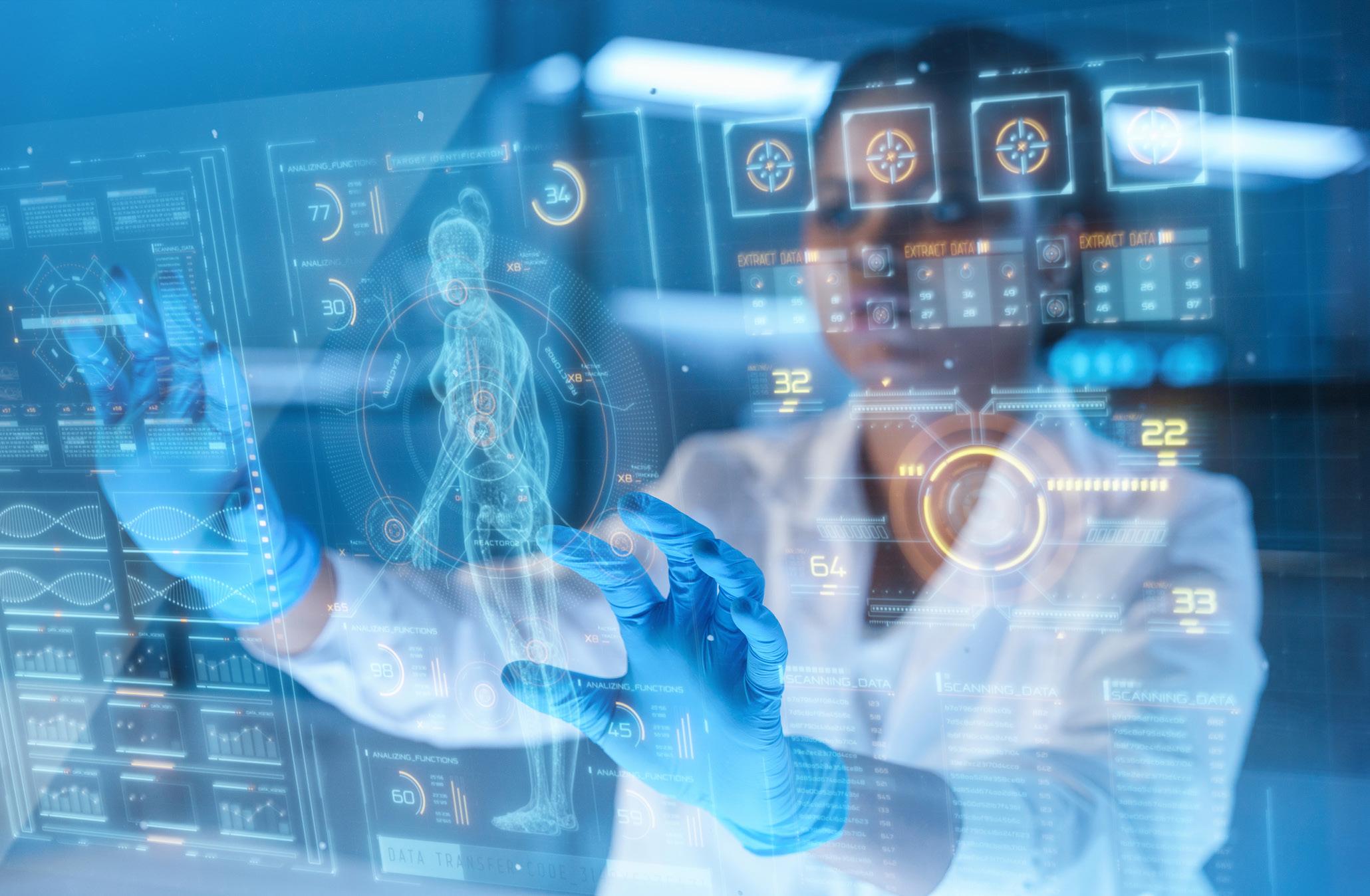
6 minute read
Transforming Emergency Medicine: Highlights From an Expert Panel on AI and Machine Learning
By Kirsten Douglass, MD, Nicholas Stark, MD, MBA, Jonathan Oskvarek, MD, MBA, and Zaid Altawil, MD on behalf of the SAEM Innovation Interest Group
Artificial intelligence (AI) and machine learning (ML) have emerged as powerful tools in transforming emergency medicine. These technologies offer promising applications that enhance decision-making, improve patient outcomes, and optimize resource allocation. In the realm of triage, AI algorithms can analyze patient data such as vital signs, symptoms, and medical history to accurately prioritize cases based on urgency. Machine learning models trained on large datasets enable the early identification of critical conditions, such as sepsis, by recognizing patterns and alerting medical staff. AI-powered imaging analysis plays a vital role in radiology, assisting in the rapid detection of abnormalities in images, thereby expediting diagnosis and treatment.
That introduction sounds pretty good, right? In full disclosure: the above paragraph was generated by ChatGPT when prompted to “write a paragraph about artificial intelligence and machine learning applications in emergency medicine.”
At SAEM23 in Austin, Texas, the SAEM Innovation Interest Group partnered with the SAEM Informatics
& Data Science Interest Group and the Emergency Medicine Innovation Collaborative to host a panel titled “Innovating in Acute Care: Artificial Intelligence and Machine Learning.” Throughout the discussion, two emergency physicians on the cutting edge of artificial intelligence and machine learning — Drs. Christian Rose and David Kim, both of Stanford Emergency Medicine — explored the current and potential future applications of these technologies. What follows is an explorative summary of their discussion.
What are artificial intelligence and machine learning?
Artificial intelligence (AI) is a broad field of computer science that focuses on creating intelligent systems capable of performing tasks that typically require human intelligence. It involves developing algorithms and models that enable machines to perceive and understand their environment, reason, make decisions, and learn from experience.
Machine learning (ML) is a subset of AI focused on the development of models that allow computers to learn and improve from data without being explicitly programmed with specific rules. Instead, ML algorithms are designed to analyze large amounts of data, identify patterns, and make predictions or take actions. ML algorithms are trained on large datasets, which help them recognize patterns and make informed decisions or predictions when presented with new data. ML is a key component of many AI systems, as it allows computers to adapt and improve their performance over time.
(In the spirit of AI and ML, the content of the above section was developed using assistance from ChatGPT)
Do you think AI will become better than emergency physicians in certain domains, such as predicting outcomes or conversing with patients?
AI may be able to help assist emergency physicians (EPs) by removing tasks that currently take up a significant amount of bandwidth on shift. Drs. Kim and Rose hope that AI will become better than humans at certain menial tasks, because this could free EPs to do more “human” work.
One of the causes of burnout is that there is more care to be delivered than we can supply. Aspects that EPs often find fulfilling about our jobs, such as talking with patients, are being cut shorter by the need for us to complete other tasks. For example, the fact that after interviewing a patient we must go and write down everything that just happened, is relatively redundant. Dr. Kim is hopeful that AI may be able to help us reduce these redundancies in our shifts and thus hopefully reduce burnout overall.
What sort of tasks in acute care may be taken over by AI?
Emergency medicine (EM) is more multifaceted than most other medical specialties, so our field may not be as susceptible to dramatic shifts from AI/ML; however, there is no shortage of things to do in the emergency department (ED) and AI may be able to help us with tasks like translating discharge instructions to another language or interpreting X-rays.
Drs. Kim and Rose hope that AI continues to evolve to assist with certain primary care and clinic abilities. One example would be replying to patient inbox messages, which would allow outpatient clinicians to spend more time performing the fulfilling and human aspects of medicine.
What kinds of patient data could be utilized by AI and ML in emergency medicine?
One of the things that makes EM unique is that data obtained can be dispersed over broad time scales. For example, EPs can gather information from chart review that would raise concern for nonaccidental trauma (NAT) or abuse, such as a suspicious number of traumatic injuries relative to a time course. EPs can do this (and are often expected to), but they do not always have the time to comb through every prior visit. A computer model could gather this information, then nudge the EP to investigate this further.
Patient monitors in the ED generate another vast dataset that an AI program could leverage. A patient is often continuously hooked up to a monitor, but much of that data is discarded when the vital signs are only charted once per hour. There is often not a practical way to search this data, so it is difficult for the EP to make use of this potentially valuable information.
Is incorporating AI and ML a good/safe idea for patient care?
What dangers can come from this?
As AI and ML become smoother through improving predictive analytics, the more invisible they become. One of the dangers is that in invisible systems, one may not always know where a piece of information or recommendation is coming from. We must question how to critically appraise a clinical recommendation. One of the worries is that if recommendations become too smooth, we will stop being critical of them. We are always doing potentially dangerous things to patients in the ED (think invasive procedures and potent medications) and must constantly question whether we are making the right decisions in patient care. Dr. Rose cautions that we must learn how any continued on Page 39

Innovation
continued from Page 37 machine-learning algorithm comes to a specific conclusion.
What sort of bias can be present in these models? How can we detect and prevent it?
Bias in these models threatens to undermine their usefulness and reinforce disparities between patients. Incomplete data sets are common, and elements of missing data may affect certain patient populations (especially in underrepresented patients). There is often a tradeoff between data set size and data quality. We must define who we are trying to reach and what gaps new data sets are trying to fill.
Some patient groups may get worse predictions from a model. For example, an insurance company may use health care expenditures to estimate the health care needs of a patient. However, disadvantaged populations may get worse care in this model, by assuming that those that sought out less care in the past have fewer health care needs. Sometimes the sickest patients are those who have not sought medical care in years, so low health care utilization does not necessarily equate to better health.
How might AI and ML change the testing that we perform?
Any time that AI and ML are going to be implemented, we must choose the outcome of interest. If the aim is “zeromiss” or 100% sensitivity, then it will result in more testing. Ultimately AI and ML can help like any tool can; it is just critical that we thoughtfully define its goals.
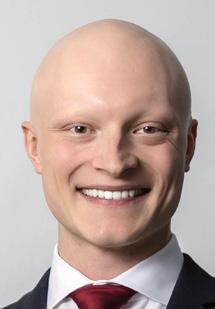
For example, imagine that AI becomes proficient at reading noncontrast head CT scans. We may get to a point where AI reads head CTs independently of radiologists, and then the physician compares the two reads. If AI’s goal is zero-miss, it will ultimately generate more false positives, which the radiologist could review to see if there is something that a human missed or if it is an artifact that AI flagged as abnormal.
In summary, while the exact applications of AI and ML in emergency medicine are uncertain, the future holds great potential for positive impacts if approached thoughtfully and intentionally.
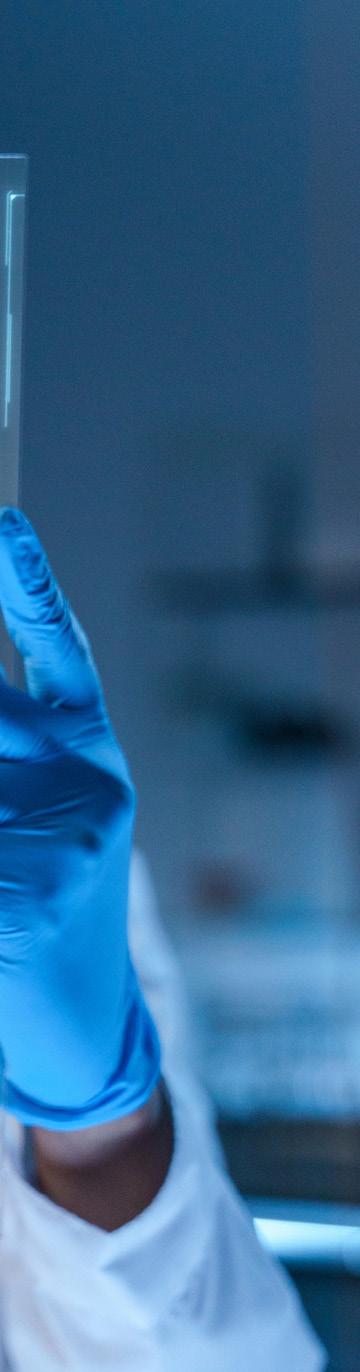
About The Authors
Dr. Douglass is beginning her fourth year of emergency medicine residency at the University of California, San Francisco (UCSF). She graduated from University of California, Davis School of Medicine.
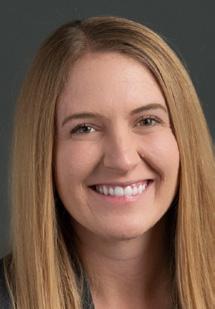
Dr. Stark is an emergency physician who works clinically at the University of California, San Francisco (UCSF) and is the vice chair of emergency medicine and director of operations at Mercy Medical Center. He is the cofounder of the national nonprofit EM Innovation Collaborative and the assistant director of the UCSF Acute Care Innovation Center. @NickStarkMD
Dr. Oskvarek is an emergency physician with US Acute Care Solutions and research co-director at Summa Health in Akron, Ohio. Jonathan has received grants to evaluate a pilot emergency department advanced alternative payment model. He also conducts research in clinician performance improvement and works with entrepreneurs.
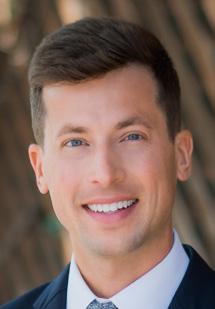
Dr. Altawil is an emergency medicine attending physician at Lawrence General Hospital in Massachusetts. He is co-founder of the Emergency Medicine Innovation Collaborative, a group of emergency medicine physicians working to cultivate collaborations, share resources, and inspire innovative change. He completed residency in emergency medicine at Boston Medical Center.
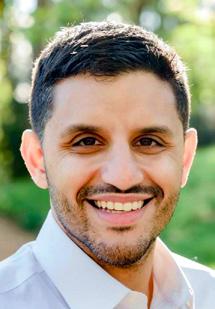