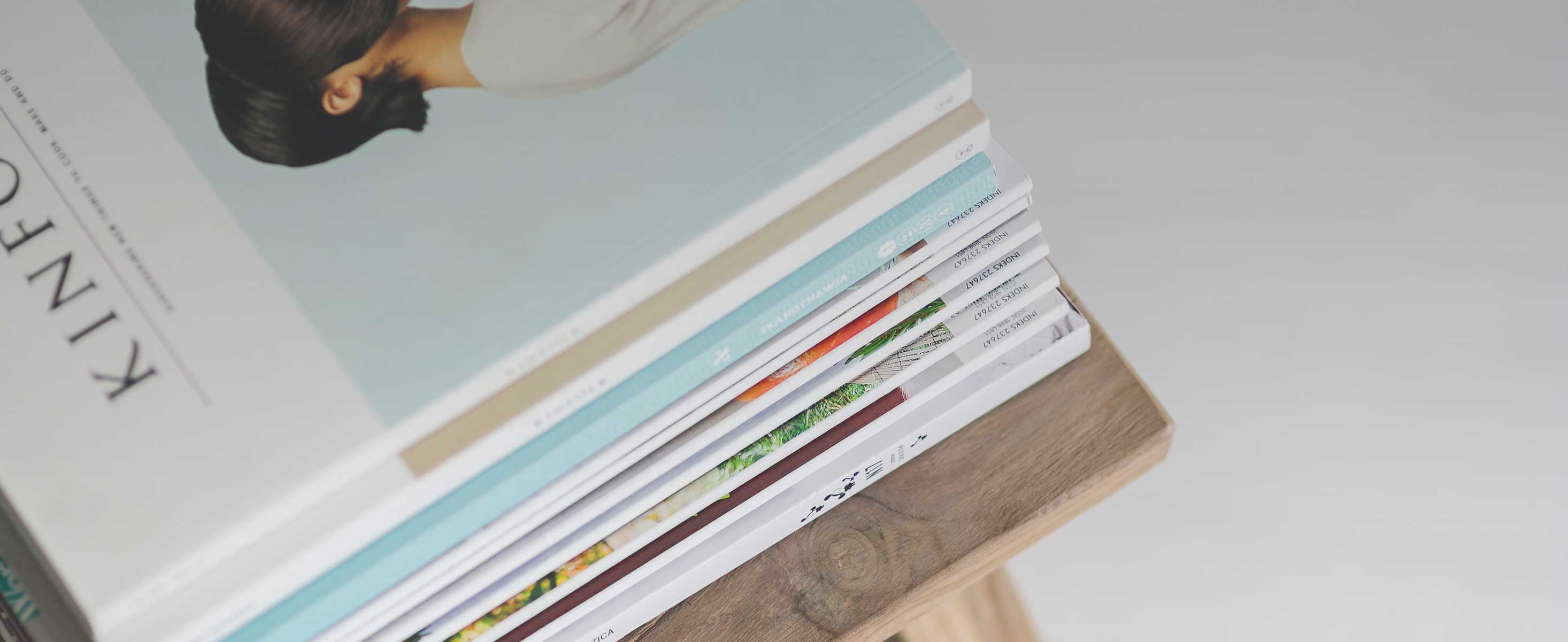
2 minute read
Invited Presentations
Clustering movement patterns and game styles: applications in biomechanics and performance analysis
Peter Lamb 1
Advertisement
1 School of Physical Education, Sport and Exercise Sciences, University of Otago The analysis of human movement and performance is often complicated by the multivariate data that represent them. Analyses are further complicated by the potentially, non-linear relationships among many of the variables in the data sample. Self-organising maps (SOM), a machine learning approach, are useful for clustering and visualising multivariate data, while preserving non-linear relationships in the data distribution, which makes them attractive for studying human behaviour from many perspectives. My research groups have applied SOMs to many sub-disciplines within sport and exercise science –in particular biomechanics and performance analysis. We have used SOMs in biomechanics most recently as a method for classifying back pain, based on patients' movement patterns. The results have led to a more fine-grained distinction between pain groups than is achieved through conventional pain provocation tests. In rugby and netball performance analysis, we have clustered match data to represent 'game styles' for real-time assessment of the coupling between a team's game style and that of their opponent. Further applications of SOMs in other sub-disciplines of sport and exercise science are discussed.
Examination of the Validity and Efficacy of GPS Generated Metabolic Load Measures.
Smith, T.B 1,2 , Tarrant, O.P 3 , McIntosh, N. 1
1 Faculty of Health, Sport and Human Performance, University of Waikato, New Zealand 2 Chiefs Rugby Club, New Zealand, 3 Dept of Physical Education and Sport Sciences, University of Limerick, Ireland
GPS determined speed and acceleration measures are common locomotion metrics in team sports. Rugby union typically consists of repeated high intensity sprints of 10-20m which isn’t long enough to reach high speeds and the acceleration “count” metric doesn’t accurately reflect workload as power output increase with acceleration duration. To measure the combined locomotion workload of acceleration, deceleration and velocity, some GPS companies include a high metabolic workload (HML) metric. We examined the relationship between HML from the STATSports Viper GPS (Courtney Hill, NI) and the time taken to complete common rugby specific intensive interval tests (rugbytests) for twenty participants. HML was set at ≥25 w/kg which is equivalent to ≥5.5 m/s and ≥±2 m/s/s. The validity of real-time HML was assessed during five matches and six training sessions. A near perfect relationship was generated in the comparison of HML against rugby tests. Realtime measurement error was large for matches and small for training. Traditional GPS measures of running distance above a specific intensive speed or the count of intensive accelerations doesn’t adequately reflect running workload in rugby union. HML has the capacity to better monitor intensive running workload to help inform training interventions and monitor injury risk.